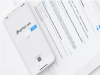
Radiation pneumonitis and fibrosis: mechanisms underlying its pathogenesis and implications for future research.
Tsoutsou Pelagia G,Koukourakis Michael I
International journal of radiation oncology, biology, physics
Radiation pneumonitis and subsequent radiation pulmonary fibrosis are the two main dose-limiting factors when irradiating the thorax that can have severe implications for patients' quality of life. In this article, the current concepts about the pathogenetic mechanisms underlying radiation pneumonitis and fibrosis are presented. The clinical course of fibrosis, a postulated acute inflammatory stage, and a late fibrotic and irreversible stage are discussed. The interplay of cells and the wide variety of molecules orchestrating the immunologic response to radiation, their interactions with specific receptors, and the cascade of events they trigger are elucidated. Finally, the implications of this knowledge with respect to the therapeutic interventions are critically presented.
10.1016/j.ijrobp.2006.08.058
Computed tomography and radiation dose images-based deep-learning model for predicting radiation pneumonitis in lung cancer patients after radiation therapy.
Radiotherapy and oncology : journal of the European Society for Therapeutic Radiology and Oncology
PURPOSE:To develop a deep learning model that combines CT and radiation dose (RD) images to predict the occurrence of radiation pneumonitis (RP) in lung cancer patients who received radical (chemo)radiotherapy. METHODS:CT, RD images and clinical parameters were obtained from 314 retrospectively-collected patients (training set) and 35 prospectively-collected patients (test-set-1) who were diagnosed with lung cancer and received radical radiotherapy in the dose range of 50 Gy and 70 Gy. Another 194 (60 Gy group, test-set-2) and 158 (74 Gy group, test-set-3) patients from the clinical trial RTOG 0617 were used for external validation. A ResNet architecture was used to develop a prediction model that combines CT and RD features. Thereafter, the CT and RD weights were adjusted by using 40 patients from test-set-2 or 3 to accommodate cohorts with different clinical settings or dose delivery patterns. Visual interpretation was implemented using a gradient-weighted class activation map (grad-CAM) to observe the area of model attention during the prediction process. To improve the usability, ready-to-use online software was developed. RESULTS:The discriminative ability of a baseline trained model had an AUC of 0.83 for test-set-1, 0.55 for test-set-2, and 0.63 for test-set-3. After adjusting CT and RD weights of the model using a subset of the RTOG-0617 subjects, the discriminatory power of test-set-2 and 3 improved to AUC 0.65 and AUC 0.70, respectively. Grad-CAM showed the regions of interest to the model that contribute to the prediction of RP. CONCLUSION:A novel deep learning approach combining CT and RD images can effectively and accurately predict the occurrence of RP, and this model can be adjusted easily to fit new cohorts.
10.1016/j.radonc.2023.109581
Oral administration of herbal medicines for radiation pneumonitis in lung cancer patients: A systematic review and meta-analysis.
PloS one
BACKGROUND:Radiation pneumonitis is a common and serious complication of radiotherapy. Many published randomized controlled studies (RCTs) reveal a growing trend of using herbal medicines as adjuvant therapy to prevent radiation pneumonitis; however, their efficacy and safety remain unexplored. OBJECTIVE:The aim of this systematic review is to evaluate the efficacy and safety of herbal medicines as adjunctive therapy for the prevention of radiation pneumonitis in patients with lung cancer who undergo radiotherapy. METHODS:We searched the following 11 databases: three English medical databases [MEDLINE (PubMed), EMBASE, The Cochrane Central Register of Controlled Trials (CENTRAL)], five Korean medical databases (Korean Studies Information, Research information Service System, KoreaMed, DBPIA, National Digital Science Library), and three Chinese medical databases [the China National Knowledge Database (CNKI), Journal Integration Platform (VIP), and WanFang Database]. The primary outcome was the incidence of radiation pneumonitis. The risk of bias was assessed using the Cochrane risk-of-bias tool. RESULTS:Twenty-two RCTs involving 1819 participants were included. The methodological quality was poor for most of the studies. Meta-analysis showed that herbal medicines combined with radiotherapy significantly reduced the incidence of radiation pneumonitis (n = 1819; RR 0.53, 95% CI 0.45-0.63, I2 = 8%) and the incidence of severe radiation pneumonitis (n = 903; RR 0.22, 95% CI 0.11-0.41, I2 = 0%). Combined therapy also improved the Karnofsky performance score (n = 420; WMD 4.62, 95% CI 1.05-8.18, I2 = 82%). CONCLUSION:There is some encouraging evidence that oral administration of herbal medicines combined with radiotherapy may benefit patients with lung cancer by preventing or minimizing radiation pneumonitis. However, due to the poor methodological quality of the identified studies, definitive conclusion could not be drawn. To confirm the merits of this approach, further rigorously designed large scale trials are warranted.
10.1371/journal.pone.0198015
The role of procalcitonin in differential diagnosis between acute radiation pneumonitis and bacterial pneumonia in lung cancer patients receiving thoracic radiotherapy.
Wang Zhiwu,Huo Bingjie,Wu Qiong,Dong Liang,Fu Haoyu,Wang Shuo,Zhang Jing
Scientific reports
Acute Radiation Pneumonitis (ARP) is one of the most common dose-limiting toxicities of thoracic radiotherapy. The accurate diagnosis of ARP remains a challenge because of the lack of a rapid biomarker capable of differentiating ARP from bacterial pneumo (BP). The aim of this study was to investigate the potential usefulness of procalcitonin (PCT) in the differential diagnosis of ARP and BP. Lung cancer patients who had undergone thoracic radiotherapy within 6 months and were admitted to hospital for ARP or BP were retrospectively analyzed. The serum levels of PCT, C-reactive protein (CRP) and white blood cells (WBC) were compared between the two groups. Receiver operating characteristic (ROC) curve was used to assess the diagnostic value of PCT, CRP and WBC in the differential diagnosis of ARP and BP and determine the best cut-off values. One hundred eighteen patients were included. Among them, seventy-seven patients were diagnosed with ARP, and 41 patients were diagnosed with BP. The PCT concentrations for patients diagnosed with ARP group were significantly lower than those in the BP group (P < 0.001). There were no differences in CRP and WBC between the two groups. The areas under the ROC curves (AUC) for PCT, CRP and WBC were 0.745, 0.589 and 0.578, respectively. The best cutoff values of PCT, CRP and WBC were 0.47 μg/L, 54.5 mg/L and 9.9 × 10/L, respectively. Low serum PCT levels are associated with ARP. PCT is a useful biomarker to distinguish ARP from BP.
10.1038/s41598-020-60063-w
A Primer on Dose-Response Data Modeling in Radiation Therapy.
International journal of radiation oncology, biology, physics
An overview of common approaches used to assess a dose response for radiation therapy-associated endpoints is presented, using lung toxicity data sets analyzed as a part of the High Dose per Fraction, Hypofractionated Treatment Effects in the Clinic effort as an example. Each component presented (eg, data-driven analysis, dose-response analysis, and calculating uncertainties on model prediction) is addressed using established approaches. Specifically, the maximum likelihood method was used to calculate best parameter values of the commonly used logistic model, the profile-likelihood to calculate confidence intervals on model parameters, and the likelihood ratio to determine whether the observed data fit is statistically significant. The bootstrap method was used to calculate confidence intervals for model predictions. Correlated behavior of model parameters and implication for interpreting dose response are discussed.
10.1016/j.ijrobp.2020.11.020
Inhibition of TGFβ1 activation prevents radiation-induced lung fibrosis.
Clinical and translational medicine
BACKGROUND:Radiotherapy is the main treatment modality for thoracic tumours, but it may induce pulmonary fibrosis. Currently, the pathogenesis of radiation-induced pulmonary fibrosis (RIPF) is unclear, and effective treatments are lacking. Transforming growth factor beta 1 (TGFβ1) plays a central role in RIPF. We found that activated TGFβ1 had better performance for radiation pneumonitis (RP) risk prediction by detecting activated and total TGFβ1 levels in patient serum. αv integrin plays key roles in TGFβ1 activation, but the role of αv integrin-mediated TGFβ1 activation in RIPF is unclear. Here, we investigated the role of αv integrin-mediated TGFβ1 activation in RIPF and the application of the integrin antagonist cilengitide to prevent RIPF. METHODS:Itgav ;Pdgfrb-Cre mice were generated by conditionally knocking out Itgav in myofibroblasts, and wild-type mice were treated with cilengitide or placebo. All mice received 16 Gy of radiation or underwent a sham radiation procedure. Lung fibrosis was measured by a modified Ashcroft score and microcomputed tomography (CT). An enzyme-linked immunosorbent assay (ELISA) was used to measure the serum TGFβ1 concentration, and total Smad2/3 and p-Smad2/3 levels were determined via Western blotting. RESULTS:Conditional Itgav knockout significantly attenuated RIPF (p < .01). Hounsfield units (HUs) in the lungs were reduced in the knockout mice compared with the control mice (p < .001). Conditional Itgav knockout decreased active TGFβ1 secretion and inhibited fibroblast p-Smad2/3 expression. Exogenous active TGFβ1, but not latent TGFβ1, reversed these reductions. Furthermore, cilengitide treatment elicited similar results and prevented RIPF. CONCLUSIONS:The present study revealed that conditional Itgav knockout and cilengitide treatment both significantly attenuated RIPF in mice by inhibiting αv integrin-mediated TGFβ1 activation. HIGHLIGHTS:Activated TGFβ1 has a superior capacity in predicting radiation pneumonitis (RP) risk and plays a vital role in the development of radiation-induced pulmonary fibrosis (RIPF). Conditional knock out Itgav in myofibroblasts prevented mice from developing RIPF. Cilengitide alleviated the development of RIPF by inhibiting αv integrin-mediated TGFβ1 activation and may be used in targeted approaches for preventing RIPF.
10.1002/ctm2.1546
Pharmacologic ACE-Inhibition Mitigates Radiation-Induced Pneumonitis by Suppressing ACE-Expressing Lung Myeloid Cells.
International journal of radiation oncology, biology, physics
PURPOSE:Radiation-induced lung injury is a major dose-limiting toxicity for thoracic radiation therapy patients. In experimental models, treatment with angiotensin converting enzyme (ACE) inhibitors mitigates radiation pneumonitis; however, the mechanism of action is not well understood. Here, we evaluate the direct role of ACE inhibition on lung immune cells. METHODS AND MATERIALS:ACE expression and activity were determined in the lung immune cell compartment of irradiated adult rats after either high dose fractionated radiation therapy to the right lung (5 fractions × 9 Gy) or a single dose of 13.5 Gy partial body irradiation. Mitigation of radiation-induced pneumonitis with the ACE-inhibitor lisinopril was evaluated in the 13.5 Gy rat partial body irradiation model. During pneumonitis, we characterized inflammation and immune cell content in the lungs and bronchoalveolar lavage fluid. In vitro mechanistic studies were performed using primary human monocytes and the human monocytic THP-1 cell line. RESULTS:In both the partial body irradiation and fractionated radiation therapy models, radiation increased ACE activity in lung immune cells. Treatment with lisinopril improved survival during radiation pneumonitis (P = .0004). Lisinopril abrogated radiation-induced increases in bronchoalveolar lavage fluid monocyte chemoattractant protein 1 (chemokine ligand 2) and MIP-1a cytokine levels (P < .0001). Treatment with lisinopril reduced both ACE expression (P = .006) and frequency of CD45 CD11b lung myeloid cells (P = .004). In vitro, radiation injury acutely increased ACE activity (P = .045) and reactive oxygen species (ROS) generation (P = .004) in human monocytes, whereas treatment with lisinopril blocked radiation-induced increases in both ACE and ROS. Radiation-induced ROS generation was blocked by pharmacologic inhibition of either NADPH oxidase 2 (P = .012) or the type 1 angiotensin receptor (P = .013). CONCLUSIONS:These data demonstrate radiation-induced ACE activation within the immune compartment promotes the pathogenesis of radiation pneumonitis, while ACE inhibition suppresses activation of proinflammatory immune cell subsets. Mechanistically, our in vitro data demonstrate radiation directly activates the ACE/type 1 angiotensin receptor pathway in immune cells and promotes generation of ROS via NADPH oxidase 2.
10.1016/j.ijrobp.2022.01.023
Effects of diabetes on the development of radiation pneumonitis.
Dong Guangtong,Li Yuxiao,Zhao Qiyao,Pang Bing,Qi Xin,Wei Junping,Hou Wei
Respiratory research
Radiation pneumonia (RP) is a common adverse reaction to radiation therapy in patients with chest tumors. Recent studies have shown that diabetes mellitus (DM), which can cause systemic multisystem damage, specifically targets lungs, and the incidence of RP in patients with a history of diabetes is higher than that in other patients with tumors who have undergone radiotherapy. DM is an important risk factor for RP in tumor patients undergoing RT, and patients with DM should be treated with caution. This article reviews research on the clinical aspects, as well as the mechanism, of the effects of diabetes on RP and suggests future research needed to reduce RP.
10.1186/s12931-021-01754-4
Radiomics and Dosiomics Signature From Whole Lung Predicts Radiation Pneumonitis: A Model Development Study With Prospective External Validation and Decision-curve Analysis.
International journal of radiation oncology, biology, physics
PURPOSE:Radiation pneumonitis (RP) is one of the common side effects of radiation therapy in the thoracic region. Radiomics and dosiomics quantify information implicit within medical images and radiation therapy dose distributions. In this study we demonstrate the prognostic potential of radiomics, dosiomics, and clinical features for RP prediction. METHODS AND MATERIALS:Radiomics, dosiomics, dose-volume histogram (DVH) metrics, and clinical parameters were obtained on 314 retrospectively collected and 35 prospectively enrolled patients diagnosed with lung cancer between 2013 to 2019. A radiomics risk score (R score) and dosiomics risk score (D score), as well as a DVH-score, were calculated based on logistic regression after feature selection. Six models were built using different combinations of R score, D score, DVH score, and clinical parameters to evaluate their added prognostic power. Overoptimism was evaluated by bootstrap resampling from the training set, and the prospectively collected cohort was used as the external test set. Model calibration and decision-curve characteristics of the best-performing models were evaluated. For ease of further evaluation, nomograms were constructed for selected models. RESULTS:A model built by integrating all of the R score, D score, and clinical parameters had the best discriminative ability with areas under the curve of 0.793 (95% confidence interval [CI], 0.735-0.851), 0.774 (95% CI, 0.762-0.786), and 0.855 (95% CI, 0.719-0.990) in the training, bootstrapping, and external test sets, respectively. The calibration curve image showed good agreement between the predicted and actual values, with a slope of 1.21 and intercept of -0.04. The decision curve image showed a positive net benefit for the final model based on the nomogram. CONCLUSIONS:Radiomic and dosiomic features have the potential to assist with the prediction of RP, and the combination of radiomics, dosiomics, and clinical parameters led to the best prognostic model in the present study.
10.1016/j.ijrobp.2022.08.047
Re-evaluating the risk factors for radiation pneumonitis in the era of immunotherapy.
Journal of translational medicine
As one of the common complications of radiotherapy, radiation pneumonia (RP) limits the prognosis of patients. Therefore, better identifying the high-risk factors that lead to RP is essential to effectively prevent its occurrence. However, as lung cancer treatment modalities are being replaced and the era of immunotherapy has arrived, literature that reviews the parameters and mode of radiotherapy, chemotherapy drugs, targeted drugs and current hot immune checkpoint inhibitors related to RP is lacking. This paper summarizes the risk factors for radiation pneumonia by retrieving and analysing previously published literature and the results of large clinical trials. The literature primarily included retrospective analyses, including clinical trials in different periods and a part of the literature review. A systematic literature search of Embase, PubMed, Web of Science, and Clinicaltrials.gov was performed for relevant publications up to 6 Dec. 2022. Search keywords include, but are not limited to, "radiation pneumonia", "pneumonia", "risk factors", "immunotherapy", etc. The factors related to RP in this paper include physical parameters of radiotherapy, including V, V, and MLD; chemoradiotherapy mode and chemotherapy drugs, including paclitaxel and gemcitabine; EGFR-TKI; ALK inhibitors; antiangiogenic drugs; immune drugs and the underlying disease of the patient. We also introduce the possible mechanism of RP. In the future, we hope that this article not only sounds the alarm for clinicians but also helps to identify a method that can effectively intervene and reduce the occurrence of RP, significantly improve the quality of life and prognosis of patients, and more effectively improve the therapeutic effect of radiation therapy.
10.1186/s12967-023-04212-5
Radiation-Induced Lung Injury: Assessment and Management.
Chest
Radiation-induced lung injury (RILI) encompasses any lung toxicity induced by radiation therapy (RT) and manifests acutely as radiation pneumonitis and chronically as radiation pulmonary fibrosis. Because most patients with thoracic and breast malignancies are expected to undergo RT in their lifetime, many with curative intent, the population at risk is significant. Furthermore, indications for thoracic RT are expanding given the advent of stereotactic body radiation therapy (SBRT) or stereotactic ablative radiotherapy (SABR) for early-stage lung cancer in nonsurgical candidates as well as oligometastatic pulmonary disease from any solid tumor. Fortunately, the incidence of serious pulmonary complications from RT has decreased secondary to advances in radiation delivery techniques. Understanding the temporal relationship between RT and injury as well as the patient, disease, and radiation factors that help distinguish RILI from other etiologies is necessary to prevent misdiagnosis. Although treatment of acute pneumonitis is dependent on clinical severity and typically responds completely to corticosteroids, accurately diagnosing and identifying patients who may progress to fibrosis is challenging. Current research advances include high-precision radiation techniques, an improved understanding of the molecular basis of RILI, the development of small and large animal models, and the identification of candidate drugs for prevention and treatment.
10.1016/j.chest.2019.03.033
Radiation pneumonia predictive model for radiotherapy in esophageal carcinoma patients.
BMC cancer
BACKGROUND:The machine learning models with dose factors and the deep learning models with dose distribution matrix have been used to building lung toxics models for radiotherapy and achieve promising results. However, few studies have integrated clinical features into deep learning models. This study aimed to explore the role of three-dimension dose distribution and clinical features in predicting radiation pneumonitis (RP) in esophageal cancer patients after radiotherapy and designed a new hybrid deep learning network to predict the incidence of RP. METHODS:A total of 105 esophageal cancer patients previously treated with radiotherapy were enrolled in this study. The three-dimension (3D) dose distributions within the lung were extracted from the treatment planning system, converted into 3D matrixes and used as inputs to predict RP with ResNet. In total, 15 clinical factors were normalized and converted into one-dimension (1D) matrixes. A new prediction model (HybridNet) was then built based on a hybrid deep learning network, which combined 3D ResNet18 and 1D convolution layers. Machine learning-based prediction models, which use the traditional dosiomic factors with and without the clinical factors as inputs, were also constructed and their predictive performance compared with that of HybridNet using tenfold cross validation. Accuracy and area under the receiver operator characteristic curve (AUC) were used to evaluate the model effect. DeLong test was used to compare the prediction results of the models. RESULTS:The deep learning-based model achieved superior prediction results compared with machine learning-based models. ResNet performed best in the group that only considered dose factors (accuracy, 0.78 ± 0.05; AUC, 0.82 ± 0.25), whereas HybridNet performed best in the group that considered both dose factors and clinical factors (accuracy, 0.85 ± 0.13; AUC, 0.91 ± 0.09). HybridNet had higher accuracy than that of Resnet (p = 0.009). CONCLUSION:Based on prediction results, the proposed HybridNet model could predict RP in esophageal cancer patients after radiotherapy with significantly higher accuracy, suggesting its potential as a useful tool for clinical decision-making. This study demonstrated that the information in dose distribution is worth further exploration, and combining multiple types of features contributes to predict radiotherapy response.
10.1186/s12885-023-11499-6