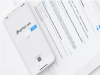
Alzheimer's disease: experimental models and reality.
Acta neuropathologica
Experimental models of Alzheimer's disease (AD) are critical to gaining a better understanding of pathogenesis and to assess the potential of novel therapeutic approaches. The most commonly used experimental animal models are transgenic mice that overexpress human genes associated with familial AD (FAD) that result in the formation of amyloid plaques. However, AD is defined by the presence and interplay of both amyloid plaques and neurofibrillary tangle pathology. The track record of success in AD clinical trials thus far has been very poor. In part, this high failure rate has been related to the premature translation of highly successful results in animal models that mirror only limited aspects of AD pathology to humans. A greater understanding of the strengths and weakness of each of the various models and the use of more than one model to evaluate potential therapies would help enhance the success of therapy translation from preclinical studies to patients. In this review, we summarize the pathological features and limitations of the major experimental models of AD, including transgenic mice, transgenic rats, various physiological models of sporadic AD and in vitro human cell culture models.
10.1007/s00401-016-1662-x
Big Data Application in Biomedical Research and Health Care: A Literature Review.
Biomedical informatics insights
Big data technologies are increasingly used for biomedical and health-care informatics research. Large amounts of biological and clinical data have been generated and collected at an unprecedented speed and scale. For example, the new generation of sequencing technologies enables the processing of billions of DNA sequence data per day, and the application of electronic health records (EHRs) is documenting large amounts of patient data. The cost of acquiring and analyzing biomedical data is expected to decrease dramatically with the help of technology upgrades, such as the emergence of new sequencing machines, the development of novel hardware and software for parallel computing, and the extensive expansion of EHRs. Big data applications present new opportunities to discover new knowledge and create novel methods to improve the quality of health care. The application of big data in health care is a fast-growing field, with many new discoveries and methodologies published in the last five years. In this paper, we review and discuss big data application in four major biomedical subdisciplines: (1) bioinformatics, (2) clinical informatics, (3) imaging informatics, and (4) public health informatics. Specifically, in bioinformatics, high-throughput experiments facilitate the research of new genome-wide association studies of diseases, and with clinical informatics, the clinical field benefits from the vast amount of collected patient data for making intelligent decisions. Imaging informatics is now more rapidly integrated with cloud platforms to share medical image data and workflows, and public health informatics leverages big data techniques for predicting and monitoring infectious disease outbreaks, such as Ebola. In this paper, we review the recent progress and breakthroughs of big data applications in these health-care domains and summarize the challenges, gaps, and opportunities to improve and advance big data applications in health care.
10.4137/BII.S31559