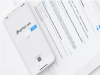
Convolutional neural network for identifying common bile duct stones based on magnetic resonance cholangiopancreatography.
Clinical radiology
AIMS:To develop an auto-categorization system based on machine learning for three-dimensional magnetic resonance cholangiopancreatography (3D MRCP) to detect choledocholithiasis from healthy and symptomatic individuals. MATERIALS AND METHODS:3D MRCP sequences from 254 cases with common bile duct (CBD) stones and 251 cases with normal CBD were enrolled to train the 3D Convolutional Neural Network (3D-CNN) model. Then 184 patients from three different hospitals (91 with positive CBD stone and 93 with normal CBD) were prospectively included to test the performance of 3D-CNN. RESULTS:With a cutoff value of 0.2754, 3D-CNN achieved the sensitivity, specificity, and accuracy of 94.51%, 92.47%, and 93.48%, respectively. In the receiver operating characteristic curve analysis, the area under the curve (AUC) for the presence or absence of CBD stones was 0.974 (95% CI, 0.940-0.992). There was no significant difference in sensitivity, specificity, and accuracy between 3D-CNN and radiologists. In addition, the performance of 3D-CNN was also evaluated in the internal test set and the external test set, respectively. The internal test set yielded an accuracy of 94.74% and AUC of 0.974 (95% CI, 0.919-0.996), and the external test set yielded an accuracy of 92.13% and AUC of 0.970 (95% CI, 0.911-0.995). CONCLUSIONS:An artificial intelligence-assisted diagnostic system for CBD stones was constructed using 3D-CNN model for 3D MRCP images. The performance of 3D-CNN model was comparable to that of radiologists in diagnosing CBD stones. 3D-CNN model maintained high performance when applied to data from other hospitals.
10.1016/j.crad.2024.02.018
Using artificial intelligence to predict choledocholithiasis: can machine learning models abate the use of MRCP in patients with biliary dysfunction?
ANZ journal of surgery
BACKGROUND:Prompt diagnosis of choledocholithiasis is crucial for reducing disease severity, preventing complications and minimizing length of stay. Magnetic resonance cholangiopancreatography (MRCP) is commonly used to evaluate patients with suspected choledocholithiasis but is expensive and may delay definitive intervention. To optimize patient care and resource utilization, we have developed five machine learning models that predict a patients' risk of choledocholithiasis based on clinical presentation and pre-MRCP investigation results. METHODS:Inpatients admitted to the Royal Hobart Hospital from 2018 to 2023 with a suspicion of choledocholithiasis were included. Exclusion criteria included prior hepatobiliary surgery, known hepatobiliary disease, or incomplete records. Variables related to clinical presentation, laboratory testing, and sonographic or CT imaging were collected. Four machine learning techniques were employed: logistic regression, XGBoost, random forest, and K-nearest neighbours. The three best performing models were combined to create an ensemble model. Model performance was compared against the American Society for Gastrointestinal Endoscopy (ASGE) choledocholithiasis risk stratification guidelines. RESULTS:Of the 222 patients included, 113 (50.9%) had choledocholithiasis. The most successful models were the random forest (accuracy: 0.79, AUROC: 0.83) and ensemble (accuracy and AUROC: 0.81). Every model outperformed the ASGE guidelines. Key variables influencing the models' predictions included common bile duct diameter, lipase, imaging evidence of cholelithiasis, and liver function tests. CONCLUSION:Machine learning models can accurately assess a patient's risk of choledocholithiasis and could assist in identifying patients who could forgo an MRCP and proceed directly to intervention. Ongoing validation on prospective data is necessary to refine their accuracy and clinical utility.
10.1111/ans.18950
A machine learning-based choledocholithiasis prediction tool to improve ERCP decision making: a proof-of-concept study.
Endoscopy
BACKGROUND:Previous studies demonstrated limited accuracy of existing guidelines for predicting choledocholithiasis, leading to overutilization of endoscopic retrograde cholangiopancreatography (ERCP). More accurate stratification may improve patient selection for ERCP and allow use of lower-risk modalities. METHODS:A machine learning model was developed using patient information from two published cohort studies that evaluated performance of guidelines in predicting choledocholithiasis. Prediction models were developed using the gradient boosting model (GBM) machine learning method. GBM performance was evaluated using 10-fold cross-validation and area under the receiver operating characteristic curve (AUC). Important predictors of choledocholithiasis were identified based on relative importance in the GBM. RESULTS:1378 patients (mean age 43.3 years; 61.2% female) were included in the GBM and 59.4% had choledocholithiasis. Eight variables were identified as predictors of choledocholithiasis. The GBM had accuracy of 71.5% (SD 2.5%) (AUC 0.79 [SD 0.06]) and performed better than the 2019 American Society for Gastrointestinal Endoscopy (ASGE) guidelines (accuracy 62.4% [SD 2.6%]; AUC 0.63 [SD 0.03]) and European Society of Gastrointestinal Endoscopy (ESGE) guidelines (accuracy 62.8% [SD 2.6%]; AUC 0.67 [SD 0.02]). The GBM correctly categorized 22% of patients directed to unnecessary ERCP by ASGE guidelines, and appropriately recommended as the next management step 48% of ERCPs incorrectly rejected by ESGE guidelines. CONCLUSIONS:A machine learning-based tool was created, providing real-time, personalized, objective probability of choledocholithiasis and ERCP recommendations. This more accurately directed ERCP use than existing ASGE and ESGE guidelines, and has the potential to reduce morbidity associated with ERCP or missed choledocholithiasis.
10.1055/a-2174-0534