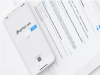
Automated detection of dental restorations using deep learning on panoramic radiographs.
Dento maxillo facial radiology
OBJECTIVES:Automatically detecting dental conditions using Artificial intelligence (AI) and reporting it visually are now a need for treatment planning and dental health management. This work presents a comprehensive computer-aided detection system to detect dental restorations. METHODS:The state-of-art ten different deep-learning detection models were used including R-CNN, Faster R-CNN, SSD, YOLOv3, and RetinaNet as detectors. ResNet-50, ResNet-101, XCeption-101, VGG16, and DarkNet53 were integrated as backbone and feature extractor in addition to efficient approaches such Side-Aware Boundary Localization, cascaded structures and simple model frameworks like Libra and Dynamic.Total 684 objects in panoramic radiographs were used to detect available three classes, namely, dental restorations, denture and implant.Each model was evaluated by mean average precision (mAP), average recall (AR), and precision-recall curve using Common Objects in Context (COCO) detection evaluation metrics. RESULTS:mAP varied between 0.755 and 0.973 for ten models explored while AR ranges between 0.605 and 0.771. Faster R-CNN RegnetX provided the best detection performance with mAP of 0.973 and AR of 0.771. Area under precision-recall curve was 0.952. Precision-recall curve indicated that errors were mainly dominated by localization confusions. CONCLUSIONS:Results showed that the proposed AI-based computer-aided system had great potential with reliable, accurate performance detecting dental restorations, denture and implant in panoramic radiographs. As training models with more data and standardization in reporting, AI-based solutions will be implemented to dental clinics for daily use soon.
10.1259/dmfr.20220244
A deep learning approach to dental restoration classification from bitewing and periapical radiographs.
Karatas Ozcan,Cakir Nazire Nurdan,Ozsariyildiz Saban Suat,Kis Hatice Cansu,Demirbuga Sezer,Gurgan Cem Abdulkadir
Quintessence international (Berlin, Germany : 1985)
OBJECTIVE:The aim of this study was to examine the success of deep learning-based convolutional neural networks (CNN) in the detection and differentiation of amalgam, composite resin, and metal-ceramic restorations from bitewing and periapical radiographs. METHOD AND MATERIALS:Five hundred and fifty bitewing and periapical radiographs were used. Eighty percent of the images were used for training, and 20% were left for testing. Twenty percent of the images allocated for training were then used for validation during learning. The image classification model was based on the application of CNN. The model used Resnet34 architecture, which is pre-trained on the ImageNet dataset. Average sensitivity, receiver operating characteristic (ROC) curve, and area under the curve (AUC) were calculated for performance evaluation of the model. RESULTS:The model training loss was 0.13, and the validation loss was 0.63. The independent test group result was 0.67. Amalgam AUC was 0.95, composite AUC was 0.95, and metal-ceramic AUC was 1.00. The average AUC was 0.97. The false positive rate in the validation set was 18, the false negative rate was 18, the true positive rate was 60, and the true negative rate was 138. The true positive rate was 0.82 for amalgam, 0.75 for composite, and 0.73 for metal-ceramic. CONCLUSION:Deep learning-based CNNs from periapical and bitewing radiographs appear to be a promising technique for the detection and differentiation of restorations.
10.3290/j.qi.b1244461
Automatic detection of teeth and dental treatment patterns on dental panoramic radiographs using deep neural networks.
Forensic sciences research
Disaster victim identification issues are especially critical and urgent after a large-scale disaster. The aim of this study was to suggest an automatic detection of natural teeth and dental treatment patterns based on dental panoramic radiographs (DPRs) using deep learning to promote its applicability as human identifiers. A total of 1 638 DPRs, of which the chronological age ranged from 20 to 49 years old, were collected from January 2000 to November 2020. This dataset consisted of natural teeth, prostheses, teeth with root canal treatment, and implants. The detection of natural teeth and dental treatment patterns including the identification of teeth number was done with a pre-trained object detection network which was a convolutional neural network modified by EfficientDet-D3. The objective metrics for the average precision were 99.1% for natural teeth, 80.6% for prostheses, 81.2% for treated root canals, and 96.8% for implants, respectively. The values for the average recall were 99.6%, 84.3%, 89.2%, and 98.1%, in the same order, respectively. This study showed outstanding performance of convolutional neural network using dental panoramic radiographs in automatically identifying teeth number and detecting natural teeth, prostheses, treated root canals, and implants.
10.1080/20961790.2022.2034714
Segmentation of Dental Restorations on Panoramic Radiographs Using Deep Learning.
Diagnostics (Basel, Switzerland)
Convolutional Neural Networks (CNNs) such as U-Net have been widely used for medical image segmentation. Dental restorations are prominent features of dental radiographs. Applying U-Net on the panoramic image is challenging, as the shape, size and frequency of different restoration types vary. We hypothesized that models trained on smaller, equally spaced rectangular image crops (tiles) of the panoramic would outperform models trained on the full image. A total of 1781 panoramic radiographs were annotated pixelwise for fillings, crowns, and root canal fillings by dental experts. We used different numbers of tiles for our experiments. Five-times-repeated three-fold cross-validation was used for model evaluation. Training with more tiles improved model performance and accelerated convergence. The F1-score for the full panoramic image was 0.7, compared to 0.83, 0.92 and 0.95 for 6, 10 and 20 tiles, respectively. For root canals fillings, which are small, cone-shaped features that appear less frequently on the radiographs, the performance improvement was even higher (+294%). Training on tiles and pooling the results thereafter improved pixelwise classification performance and reduced the time to model convergence for segmenting dental restorations. Segmentation of panoramic radiographs is biased towards more frequent and extended classes. Tiling may help to overcome this bias and increase accuracy.
10.3390/diagnostics12061316
Extended Arm of Precision in Prosthodontics: Artificial Intelligence.
Cureus
Dentistry based on artificial intelligence (AI) is not a myth but turning into a reality. AI has revolutionized medicine and dentistry in various ways. AI is a technology that uses machines to imitate intelligent human behavior. AI is gaining popularity worldwide because of its significant impact and breakthrough in the field of intelligence innovation. It is a lifesaver in dentistry, particularly in the field of prosthodontics, because it aids in the design of prostheses and the fabrication of functional maxillofacial appliances. It also helps in the processes of patient documentation, diagnosis, treatment planning, and patient management, allowing oral healthcare professionals to work smarter rather than harder. While it cannot replace the work of a dentist because dentistry is not about disease diagnosis, it does involve correlation with other clinical findings and provides treatment to the patient. The integration of AI and digitization has brought a new paradigm in dentistry, with extremely promising prospects. The availability of insufficient and inaccurate data is now the only barrier to the deployment of AI. Therefore, dentists and clinicians must focus on collecting and entering authentic data into their database, which will be completely utilized for AI in dentistry shortly. This study focuses on various applications of AI in prosthodontics along with its limitations and future scope.
10.7759/cureus.30962
Artificial intelligence in prosthodontics.
Bioinformation
Artificial Intelligence (AI) is gaining popularity worldwide owing to its significant impact in science and innovation. The role of AI in prosthodontics has increased significantly in recent years. AI is used for diagnosis, decision-making, prognosis, treatment planning and prediction of outcomes. Integration of AI into prosthodontics can enhance the accuracy and precision of dental practice. However, limited datasets are a major constraint in its practical applications.
10.6026/973206300200238
The Potential of Artificial Intelligence in Prosthodontics: A Comprehensive Review.
Medical science monitor : international medical journal of experimental and clinical research
Prosthodontics is a dental subspecialty that includes the preparation of dental prosthetics for missing or damaged teeth. It increasingly uses computer-assisted technologies for planning and preparing dental prosthetics. This study aims to present the findings from a systematic review of publications on artificial intelligence (AI) in prosthodontics to identify current trends and future opportunities. The review question was "What are the applications of AI in prosthodontics and how good is their performance in prosthodontics?" Electronic searching in the Web of Science, ScienceDirect, PubMed, and Cochrane Library was conducted. The search was limited to full text from January 2012 to January 2024. Quadas-2 was used for assessing quality and potential risk of bias for the selected studies. A total of 1925 studies were identified in the initial search. After removing the duplicates and applying exclusion criteria, a total of 30 studies were selected for this review. Results of the Quadas-2 assessment of included studies found that a total of 18.3% of studies were identified as low risk of bias studies, whereas 52.6% and 28.9% of included studies were identified as studies with high and unclear risk of bias, respectively. Although they are still developing, AI models have already shown promise in the areas of dental charting, tooth shade selection, automated restoration design, mapping the preparation finishing line, manufacturing casting optimization, predicting facial changes in patients wearing removable prostheses, and designing removable partial dentures.
10.12659/MSM.944310
The Use and Performance of Artificial Intelligence in Prosthodontics: A Systematic Review.
Bernauer Selina A,Zitzmann Nicola U,Joda Tim
Sensors (Basel, Switzerland)
(1) Background: The rapid pace of digital development in everyday life is also reflected in dentistry, including the emergence of the first systems based on artificial intelligence (AI). This systematic review focused on the recent scientific literature and provides an overview of the application of AI in the dental discipline of prosthodontics. (2) Method: According to a modified PICO-strategy, an electronic (MEDLINE, EMBASE, CENTRAL) and manual search up to 30 June 2021 was carried out for the literature published in the last five years reporting the use of AI in the field of prosthodontics. (3) Results: 560 titles were screened, of which 30 abstracts and 16 full texts were selected for further review. Seven studies met the inclusion criteria and were analyzed. Most of the identified studies reported the training and application of an AI system ( = 6) or explored the function of an intrinsic AI system in a CAD software ( = 1). (4) Conclusions: While the number of included studies reporting the use of AI was relatively low, the summary of the obtained findings by the included studies represents the latest AI developments in prosthodontics demonstrating its application for automated diagnostics, as a predictive measure, and as a classification or identification tool. In the future, AI technologies will likely be used for collecting, processing, and organizing patient-related datasets to provide patient-centered, individualized dental treatment.
10.3390/s21196628
Computer-aided design and 3-dimensional artificial/convolutional neural network for digital partial dental crown synthesis and validation.
Scientific reports
The current multiphase, invitro study developed and validated a 3-dimensional convolutional neural network (3D-CNN) to generate partial dental crowns (PDC) for use in restorative dentistry. The effectiveness of desktop laser and intraoral scanners in generating data for the purpose of 3D-CNN was first evaluated (phase 1). There were no significant differences in surface area [t-stat(df) = - 0.01 (10), mean difference = - 0.058, P > 0.99] and volume [t-stat(df) = 0.357(10)]. However, the intraoral scans were chosen for phase 2 as they produced a greater level of volumetric details (343.83 ± 43.52 mm) compared to desktop laser scanning (322.70 ± 40.15 mm). In phase 2, 120 tooth preparations were digitally synthesized from intraoral scans, and two clinicians designed the respective PDCs using computer-aided design (CAD) workflows on a personal computer setup. Statistical comparison by 3-factor ANOVA demonstrated significant differences in surface area (P < 0.001), volume (P < 0.001), and spatial overlap (P < 0.001), and therefore only the most accurate PDCs (n = 30) were picked to train the neural network (Phase 3). The current 3D-CNN produced a validation accuracy of 60%, validation loss of 0.68-0.87, sensitivity of 1.00, precision of 0.50-0.83, and serves as a proof-of-concept that 3D-CNN can predict and generate PDC prostheses in CAD for restorative dentistry.
10.1038/s41598-023-28442-1
A system for designing removable partial dentures using artificial intelligence. Part 1. Classification of partially edentulous arches using a convolutional neural network.
Takahashi Toshihito,Nozaki Kazunori,Gonda Tomoya,Ikebe Kazunori
Journal of prosthodontic research
PURPOSE:The purpose of this study was to develop a method for classifying dental arches using a convolutional neural network (CNN) as the first step in a system for designing removable partial dentures. METHODS:Using 1184 images of dental arches (maxilla: 748 images; mandible: 436 images), arches were classified into four arch types: edentulous, intact dentition, arches with posterior tooth loss, and arches with bounded edentulous space. A CNN method to classify images was developed using Tensorflow and Keras deep learning libraries. After completion of the learning procedure, the diagnostic accuracy, precision, recall, F-measure and area under the curve (AUC) for each jaw were calculated for diagnostic performance of learning. The classification was also predicted using other images, and percentages of correct predictions (PCPs) were calculated. The PCPs were compared with the Kruskal-Wallis test (p = 0.05). RESULTS:The diagnostic accuracy was 99.5% for the maxilla and 99.7% for the mandible. The precision, recall, and F-measure for both jaws were 0.25, 1.0 and 0.4, respectively. The AUC was 0.99 for the maxilla and 0.98 for the mandible. The PCPs of the classifications were more than 95% for all types of dental arch. There were no significant differences among the four types of dental arches in the mandible. CONCLUSIONS:The results of this study suggest that dental arches can be classified and predicted using a CNN. Future development of systems for designing removable partial dentures will be made possible using this and other AI technologies.
10.2186/jpr.JPOR_2019_354
Efficient Computer-Aided Design of Dental Inlay Restoration: A Deep Adversarial Framework.
Tian Sukun,Wang Miaohui,Yuan Fulai,Dai Ning,Sun Yuchun,Xie Wuyuan,Qin Jing
IEEE transactions on medical imaging
Restoring the normal masticatory function of broken teeth is a challenging task primarily due to the defect location and size of a patient's teeth. In recent years, although some representative image-to-image transformation methods (e.g. Pix2Pix) can be potentially applicable to restore the missing crown surface, most of them fail to generate dental inlay surface with realistic crown details (e.g. occlusal groove) that are critical to the restoration of defective teeth with varying shapes. In this article, we design a computer-aided Deep Adversarial-driven dental Inlay reStoration (DAIS) framework to automatically reconstruct a realistic surface for a defective tooth. Specifically, DAIS consists of a Wasserstein generative adversarial network (WGAN) with a specially designed loss measurement, and a new local-global discriminator mechanism. The local discriminator focuses on missing regions to ensure the local consistency of a generated occlusal surface, while the global discriminator aims at defective teeth and adjacent teeth to assess if it is coherent as a whole. Experimental results demonstrate that DAIS is highly efficient to deal with a large area of missing teeth in arbitrary shapes and generate realistic occlusal surface completion. Moreover, the designed watertight inlay prostheses have enough anatomical morphology, thus providing higher clinical applicability compared with more state-of-the-art methods.
10.1109/TMI.2021.3077334
DCPR-GAN: Dental Crown Prosthesis Restoration Using Two-Stage Generative Adversarial Networks.
Tian Sukun,Wang Miaohui,Dai Ning,Ma Haifeng,Li Linlin,Fiorenza Luca,Sun Yuchun,Li Yangmin
IEEE journal of biomedical and health informatics
Restoring the correct masticatory function of broken teeth is the basis of dental crown prosthesis rehabilitation. However, it is a challenging task primarily due to the complex and personalized morphology of the occlusal surface. In this article, we address this problem by designing a new two-stage generative adversarial network (GAN) to reconstruct a dental crown surface in the data-driven perspective. Specifically, in the first stage, a conditional GAN (CGAN) is designed to learn the inherent relationship between the defective tooth and the target crown, which can solve the problem of the occlusal relationship restoration. In the second stage, an improved CGAN is further devised by considering an occlusal groove parsing network (GroNet) and an occlusal fingerprint constraint to enforce the generator to enrich the functional characteristics of the occlusal surface. Experimental results demonstrate that the proposed framework significantly outperforms the state-of-the-art deep learning methods in functional occlusal surface reconstruction using a real-world patient database. Moreover, the standard deviation (SD) and root mean square (RMS) between the generated occlusal surface and the target crown calculated by our method are both less than 0.161 mm. Importantly, the designed dental crown have enough anatomical morphology and higher clinical applicability.
10.1109/JBHI.2021.3119394
Time efficiency, occlusal morphology, and internal fit of anatomic contour crowns designed by dental software powered by generative adversarial network: A comparative study.
Journal of dentistry
OBJECTIVES:To evaluate the time efficiency, occlusal morphology, and internal fit of dental crowns designed using generative adversarial network (GAN)-based dental software compared to conventional dental software. METHODS:Thirty datasets of partial arch scans for prepared posterior teeth were analyzed. Each crown was designed on each abutment using GAN-based software (AI) and conventional dental software (non-AI). The AI and non-AI groups were compared in terms of time efficiency by measuring the elapsed work time. The difference in the occlusal morphology of the crowns before and after design optimization and the internal fit of the crown to the prepared abutment were also evaluated by superimposition for each software. Data were analyzed using independent t tests or Mann-Whitney test with statistical significance (α=.05). RESULTS:The working time was significantly less for the AI group than the non-AI group at T1, T5, and T6 (P≤.043). The working time with AI was significantly shorter at T1, T3, T5, and T6 for the intraoral scan (P≤.036). Only at T2 (P≤.001) did the cast scan show a significant difference between the two groups. The crowns in the AI group showed less deviation in occlusal morphology and significantly better internal fit to the abutment than those in the non-AI group (both P<.001). CONCLUSIONS:Crowns designed by AI software showed improved outcomes than that designed by non-AI software, in terms of time efficiency, difference in occlusal morphology, and internal fit. CLINICAL SIGNIFICANCE:The GAN-based software showed better time efficiency and less deviation in occlusal morphology during the design process than the conventional software, suggesting a higher probability of optimized outcomes of crown design.
10.1016/j.jdent.2023.104739
A data-driven approach for the partial reconstruction of individual human molar teeth using generative deep learning.
Frontiers in artificial intelligence
Background and objective:Due to the high prevalence of dental caries, fixed dental restorations are regularly required to restore compromised teeth or replace missing teeth while retaining function and aesthetic appearance. The fabrication of dental restorations, however, remains challenging due to the complexity of the human masticatory system as well as the unique morphology of each individual dentition. Adaptation and reworking are frequently required during the insertion of fixed dental prostheses (FDPs), which increase cost and treatment time. This article proposes a data-driven approach for the partial reconstruction of occlusal surfaces based on a data set that comprises 92 3D mesh files of full dental crown restorations. Methods:A Generative Adversarial Network (GAN) is considered for the given task in view of its ability to represent extensive data sets in an unsupervised manner with a wide variety of applications. Having demonstrated good capabilities in terms of image quality and training stability, StyleGAN-2 has been chosen as the main network for generating the occlusal surfaces. A 2D projection method is proposed in order to generate 2D representations of the provided 3D tooth data set for integration with the StyleGAN architecture. The reconstruction capabilities of the trained network are demonstrated by means of 4 common inlay types using a Bayesian Image Reconstruction method. This involves pre-processing the data in order to extract the necessary information of the tooth preparations required for the used method as well as the modification of the initial reconstruction loss. Results:The reconstruction process yields satisfactory visual and quantitative results for all preparations with a root mean square error (RMSE) ranging from 0.02 mm to 0.18 mm. When compared against a clinical procedure for CAD inlay fabrication, the group of dentists preferred the GAN-based restorations for 3 of the total 4 inlay geometries. Conclusions:This article shows the effectiveness of the StyleGAN architecture with a downstream optimization process for the reconstruction of 4 different inlay geometries. The independence of the reconstruction process and the initial training of the GAN enables the application of the method for arbitrary inlay geometries without time-consuming retraining of the GAN.
10.3389/frai.2024.1339193
Tooth morphology, internal fit, occlusion and proximal contacts of dental crowns designed by deep learning-based dental software: A comparative study.
Journal of dentistry
OBJECTIVES:This study compared the tooth morphology, internal fit, occlusion, and proximal contacts of dental crowns automatically generated via two deep learning (DL)-based dental software systems with those manually designed by an experienced dental technician using conventional software. METHODS:Thirty partial arch scans of prepared posterior teeth were used. The crowns were designed using two DL-based methods (AA and AD) and a technician-based method (NC). The crown design outcomes were three-dimensionally compared, focusing on tooth morphology, internal fit, occlusion, and proximal contacts, by calculating the geometric relationship. Statistical analysis utilized the independent t-test, Mann-Whitney test, one-way ANOVA, and Kruskal-Wallis test with post hoc pairwise comparisons (α = 0.05). RESULTS:The AA and AD groups, with the NC group as a reference, exhibited no significant tooth morphology discrepancies across entire external or occlusal surfaces. The AD group exhibited higher root mean square and positive average values on the axial surface (P < .05). The AD and NC groups exhibited a better internal fit than the AA group (P < .001). The cusp angles were similar across all groups (P = .065). The NC group yielded more occlusal contact points than the AD group (P = .006). Occlusal and proximal contact intensities varied among the groups (both P < .001). CONCLUSIONS:Crowns designed by using both DL-based software programs exhibited similar morphologies on the occlusal and axial surfaces; however, they differed in internal fit, occlusion, and proximal contacts. Their overall performance was clinically comparable to that of the technician-based method in terms of the internal fit and number of occlusal contact points. CLINICAL SIGNIFICANCE:DL-based dental software for crown design can streamline the digital workflow in restorative dentistry, ensuring clinically-acceptable outcomes on tooth morphology, internal fit, occlusion, and proximal contacts. It can minimize the necessity of additional design optimization by dental technician.
10.1016/j.jdent.2023.104830
Personalized design technique for the dental occlusal surface based on conditional generative adversarial networks.
Yuan Fulai,Dai Ning,Tian Sukun,Zhang Bei,Sun Yuchun,Yu Qing,Liu Hao
International journal for numerical methods in biomedical engineering
The tooth defect is a frequently occurring disease within the field of dental clinic. However, the traditional manual restoration for the defective tooth needs an especially long treatment time, and dental computer aided design and manufacture (CAD/CAM) systems fail to restore the personalized anatomical features of natural teeth. Aiming to address the shortcomings of existed methods, this article proposes an intelligent network model for designing tooth crown surface based on conditional generative adversarial networks. Then, the data set for training the network model is constructed via generating depth maps of 3D tooth models scanned by the intraoral. Through adversarial training, the network model is able to generate tooth occlusal surface under the constraint of the space occlusal relationship, the perceptual loss, and occlusal groove filter loss. Finally, we carry out the assessment experiments for the quality of the occlusal surface and the occlusal relationship with the opposing tooth. The experimental results demonstrate that our method can automatically reconstruct the personalized anatomical features on occlusal surface and shorten the treatment time while restoring the full functionality of the defective tooth.
10.1002/cnm.3321
Morphology and fracture behavior of lithium disilicate dental crowns designed by human and knowledge-based AI.
Journal of the mechanical behavior of biomedical materials
This study aimed to compare the occlusal morphology and fracture behavior of lithium disilicate ceramic dental crowns on 12 human participants' premolar #45 designed by a knowledge-based AI (CEREC, biogeneric individual function, BI) and different human personnel (experienced technician, TD, and trained dental students, AD) using CAD software. Digital datasets of crown design were best-fit aligned with the original teeth to evaluate profile and volume discrepancies of the occlusal morphology, and difference in the functional cuspal angle. Milled and sintered lithium disilicate crowns were resin-luted to 3D-printed dental casts and were subjected to axial load-to-fracture test. The fracture loads and failure modes were recorded and examined. Repeated measures ANOVA with LSD post-hoc test, Kruskal-Wallis test, Pearson's correlation coefficient, paired t-test, and chi-square exact test were used for statistical analyses (α = 0.05). BI-generated crowns showed the highest occlusal profile discrepancy (0.3677 ± 0.0388 mm), whereas human-CAD designed crowns showed higher conformity to the original teeth (0.3254 ± 0.0515 mm for TD, 0.3571 ± 0.0820 for AD; z-difference method; p < 0.001). Cusp angle values were significantly different in all groups except BI and TD (54.76 ± 3.81° for the original teeth, 70.84 ± 4.31° for BI, 67.45 ± 5.30° for TD, and 62.30 ± 7.92° for AD; p < 0.001). Although all three groups of crown designs could achieve clinically acceptable fracture resistance (1556.09 ± 525.68 N for BI, 1486.00 ± 520.08 N for TD, 1425.77 ± 433.34 for AD; p = 0.505) such that no significant difference in fracture strength was found, most crowns presented catastrophic bulk fracture that was not clinically restorable because of the substrate fracture. Group BI had a significantly higher percentage of restorable substrate damage than TD (p = 0.014) and AD (p < 0.001). In conclusion, in designing lithium disilicate dental crown, CAD design with human may be better than knowledge-based AI.
10.1016/j.jmbbm.2022.105256
[Independent innovation research, development and transformation of precise bionic repair technology for oral prosthesis].
Sun Y C,Guo Y Q,Chen H,Deng K H,Li W W
Beijing da xue xue bao. Yi xue ban = Journal of Peking University. Health sciences
According to the fourth national oral health epidemiological survey report (2018), billions of teeth are lost or missing in China, inducing chewing dysfunction, which is necessary to build physiological function using restorations. Digital technology improves the efficiency and accuracy of oral restoration, with the application of three-dimensional scans, computer-aided design (CAD), computer-aided manufacturing (CAM), bionic material design and so on. However, the basic research and product development of digital technology in China lack international competitiveness, with related products basically relying on imports, including denture 3D design software, 3D oral printers, and digitally processed materials. To overcome these difficulties, from 2001, Yuchun Sun's team, from Peking University School and Hospital of Stomatology, developed a series of studies in artificial intelligence design and precision bionics manufacturing of complex oral prostheses. The research included artificial intelligence design technology for complex oral prostheses, 3D printing systems for oral medicine, biomimetic laminated zirconia materials and innovative application of digital prosthetics in clinical practice. The research from 2001 to 2007 was completed under the guidance of Prof. Peijun Lv and Prof. Yong Wang. Under the support of the National Natural Science Foundation of China, the National Science and Technology Support Program, National High-Tech R & D Program (863 Program) and Beijing Training Project for the Leading Talents in S & T, Yuchun Sun's team published over 200 papers in the relevant field, authorized 49 national invention patents and 1 U.S. invention patent and issued 2 national standards. It also developed 8 kinds of core technology products in digital oral prostheses and 3 kinds of clinical diagnosis and treatment programs, which significantly improved the design efficiency of complex oral prostheses, the fabrication accuracy of metal prostheses and the bionic performance of ceramic materials. Compared with similar international technologies, the program doubled the efficiency of bionic design and manufacturing accuracy and reduced the difficulty of diagnosis and cost of treatment and application by 50%, with the key indicators of those products reaching the international leading level. This program not only helped to realize precision, intelligence and efficiency during prostheses but also provided functional and aesthetic matches for patients after prostheses. The program was rewarded with the First Technical Innovation Prize of the Beijing Science and Technology Awards (2020), Gold Medal of Medical Research Group in the First Medical Science and Technology Innovation Competition of National Health Commission of the People's Republic of China (2020) and Best Creative Award in the First Translational Medical Innovation Competition of Capital (2017). This paper is a review of the current situation of artificial intelligence design and precision bionics manufacturing of complex oral prosthesis.
Efficient complete denture metal base design via a dental feature-driven segmentation network.
Computers in biology and medicine
BACKGROUND AND OBJECTIVE:Complete denture is a common restorative treatment in dental patients and the design of the core components (major connector and retentive mesh) of complete denture metal base (CDMB) is the basis of successful restoration. However, the automated design process of CDMB has become a challenging task primarily due to the complexity of manual interaction, low personalization, and low design accuracy. METHODS:To solve the existing problems, we develop a computer-aided Segmentation Network-driven CDMB design framework, called CDMB-SegNet, to automatically generate personalized digital design boundaries for complete dentures of edentulous patients. Specifically, CDMB-SegNet consists of a novel upright-orientation adjustment module (UO-AM), a dental feature-driven segmentation network, and a specific boundary-optimization design module (BO-DM). UO-AM automatically identifies key points for locating spatial attitude of the three-dimensional dental model with arbitrary posture, while BO-DM can result in smoother and more personalized designs for complete denture. In addition, to achieve efficient and accurate feature extraction and segmentation of 3D edentulous models with irregular gingival tissues, the light-weight backbone network is also incorporated into CDMB-SegNet. RESULTS:Experimental results on a large clinical dataset showed that CDMB-SegNet can achieve superior performance over the state-of-the-art methods. Quantitative evaluation (major connector/retentive mesh) showed improved Accuracy (98.54 ± 0.58 %/97.73 ± 0.92 %) and IoU (87.42 ± 5.48 %/70.42 ± 7.95 %), and reduced Maximum Symmetric Surface Distance (4.54 ± 2.06 mm/4.62 ± 1.68 mm), Average Symmetric Surface Distance (1.45 ± 0.63mm/1.28 ± 0.54 mm), Roughness Rate (6.17 ± 1.40 %/6.80 ± 1.23 %) and Vertices Number (23.22 ± 1.85/43.15 ± 2.72). Moreover, CDMB-SegNet shortened the overall design time to around 4 min, which is one tenth of the comparison methods. CONCLUSIONS:CDMB-SegNet is the first intelligent neural network for automatic CDMB design driven by oral big data and dental features. The designed CDMB is able to couple with patient's personalized dental anatomical morphology, providing higher clinical applicability compared with the state-of-the-art methods.
10.1016/j.compbiomed.2024.108550
Evaluating the Accuracy of AI-Based Software vs Human Interpretation in the Diagnosis of Dental Caries Using Intraoral Radiographs: An RCT.
Journal of pharmacy & bioallied sciences
Background:Dental caries is a prevalent oral health issue, often diagnosed through intraoral radiographs. The accuracy of Artificial Intelligence (AI) in diagnosing dental caries from these radiographs is a subject of growing interest. Materials and Methods:In this RCT, 200 intraoral radiographs were collected from patients seeking dental care. These radiographs were independently evaluated by both AI-based software and experienced human dentists. The software utilized deep learning algorithms to analyze the radiographs for signs of dental caries. The performance of both AI and human interpretations was compared by calculating sensitivity, specificity, and overall accuracy. Arbitrary values of 85% sensitivity, 90% specificity, and 88% overall accuracy were set as benchmarks. Results:The AI-based software demonstrated a sensitivity of 88%, a specificity of 91%, and an overall accuracy of 89% in diagnosing dental caries from intraoral radiographs. Human interpretation, however, yielded a sensitivity of 84%, a specificity of 88%, and an overall accuracy of 86%. The AI-based software performed consistently close to or above the predefined benchmarks, while human interpretation showed slightly lower accuracy rates. Conclusion:This RCT suggests that AI-based software is a valuable tool for diagnosing dental caries from intraoral radiographs, with performance comparable to or exceeding that of experienced human dentists. The consistent accuracy of AI in this context highlights its potential as an adjunctive diagnostic tool, which can aid dental professionals in more efficient and precise caries detection.
10.4103/jpbs.jpbs_1029_23
Effects of 3D-Printing Technology and Cement Type on the Fracture Resistance of Permanent Resin Crowns for Primary Teeth.
The International journal of prosthodontics
PURPOSE:To evaluate the fracture resistance of permanent resin crowns for primary teeth produced using two different 3D-printing technologies (digital light processing [DLP] and stereolithography [SLA]) and cemented with various luting cements (glass ionomer, resin-modified glass ionomer, and self-adhesive resin cement), whether thermally aged or not. MATERIALS AND METHODS:A typodont primary mandibular second molar tooth was prepared and scanned, and a restoration design was created with web-based artificial intelligence (AI) dental software. A total of 96 crowns were prepared, and 12 experimental groups were generated according to the cement type, 3Dprinting technology (DLP or SLA), and thermal aging. Fracture resistance values and failure types of the specimens were noted. The results were statistically analyzed with three-way ANOVA and Tukey HSD tests (α = .05). RESULTS:The results of the three-way ANOVA showed that there was an interaction among the factors (3D-printing technology, cement type, and thermal aging) (P = .003). Thermal aging significantly decreased the fracture resistance values in all experimental groups. DLP-printed crowns showed higher fracture resistance values than SLA-printed crowns. Cement type also affected the fracture resistance, with glass ionomer cement showing the lowest values after aging. Resin-modified glass ionomer and resin cements were more preferable for 3D-printed crowns. CONCLUSIONS:The type of cement and the 3D-printing technology significantly influenced the fracture resistance of 3D-printed permanent resin crowns for primary teeth, and it was decided that these crowns would be able to withstand masticatory forces in children.
10.11607ijp.8927
A novel collaborative learning model for mixed dentition and fillings segmentation in panoramic radiographs.
Journal of dentistry
INTRODUCTION:It is critical for dentists to identify and differentiate primary and permanent teeth, fillings, dental restorations and areas with pathological findings when reviewing dental radiographs to ensure that an accurate diagnosis is made and the optimal treatment can be planned. Unfortunately, dental radiographs are sometimes read incorrectly due to human error or low-quality images. While secondary or group review can help catch errors, many dentists work in practice alone and/or do not have time to review all of their patients' radiographs with another dentist. Artificial intelligence may facilitate the accurate interpretation of radiographs. To help support the review of panoramic radiographs, we developed a novel collaborative learning model that simultaneously identifies and differentiates primary and permanent teeth and detects fillings. METHODS:We used publicly accessible dental panoramic radiographic images and images obtained from the University of Missouri-Kansas City, School of Dentistry to develop and optimize two high-performance classifiers: (1) a system for tooth segmentation that can differentiate primary and permanent teeth and (2) a system to detect dental fillings. RESULTS:By utilizing these high-performance classifiers, we created models that can identify primary and permanent teeth (mean average precision [mAP] 95.32 % and performance [F-1] 92.50 %), as well as their associated dental fillings (mAP 91.53 % and F-1 91.00 %). We also designed a novel method for collaborative learning that utilizes these two classifiers to enhance recognition performance (mAP 94.09 % and F-1 93.41 %). CONCLUSIONS:Our model improves upon the existing machine learning models to simultaneously identify and differentiate primary and permanent teeth, and to identify any associated fillings. CLINICAL SIGNIFICANCE:Human error can lead to incorrect readings of panoramic radiographs. By developing artificial intelligence and machine learning methods to analyze panoramic radiographs, dentists can use this information to support their radiograph interpretations, help communicate the information to patients, and assist dental students learning to read radiographs.
10.1016/j.jdent.2023.104779
Machine Learning Analysis of Microtensile Bond Strength of Dental Adhesives.
Journal of dental research
Dental adhesives provide retention to composite fillings in dental restorations. Microtensile bond strength (µTBS) test is the most used laboratory test to evaluate bonding performance of dental adhesives. The traditional approach for developing dental adhesives involves repetitive laboratory measurements, which consumes enormous time and resources. Machine learning (ML) is a promising tool for accelerating this process. This study aimed to develop ML models to predict the µTBS of dental adhesives using their chemical features and to identify important contributing factors for µTBS. Specifically, the chemical composition and µTBS information of 81 dental adhesives were collected from the manufacturers and the literature. The average µTBS value of each adhesive was labeled as either 0 (if <36 MPa) or 1 (if ≥36 MPa) to denote the low and high µTBS classes. The initial 9-feature data set comprised pH, HEMA, BisGMA, UDMA, MDP, PENTA, filler, fluoride, and organic solvent (OS) as input features. Nine ML algorithms, including logistic regression, k-nearest neighbor, support vector machine, decision trees and tree-based ensembles, and multilayer perceptron, were implemented for model development. Feature importance analysis identified MDP, pH, OS, and HEMA as the top 4 contributing features, which were used to construct a 4-feature data set. Grid search with stratified 10-fold cross-validation (CV) was employed for hyperparameter tunning and model performance evaluation using 2 metrics, the area under the receiver operating characteristic curve (AUC) and accuracy. The 4-feature data set generated slightly better performance than the 9-feature data set, with the highest AUC score of 0.90 and accuracy of 0.81 based on stratified CV. In conclusion, ML is an effective tool for predicting dental adhesives with low and high µTBS values and for identifying important chemical features contributing to the µTBS. The ML-based data-driven approach has great potential to accelerate the discovery of new dental adhesives and other dental materials.
10.1177/00220345231175868
Morphology and mechanical performance of dental crown designed by 3D-DCGAN.
Dental materials : official publication of the Academy of Dental Materials
OBJECTIVES:This study utilised an Artificial Intelligence (AI) method, namely 3D-Deep Convolutional Generative Adversarial Network (3D-DCGAN), which is one of the true 3D machine learning methods, as an automatic algorithm to design a dental crown. METHODS:Six hundred sets of digital casts containing mandibular second premolars and their adjacent and antagonist teeth obtained from healthy personnel were machine-learned using 3D-DCGAN. Additional 12 sets of data were used as the test dataset, whereas the natural second premolars in the test dataset were compared with the designs in (1) 3D-DCGAN, (2) CEREC Biogeneric, and (3) CAD for morphological parameters of 3D similarity, cusp angle, occlusal contact point number and area, and in silico fatigue simulations with finite element (FE) using lithium disilicate material. RESULTS:The 3D-DCGAN design and natural teeth had the lowest discrepancy in morphology compared with the other groups (root mean square value = 0.3611). The Biogeneric design showed a significantly (p < 0.05) higher cusp angle (67.11°) than that of the 3D-DCGAN design (49.43°) and natural tooth (54.05°). No significant difference was observed in the number and area of occlusal contact points among the four groups. FE analysis showed that the 3D-DCGAN design had the best match to the natural tooth regarding the stress distribution in the crown. The 3D-DCGAN design was subjected to 26.73 MPa and the natural tooth was subjected to 23.97 MPa stress at the central fossa area under physiological occlusal force (300 N); the two groups showed similar fatigue lifetimes (F-N curve) under simulated cyclic loading of 100-400 N. Designs with Biogeneric or technician would yield respectively higher or lower fatigue lifetime than natural teeth. SIGNIFICANCE:This study demonstrated that 3D-DCGAN could be utilised to design personalised dental crowns with high accuracy that can mimic both the morphology and biomechanics of natural teeth.
10.1016/j.dental.2023.02.001
Precise tooth design using deep learning-based templates.
Journal of dentistry
OBJECTIVES:In prosthodontic procedures, traditional computer-aided design (CAD) is often time-consuming and lacks accuracy in shape restoration. In this study, we combined implicit template and deep learning (DL) to construct a precise neural network for personalized tooth defect restoration. METHODS:Ninety models of right maxillary central incisor (80 for training, 10 for validation) were collected. A DL model named ToothDIT was trained to establish an implicit template and a neural network capable of predicting unique identifications. In the validation stage, teeth in validation set were processed into corner, incisive, and medium defects. The defective teeth were inputted into ToothDIT to predict the unique identification, which actuated the deformation of the implicit template to generate the highly customized template (DIT) for the target tooth. Morphological restorations were executed with templates from template shape library (TSL), average tooth template (ATT), and DIT in Exocad (GmbH, Germany). RMS, width, length, aspect ratio, incisal edge curvature, incisive end retraction, and guiding inclination were introduced to assess the restorative accuracy. Statistical analysis was conducted using two-way ANOVA and paired t-test for overall and detailed differences. RESULTS:DIT displayed significantly smaller RMS than TSL and ATT. In 2D detailed analysis, DIT exhibited significantly less deviations from the natural teeth compared to TSL and ATT. CONCLUSION:The proposed DL model successfully reconstructed the morphology of anterior teeth with various degrees of defects and achieved satisfactory accuracy. This approach provides a more reliable reference for prostheses design, resulting in enhanced accuracy in morphological restoration. CLINICAL SIGNIFICANCE:This DL model holds promise in assisting dentists and technicians in obtaining morphology templates that closely resemble the original shape of the defective teeth. These customized templates serve as a foundation for enhancing the efficiency and precision of digital restorative design for defective teeth.
10.1016/j.jdent.2024.104971
Prediction of facial deformation after complete denture prosthesis using BP neural network.
Cheng Cheng,Cheng Xiaosheng,Dai Ning,Jiang Xiaotong,Sun Yuchun,Li Weiwei
Computers in biology and medicine
With the accelerated aging of world population, complete denture prosthesis plays an increasingly important role in mouth rehabilitation. In addition to recovering stomatognathic system function, restoring the appearance of a third of the area under the face has become a great challenge in complete denture prosthesis. This study analyzes the interactive relationship between the appearance of a third of the area under the face and complete denture, and proposes a new method to predict facial deformation after complete denture prosthesis. Firstly, to improve computational efficiency, the feature template is constructed to replace the deformed facial region. Secondly, a forecast model of elastic deformation is constructed using BP neural network and predicts elastic deformation amount because of the inhomogeneous, anisotropic and nonlinear material properties of soft tissue. Finally, a new feature template is calculated using deformation amount, and the deformation of preoperative model is simulated using Laplacian deformation technique. The average error rates of different hidden layer nodes in the neural network are analysed. Deformation and postoperative models are superimposed for match analysis. Experimental results show that this method can predict facial soft tissue deformation quickly and accurately.
10.1016/j.compbiomed.2015.08.018
Accuracy Comparison between Robot-Assisted Dental Implant Placement and Static/Dynamic Computer-Assisted Implant Surgery: A Systematic Review and Meta-Analysis of In Vitro Studies.
Medicina (Kaunas, Lithuania)
: The present systematic review and meta-analysis undertake a comparison of studies that examine the accuracy of robot-assisted dental implant placement in relation to static computer-assisted implant surgery (SCAIS), dynamic computer-assisted implant surgery (DCAIS), and freehand procedures. This study aims to provide a comprehensive understanding of the precision of robot-assisted dental implant placement and its comparative efficacy in relation to other placement techniques. : The guidelines recommended by Preferred Reporting Items for Systematic Reviews and Meta-Analyses (PRISMA) were used to organize and compose this review. Four electronic databases (PubMed, Web of Science, Scopus, and Cochrane) were systematically searched for pertinent articles. Articles were selected following the inclusion and exclusion criteria. Qualitative and quantitative analyses of the selected articles were performed. : The initial electronic search resulted in 1087 hits. Based on the inclusion and exclusion criteria, five articles were selected for qualitative analysis, out of which three were considered for quantitative analysis. Three parameters were considered for accuracy evaluation (angular, coronal, and apical deviation). The mean angular deviation was -1.22 degrees (95% CI, -1.06--1.39), the mean coronal deviation was -0.15 mm (95% CI, -0.24--0.07), and the mean apical deviation was -0.19 mm (95% CI, -0.27--0.10). : The robotic implant system was found to have significantly lower angular deviations and insignificantly lower coronal and apical deviations compared to DCAIS. Within the limitations of this review, it can be concluded that robot-assisted implant placement in resin models permits higher accuracy compared to DCAIS and SCAIS systems. However, due to the limited number of comparative studies with high heterogeneity, the findings of this review should be interpreted with caution. Further research is necessary to confirm the clinical application of robotics in implant surgery.
10.3390/medicina60010011
Generative deep learning approaches for the design of dental restorations: A narrative review.
Journal of dentistry
OBJECTIVES:This study aims to explore and discuss recent advancements in tooth reconstruction utilizing deep learning (DL) techniques. A review on new DL methodologies in partial and full tooth reconstruction is conducted. DATA/SOURCES:PubMed, Google Scholar, and IEEE Xplore databases were searched for articles from 2003 to 2023. STUDY SELECTION:The review includes 9 articles published from 2018 to 2023. The selected articles showcase novel DL approaches for tooth reconstruction, while those concentrating solely on the application or review of DL methods are excluded. The review shows that data is acquired via intraoral scans or laboratory scans of dental plaster models. Common data representations are depth maps, point clouds, and voxelized point clouds. Reconstructions focus on single teeth, using data from adjacent teeth or the entire jaw. Some articles include antagonist teeth data and features like occlusal grooves and gap distance. Primary network architectures include Generative Adversarial Networks (GANs) and Transformers. Compared to conventional digital methods, DL-based tooth reconstruction reports error rates approximately two times lower. CONCLUSIONS:Generative DL models analyze dental datasets to reconstruct missing teeth by extracting insights into patterns and structures. Through specialized application, these models reconstruct morphologically and functionally sound dental structures, leveraging information from the existing teeth. The reported advancements facilitate the feasibility of DL-based dental crown reconstruction. Beyond GANs and Transformers with point clouds or voxels, recent studies indicate promising outcomes with diffusion-based architectures and innovative data representations like wavelets for 3D shape completion and inference problems. CLINICAL SIGNIFICANCE:Generative network architectures employed in the analysis and reconstruction of dental structures demonstrate notable proficiency. The enhanced accuracy and efficiency of DL-based frameworks hold the potential to enhance clinical outcomes and increase patient satisfaction. The reduced reconstruction times and diminished requirement for manual intervention may lead to cost savings and improved accessibility of dental services.
10.1016/j.jdent.2024.104988
Deep learning-based detection of dental prostheses and restorations.
Takahashi Toshihito,Nozaki Kazunori,Gonda Tomoya,Mameno Tomoaki,Ikebe Kazunori
Scientific reports
The purpose of this study is to develop a method for recognizing dental prostheses and restorations of teeth using a deep learning. A dataset of 1904 oral photographic images of dental arches (maxilla: 1084 images; mandible: 820 images) was used in the study. A deep-learning method to recognize the 11 types of dental prostheses and restorations was developed using TensorFlow and Keras deep learning libraries. After completion of the learning procedure, the average precision of each prosthesis, mean average precision, and mean intersection over union were used to evaluate learning performance. The average precision of each prosthesis varies from 0.59 to 0.93. The mean average precision and mean intersection over union of this system were 0.80 and 0.76, respectively. More than 80% of metallic dental prostheses were detected correctly, but only 60% of tooth-colored prostheses were detected. The results of this study suggest that dental prostheses and restorations that are metallic in color can be recognized and predicted with high accuracy using deep learning; however, those with tooth color are recognized with moderate accuracy.
10.1038/s41598-021-81202-x
Predicting the Debonding of CAD/CAM Composite Resin Crowns with AI.
Yamaguchi S,Lee C,Karaer O,Ban S,Mine A,Imazato S
Journal of dental research
A preventive measure for debonding has not been established and is highly desirable to improve the survival rate of computer-aided design/computer-aided manufacturing (CAD/CAM) composite resin (CR) crowns. The aim of this study was to assess the usefulness of deep learning with a convolution neural network (CNN) method to predict the debonding probability of CAD/CAM CR crowns from 2-dimensional images captured from 3-dimensional (3D) stereolithography models of a die scanned by a 3D oral scanner. All cases of CAD/CAM CR crowns were manufactured from April 2014 to November 2015 at the Division of Prosthodontics, Osaka University Dental Hospital (Ethical Review Board at Osaka University, approval H27-E11). The data set consisted of a total of 24 cases: 12 trouble-free and 12 debonding as known labels. A total of 8,640 images were randomly divided into 6,480 training and validation images and 2,160 test images. Deep learning with a CNN method was conducted to develop a learning model to predict the debonding probability. The prediction accuracy, precision, recall, F-measure, receiver operating characteristic, and area under the curve of the learning model were assessed for the test images. Also, the mean calculation time was measured during the prediction for the test images. The prediction accuracy, precision, recall, and F-measure values of deep learning with a CNN method for the prediction of the debonding probability were 98.5%, 97.0%, 100%, and 0.985, respectively. The mean calculation time was 2 ms/step for 2,160 test images. The area under the curve was 0.998. Artificial intelligence (AI) technology-that is, the deep learning with a CNN method established in this study-demonstrated considerably good performance in terms of predicting the debonding probability of a CAD/CAM CR crown with 3D stereolithography models of a die scanned from patients.
10.1177/0022034519867641
Evaluation of the efficiency, trueness, and clinical application of novel artificial intelligence design for dental crown prostheses.
Dental materials : official publication of the Academy of Dental Materials
OBJECTIVE:The unique structure of human teeth limits dental repair to custom-made solutions. The production process requires a lot of time and manpower. At present, artificial intelligence (AI) has begun to be used in the medical field and improve efficiency. This study attempted to design a variety of dental restorations using AI and evaluate their clinical applicability. METHODS:Using inlay and crown restoration types commonly used in dental standard models, we compared differences in artificial wax-up carving (wax-up), artificial digital designs (digital) and AI designs (AI). The AI system was designed using computer calculations, and the other two methods were designed by humans. Restorations were made by 3D printing resin material. Image evaluations were compared with cone beam computed tomography (CBCT) by calculating the root mean squared error. RESULTS:Surface truth results showed that AI (68.4 µm) and digital-designed crowns (51.0 µm) had better reproducibility. Using AI for the crown reduced the time spent by 400% (compared to digital) and 900% (compared to wax-up). Optical microscopic and CBCT images showed that AI and digital designs had close margin gaps (p < 0.05). The margin gap of the crown showed that the wax-up group was 4.1 and 4.3 times greater than those of the AI and digital crowns, respectively. Therefore, the utilization of artificial intelligence can assist in the production of dental restorations, thereby enhancing both production efficiency and accuracy. SIGNIFICANCE:It is expected that the development of AI can contribute to the reproducibility, efficiency, and goodness of fit of dental restorations.
10.1016/j.dental.2023.10.013
Deep learning in the overall process of implant prosthodontics: A state-of-the-art review.
Clinical implant dentistry and related research
Artificial intelligence represented by deep learning has attracted attention in the field of dental implant restoration. It is widely used in surgical image analysis, implant plan design, prosthesis shape design, and prognosis judgment. This article mainly describes the research progress of deep learning in the whole process of dental implant prosthodontics. It analyzes the limitations of current research, and looks forward to the future development direction.
10.1111/cid.13307
Influence of dental fillings and tooth type on the performance of a novel artificial intelligence-driven tool for automatic tooth segmentation on CBCT images - A validation study.
Fontenele Rocharles Cavalcante,Gerhardt Maurício do Nascimento,Pinto Jáder Camilo,Van Gerven Adriaan,Willems Holger,Jacobs Reinhilde,Freitas Deborah Queiroz
Journal of dentistry
OBJECTIVES:To assess the influence of dental fillings on the performance of an artificial intelligence (AI)-driven tool for tooth segmentation on cone-beam computed tomography (CBCT) according to the type of tooth. METHODS:A total of 175 CBCT scans (500 teeth) were recruited for performing training (140 CBCT scans - 400 teeth) and validation (35 CBCT scans - 100 teeth) of the AI convolutional neural networks. The test dataset involved 74 CBCT scans (226 teeth), which was further divided into control and experimental groups depending on the presence of dental filling: without filling (control group: 24 CBCT scans - 113 teeth) and with coronal and/or root filling (experimental group: 50 CBCT scans - 113 teeth). The segmentation performance for both groups was assessed. Additionally, 10% of each tooth type (anterior, premolar, and molar) was randomly selected for time analysis according to manual, AI-based and refined-AI segmentation methods. RESULTS:The presence of fillings significantly influenced the segmentation performance (p<0.05). However, the accuracy metrics showed an excellent range of values for both control (95% Hausdorff Distance (95% HD): 0.01-0.08 mm; Intersection over union (IoU): 0.97-0.99; Dice similarity coefficient (DSC): 0.98-0.99; Precision: 1.00; Recall: 0.97-0.99; Accuracy: 1.00) and experimental groups (95% HD: 0.17-0.25 mm; IoU: 0.91-0.95; DSC: 0.95-0.97; Precision:1.00; Recall: 0.91-0.95; Accuracy: 0.99-1.00). The time analysis showed that the AI-based segmentation was significantly faster with a mean time of 29.8 s (p<0.001). CONCLUSIONS:The proposed AI-driven tool allowed an accurate and time-efficient approach for the segmentation of teeth on CBCT images irrespective of the presence of high-density dental filling material and the type of tooth. CLINICAL SIGNIFICANCE:Tooth segmentation is a challenging and time-consuming task, mainly in the presence of artifacts generated by dental filling material. The proposed AI-driven tool could offer a clinically acceptable approach for tooth segmentation, to be applied in the digital dental workflows considering its time efficiency and high accuracy regardless of the presence of dental fillings.
10.1016/j.jdent.2022.104069
An artificial intelligence system using machine-learning for automatic detection and classification of dental restorations in panoramic radiography.
Abdalla-Aslan Ragda,Yeshua Talia,Kabla Daniel,Leichter Isaac,Nadler Chen
Oral surgery, oral medicine, oral pathology and oral radiology
OBJECTIVES:The aim of this study was to develop a computer vision algorithm based on artificial intelligence, designed to automatically detect and classify various dental restorations on panoramic radiographs. STUDY DESIGN:A total of 738 dental restorations in 83 anonymized panoramic images were analyzed. Images were automatically cropped to obtain the region of interest containing maxillary and mandibular alveolar ridges. Subsequently, the restorations were segmented by using a local adaptive threshold. The segmented restorations were classified into 11 categories, and the algorithm was trained to classify them. Numerical features based on the shape and distribution of gray level values extracted by the algorithm were used for classifying the restorations into different categories. Finally, a Cubic Support Vector Machine algorithm with Error-Correcting Output Codes was used with a cross-validation approach for the multiclass classification of the restorations according to these features. RESULTS:The algorithm detected 94.6% of the restorations. Classification eliminated all erroneous marks, and ultimately, 90.5% of the restorations were marked on the image. The overall accuracy of the classification stage in discriminating between the true restoration categories was 93.6%. CONCLUSIONS:This machine-learning algorithm demonstrated excellent performance in detecting and classifying dental restorations on panoramic images.
10.1016/j.oooo.2020.05.012
Automated detection of posterior restorations in permanent teeth using artificial intelligence on intraoral photographs.
Journal of dentistry
OBJECTIVES:Intraoral photographs might be considered the machine-readable equivalent of a clinical-based visual examination and can potentially be used to detect and categorize dental restorations. The first objective of this study was to develop a deep learning-based convolutional neural network (CNN) for automated detection and categorization of posterior composite, cement, amalgam, gold and ceramic restorations on clinical photographs. Second, this study aimed to determine the diagnostic accuracy for the developed CNN (test method) compared to that of an expert evaluation (reference standard). METHODS:The whole image set of 1761 images (483 of unrestored teeth, 570 of composite restorations, 213 of cements, 278 of amalgam restorations, 125 of gold restorations and 92 of ceramic restorations) was divided into a training set (N = 1407, 401, 447, 66, 231, 93, and 169, respectively) and a test set (N = 354, 82, 123, 26, 47, 32, and 44). The expert diagnoses served as a reference standard for cyclic training and repeated evaluation of the CNN (ResNeXt-101-32 × 8d), which was trained by using image augmentation and transfer learning. Statistical analysis included the calculation of contingency tables, areas under the receiver operating characteristic curve and saliency maps. RESULTS:After training was complete, the CNN was able to categorize restorations correctly with the following diagnostic accuracy values: 94.9% for unrestored teeth, 92.9% for composites, 98.3% for cements, 99.2% for amalgam restorations, 99.4% for gold restorations and 97.8% for ceramic restorations. CONCLUSIONS:It was possible to categorize different types of posterior restorations on intraoral photographs automatically with a good diagnostic accuracy. CLINICAL SIGNIFICANCE:Dental diagnostics might be supported by artificial intelligence-based algorithms in the future. However, further improvements are needed to increase accuracy and practicability.
10.1016/j.jdent.2022.104124
Artificial intelligence in fixed implant prosthodontics: a retrospective study of 106 implant-supported monolithic zirconia crowns inserted in the posterior jaws of 90 patients.
Lerner Henriette,Mouhyi Jaafar,Admakin Oleg,Mangano Francesco
BMC oral health
BACKGROUND:Artificial intelligence (AI) is a branch of computer science concerned with building smart software or machines capable of performing tasks that typically require human intelligence. We present a protocol for the use of AI to fabricate implant-supported monolithic zirconia crowns (MZCs) cemented on customized hybrid abutments. METHODS:The study protocol consisted of: (1) intraoral scan of the implant position; (2) design of the individual abutment and temporary crown using computer-aided design (CAD) software; (3) milling of the zirconia abutment and the temporary polymethyl-methacrylate (PMMA) crown, with extraoral cementation of the zirconia abutment on the relative titanium bonding base, to generate an individual hybrid abutment; (4) clinical application of the hybrid abutment and the temporary PMMA crown; (5) intraoral scan of the hybrid abutment; (6) CAD of the final crown with automated margin line design using AI; (7) milling, sintering and characterisation of the final MZC; and (8) clinical application of the MZC. The outcome variables were mathematical (quality of the fabrication of the individual zirconia abutment) and clinical, such as (1) quality of the marginal adaptation, (2) of interproximal contact points and (3) of occlusal contacts, (4) chromatic integration, (5) survival and (6) success of MZCs. A careful statistical analysis was performed. RESULTS:90 patients (35 males, 55 females; mean age 53.3 ± 13.7 years) restored with 106 implant-supported MZCs were included in the study. The follow-up varied from 6 months to 3 years. The quality of the fabrication of individual hybrid abutments revealed a mean deviation of 44 μm (± 6.3) between the original CAD design of the zirconia abutment, and the mesh of the zirconia abutment captured intraorally at the end of the provisionalization. At the delivery of the MZCs, the marginal adaptation, quality of interproximal and occlusal contacts, and aesthetic integration were excellent. The three-year cumulative survival and success of the MZCs were 99.0% and 91.3%, respectively. CONCLUSIONS:AI seems to represent a reliable tool for the restoration of single implants with MZCs cemented on customised hybrid abutments via a full digital workflow. Further studies are needed to confirm these positive results.
10.1186/s12903-020-1062-4
Current Trends in the Reconstruction and Rehabilitation of Jaw following Ablative Surgery.
Cancers
The reconstruction and rehabilitation of jaws following ablative surgery have been transformed in recent years by the development of computer-assisted surgery and virtual surgical planning. In this narrative literature review, we aim to discuss the current state-of-the-art jaw reconstruction, and to preview the potential future developments. The application of patient-specific implants and the "jaw-in-a-day technique" have made the fast restoration of jaws' function and aesthetics possible. The improved efficiency of primary reconstructive surgery allows for the rehabilitation of neurosensory function following ablative surgery. Currently, a great deal of research has been conducted on augmented/mixed reality, artificial intelligence, virtual surgical planning for soft tissue reconstruction, and the rehabilitation of the stomatognathic system. This will lead to an even more exciting future for the functional reconstruction and rehabilitation of the jaw following ablative surgery.
10.3390/cancers14143308
Development of an artificial intelligence-based algorithm to classify images acquired with an intraoral scanner of individual molar teeth into three categories.
Eto Nozomi,Yamazoe Junichi,Tsuji Akiko,Wada Naohisa,Ikeda Noriaki
PloS one
BACKGROUND:Forensic dentistry identifies deceased individuals by comparing postmortem dental charts, oral-cavity pictures and dental X-ray images with antemortem records. However, conventional forensic dentistry methods are time-consuming and thus unable to rapidly identify large numbers of victims following a large-scale disaster. OBJECTIVE:Our goal is to automate the dental filing process by using intraoral scanner images. In this study, we generated and evaluated an artificial intelligence-based algorithm that classified images of individual molar teeth into three categories: (1) full metallic crown (FMC); (2) partial metallic restoration (In); or (3) sound tooth, carious tooth or non-metallic restoration (CNMR). METHODS:A pre-trained model was created using oral-cavity pictures from patients. Then, the algorithm was generated through transfer learning and training with images acquired from cadavers by intraoral scanning. Cross-validation was performed to reduce bias. The ability of the model to classify molar teeth into the three categories (FMC, In or CNMR) was evaluated using four criteria: precision, recall, F-measure and overall accuracy. RESULTS:The average value (variance) was 0.952 (0.000140) for recall, 0.957 (0.0000614) for precision, 0.952 (0.000145) for F-measure, and 0.952 (0.000142) for overall accuracy when the algorithm was used to classify images of molar teeth acquired from cadavers by intraoral scanning. CONCLUSION:We have created an artificial intelligence-based algorithm that analyzes images acquired with an intraoral scanner and classifies molar teeth into one of three types (FMC, In or CNMR) based on the presence/absence of metallic restorations. Furthermore, the accuracy of the algorithm reached about 95%. This algorithm was constructed as a first step toward the development of an automated system that generates dental charts from images acquired by an intraoral scanner. The availability of such a system would greatly increase the efficiency of personal identification in the event of a major disaster.
10.1371/journal.pone.0261870
Accuracy of artificial intelligence-designed single-molar dental prostheses: A feasibility study.
The Journal of prosthetic dentistry
STATEMENT OF PROBLEM:Computer-aided design and computer-aided manufacturing (CAD-CAM) technology has greatly improved the efficiency of the fabrication of dental prostheses. However, the design process (CAD stage) is still time-consuming and labor intensive. PURPOSE:The purpose of this feasibility study was to investigate the accuracy of a novel artificial intelligence (AI) system in designing biomimetic single-molar dental prostheses by comparing and matching them to the natural molar teeth. MATERIAL AND METHODS:A total of 169 maxillary casts were obtained from healthy dentate participants. The casts were digitized, duplicated, and processed with the removal of the maxillary right first molar. A total of 159 pairs of original and processed casts were input into the Generative Adversarial Networks (GANs) for training. In validation, 10 sets of processed casts were input into the AI system, and 10 AI-designed teeth were generated through backpropagation. Individual AI-designed teeth were then superimposed onto each of the 10 original teeth, and the morphological differences in mean Hausdorff distance were measured. True reconstruction was defined as correct matching between the AI-designed and original teeth with the smallest mean Hausdorff distance. The ratio of true reconstruction was calculated as the Intersection-over-Union. The reconstruction performance of the AI system was determined by the Hausdorff distance and Intersection-over-Union. RESULTS:Data of validation showed that the mean Hausdorff distance ranged from 0.441 to 0.752 mm and the Intersection-over-Union of the system was 0.600 (60%). CONCLUSIONS:This study demonstrated the feasibility of AI in designing single-molar dental prostheses. With further training and optimization of algorithms, the accuracy of biomimetic AI-designed dental prostheses could be further enhanced.
10.1016/j.prosdent.2022.12.004
Artificial intelligence-designed single molar dental prostheses: A protocol of prospective experimental study.
PloS one
BACKGROUND:Dental prostheses, which aim to replace missing teeth and to restore patients' appearance and oral functions, should be biomimetic and thus adopt the occlusal morphology and three-dimensional (3D) position of healthy natural teeth. Since the teeth of an individual subject are controlled by the same set of genes (genotype) and are exposed to mostly identical oral environment (phenotype), the occlusal morphology and 3D position of teeth of an individual patient are inter-related. It is hypothesized that artificial intelligence (AI) can automate the design of single-tooth dental prostheses after learning the features of the remaining dentition. MATERIALS AND METHODS:This article describes the protocol of a prospective experimental study, which aims to train and to validate the AI system for design of single molar dental prostheses. Maxillary and mandibular dentate teeth models will be collected and digitized from at least 250 volunteers. The (original) digitized maxillary teeth models will be duplicated and processed by removal of right maxillary first molars (FDI tooth 16). Teeth models will be randomly divided into training and validation sets. At least 200 training sets of the original and the processed digitalized teeth models will be input into 3D Generative Adversarial Network (GAN) for training. Among the validation sets, tooth 16 will be generated by AI on 50 processed models and the morphology and 3D position of AI-generated tooth will be compared to that of the natural tooth in the original maxillary teeth model. The use of different GAN algorithms and the need of antagonist mandibular teeth model will be investigated. Results will be reported following the CONSORT-AI.
10.1371/journal.pone.0268535
Evolution of Aesthetic Dentistry.
Blatz M B,Chiche G,Bahat O,Roblee R,Coachman C,Heymann H O
Journal of dental research
One of the main goals of dental treatment is to mimic teeth and design smiles in a most natural and aesthetic manner, based on the individual and specific needs of the patient. Possibilities to reach that goal have significantly improved over the last decade through new and specific treatment modalities, steadily enhanced and more aesthetic dental materials, and novel techniques and technologies. This article gives an overview of the evolution of aesthetic dentistry over the past 100 y from a historical point of view and highlights advances in the development of dental research and clinical interventions that have contributed the science and art of aesthetic dentistry. Among the most noteworthy advancements over the past decade are the establishment of universal aesthetic rules and guidelines based on the assessment of natural aesthetic parameters, anatomy, and physiognomy; the development of tooth whitening and advanced restorative as well as prosthetic materials and techniques, supported by the pioneering discovery of dental adhesion; the significant progress in orthodontics and periodontal as well as oral and maxillofacial surgery; and, most recently, the implementation of digital technologies in the 3-dimensional planning and realization of truly natural, individual, and aesthetic smiles. In the future, artificial intelligence and machine learning will likely lead to automation of aesthetic evaluation, smile design, and treatment-planning processes.
10.1177/0022034519875450
Artificial intelligence models for tooth-supported fixed and removable prosthodontics: A systematic review.
The Journal of prosthetic dentistry
STATEMENT OF PROBLEM:Artificial intelligence applications are increasing in prosthodontics. Still, the current development and performance of artificial intelligence in prosthodontic applications has not yet been systematically documented and analyzed. PURPOSE:The purpose of this systematic review was to assess the performance of the artificial intelligence models in prosthodontics for tooth shade selection, automation of restoration design, mapping the tooth preparation finishing line, optimizing the manufacturing casting, predicting facial changes in patients with removable prostheses, and designing removable partial dentures. MATERIAL AND METHODS:An electronic systematic review was performed in MEDLINE/PubMed, EMBASE, Web of Science, Cochrane, and Scopus. A manual search was also conducted. Studies with artificial intelligence models were selected based on 6 criteria: tooth shade selection, automated fabrication of dental restorations, mapping the finishing line of tooth preparations, optimizing the manufacturing casting process, predicting facial changes in patients with removable prostheses, and designing removable partial dentures. Two investigators independently evaluated the quality assessment of the studies by applying the Joanna Briggs Institute Critical Appraisal Checklist for Quasi-Experimental Studies (nonrandomized experimental studies). A third investigator was consulted to resolve lack of consensus. RESULTS:A total of 36 articles were reviewed and classified into 6 groups based on the application of the artificial intelligence model. One article reported on the development of an artificial intelligence model for tooth shade selection, reporting better shade matching than with conventional visual selection; 14 articles reported on the feasibility of automated design of dental restorations using different artificial intelligence models; 1 artificial intelligence model was able to mark the margin line without manual interaction with an average accuracy ranging from 90.6% to 97.4%; 2 investigations developed artificial intelligence algorithms for optimizing the manufacturing casting process, reporting an improvement of the design process, minimizing the porosity on the cast metal, and reducing the overall manufacturing time; 1 study proposed an artificial intelligence model that was able to predict facial changes in patients using removable prostheses; and 17 investigations that developed clinical decision support, expert systems for designing removable partial dentures for clinicians and educational purposes, computer-aided learning with video interactive programs for student learning, and automated removable partial denture design. CONCLUSIONS:Artificial intelligence models have shown the potential for providing a reliable diagnostic tool for tooth shade selection, automated restoration design, mapping the preparation finishing line, optimizing the manufacturing casting, predicting facial changes in patients with removable prostheses, and designing removable partial dentures, but they are still in development. Additional studies are needed to further develop and assess their clinical performance.
10.1016/j.prosdent.2021.06.001
Artificial intelligence applications in restorative dentistry: A systematic review.
The Journal of prosthetic dentistry
STATEMENT OF PROBLEM:Artificial intelligence (AI) applications are increasing in restorative procedures. However, the current development and performance of AI in restorative dentistry applications has not yet been systematically documented and analyzed. PURPOSE:The purpose of this systematic review was to identify and evaluate the ability of AI models in restorative dentistry to diagnose dental caries and vertical tooth fracture, detect tooth preparation margins, and predict restoration failure. MATERIAL AND METHODS:An electronic systematic review was performed in 5 databases: MEDLINE/PubMed, EMBASE, World of Science, Cochrane, and Scopus. A manual search was also conducted. Studies with AI models were selected based on 4 criteria: diagnosis of dental caries, diagnosis of vertical tooth fracture, detection of the tooth preparation finishing line, and prediction of restoration failure. Two investigators independently evaluated the quality assessment of the studies by applying the Joanna Briggs Institute (JBI) Critical Appraisal Checklist for Quasi-Experimental Studies (nonrandomized experimental studies). A third investigator was consulted to resolve lack of consensus. RESULTS:A total of 34 articles were included in the review: 29 studies included AI techniques for the diagnosis of dental caries or the elaboration of caries and postsensitivity prediction models, 2 for the diagnosis of vertical tooth fracture, 1 for the tooth preparation finishing line location, and 2 for the prediction of the restoration failure. Among the studies reviewed, the AI models tested obtained a caries diagnosis accuracy ranging from 76% to 88.3%, sensitivity ranging from 73% to 90%, and specificity ranging from 61.5% to 93%. The caries prediction accuracy among the studies ranged from 83.6% to 97.1%. The studies reported an accuracy for the vertical tooth fracture diagnosis ranging from 88.3% to 95.7%. The article using AI models to locate the finishing line reported an accuracy ranging from 90.6% to 97.4%. CONCLUSIONS:AI models have the potential to provide a powerful tool for assisting in the diagnosis of caries and vertical tooth fracture, detecting the tooth preparation margin, and predicting restoration failure. However, the dental applications of AI models are still in development. Further studies are required to assess the clinical performance of AI models in restorative dentistry.
10.1016/j.prosdent.2021.02.010