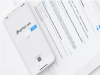
Using machine-learning algorithms to identify patients at high risk of upper gastrointestinal lesions for endoscopy.
Liu Yongjia,Lin Da,Li Lan,Chen Yu,Wen Jiayao,Lin Yiguang,He Xingxiang
Journal of gastroenterology and hepatology
BACKGROUND AND AIM:Endoscopic screening for early detection of upper gastrointestinal (UGI) lesions is important. However, population-based endoscopic screening is difficult to implement in populous countries. By identifying high-risk individuals from the general population, the screening targets can be narrowed to individuals who are in most need of an endoscopy. This study was designed to develop an artificial intelligence (AI)-based model to predict patient risk of UGI lesions to identify high-risk individuals for endoscopy. METHODS:A total of 620 patients (from 5300 participants) were equally allocated into 10 parts for 10-fold cross validation experiments. The machine-learning predictive models for UGI lesion risk were constructed using random forest, logistic regression, decision tree, and support vector machine (SVM) algorithms. A total of 48 variables covering lifestyles, social-economic status, clinical symptoms, serological results, and pathological data were used in the model construction. RESULTS:The accuracies of the four models were between 79.3% and 93.4% in the training set and between 77.2% and 91.2% in the testing dataset (logistics regression: 77.2%; decision tree: 87.3%; random forest: 88.2%; SVM: 91.2%;). The AUCs of four models showed impressive predictive ability. Comparing the four models with the different algorithms, the SVM model featured the best sensitivity and specificity in all datasets tested. CONCLUSIONS:Machine-learning algorithms can accurately and reliably predict the risk of UGI lesions based on readily available parameters. The predictive models have the potential to be used clinically for identifying patients with high risk of UGI lesions and stratifying patients for necessary endoscopic screening.
10.1111/jgh.15530
Deep Learning for Histopathological Assessment of Esophageal Adenocarcinoma Precursor Lesions.
Modern pathology : an official journal of the United States and Canadian Academy of Pathology, Inc
Histopathological assessment of esophageal biopsies is a key part in the management of patients with Barrett esophagus (BE) but prone to observer variability and reliable diagnostic methods are needed. Artificial intelligence (AI) is emerging as a powerful tool for aided diagnosis but often relies on abstract test and validation sets while real-world behavior is unknown. In this study, we developed a 2-stage AI system for histopathological assessment of BE-related dysplasia using deep learning to enhance the efficiency and accuracy of the pathology workflow. The AI system was developed and trained on 290 whole-slide images (WSIs) that were annotated at glandular and tissue levels. The system was designed to identify individual glands, grade dysplasia, and assign a WSI-level diagnosis. The proposed method was evaluated by comparing the performance of our AI system with that of a large international and heterogeneous group of 55 gastrointestinal pathologists assessing 55 digitized biopsies spanning the complete spectrum of BE-related dysplasia. The AI system correctly graded 76.4% of the WSIs, surpassing the performance of 53 out of the 55 participating pathologists. Furthermore, the receiver-operating characteristic analysis showed that the system's ability to predict the absence (nondysplastic BE) versus the presence of any dysplasia was with an area under the curve of 0.94 and a sensitivity of 0.92 at a specificity of 0.94. These findings demonstrate that this AI system has the potential to assist pathologists in assessment of BE-related dysplasia. The system's outputs could provide a reliable and consistent secondary diagnosis in challenging cases or be used for triaging low-risk nondysplastic biopsies, thereby reducing the workload of pathologists and increasing throughput.
10.1016/j.modpat.2024.100531
Systematic review with meta-analysis: artificial intelligence in the diagnosis of oesophageal diseases.
Alimentary pharmacology & therapeutics
BACKGROUND:Artificial intelligence (AI) has recently been applied to endoscopy and questionnaires for the evaluation of oesophageal diseases (ODs). AIM:We performed a systematic review with meta-analysis to evaluate the performance of AI in the diagnosis of malignant and benign OD. METHODS:We searched MEDLINE, EMBASE, EMBASE Classic and the Cochrane Library. A bivariate random-effect model was used to calculate pooled diagnostic efficacy of AI models and endoscopists. The reference tests were histology for neoplasms and the clinical and instrumental diagnosis for gastro-oesophageal reflux disease (GERD). The pooled area under the summary receiver operating characteristic (AUROC), sensitivity, specificity, positive and negative likelihood ratio (PLR and NLR) and diagnostic odds ratio (DOR) were estimated. RESULTS:For the diagnosis of Barrett's neoplasia, AI had AUROC of 0.90, sensitivity 0.89, specificity 0.86, PLR 6.50, NLR 0.13 and DOR 50.53. AI models' performance was comparable with that of endoscopists (P = 0.35). For the diagnosis of oesophageal squamous cell carcinoma, the AUROC, sensitivity, specificity, PLR, NLR and DOR were 0.97, 0.95, 0.92, 12.65, 0.05 and DOR 258.36, respectively. In this task, AI performed better than endoscopists although without statistically significant differences. In the detection of abnormal intrapapillary capillary loops, the performance of AI was: AUROC 0.98, sensitivity 0.94, specificity 0.94, PLR 14.75, NLR 0.07 and DOR 225.83. For the diagnosis of GERD based on questionnaires, the AUROC, sensitivity, specificity, PLR, NLR and DOR were 0.99, 0.97, 0.97, 38.26, 0.03 and 1159.6, respectively. CONCLUSIONS:AI demonstrated high performance in the clinical and endoscopic diagnosis of OD.
10.1111/apt.16778
Artificial intelligence in endoscopy: Overview, applications, and future directions.
Saudi journal of gastroenterology : official journal of the Saudi Gastroenterology Association
Since the emergence of artificial intelligence (AI) in medicine, endoscopy applications in gastroenterology have been at the forefront of innovations. The ever-increasing number of studies necessitates the need to organize and classify applications in a useful way. Separating AI capabilities by computer aided detection (CADe), diagnosis (CADx), and quality assessment (CADq) allows for a systematic evaluation of each application. CADe studies have shown promise in accurate detection of esophageal, gastric and colonic neoplasia as well as identifying sources of bleeding and Crohn's disease in the small bowel. While more advanced CADx applications employ optical biopsies to give further information to characterize neoplasia and grade inflammatory disease, diverse CADq applications ensure quality and increase the efficiency of procedures. Future applications show promise in advanced therapeutic modalities and integrated systems that provide multimodal capabilities. AI is set to revolutionize clinical decision making and performance of endoscopy.
10.4103/sjg.sjg_286_23
Scoping out the future: The application of artificial intelligence to gastrointestinal endoscopy.
World journal of gastrointestinal oncology
Artificial intelligence (AI) is a quickly expanding field in gastrointestinal endoscopy. Although there are a myriad of applications of AI ranging from identification of bleeding to predicting outcomes in patients with inflammatory bowel disease, a great deal of research has focused on the identification and classification of gastrointestinal malignancies. Several of the initial randomized, prospective trials utilizing AI in clinical medicine have centered on polyp detection during screening colonoscopy. In addition to work focused on colorectal cancer, AI systems have also been applied to gastric, esophageal, pancreatic, and liver cancers. Despite promising results in initial studies, the generalizability of most of these AI systems have not yet been evaluated. In this article we review recent developments in the field of AI applied to gastrointestinal oncology.
10.4251/wjgo.v14.i5.989
Artificial intelligence-based computer-aided diagnosis system supports diagnosis of lymph node metastasis in esophageal squamous cell carcinoma: A multicenter study.
Heliyon
Background:This study aimed to develop an artificial intelligence-based computer-aided diagnosis system (AI-CAD) emulating the diagnostic logic of radiologists for lymph node metastasis (LNM) in esophageal squamous cell carcinoma (ESCC) patients, which contributed to clinical treatment decision-making. Methods:A total of 689 ESCC patients with PET/CT images were enrolled from three hospitals and divided into a training cohort and two external validation cohorts. 452 CT images from three publicly available datasets were also included for pretraining the model. Anatomic information from CT images was first obtained automatically using a U-Net-based multi-organ segmentation model, and metabolic information from PET images was subsequently extracted using a gradient-based approach. AI-CAD was developed in the training cohort and externally validated in two validation cohorts. Results:The AI-CAD achieved an accuracy of 0.744 for predicting pathological LNM in the external cohort and a good agreement with a human expert in two external validation cohorts (kappa = 0.674 and 0.587, < 0.001). With the aid of AI-CAD, the human expert's diagnostic performance for LNM was significantly improved (accuracy [95% confidence interval]: 0.712 [0.669-0.758] vs. 0.833 [0.797-0.865], specificity [95% confidence interval]: 0.697 [0.636-0.753] vs. 0.891 [0.851-0.928]; < 0.001) among patients underwent lymphadenectomy in the external validation cohorts. Conclusions:The AI-CAD could aid in preoperative diagnosis of LNM in ESCC patients and thereby support clinical treatment decision-making.
10.1016/j.heliyon.2023.e14030
The Importance of Artificial Intelligence in Upper Gastrointestinal Endoscopy.
Diagnostics (Basel, Switzerland)
Recently, there has been a growing interest in the application of artificial intelligence (AI) in medicine, especially in specialties where visualization methods are applied. AI is defined as a computer's ability to achieve human cognitive performance, which is accomplished through enabling computer "learning". This can be conducted in two ways, as machine learning and deep learning. Deep learning is a complex learning system involving the application of artificial neural networks, whose algorithms imitate the human form of learning. Upper gastrointestinal endoscopy allows examination of the esophagus, stomach and duodenum. In addition to the quality of endoscopic equipment and patient preparation, the performance of upper endoscopy depends on the experience and knowledge of the endoscopist. The application of artificial intelligence in endoscopy refers to computer-aided detection and the more complex computer-aided diagnosis. The application of AI in upper endoscopy is aimed at improving the detection of premalignant and malignant lesions, with special attention on the early detection of dysplasia in Barrett's esophagus, the early detection of esophageal and stomach cancer and the detection of infection. Artificial intelligence reduces the workload of endoscopists, is not influenced by human factors and increases the diagnostic accuracy and quality of endoscopic methods.
10.3390/diagnostics13182862
Artificial intelligence and upper gastrointestinal endoscopy: what is the optimal study design?
Minerva surgery
Upper gastrointestinal cancers (i.e., esophageal and gastric cancers) are common cancers worldwide with high mortality and morbidity. Although there is no randomized controlled trial-based evidence, early detection with endoscopy is expected to positively affect prognosis and morbidity. However, endoscopic procedures are always accompanied by human-induced errors such as overlooking of neoplasia and cancers. Recently, the use of artificial intelligence (AI) during upper gastrointestinal endoscopy is catching attention because it is expected to reduce human-induced variability of the examination. This review article introduces the overview of the expectation and current status of the AI tools for upper gastrointestinal endoscopy and shares possible challenges and corresponding solutions with readers.
10.23736/S2724-5691.22.09810-0
Endoscopists' diagnostic accuracy in detecting upper gastrointestinal neoplasia in the framework of artificial intelligence studies.
Endoscopy
BACKGROUND:Estimates on miss rates for upper gastrointestinal neoplasia (UGIN) rely on registry data or old studies. Quality assurance programs for upper GI endoscopy are not fully established owing to the lack of infrastructure to measure endoscopists' competence. We aimed to assess endoscopists' accuracy for the recognition of UGIN exploiting the framework of artificial intelligence (AI) validation studies. METHODS:Literature searches of databases (PubMed/MEDLINE, EMBASE, Scopus) up to August 2020 were performed to identify articles evaluating the accuracy of individual endoscopists for the recognition of UGIN within studies validating AI against a histologically verified expert-annotated ground-truth. The main outcomes were endoscopists' pooled sensitivity, specificity, positive and negative predictive value (PPV/NPV), and area under the curve (AUC) for all UGIN, for esophageal squamous cell neoplasia (ESCN), Barrett esophagus-related neoplasia (BERN), and gastric adenocarcinoma (GAC). RESULTS:Seven studies (2 ESCN, 3 BERN, 1 GAC, 1 UGIN overall) with 122 endoscopists were included. The pooled endoscopists' sensitivity and specificity for UGIN were 82 % (95 % confidence interval [CI] 80 %-84 %) and 79 % (95 %CI 76 %-81 %), respectively. Endoscopists' accuracy was higher for GAC detection (AUC 0.95 [95 %CI 0.93-0.98]) than for ESCN (AUC 0.90 [95 %CI 0.88-0.92]) and BERN detection (AUC 0.86 [95 %CI 0.84-0.88]). Sensitivity was higher for Eastern vs. Western endoscopists (87 % [95 %CI 84 %-89 %] vs. 75 % [95 %CI 72 %-78 %]), and for expert vs. non-expert endoscopists (85 % [95 %CI 83 %-87 %] vs. 71 % [95 %CI 67 %-75 %]). CONCLUSION:We show suboptimal accuracy of endoscopists for the recognition of UGIN even within a framework that included a higher prevalence and disease awareness. Future AI validation studies represent a framework to assess endoscopist competence.
10.1055/a-1500-3730
Artificial intelligence in gastroenterology and hepatology: how to advance clinical practice while ensuring health equity.
Gut
Artificial intelligence (AI) and machine learning (ML) systems are increasingly used in medicine to improve clinical decision-making and healthcare delivery. In gastroenterology and hepatology, studies have explored a myriad of opportunities for AI/ML applications which are already making the transition to bedside. Despite these advances, there is a risk that biases and health inequities can be introduced or exacerbated by these technologies. If unrecognised, these technologies could generate or worsen systematic racial, ethnic and sex disparities when deployed on a large scale. There are several mechanisms through which AI/ML could contribute to health inequities in gastroenterology and hepatology, including diagnosis of oesophageal cancer, management of inflammatory bowel disease (IBD), liver transplantation, colorectal cancer screening and many others. This review adapts a framework for ethical AI/ML development and application to gastroenterology and hepatology such that clinical practice is advanced while minimising bias and optimising health equity.
10.1136/gutjnl-2021-326271
Assessment of Narrow-Band Imaging Algorithm for Video Capsule Endoscopy Based on Decorrelated Color Space for Esophageal Cancer: Part II, Detection and Classification of Esophageal Cancer.
Cancers
Esophageal carcinoma (EC) is a prominent contributor to cancer-related mortality since it lacks discernible features in its first phases. Multiple studies have shown that narrow-band imaging (NBI) has superior accuracy, sensitivity, and specificity in detecting EC compared to white light imaging (WLI). Thus, this study innovatively employs a color space linked to décor to transform WLIs into NBIs, offering a novel approach to enhance the detection capabilities of EC in its early stages. In this study a total of 3415 WLI along with the corresponding 3415 simulated NBI images were used for analysis combined with the YOLOv5 algorithm to train the WLI images and the NBI images individually showcasing the adaptability of advanced object detection techniques in the context of medical image analysis. The evaluation of the model's performance was based on the produced confusion matrix and five key metrics: precision, recall, specificity, accuracy, and F1-score of the trained model. The model underwent training to accurately identify three specific manifestations of EC, namely dysplasia, squamous cell carcinoma (SCC), and polyps demonstrates a nuanced and targeted analysis, addressing diverse aspects of EC pathology for a more comprehensive understanding. The NBI model effectively enhanced both its recall and accuracy rates in detecting dysplasia cancer, a pre-cancerous stage that might improve the overall five-year survival rate. Conversely, the SCC category decreased its accuracy and recall rate, although the NBI and WLI models performed similarly in recognizing the polyp. The NBI model demonstrated an accuracy of 0.60, 0.81, and 0.66 in the dysplasia, SCC, and polyp categories, respectively. Additionally, it attained a recall rate of 0.40, 0.73, and 0.76 in the same categories. The WLI model demonstrated an accuracy of 0.56, 0.99, and 0.65 in the dysplasia, SCC, and polyp categories, respectively. Additionally, it obtained a recall rate of 0.39, 0.86, and 0.78 in the same categories, respectively. The limited number of training photos is the reason for the suboptimal performance of the NBI model which can be improved by increasing the dataset.
10.3390/cancers16030572
Artificial Intelligence-Assisted Endoscopic Diagnosis of Early Upper Gastrointestinal Cancer: A Systematic Review and Meta-Analysis.
Frontiers in oncology
Objective:The aim of this study was to assess the diagnostic ability of artificial intelligence (AI) in the detection of early upper gastrointestinal cancer (EUGIC) using endoscopic images. Methods:Databases were searched for studies on AI-assisted diagnosis of EUGIC using endoscopic images. The pooled area under the curve (AUC), sensitivity, specificity, positive likelihood ratio (PLR), negative likelihood ratio (NLR), and diagnostic odds ratio (DOR) with 95% confidence interval (CI) were calculated. Results:Overall, 34 studies were included in our final analysis. Among the 17 image-based studies investigating early esophageal cancer (EEC) detection, the pooled AUC, sensitivity, specificity, PLR, NLR, and DOR were 0.98, 0.95 (95% CI, 0.95-0.96), 0.95 (95% CI, 0.94-0.95), 10.76 (95% CI, 7.33-15.79), 0.07 (95% CI, 0.04-0.11), and 173.93 (95% CI, 81.79-369.83), respectively. Among the seven patient-based studies investigating EEC detection, the pooled AUC, sensitivity, specificity, PLR, NLR, and DOR were 0.98, 0.94 (95% CI, 0.91-0.96), 0.90 (95% CI, 0.88-0.92), 6.14 (95% CI, 2.06-18.30), 0.07 (95% CI, 0.04-0.11), and 69.13 (95% CI, 14.73-324.45), respectively. Among the 15 image-based studies investigating early gastric cancer (EGC) detection, the pooled AUC, sensitivity, specificity, PLR, NLR, and DOR were 0.94, 0.87 (95% CI, 0.87-0.88), 0.88 (95% CI, 0.87-0.88), 7.20 (95% CI, 4.32-12.00), 0.14 (95% CI, 0.09-0.23), and 48.77 (95% CI, 24.98-95.19), respectively. Conclusions:On the basis of our meta-analysis, AI exhibited high accuracy in diagnosis of EUGIC. Systematic Review Registration:https://www.crd.york.ac.uk/PROSPERO/, identifier PROSPERO (CRD42021270443).
10.3389/fonc.2022.855175
Deep learning enhanced the diagnostic merit of serum glycome for multiple cancers.
iScience
Protein glycosylation is associated with the pathogenesis of various cancers. The utilization of certain glycans in cancer diagnosis models holds promise, yet their accuracy is not always guaranteed. Here, we investigated the utility of deep learning techniques, specifically random forests combined with transfer learning, in enhancing serum glycome's discriminative power for cancer diagnosis (including ovarian cancer, non-small cell lung cancer, gastric cancer, and esophageal cancer). We started with ovarian cancer and demonstrated that transfer learning can achieve superior performance in data-disadvantaged cohorts (AUROC >0.9), outperforming the approach of PLS-DA. We identified a serum glycan-biomarker panel including 18 serum N-glycans and 4 glycan derived traits, most of which were featured with sialylation. Furthermore, we validated advantage of the transfer learning scheme across other cancer groups. These findings highlighted the superiority of transfer learning in improving the performance of glycans-based cancer diagnosis model and identifying cancer biomarkers, providing a new high-fidelity cancer diagnosis venue.
10.1016/j.isci.2023.108715
Magnetic Resonance Imaging of the Gastrointestinal Tract: Current Role, Recent Advancements and Future Prospectives.
Diagnostics (Basel, Switzerland)
This review focuses on the role of magnetic resonance imaging (MRI) in the evaluation of the gastrointestinal tract (GI MRI), analyzing the major technical advances achieved in this field, such as diffusion-weighted imaging, molecular imaging, motility studies, and artificial intelligence. Today, MRI performed with the more advanced imaging techniques allows accurate assessment of many bowel diseases, particularly inflammatory bowel disease and rectal cancer; in most of these diseases, MRI is invaluable for diagnosis, staging, and disease monitoring under treatment. Several MRI parameters are currently considered activity biomarkers for inflammation and neoplastic disease. Furthermore, in younger patients with acute or chronic GI disease, MRI can be safely used for short-term follow-up studies in many critical clinical situations because it is radiation-free. MRI assessment of functional gastro-esophageal and small bowel disorders is still in its infancy but very promising, while it is well established and widely used for dynamic assessment of anorectal and pelvic floor dysfunction; MRI motility biomarkers have also been described. There are still some limitations to GI MRI related to high cost and limited accessibility. However, technical advances are expected, such as faster sequences, more specific intestinal contrast agents, AI analysis of MRI data, and possibly increased accessibility to GI MRI studies. Clinical interest in the evaluation of bowel disease using MRI is already very high, but is expected to increase significantly in the coming years.
10.3390/diagnostics13142410
Clinical evaluation of deep learning-based automatic clinical target volume segmentation: a single-institution multi-site tumor experience.
La Radiologia medica
PURPOSE:The large variability in tumor appearance and shape makes manual delineation of the clinical target volume (CTV) time-consuming, and the results depend on the oncologists' experience. Whereas deep learning techniques have allowed oncologists to automate the CTV delineation, multi-site tumor analysis is often lacking in the literature. This study aimed to evaluate the deep learning models that automatically contour CTVs of tumors at various sites on computed tomography (CT) images from objective and subjective perspectives. METHODS AND MATERIALS:577 patients were selected for the present study, including nasopharyngeal (n = 34), esophageal (n = 40), breast-conserving surgery (BCS) (left-sided, n = 71; right-sided, n = 71), breast-radical mastectomy (BRM) (left-sided, n = 43; right-sided, n = 37), cervical (radical radiotherapy, n = 45; postoperative, n = 85), prostate (n = 42), and rectal (n = 109) carcinomas. Manually delineated CTV contours by radiation oncologists are served as ground truth. Four models were evaluated: Flexnet, Unet, Vnet, and Segresnet, which are commercially available in the medical product "AccuLearning AI model training platform". The data were divided into the training, validation, and testing set at a ratio of 5:1:4. The geometric metrics, including Dice Similarity Coefficient (DSC) and Hausdorff Distance (HD), were calculated for objective evaluation. For subjective assessment, oncologists rated the segmentation contours of the testing set visually. RESULTS:High correlations were observed between automatic and manual contours. Based on the results of the independent test group, most of the patients achieved satisfactory quantitative results (DSC > 0.8), except for patients with esophageal carcinoma (DSC: 0.62-0.64). The subjective review indicated that 82.65% of predicted CTVs scored either as clinically accepting (8.68%) or requiring minor revision (73.97%), and no patients were scored as rejected. CONCLUSION:This experimental work demonstrated that auto-generated contours could serve as an initial template to help oncologists save time in CTV delineation. The deep learning-based auto-segmentations achieve acceptable accuracy and show the potential to improve clinical efficiency for radiotherapy of a variety of cancer.
10.1007/s11547-023-01690-x
Automatic detection of squamous cell carcinoma metastasis in esophageal lymph nodes using semantic segmentation.
Pan Yi,Sun Zhuo,Wang Wenmiao,Yang Zhaoyang,Jia Jia,Feng Xiaolong,Wang Yaxi,Fang Qing,Li Jiangtao,Dai Hongtian,Ku Calvin,Wang Shuhao,Liu Cancheng,Xue Liyan,Lyu Ning,Zou Shuangmei
Clinical and translational medicine
Esophageal squamous cell carcinoma (ESCC) is more prevalent than esophageal adenocarcinoma in Asia, especially in China, where more than half of ESCC cases occur worldwide. Many studies have reported that the automatic detection of lymph node metastasis using semantic segmentation shows good performance in breast cancer and other adenocarcinomas. However, the detection of squamous cell carcinoma metastasis in hematoxylin-eosin (H&E)-stained slides has never been reported. We collected a training set of 110 esophageal lymph node slides with metastasis and 132 lymph node slides without metastasis. An iPad-based annotation system was used to draw the contours of the cancer metastasis region. A DeepLab v3 model was trained to achieve the best fit with the training data. The learned model could estimate the probability of metastasis. To evaluate the effectiveness of the detection model of learned metastasis, we used another large cohort of clinical H&E-stained esophageal lymph node slides containing 795 esophageal lymph nodes from 154 esophageal cancer patients. The basic authenticity label for each slide was confirmed by experienced pathologists. After filtering isolated noise in the prediction, we obtained an accuracy of 94%. Furthermore, we applied the learned model to throat and lung lymph node squamous cell carcinoma metastases and achieved the following promising results: an accuracy of 96.7% in throat cancer and an accuracy of 90% in lung cancer. In this work, we organized an annotated dataset of H&E-stained esophageal lymph node and trained a deep neural network to detect lymph node metastasis in H&E-stained slides of squamous cell carcinoma automatically. Moreover, it is possible to use this model to detect lymph nodes metastasis in squamous cell carcinoma from other organs. This study directly demonstrates the potential for determining the localization of squamous cell carcinoma metastases in lymph node and assisting in pathological diagnosis.
10.1002/ctm2.129
A Radiotherapy Dose Map-Guided Deep Learning Method for Predicting Pathological Complete Response in Esophageal Cancer Patients after Neoadjuvant Chemoradiotherapy Followed by Surgery.
Biomedicines
Esophageal cancer is a deadly disease, and neoadjuvant chemoradiotherapy can improve patient survival, particularly for patients achieving a pathological complete response (ypCR). However, existing imaging methods struggle to accurately predict ypCR. This study explores computer-aided detection methods, considering both imaging data and radiotherapy dose variations to enhance prediction accuracy. It involved patients with node-positive esophageal squamous cell carcinoma undergoing neoadjuvant chemoradiotherapy and surgery, with data collected from 2014 to 2017, randomly split into five subsets for 5-fold cross-validation. The algorithm DCRNet, an advanced version of OCRNet, integrates RT dose distribution into dose contextual representations (DCR), combining dose and pixel representation with ten soft regions. Among the 80 enrolled patients (mean age 55.68 years, primarily male, with stage III disease and middle-part lesions), the ypCR rate was 28.75%, showing no significant demographic or disease differences between the ypCR and non-ypCR groups. Among the three summarization methods, the maximum value across the CTV method produced the best results with an AUC of 0.928. The HRNetV2p model with DCR performed the best among the four backbone models tested, with an AUC of 0.928 (95% CI, 0.884-0.972) based on 5-fold cross-validation, showing significant improvement compared to other models. This underscores DCR-equipped models' superior AUC outcomes. The study highlights the potential of dose-guided deep learning in ypCR prediction, necessitating larger, multicenter studies to validate the results.
10.3390/biomedicines11113072
Applications and Prospects of Artificial Intelligence-Assisted Endoscopic Ultrasound in Digestive System Diseases.
Diagnostics (Basel, Switzerland)
Endoscopic ultrasound (EUS) has emerged as a widely utilized tool in the diagnosis of digestive diseases. In recent years, the potential of artificial intelligence (AI) in healthcare has been gradually recognized, and its superiority in the field of EUS is becoming apparent. Machine learning (ML) and deep learning (DL) are the two main AI algorithms. This paper aims to outline the applications and prospects of artificial intelligence-assisted endoscopic ultrasound (EUS-AI) in digestive diseases over the past decade. The results demonstrated that EUS-AI has shown superiority or at least equivalence to traditional methods in the diagnosis, prognosis, and quality control of subepithelial lesions, early esophageal cancer, early gastric cancer, and pancreatic diseases including pancreatic cystic lesions, autoimmune pancreatitis, and pancreatic cancer. The implementation of EUS-AI has opened up new avenues for individualized precision medicine and has introduced novel diagnostic and treatment approaches for digestive diseases.
10.3390/diagnostics13172815
Deep Learning for Automatic Gross Tumor Volumes Contouring in Esophageal Cancer Based on Contrast-Enhanced Computed Tomography Images: A Multi-Institutional Study.
International journal of radiation oncology, biology, physics
PURPOSE:To develop and externally validate an automatic artificial intelligence (AI) tool for delineating gross tumor volume (GTV) in patients with esophageal squamous cell carcinoma (ESCC), which can assist in neo-adjuvant or radical radiation therapy treatment planning. METHODS AND MATERIALS:In this multi-institutional study, contrast-enhanced CT images from 580 eligible ESCC patients were retrospectively collected. The GTV contours delineated by 2 experts via consensus were used as ground truth. A 3-dimensional deep learning model was developed for GTV contouring in the training cohort and internally and externally validated in 3 validation cohorts. The AI tool was compared against 12 board-certified experts in 25 patients randomly selected from the external validation cohort to evaluate its assistance in improving contouring performance and reducing variation. Contouring performance was measured using dice similarity coefficient (DSC) and average surface distance. Additionally, our previously established radiomics model for predicting pathologic complete response was used to compare AI-generated and ground truth contours, to assess the potential of the AI contouring tool in radiomics analysis. RESULTS:The AI tool demonstrated good GTV contouring performance in multicenter validation cohorts, with median DSC values of 0.865, 0.876, and 0.866 and median average surface distance values of 0.939, 0.789, and 0.875 mm, respectively. Furthermore, the AI tool significantly improved contouring performance for half of 12 board-certified experts (DSC values, 0.794-0.835 vs 0.856-0.881, P = .003-0.048), reduced the intra- and interobserver variations by 37.4% and 55.2%, respectively, and saved contouring time by 77.6%. In the radiomics analysis, 88.7% of radiomic features from ground truth and AI-generated contours demonstrated stable reproducibility, and similar pathologic complete response prediction performance for these contours (P = .430) was observed. CONCLUSIONS:Our AI contouring tool can improve GTV contouring performance and facilitate radiomics analysis in ESCC patients, which indicates its potential for GTV contouring during radiation therapy treatment planning and radiomics studies.
10.1016/j.ijrobp.2024.02.035
Preparing Well for Esophageal Endoscopic Detection Using a Hybrid Model and Transfer Learning.
Cancers
Early detection of esophageal cancer through endoscopic imaging is pivotal for effective treatment. However, the intricacies of endoscopic diagnosis, contingent on the physician's expertise, pose challenges. Esophageal cancer features often manifest ambiguously, leading to potential confusions with other inflammatory esophageal conditions, thereby complicating diagnostic accuracy. In recent times, computer-aided diagnosis has emerged as a promising solution in medical imaging, particularly within the domain of endoscopy. Nonetheless, contemporary AI-based diagnostic models heavily rely on voluminous data sources, limiting their applicability, especially in scenarios with scarce datasets. To address this limitation, our study introduces novel data training strategies based on transfer learning, tailored to optimize performance with limited data. Additionally, we propose a hybrid model integrating EfficientNet and Vision Transformer networks to enhance prediction accuracy. Conducting rigorous evaluations on a carefully curated dataset comprising 1002 endoscopic images (comprising 650 white-light images and 352 narrow-band images), our model achieved exceptional outcomes. Our combined model achieved an accuracy of 96.32%, precision of 96.44%, recall of 95.70%, and f1-score of 96.04%, surpassing state-of-the-art models and individual components, substantiating its potential for precise medical image classification. The AI-based medical image prediction platform presents several advantageous characteristics, encompassing superior prediction accuracy, a compact model size, and adaptability to low-data scenarios. This research heralds a significant stride in the advancement of computer-aided endoscopic imaging for improved esophageal cancer diagnosis.
10.3390/cancers15153783
Novel milestones for early esophageal carcinoma: From bench to bed.
World journal of gastrointestinal oncology
Esophageal cancer (EC) is the seventh most common cancer worldwide, and esophageal squamous cell carcinoma (ESCC) accounts for the majority of cases of EC. To effectively diagnose and treat ESCC and improve patient prognosis, timely diagnosis in the initial phase of the illness is necessary. This article offers a detailed summary of the latest advancements and emerging technologies in the timely identification of ECs. Molecular biology and epigenetics approaches involve the use of molecular mechanisms combined with fluorescence quantitative polymerase chain reaction (qPCR), high-throughput sequencing technology (next-generation sequencing), and digital PCR technology to study endogenous or exogenous biomolecular changes in the human body and provide a decision-making basis for the diagnosis, treatment, and prognosis of diseases. The investigation of the microbiome is a swiftly progressing area in human cancer research, and microorganisms with complex functions are potential components of the tumor microenvironment. The intratumoral microbiota was also found to be connected to tumor progression. The application of endoscopy as a crucial technique for the early identification of ESCC has been essential, and with ongoing advancements in technology, endoscopy has continuously improved. With the advancement of artificial intelligence (AI) technology, the utilization of AI in the detection of gastrointestinal tumors has become increasingly prevalent. The implementation of AI can effectively resolve the discrepancies among observers, improve the detection rate, assist in predicting the depth of invasion and differentiation status, guide the pericancerous margins, and aid in a more accurate diagnosis of ESCC.
10.4251/wjgo.v16.i4.1104
Artificial Intelligence in the Management of Barrett's Esophagus and Early Esophageal Adenocarcinoma.
Cancers
Esophageal adenocarcinoma is increasing in incidence and is the most common subtype of esophageal cancer in Western societies. The stepwise progression of Barrett´s metaplasia to high-grade dysplasia and invasive adenocarcinoma provides an opportunity for screening and surveillance. There are important unresolved issues, which include (i) refining the definition of the screening population in order to avoid unnecessary invasive diagnostics, (ii) a more precise prediction of the (very heterogeneous) individual progression risk from metaplasia to invasive cancer in order to better tailor surveillance recommendations, (iii) improvement of the quality of endoscopy in order to reduce the high miss rate for early neoplastic lesions, and (iv) support for the diagnosis of tumor infiltration depth in order to guide treatment decisions. Artificial intelligence (AI) systems might be useful as a support to better solve the above-mentioned issues.
10.3390/cancers14081918
Identification of Early Esophageal Cancer by Semantic Segmentation.
Journal of personalized medicine
Early detection of esophageal cancer has always been difficult, thereby reducing the overall five-year survival rate of patients. In this study, semantic segmentation was used to predict and label esophageal cancer in its early stages. U-Net was used as the basic artificial neural network along with Resnet to extract feature maps that will classify and predict the location of esophageal cancer. A total of 75 white-light images (WLI) and 90 narrow-band images (NBI) were used. These images were classified into three categories: normal, dysplasia, and squamous cell carcinoma. After labeling, the data were divided into a training set, verification set, and test set. The training set was approved by the encoder-decoder model to train the prediction model. Research results show that the average time of 111 ms is used to predict each image in the test set, and the evaluation method is calculated in pixel units. Sensitivity is measured based on the severity of the cancer. In addition, NBI has higher accuracy of 84.724% when compared with the 82.377% accuracy rate of WLI, thereby making it a suitable method to detect esophageal cancer using the algorithm developed in this study.
10.3390/jpm12081204
The current state of artificial intelligence in endoscopic diagnosis of early esophageal squamous cell carcinoma.
Frontiers in oncology
Esophageal squamous cell carcinoma (ESCC) is a common malignant tumor of the digestive tract. The most effective method of reducing the disease burden in areas with a high incidence of esophageal cancer is to prevent the disease from developing into invasive cancer through screening. Endoscopic screening is key for the early diagnosis and treatment of ESCC. However, due to the uneven professional level of endoscopists, there are still many missed cases because of failure to recognize lesions. In recent years, along with remarkable progress in medical imaging and video evaluation technology based on deep machine learning, the development of artificial intelligence (AI) is expected to provide new auxiliary methods of endoscopic diagnosis and the treatment of early ESCC. The convolution neural network (CNN) in the deep learning model extracts the key features of the input image data using continuous convolution layers and then classifies images through full-layer connections. The CNN is widely used in medical image classification, and greatly improves the accuracy of endoscopic image classification. This review focuses on the AI-assisted diagnosis of early ESCC and prediction of early ESCC invasion depth under multiple imaging modalities. The excellent image recognition ability of AI is suitable for the detection and diagnosis of ESCC and can reduce missed diagnoses and help endoscopists better complete endoscopic examinations. However, the selective bias used in the training dataset of the AI system affects its general utility.
10.3389/fonc.2023.1198941
Endoscopic Evaluation of Pathological Complete Response Using Deep Neural Network in Esophageal Cancer Patients Who Received Neoadjuvant Chemotherapy-Multicenter Retrospective Study from Four Japanese Esophageal Centers.
Annals of surgical oncology
BACKGROUND:Detecting pathological complete response (pCR) before surgery would facilitate nonsurgical approach after neoadjuvant chemotherapy (NAC). We developed an artificial intelligence (AI)-guided pCR evaluation using a deep neural network to identify pCR before surgery. METHODS:This study examined resectable esophageal squamous cell carcinoma (ESCC) patients who underwent esophagectomy after NAC. The same number of histological responders without pCR and non-responders were randomly selected based on the number of pCR patients. Endoscopic images were analyzed using a deep neural network. A test dataset consisting of 20 photos was used for validation. The sensitivity, specificity, positive predictive value (PPV), and negative predictive value (NPV) of AI and four experienced endoscopists' pCR evaluations were calculated. For pathological response evaluation, Japanese Classification of Esophageal Cancer was used. RESULTS:The study enrolled 123 patients, including 41 patients with pCR, the same number of histological responders without pCR, and non-responders [grade 0, 5 (4%); grade 1a, 36 (30%); grade 1b, 21 (17%); grade 2, 20 (16%); grade 3, 41 (33%)]. In 20 models, the median values of sensitivity, specificity, PPV, NPV, and accuracy for endoscopic response (ER) detection were 60%, 81%, 77%, 67%, and 70%, respectively. Similarly, the endoscopists' median of these was 43%, 90%, 85%, 65%, and 66%, respectively. CONCLUSIONS:This proof-of-concept study demonstrated that the AI-guided endoscopic response evaluation after NAC could identify pCR with moderate accuracy. The current AI algorithm might guide an individualized treatment strategy including nonsurgical approach in ESCC patients through prospective studies with careful external validation to demonstrate the clinical value of this diagnostic approach including primary tumor and lymph node.
10.1245/s10434-023-13862-0
Automated artificial intelligence-based phase-recognition system for esophageal endoscopic submucosal dissection (with video).
Gastrointestinal endoscopy
BACKGROUND AND AIMS:Endoscopic submucosal dissection (ESD) for superficial esophageal cancer is a multistep treatment involving several endoscopic processes. Although analyzing each phase separately is worthwhile, it is not realistic in practice owing to the need for considerable manpower. To solve this problem, we aimed to establish a state-of-the-art artificial intelligence (AI)-based system, specifically, an automated phase-recognition system that can automatically identify each endoscopic phase based on video images. METHODS:Ninety-four videos of ESD procedures for superficial esophageal cancer were evaluated in this single-center study. A deep neural network-based phase-recognition system was developed in an automated manner to recognize each of the endoscopic phases. The system was trained with the use of videos that were annotated and verified by 2 GI endoscopists. RESULTS:The overall accuracy of the AI model for automated phase recognition was 90%, and the average precision, recall, and F value rates were 91%, 90%, and 90%, respectively. Two representative ESD videos predicted by the model indicated the usability of AI in clinical practice. CONCLUSIONS:We demonstrated that an AI-based automated phase-recognition system for esophageal ESD can be established with high accuracy. To the best of our knowledge, this is the first report on automated recognition of ESD treatment phases. Because this system enabled a detailed analysis of phases, collecting large volumes of data in the future may help to identify quality indicators for treatment techniques and uncover unmet medical needs that necessitate the creation of new treatment methods and devices.
10.1016/j.gie.2023.12.037
The use of an artificial intelligence algorithm for circulating tumor cell detection in patients with esophageal cancer.
Oncology letters
Despite recent advances in multidisciplinary treatments of esophageal squamous cell carcinoma (ESCC), patients frequently suffer from distant metastasis after surgery. For numerous types of cancer, circulating tumor cells (CTCs) are considered predictors of distant metastasis, therapeutic response and prognosis. However, as more markers of cytopathological heterogeneity are discovered, the overall detection process for the expression of these markers in CTCs becomes increasingly complex and time consuming. In the present study, the use of a convolutional neural network (CNN)-based artificial intelligence (AI) for CTC detection was assessed using KYSE ESCC cell lines and blood samples from patients with ESCC. The AI algorithm distinguished KYSE cells from peripheral blood-derived mononuclear cells (PBMCs) from healthy volunteers, accompanied with epithelial cell adhesion molecule (EpCAM) and nuclear DAPI staining, with an accuracy of >99.8% when the AI was trained on the same KYSE cell line. In addition, AI trained on KYSE520 distinguished KYSE30 from PBMCs with an accuracy of 99.8%, despite the marked differences in EpCAM expression between the two KYSE cell lines. The average accuracy of distinguishing KYSE cells from PBMCs for the AI and four researchers was 100 and 91.8%, respectively (P=0.011). The average time to complete cell classification for 100 images by the AI and researchers was 0.74 and 630.4 sec, respectively (P=0.012). The average number of EpCAM-positive/DAPI-positive cells detected in blood samples by the AI was 44.5 over 10 patients with ESCC and 2.4 over 5 healthy volunteers (P=0.019). These results indicated that the CNN-based image processing algorithm for CTC detection provides a higher accuracy and shorter analysis time compared to humans, suggesting its applicability for clinical use in patients with ESCC. Moreover, the finding that AI accurately identified even EpCAM-negative KYSEs suggested that the AI algorithm may distinguish CTCs based on as yet unknown features, independent of known marker expression.
10.3892/ol.2023.13906
Deep Learning for Automated Contouring of Gross Tumor Volumes in Esophageal Cancer.
Frontiers in oncology
Purpose:The aim of this study was to propose and evaluate a novel three-dimensional (3D) V-Net and two-dimensional (2D) U-Net mixed (VUMix-Net) architecture for a fully automatic and accurate gross tumor volume (GTV) in esophageal cancer (EC)-delineated contours. Methods:We collected the computed tomography (CT) scans of 215 EC patients. 3D V-Net, 2D U-Net, and VUMix-Net were developed and further applied simultaneously to delineate GTVs. The Dice similarity coefficient (DSC) and 95th-percentile Hausdorff distance (95HD) were used as quantitative metrics to evaluate the performance of the three models in ECs from different segments. The CT data of 20 patients were randomly selected as the ground truth (GT) masks, and the corresponding delineation results were generated by artificial intelligence (AI). Score differences between the two groups (GT versus AI) and the evaluation consistency were compared. Results:In all patients, there was a significant difference in the 2D DSCs from U-Net, V-Net, and VUMix-Net (p=0.01). In addition, VUMix-Net showed achieved better 3D-DSC and 95HD values. There was a significant difference among the 3D-DSC (mean ± STD) and 95HD values for upper-, middle-, and lower-segment EC (p<0.001), and the middle EC values were the best. In middle-segment EC, VUMix-Net achieved the highest 2D-DSC values (p<0.001) and lowest 95HD values (p=0.044). Conclusion:The new model (VUMix-Net) showed certain advantages in delineating the GTVs of EC. Additionally, it can generate the GTVs of EC that meet clinical requirements and have the same quality as human-generated contours. The system demonstrated the best performance for the ECs of the middle segment.
10.3389/fonc.2022.892171
Modern Diagnosis of Early Esophageal Cancer: From Blood Biomarkers to Advanced Endoscopy and Artificial Intelligence.
Visaggi Pierfrancesco,Barberio Brigida,Ghisa Matteo,Ribolsi Mentore,Savarino Vincenzo,Fassan Matteo,Valmasoni Michele,Marchi Santino,de Bortoli Nicola,Savarino Edoardo
Cancers
Esophageal cancer (EC) is the seventh most common cancer and the sixth cause of cancer death worldwide. Histologically, esophageal squamous cell carcinoma (ESCC) and esophageal adenocarcinoma (EAC) account for up to 90% and 20% of all ECs, respectively. Clinical symptoms such as dysphagia, odynophagia, and bolus impaction occur late in the natural history of the disease, and the diagnosis is often delayed. The prognosis of ESCC and EAC is poor in advanced stages, being survival rates less than 20% at five years. However, when the diagnosis is achieved early, curative treatment is possible, and survival exceeds 80%. For these reasons, mass screening strategies for EC are highly desirable, and several options are currently under investigation. Blood biomarkers offer an inexpensive, non-invasive screening strategy for cancers, and novel technologies have allowed the identification of candidate markers for EC. The esophagus is easily accessible via endoscopy, and endoscopic imaging represents the gold standard for cancer surveillance. However, lesion recognition during endoscopic procedures is hampered by interobserver variability. To fill this gap, artificial intelligence (AI) has recently been explored and provided encouraging results. In this review, we provide a summary of currently available options to achieve early diagnosis of EC, focusing on blood biomarkers, advanced endoscopy, and AI.
10.3390/cancers13133162
Artificial intelligence in esophageal cancer diagnosis and treatment: where are we now?-a narrative review.
Annals of translational medicine
Background and Objective:Artificial intelligence (AI) use is becoming increasingly prevalent directly or indirectly in daily clinical practice, including esophageal cancer (EC) diagnosis and treatment. Although the limits of its adoption and their clinical benefits are still unknown, any physician related to EC patients' management should be aware of the status and future perspectives of AI use in their field. The purpose of this review is to summarize the existing literature regarding the role of AI in diagnosis and treatment of EC. We have focused on the aids AI entails in the management of this pathology and we have tried to offer an updated perspective to maximize current applications and to identify potential future uses of it. Methods:Data concerning AI applied to EC diagnosis and treatment is not limited, including direct (those specifically related to them) and indirect (those referring to other specialties as radiology or pathology), applications. However, the clinical relevance of the discussed and presented models is still unknown. We performed a research in PubMed of English and Spanish written studies from January 1970 to June 2022. Key Content and Findings:Information regarding the role of AI in EC diagnosis and treatment has increased exponentially in recent years. Several models, including different variables and features have been investigated and some of them internally and externally validated. However, the main challenge remains to apply and introduce all these data into clinical practice, and, as some of the discussed studies argue, if the models are able to enhance experienced endoscopists' judgement. Although AI use is increasing steadily in different medical specialties, the truth is, most of the time, the gap between model development and clinical implementation is not closed. Learning to understand the routinely application of AI, as well as future improvements, would lead to a broadened adoption. Conclusions:Physicians should be aware of the multiple current clinical uses of AI in EC diagnosis and treatment and should take part in their clinical application and future developments to enhance patient care.
10.21037/atm-22-3977
Global research trends of artificial intelligence applied in esophageal carcinoma: A bibliometric analysis (2000-2022) CiteSpace and VOSviewer.
Frontiers in oncology
Objective:Using visual bibliometric analysis, the application and development of artificial intelligence in clinical esophageal cancer are summarized, and the research progress, hotspots, and emerging trends of artificial intelligence are elucidated. Methods:On April 7th, 2022, articles and reviews regarding the application of AI in esophageal cancer, published between 2000 and 2022 were chosen from the Web of Science Core Collection. To conduct co-authorship, co-citation, and co-occurrence analysis of countries, institutions, authors, references, and keywords in this field, VOSviewer (version 1.6.18), CiteSpace (version 5.8.R3), Microsoft Excel 2019, R 4.2, an online bibliometric platform (http://bibliometric.com/) and an online browser plugin (https://www.altmetric.com/) were used. Results:A total of 918 papers were included, with 23,490 citations. 5,979 authors, 39,962 co-cited authors, and 42,992 co-cited papers were identified in the study. Most publications were from China (317). In terms of the H-index (45) and citations (9925), the United States topped the list. The journal "New England Journal of Medicine" of Medicine, General & Internal (IF = 91.25) published the most studies on this topic. The University of Amsterdam had the largest number of publications among all institutions. The past 22 years of research can be broadly divided into two periods. The 2000 to 2016 research period focused on the classification, identification and comparison of esophageal cancer. Recently (2017-2022), the application of artificial intelligence lies in endoscopy, diagnosis, and precision therapy, which have become the frontiers of this field. It is expected that closely esophageal cancer clinical measures based on big data analysis and related to precision will become the research hotspot in the future. Conclusions:An increasing number of scholars are devoted to artificial intelligence-related esophageal cancer research. The research field of artificial intelligence in esophageal cancer has entered a new stage. In the future, there is a need to continue to strengthen cooperation between countries and institutions. Improving the diagnostic accuracy of esophageal imaging, big data-based treatment and prognosis prediction through deep learning technology will be the continuing focus of research. The application of AI in esophageal cancer still has many challenges to overcome before it can be utilized.
10.3389/fonc.2022.972357
The diagnostic accuracy of artificial intelligence in thoracic diseases: A protocol for systematic review and meta-analysis.
Medicine
INTRODUCTION:Thoracic diseases include a variety of common human primary malignant tumors, among which lung cancer and esophageal cancer are among the top 10 in cancer incidence and mortality. Early diagnosis is an important part of cancer treatment, so artificial intelligence (AI) systems have been developed for the accurate and automated detection and diagnosis of thoracic tumors. However, the complicated AI structure and image processing made the diagnosis result of AI-based system unstable. The purpose of this study is to systematically review published evidence to explore the accuracy of AI systems in diagnosing thoracic cancers. METHODS AND ANALYSIS:We will conduct a systematic review and meta-analysis of the diagnostic accuracy of AI systems for the prediction of thoracic diseases. The primary objective is to assess the diagnostic accuracy of thoracic cancers, including assessing potential biases and calculating combined estimates of sensitivity, specificity, and area under the receiver operating characteristic curve (AUC). The secondary objective is to evaluate the factors associated with different models, classifiers, and radiomics information. We will search databases such as PubMed/MEDLINE, Embase (via OVID), and the Cochrane Library. Two reviewers will independently screen titles and abstracts, perform full article reviews and extract study data. We will report study characteristics and assess methodological quality using the Quality Assessment of Diagnostic Accuracy Studies 2 (QUADAS-2) tool. RevMan 5.3 and Meta-disc 1.4 software will be used for data synthesis. If pooling is appropriate, we will produce summary receiver operating characteristic (SROC) curves, summary operating points (pooled sensitivity and specificity), and 95% confidence intervals around the summary operating points. Methodological subgroup and sensitivity analyses will be performed to explore heterogeneity. PROSPERO REGISTRATION NUMBER:CRD42019135247.
10.1097/MD.0000000000019114
The Applications of Artificial Intelligence in Digestive System Neoplasms: A Review.
Health data science
Importance:Digestive system neoplasms (DSNs) are the leading cause of cancer-related mortality with a 5-year survival rate of less than 20%. Subjective evaluation of medical images including endoscopic images, whole slide images, computed tomography images, and magnetic resonance images plays a vital role in the clinical practice of DSNs, but with limited performance and increased workload of radiologists or pathologists. The application of artificial intelligence (AI) in medical image analysis holds promise to augment the visual interpretation of medical images, which could not only automate the complicated evaluation process but also convert medical images into quantitative imaging features that associated with tumor heterogeneity. Highlights:We briefly introduce the methodology of AI for medical image analysis and then review its clinical applications including clinical auxiliary diagnosis, assessment of treatment response, and prognosis prediction on 4 typical DSNs including esophageal cancer, gastric cancer, colorectal cancer, and hepatocellular carcinoma. Conclusion:AI technology has great potential in supporting the clinical diagnosis and treatment decision-making of DSNs. Several technical issues should be overcome before its application into clinical practice of DSNs.
10.34133/hds.0005
Effect of Specimen Processing Technique on Cell Detection and Classification by Artificial Intelligence.
American journal of clinical pathology
OBJECTIVES:Cytomorphology is known to differ depending on the processing technique, and these differences pose a problem for automated diagnosis using deep learning. We examined the as-yet unclarified relationship between cell detection or classification using artificial intelligence (AI) and the AutoSmear (Sakura Finetek Japan) and liquid-based cytology (LBC) processing techniques. METHODS:The "You Only Look Once" (YOLO), version 5x, algorithm was trained on the AutoSmear and LBC preparations of 4 cell lines: lung cancer (LC), cervical cancer (CC), malignant pleural mesothelioma (MM), and esophageal cancer (EC). Detection and classification rates were used to evaluate the accuracy of cell detection. RESULTS:When preparations of the same processing technique were used for training and detection in the 1-cell (1C) model, the AutoSmear model had a higher detection rate than the LBC model. When different processing techniques were used for training and detection, detection rates of LC and CC were significantly lower in the 4-cell (4C) model than in the 1C model, and those of MM and EC were approximately 10% lower in the 4C model. CONCLUSIONS:In AI-based cell detection and classification, attention should be paid to cells whose morphologies change significantly depending on the processing technique, further suggesting the creation of a training model.
10.1093/ajcp/aqac178
Implementation of artificial intelligence in upper gastrointestinal endoscopy.
DEN open
The application of artificial intelligence (AI) using deep learning has significantly expanded in the field of esophagogastric endoscopy. Recent studies have shown promising results in detecting and differentiating early gastric cancer using AI tools built using white light, magnified, or image-enhanced endoscopic images. Some studies have reported the use of AI tools to predict the depth of early gastric cancer based on endoscopic images. Similarly, studies based on using AI for detecting early esophageal cancer have also been reported, with an accuracy comparable to that of endoscopy specialists. Moreover, an AI system, developed to diagnose pharyngeal cancer, has shown promising performance with high sensitivity. These reports suggest that, if introduced for regular use in clinical settings, AI systems can significantly reduce the burden on physicians. This review summarizes the current status of AI applications in the upper gastrointestinal tract and presents directions for clinical practice implementation and future research.
10.1002/deo2.72
Preoperative prediction of clinical and pathological stages for patients with esophageal cancer using PET/CT radiomics.
Insights into imaging
BACKGROUND:Preoperative stratification is critical for the management of patients with esophageal cancer (EC). To investigate the feasibility and accuracy of PET-CT-based radiomics in preoperative prediction of clinical and pathological stages for patients with EC. METHODS:Histologically confirmed 100 EC patients with preoperative PET-CT images were enrolled retrospectively and randomly divided into training and validation cohorts at a ratio of 7:3. The maximum relevance minimum redundancy (mRMR) was applied to select optimal radiomics features from PET, CT, and fused PET-CT images, respectively. Logistic regression (LR) was applied to classify the T stage (T vs. T), lymph node metastasis (LNM) (LNM vs. LNM), and pathological state (pstage) (I-II vs. III-IV) with features from CT (CT_LR_Score), PET (PET_LR_Score), fused PET/CT (Fused_LR_Score), and combined CT and PET features (CT + PET_LR_Score), respectively. RESULTS:Seven, 10, and 7 CT features; 7, 8, and 7 PET features; and 3, 6, and 3 fused PET/CT features were selected using mRMR for the prediction of T stage, LNM, and pstage, respectively. The area under curves (AUCs) for T stage, LNM, and pstage prediction in the validation cohorts were 0.846, 0.756, 0.665, and 0.815; 0.769, 0.760, 0.665, and 0.824; and 0.727, 0.785, 0.689, and 0.837 for models of CT_LR_Score, PET_ LR_Score, Fused_ LR_Score, and CT + PET_ LR_Score, respectively. CONCLUSIONS:Accurate prediction ability was observed with combined PET and CT radiomics in the prediction of T stage, LNM, and pstage for EC patients. CRITICAL RELEVANCE STATEMENT:PET/CT radiomics is feasible and promising to stratify stages for esophageal cancer preoperatively. KEY POINTS:• PET-CT radiomics achieved the best performance for Node and pathological stage prediction. • CT radiomics achieved the best AUC for T stage prediction. • PET-CT radiomics is feasible and promising to stratify stages for EC preoperatively.
10.1186/s13244-023-01528-0
Third Eye? The Assistance of Artificial Intelligence (AI) in the Endoscopy of Gastrointestinal Neoplasms.
Journal of clinical medicine
Gastrointestinal cancers are characterized by high incidence and mortality. However, there are well-established methods of screening. The endoscopy exam provides the macroscopical image and enables harvesting the tissue samples for further histopathological diagnosis. The efficiency of endoscopies relies not only on proper patient preparation, but also on the skills of the personnel conducting the exam. In recent years, a number of reports concerning the application of artificial intelligence (AI) in medicine have arisen. Numerous studies aimed to assess the utility of deep learning/ neural network systems supporting endoscopies. In this review, we summarized the most recent reports and randomized clinical trials regarding the application of AI in screening and surveillance of gastrointestinal cancers among patients suffering from esophageal, gastric, and colorectal cancer, along with the advantages, limitations, and controversies of those novel solutions.
10.3390/jcm12216721
Revolutionizing healthcare by use of artificial intelligence in esophageal carcinoma - a narrative review.
Annals of medicine and surgery (2012)
Esophageal cancer is a major cause of cancer-related mortality worldwide, with significant regional disparities. Early detection of precursor lesions is essential to improve patient outcomes. Artificial intelligence (AI) techniques, including deep learning and machine learning, have proved to be of assistance to both gastroenterologists and pathologists in the diagnosis and characterization of upper gastrointestinal malignancies by correlating with the histopathology. The primary diagnostic method in gastroenterology is white light endoscopic evaluation, but conventional endoscopy is partially inefficient in detecting esophageal cancer. However, other endoscopic modalities, such as narrow-band imaging, endocytoscopy, and endomicroscopy, have shown improved visualization of mucosal structures and vasculature, which provides a set of baseline data to develop efficient AI-assisted predictive models for quick interpretation. The main challenges in managing esophageal cancer are identifying high-risk patients and the disease's poor prognosis. Thus, AI techniques can play a vital role in improving the early detection and diagnosis of precursor lesions, assisting gastroenterologists in performing targeted biopsies and real-time decisions of endoscopic mucosal resection or endoscopic submucosal dissection. Combining AI techniques and endoscopic modalities can enhance the diagnosis and management of esophageal cancer, improving patient outcomes and reducing cancer-related mortality rates. The aim of this review is to grasp a better understanding of the application of AI in the diagnosis, treatment, and prognosis of esophageal cancer and how computer-aided diagnosis and computer-aided detection can act as vital tools for clinicians in the long run.
10.1097/MS9.0000000000001175
Diagnostic Performance of Artificial Intelligence-Based Models for the Detection of Early Esophageal Cancers in Barret's Esophagus: A Meta-Analysis of Patient-Based Studies.
Cureus
Introduction Barret's esophagus (BE) is a precursor of adenocarcinoma of the esophagus. The detection of high-grade dysplasia and adenocarcinoma at an early stage can improve survival but is very challenging. Artificial intelligence (AI)-based models have been claimed to improve diagnostic accuracy. The aim of the current study was to carry out a meta-analysis of papers reporting the results of artificial intelligence-based models used in real-time white light endoscopy of patients with BE to detect early esophageal adenocarcinoma (EEAC). Methods This meta-analysis was registered with the International Prospective Register of Systematic Reviews (PROSPERO; Reg No. CRD42021246148) and its conduction and reporting followed the Preferred Reporting Items for Systematic Review and Meta-Analysis of Diagnostic Test Accuracy (PRISMA-DTA) statement guidelines. All peer-reviewed and preprint original articles that reported the sensitivity and specificity of AI-based models on white light endoscopic imaging as an index test against the standard criterion of histologically proven early oesophageal cancer on the background of Barret's esophagus reported as per-patient analysis were considered for inclusion. There was no restriction on type and year of publication, however, articles published in the English language were searched. The search engines used included Medline, PubMed, EMBASE, EMCARE, AMED, BNI, and HMIC. The search strategy included the following keywords for all search engines: ("Esophageal Cancer" OR "Esophageal Neoplasms" OR " Oesophageal Cancer" OR "Oesophageal Neoplasms" OR "Barrett's Esophagus" OR "Barrett's Oesophagus") And ("Artificial Intelligence" OR "Deep Learning" OR "Machine Learning" OR "Convolutional Network"). This search was conducted on November 30, 2020. Duplicate studies were excluded. Studies that reported more than one dataset per patient for the diagnostic accuracy of the AI-based model were included twice. Quantitative and qualitative data, including first author, year of publication, true positives (TP), false negatives (FN), false positives (FP), true negatives (TN), the threshold of the index test, and country where the study was conducted, were extracted using a data extraction sheet. The Quality Appraisal for Diverse Studies 2 (QUADS-2) tool was used to assess the quality of each study. Data were analyzed using MetaDTA, interactive online software for meta-analysis of diagnostic studies. The diagnostic performance of the meta-analysis was assessed by a summary receiver operating characteristics (sROC) plot. A meta-analysis tree was constructed using MetaDTA software to determine the effect of cumulative sensitivity and specificity on surveillance of patients with BE in terms of miss rate and overdiagnosis. Results The literature search revealed 171 relevant records. After removing duplicates, 117 records were screened. Full-text articles of 28 studies were assessed for eligibility. Only three studies reporting four datasets met the inclusion criteria. The summary sensitivity and specificity of AI-based models were 0.90 (95% CI, 0.83- 0.944) and 0.86 (95% CI, 0.781-0.91), respectively. The area under the curve for all the available evidence was 0.88. Conclusion Collective evidence for the routine usage of AI-based models in the detection of EEAC is encouraging but is limited by the low number of studies. Further prospective studies reporting the patient-based diagnostic accuracy of such models are required.
10.7759/cureus.15447
Advanced imaging and artificial intelligence for Barrett's esophagus: What we should and soon will do.
World journal of gastroenterology
Barrett's esophagus (BE) is a well-established risk factor for esophageal adenocarcinoma. It is recommended that patients have regular endoscopic surveillance, with the ultimate goal of detecting early-stage neoplastic lesions before they can progress to invasive carcinoma. Detection of both dysplasia or early adenocarcinoma permits curative endoscopic treatments, and with this aim, thorough endoscopic assessment is crucial and improves outcomes. The burden of missed neoplasia in BE is still far from being negligible, likely due to inappropriate endoscopic surveillance. Over the last two decades, advanced imaging techniques, moving from traditional dye-spray chromoendoscopy to more practical virtual chromoendoscopy technologies, have been introduced with the aim to enhance neoplasia detection in BE. As witnessed in other fields, artificial intelligence (AI) has revolutionized the field of diagnostic endoscopy and is set to cover a pivotal role in BE as well. The aim of this commentary is to comprehensively summarize present evidence, recent research advances, and future perspectives regarding advanced imaging technology and AI in BE; the combination of computer-aided diagnosis to a widespread adoption of advanced imaging technologies is eagerly awaited. It will also provide a useful step-by-step approach for performing high-quality endoscopy in BE, in order to increase the diagnostic yield of endoscopy in clinical practice.
10.3748/wjg.v28.i11.1113
Use of the Electronic Health Record to Target Patients for Non-endoscopic Barrett's Esophagus Screening.
Digestive diseases and sciences
BACKGROUND:Clinical prediction models targeting patients for Barrett's esophagus (BE) screening include data obtained by interview, questionnaire, and body measurements. A tool based on electronic health records (EHR) data could reduce cost and enhance usability, particularly if combined with non-endoscopic BE screening methods. AIMS:To determine whether EHR-based data can identify BE patients. METHODS:We performed a retrospective review of patients ages 50-75 who underwent a first-time esophagogastroduodenoscopy. Data extracted from the EHR included demographics and BE risk factors. Endoscopy and pathology reports were reviewed for histologically confirmed BE. Screening criteria modified from clinical guidelines were assessed for association with BE. Subsequently, a score based on multivariate logistic regression was developed and assessed for its ability to identify BE subjects. RESULTS:A total of 2931 patients were assessed, and BE was found in 1.9%. Subjects who met screening criteria were more likely to have BE (3.3% vs. 1.1%, p = 0.001), and the criteria predicted BE with an AUROC of 0.65 (95% CI 0.59-0.71). A score based on logistic regression modeling included gastroesophageal reflux disease, sex, body mass index, and ever-smoker status and identified BE subjects with an AUROC of 0.71 (95% CI 0.64-0.77). Both prediction tools produced higher AUROCs in women than in men. CONCLUSIONS:EHR-based BE risk prediction tools identify BE patients with fair accuracy. While these tools may improve the efficiency of patient targeting for BE screening in the primary care setting, challenges remain to identify high-risk patients for non-invasive BE screening in clinical practice.
10.1007/s10620-019-05707-2
Encoding LC-MS-Based Untargeted Metabolomics Data into Images toward AI-Based Clinical Diagnosis.
Analytical chemistry
Liquid chromatography-mass spectrometry (LC-MS)-based untargeted metabolomics provides comprehensive and quantitative profiling of metabolites in clinical investigations. The use of whole metabolome profiles is a promising strategy for disease diagnosis but technically challenging. Here, we developed an approach, namely MetImage, to encode LC-MS-based untargeted metabolomics data into multi-channel digital images. Then, the images that represent the comprehensive metabolome profiles can be employed for developing deep learning-based AI models toward clinical diagnosis. In this work, we demonstrated the application of MetImage for clinical screening of esophageal squamous cell carcinoma (ESCC) in a clinical cohort with 1104 participants. A convolutional neuronal network-based AI model was trained to distinguish ESCC screening positive and negative subjects using their serum metabolomics data. Superior performances such as sensitivity (85%), specificity (92%), and area under curve (0.95) were validated in an independent testing cohort ( = 442). Importantly, we demonstrated that our AI-based ESCC screening model is not a "black box". The encoded images reserved the characteristics of mass spectra from the raw LC-MS data; therefore, metabolite identifications in key image features were readily achieved. Altogether, MetImage is a unique approach that encodes raw LC-MS-based untargeted metabolomics data into images and facilitates the utilization of whole metabolome profiles for AI-based clinical applications with improved interpretability.
10.1021/acs.analchem.2c05079
Use of a convolutional neural network for classifying microvessels of superficial esophageal squamous cell carcinomas.
Uema Ryotaro,Hayashi Yoshito,Tashiro Taku,Saiki Hirotsugu,Kato Minoru,Amano Takahiro,Tani Mizuki,Yoshihara Takeo,Inoue Takanori,Kimura Keiichi,Iwatani Shuko,Sakatani Akihiko,Yoshii Shunsuke,Tsujii Yoshiki,Shinzaki Shinichiro,Iijima Hideki,Takehara Tetsuo
Journal of gastroenterology and hepatology
BACKGROUND AND AIM:The morphological diagnosis of microvessels on the surface of superficial esophageal squamous cell carcinomas using magnifying endoscopy with narrow-band imaging is widely used in clinical practice. Nevertheless, inconsistency, even among experts, remains a problem. We constructed a convolutional neural network-based computer-aided diagnosis system to classify the microvessels of superficial esophageal squamous cell carcinomas and evaluated its diagnostic performance. METHODS:In this retrospective study, a cropped magnifying endoscopy with narrow-band images from superficial esophageal squamous cell carcinoma lesions was used as the dataset. All images were assessed by three experts, and classified into three classes, Type B1, B2, and B3, based on the Japan Esophagus Society classification. The dataset was divided into training and validation datasets. A convolutional neural network model (ResNeXt-101) was trained and tuned with the training dataset. To evaluate diagnostic accuracy, the validation dataset was assessed by the computer-aided diagnosis system and eight endoscopists. RESULTS:In total, 1777 and 747 cropped images (total, 393 lesions) were included in the training and validation datasets, respectively. The diagnosis system took 20.3 s to evaluate the 747 images in the validation dataset. The microvessel classification accuracy of the computer-aided diagnosis system was 84.2%, which was higher than the average of the eight endoscopists (77.8%, P < 0.001). The area under the receiver operating characteristic curves for diagnosing Type B1, B2, and B3 vessels were 0.969, 0.948, and 0.973, respectively. CONCLUSIONS:The computer-aided diagnosis system showed remarkable performance in the classification of microvessels on superficial esophageal squamous cell carcinomas.
10.1111/jgh.15479
A deep learning system for detection of early Barrett's neoplasia: a model development and validation study.
The Lancet. Digital health
BACKGROUND:Computer-aided detection (CADe) systems could assist endoscopists in detecting early neoplasia in Barrett's oesophagus, which could be difficult to detect in endoscopic images. The aim of this study was to develop, test, and benchmark a CADe system for early neoplasia in Barrett's oesophagus. METHODS:The CADe system was first pretrained with ImageNet followed by domain-specific pretraining with GastroNet. We trained the CADe system on a dataset of 14 046 images (2506 patients) of confirmed Barrett's oesophagus neoplasia and non-dysplastic Barrett's oesophagus from 15 centres. Neoplasia was delineated by 14 Barrett's oesophagus experts for all datasets. We tested the performance of the CADe system on two independent test sets. The all-comers test set comprised 327 (73 patients) non-dysplastic Barrett's oesophagus images, 82 (46 patients) neoplastic images, 180 (66 of the same patients) non-dysplastic Barrett's oesophagus videos, and 71 (45 of the same patients) neoplastic videos. The benchmarking test set comprised 100 (50 patients) neoplastic images, 300 (125 patients) non-dysplastic images, 47 (47 of the same patients) neoplastic videos, and 141 (82 of the same patients) non-dysplastic videos, and was enriched with subtle neoplasia cases. The benchmarking test set was evaluated by 112 endoscopists from six countries (first without CADe and, after 6 weeks, with CADe) and by 28 external international Barrett's oesophagus experts. The primary outcome was the sensitivity of Barrett's neoplasia detection by general endoscopists without CADe assistance versus with CADe assistance on the benchmarking test set. We compared sensitivity using a mixed-effects logistic regression model with conditional odds ratios (ORs; likelihood profile 95% CIs). FINDINGS:Sensitivity for neoplasia detection among endoscopists increased from 74% to 88% with CADe assistance (OR 2·04; 95% CI 1·73-2·42; p<0·0001 for images and from 67% to 79% [2·35; 1·90-2·94; p<0·0001] for video) without compromising specificity (from 89% to 90% [1·07; 0·96-1·19; p=0·20] for images and from 96% to 94% [0·94; 0·79-1·11; ] for video; p=0·46). In the all-comers test set, CADe detected neoplastic lesions in 95% (88-98) of images and 97% (90-99) of videos. In the benchmarking test set, the CADe system was superior to endoscopists in detecting neoplasia (90% vs 74% [OR 3·75; 95% CI 1·93-8·05; p=0·0002] for images and 91% vs 67% [11·68; 3·85-47·53; p<0·0001] for video) and non-inferior to Barrett's oesophagus experts (90% vs 87% [OR 1·74; 95% CI 0·83-3·65] for images and 91% vs 86% [2·94; 0·99-11·40] for video). INTERPRETATION:CADe outperformed endoscopists in detecting Barrett's oesophagus neoplasia and, when used as an assistive tool, it improved their detection rate. CADe detected virtually all neoplasia in a test set of consecutive cases. FUNDING:Olympus.
10.1016/S2589-7500(23)00199-1
Artificial intelligence system for the detection of Barrett's esophagus.
World journal of gastroenterology
BACKGROUND:Barrett's esophagus (BE), which has increased in prevalence worldwide, is a precursor for esophageal adenocarcinoma. Although there is a gap in the detection rates between endoscopic BE and histological BE in current research, we trained our artificial intelligence (AI) system with images of endoscopic BE and tested the system with images of histological BE. AIM:To assess whether an AI system can aid in the detection of BE in our setting. METHODS:Endoscopic narrow-band imaging (NBI) was collected from Chung Shan Medical University Hospital and Changhua Christian Hospital, resulting in 724 cases, with 86 patients having pathological results. Three senior endoscopists, who were instructing physicians of the Digestive Endoscopy Society of Taiwan, independently annotated the images in the development set to determine whether each image was classified as an endoscopic BE. The test set consisted of 160 endoscopic images of 86 cases with histological results. RESULTS:Six pre-trained models were compared, and EfficientNetV2B2 (accuracy [ACC]: 0.8) was selected as the backbone architecture for further evaluation due to better ACC results. In the final test, the AI system correctly identified 66 of 70 cases of BE and 85 of 90 cases without BE, resulting in an ACC of 94.37%. CONCLUSION:Our AI system, which was trained by NBI of endoscopic BE, can adequately predict endoscopic images of histological BE. The ACC, sensitivity, and specificity are 94.37%, 94.29%, and 94.44%, respectively.
10.3748/wjg.v29.i48.6198
Efficacy of Digestive Endoscope Based on Artificial Intelligence System in Diagnosing Early Esophageal Carcinoma.
Computational and mathematical methods in medicine
Objective:To explore the efficacy of digestive endoscopy (DEN) based on artificial intelligence (AI) system in diagnosing early esophageal carcinoma. Methods:The clinical data of 300 patients with suspected esophageal carcinoma treated in our hospital from January 2018 to January 2020 were retrospectively analyzed; among them, 198 were diagnosed with esophageal carcinoma after pathological examination, and 102 had benign esophageal lesion. An AI system based on convolutional neural network (CNN) was adopted to assess the DEN images of patients with early esophageal carcinoma. A total of 200 patients (148 with early esophageal carcinoma and 52 with benign esophageal lesion) were selected as the learning group for the Inception V3 image classification system to learn; and the rest 100 patients (50 with early esophageal carcinoma and 50 with benign esophageal lesion) were included in the diagnosis group for the Inception V3 system to assist the narrow-band imaging (NBI) with diagnosis. The diagnosis results from Inception V3-assisted NBI were compared with those from imaging physicians, and the diagnostic efficacy diagram was drawn. Results:The diagnosis rate of AI-NBI was significantly faster than that of physician diagnosis (0.02 ± 0.01 vs. 5.65 ± 0.32 s (mean rate of two physicians), < 0.001); between AI-NBI diagnosis and physician diagnosis, no statistical differences in sensitivity (90.0% vs. 92.0%), specificity (92.0% vs. 94.0%), and accuracy (91.0% vs. 93.0%) were observed ( > 0.05); and according to the ROC curves, AUC (95% CI) of AI-NBI diagnosis = 0.910 (0.845-0.975), and AUC (95% CI) of physician diagnosis = 0.930 (0.872-0.988). Conclusion:CNN-based AI system can assist NBI in screening early esophageal carcinoma, which has a good application prospect in the clinical diagnosis of early esophageal carcinoma.
10.1155/2022/9018939
Artificial intelligence for diagnosing microvessels of precancerous lesions and superficial esophageal squamous cell carcinomas: a multicenter study.
Surgical endoscopy
BACKGROUND:Intrapapillary capillary loop (IPCL) is an important factor for predicting invasion depth of esophageal squamous cell carcinoma (ESCC). The invasion depth is closely related to the selection of treatment strategy. However, diagnosis of IPCLs is complicated and subject to interobserver variability. This study aimed to develop an artificial intelligence (AI) system to predict IPCLs subtypes of precancerous lesions and superficial ESCC. METHODS:Images of magnifying endoscopy with narrow band imaging from three hospitals were collected retrospectively. IPCLs subtypes were annotated on images by expert endoscopists according to Japanese Endoscopic Society classification. The performance of the AI system was evaluated using internal and external validation datasets (IVD and EVD) and compared with that of the 11 endoscopists. RESULTS:A total of 7094 images from 685 patients were used to train and validate the AI system. The combined accuracy of the AI system for diagnosing IPCLs subtypes in IVD and EVD was 91.3% and 89.8%, respectively. The AI system achieved better performance than endoscopists in predicting IPCLs subtypes and invasion depth. The ability of junior endoscopists to diagnose IPCLs subtypes (combined accuracy: 84.7% vs 78.2%, P < 0.0001) and invasion depth (combined accuracy: 74.4% vs 67.9%, P < 0.0001) were significantly improved with AI system assistance. Although there was no significant differences, the performance of senior endoscopists was slightly elevated. CONCLUSIONS:The proposed AI system could improve the diagnostic ability of endoscopists to predict IPCLs classification of precancerous lesions and superficial ESCC.
10.1007/s00464-022-09353-0
Enabling large-scale screening of Barrett's esophagus using weakly supervised deep learning in histopathology.
Nature communications
Timely detection of Barrett's esophagus, the pre-malignant condition of esophageal adenocarcinoma, can improve patient survival rates. The Cytosponge-TFF3 test, a non-endoscopic minimally invasive procedure, has been used for diagnosing intestinal metaplasia in Barrett's. However, it depends on pathologist's assessment of two slides stained with H&E and the immunohistochemical biomarker TFF3. This resource-intensive clinical workflow limits large-scale screening in the at-risk population. To improve screening capacity, we propose a deep learning approach for detecting Barrett's from routinely stained H&E slides. The approach solely relies on diagnostic labels, eliminating the need for expensive localized expert annotations. We train and independently validate our approach on two clinical trial datasets, totaling 1866 patients. We achieve 91.4% and 87.3% AUROCs on discovery and external test datasets for the H&E model, comparable to the TFF3 model. Our proposed semi-automated clinical workflow can reduce pathologists' workload to 48% without sacrificing diagnostic performance, enabling pathologists to prioritize high risk cases.
10.1038/s41467-024-46174-2
Machine learning-based automated sponge cytology for screening of oesophageal squamous cell carcinoma and adenocarcinoma of the oesophagogastric junction: a nationwide, multicohort, prospective study.
The lancet. Gastroenterology & hepatology
BACKGROUND:Oesophageal squamous cell carcinoma and adenocarcinoma of the oesophagogastric junction have a dismal prognosis, and early detection is key to reduce mortality. However, early detection depends on upper gastrointestinal endoscopy, which is not feasible to implement at a population level. We aimed to develop and validate a fully automated machine learning-based prediction tool integrating a minimally invasive sponge cytology test and epidemiological risk factors for screening of oesophageal squamous cell carcinoma and adenocarcinoma of the oesophagogastric junction before endoscopy. METHODS:For this multicohort prospective study, we enrolled participants aged 40-75 years undergoing upper gastrointestinal endoscopy screening at 39 tertiary or secondary hospitals in China for model training and testing, and included community-based screening participants for further validation. All participants underwent questionnaire surveys, sponge cytology testing, and endoscopy in a sequential manner. We trained machine learning models to predict a composite outcome of high-grade lesions, defined as histology-confirmed high-grade intraepithelial neoplasia and carcinoma of the oesophagus and oesophagogastric junction. The predictive features included 105 cytological and 15 epidemiological features. Model performance was primarily measured with the area under the receiver operating characteristic curve (AUROC) and average precision. The performance measures for cytologists with AI assistance was also assessed. FINDINGS:Between Jan 1, 2021, and June 30, 2022, 17 498 eligible participants were involved in model training and validation. In the testing set, the AUROC of the final model was 0·960 (95% CI 0·937 to 0·977) and the average precision was 0·482 (0·470 to 0·494). The model achieved similar performance to consensus of cytologists with AI assistance (AUROC 0·955 [95% CI 0·933 to 0·975]; p=0·749; difference 0·005, 95% CI, -0·011 to 0·020). If the model-defined moderate-risk and high-risk groups were referred for endoscopy, the sensitivity was 94·5% (95% CI 88·8 to 97·5), specificity was 91·9% (91·2 to 92·5), and the predictive positive value was 18·4% (15·6 to 21·6), and 90·3% of endoscopies could be avoided. Further validation in community-based screening showed that the AUROC of the model was 0·964 (95% CI 0·920 to 0·990), and 92·8% of endoscopies could be avoided after risk stratification. INTERPRETATION:We developed a prediction tool with favourable performance for screening of oesophageal squamous cell carcinoma and adenocarcinoma of the oesophagogastric junction. This approach could prevent the need for endoscopy screening in many low-risk individuals and ensure resource optimisation by prioritising high-risk individuals. FUNDING:Science and Technology Commission of Shanghai Municipality.
10.1016/S2468-1253(23)00004-3
Artificial intelligence for detecting and delineating the extent of superficial esophageal squamous cell carcinoma and precancerous lesions under narrow-band imaging (with video).
Gastrointestinal endoscopy
BACKGROUND AND AIMS:Although narrow-band imaging (NBI) is a useful modality for detecting and delineating esophageal squamous cell carcinoma (ESCC), there is a risk of incorrectly determining the margins of some lesions even with NBI. This study aimed to develop an artificial intelligence (AI) system for detecting superficial ESCC and precancerous lesions and delineating the extent of lesions under NBI. METHODS:Nonmagnified NBI images from 4 hospitals were collected and annotated. Internal and external image test datasets were used to evaluate the detection and delineation performance of the system. The delineation performance of the system was compared with that of endoscopists. Furthermore, the system was directly integrated into the endoscopy equipment, and its real-time diagnostic capability was prospectively estimated. RESULTS:The system was trained and tested using 10,047 still images and 140 videos from 1112 patients and 1183 lesions. In the image testing, the accuracy of the system in detecting lesions in internal and external tests was 92.4% and 89.9%, respectively. The accuracy of the system in delineating extents in internal and external tests was 88.9% and 87.0%, respectively. The delineation performance of the system was superior to that of junior endoscopists and similar to that of senior endoscopists. In the prospective clinical evaluation, the system exhibited satisfactory performance, with an accuracy of 91.4% in detecting lesions and an accuracy of 85.9% in delineating extents. CONCLUSIONS:The proposed AI system could accurately detect superficial ESCC and precancerous lesions and delineate the extent of lesions under NBI.
10.1016/j.gie.2022.12.003
Artificial intelligence for detecting superficial esophageal squamous cell carcinoma under multiple endoscopic imaging modalities: A multicenter study.
Yuan Xiang-Lei,Guo Lin-Jie,Liu Wei,Zeng Xian-Hui,Mou Yi,Bai Shuai,Pan Zhen-Guo,Zhang Tao,Pu Wen-Feng,Wen Chun,Wang Jun,Zhou Zheng-Duan,Feng Jing,Hu Bing
Journal of gastroenterology and hepatology
BACKGROUND AND AIM:Diagnosis of esophageal squamous cell carcinoma (ESCC) is complicated and requires substantial expertise and experience. This study aimed to develop an artificial intelligence (AI) system for detecting superficial ESCC under multiple endoscopic imaging modalities. METHODS:Endoscopic images were retrospectively collected from West China Hospital, Sichuan University as a training dataset and an independent internal validation dataset. Images from other four hospitals were used as an external validation dataset. The AI system was compared with 11 experienced endoscopists. Furthermore, videos were collected to assess the performance of the AI system. RESULTS:A total of 53 933 images from 2621 patients and 142 videos from 19 patients were used to develop and validate the AI system. In the internal and external validation datasets, the performance of the AI system under all or different endoscopic imaging modalities was satisfactory, with sensitivity of 92.5-99.7%, specificity of 78.5-89.0%, and area under the receiver operating characteristic curves of 0.906-0.989. The AI system achieved comparable performance with experienced endoscopists. Regarding superficial ESCC confined to the epithelium, the AI system was more sensitive than experienced endoscopists on white-light imaging (90.8% vs 82.5%, P = 0.022). Moreover, the AI system exhibited good performance in videos, with sensitivity of 89.5-100% and specificity of 73.7-89.5%. CONCLUSIONS:We developed an AI system that showed comparable performance with experienced endoscopists in detecting superficial ESCC under multiple endoscopic imaging modalities and might provide valuable support for inexperienced endoscopists, despite requiring further evaluation.
10.1111/jgh.15689
Development and validation of artificial neural networks model for detection of Barrett's neoplasia: a multicenter pragmatic nonrandomized trial (with video).
Gastrointestinal endoscopy
BACKGROUND AND AIMS:The aim of this study was to develop and externally validate a computer-aided detection (CAD) system for the detection and localization of Barrett's neoplasia and assess its performance compared with that of general endoscopists in a statistically powered multicenter study by using real-time video sequences. METHODS:In phase 1, the hybrid visual geometry group 16-SegNet model was trained by the use of 75,198 images and videos (96 patients) of neoplastic and 1,014,973 images and videos (65 patients) of nonneoplastic Barrett's esophagus. In phase 2, image-based validation was performed on a separate dataset of 107 images (20 patients) of neoplastic and 364 images (14 patients) of nonneoplastic Barrett's esophagus. In phase 3 (video-based external validation) we designed a real-time video-based study with 32 videos (32 patients) of neoplastic and 43 videos (43 patients) of nonneoplastic Barrett's esophagus from 4 European centers to compare the performance of the CAD model with that of 6 nonexpert endoscopists. The primary endpoint was the sensitivity of CAD diagnosis of Barrett's neoplasia. RESULTS:In phase 2, CAD detected Barrett's neoplasia with sensitivity, specificity, and accuracy of 95.3%, 94.5%, and 94.7%, respectively. In phase 3, the CAD system detected Barrett's neoplasia with sensitivity, specificity, negative predictive value, and accuracy of 93.8%, 90.7%, 95.1%, and 92.0%, respectively, compared with the endoscopists' performance of 63.5%, 77.9%, 74.2%, and 71.8%, respectively (P < .05 in all parameters). The CAD system localized neoplastic lesions with accuracy, mean precision, and mean intersection over union of 100%, 0.62, and 0.54, respectively, when compared with at least 1 of the expert markings. The processing speed of the CAD detection and localization were 5 ms/image and 33 ms/image, respectively. CONCLUSION:To our knowledge, this is the first study describing external (multicenter) validation of AI algorithms for the detection of Barrett's neoplasia on real-time endoscopic videos. The CAD system in this study significantly outperformed nonexpert endoscopists on real-time video-based assessment, achieving >90% sensitivity for neoplasia detection. This result needs to be validated during real-time endoscopic assessment.
10.1016/j.gie.2022.10.031
Human-Like Artificial Intelligent System for Predicting Invasion Depth of Esophageal Squamous Cell Carcinoma Using Magnifying Narrow-Band Imaging Endoscopy: A Retrospective Multicenter Study.
Clinical and translational gastroenterology
INTRODUCTION:Endoscopic evaluation is crucial for predicting the invasion depth of esophagus squamous cell carcinoma (ESCC) and selecting appropriate treatment strategies. Our study aimed to develop and validate an interpretable artificial intelligence-based invasion depth prediction system (AI-IDPS) for ESCC. METHODS:We reviewed the PubMed for eligible studies and collected potential visual feature indices associated with invasion depth. Multicenter data comprising 5,119 narrow-band imaging magnifying endoscopy images from 581 patients with ESCC were collected from 4 hospitals between April 2016 and November 2021. Thirteen models for feature extraction and 1 model for feature fitting were developed for AI-IDPS. The efficiency of AI-IDPS was evaluated on 196 images and 33 consecutively collected videos and compared with a pure deep learning model and performance of endoscopists. A crossover study and a questionnaire survey were conducted to investigate the system's impact on endoscopists' understanding of the AI predictions. RESULTS:AI-IDPS demonstrated the sensitivity, specificity, and accuracy of 85.7%, 86.3%, and 86.2% in image validation and 87.5%, 84%, and 84.9% in consecutively collected videos, respectively, for differentiating SM2-3 lesions. The pure deep learning model showed significantly lower sensitivity, specificity, and accuracy (83.7%, 52.1% and 60.0%, respectively). The endoscopists had significantly improved accuracy (from 79.7% to 84.9% on average, P = 0.03) and comparable sensitivity (from 37.5% to 55.4% on average, P = 0.27) and specificity (from 93.1% to 94.3% on average, P = 0.75) after AI-IDPS assistance. DISCUSSION:Based on domain knowledge, we developed an interpretable system for predicting ESCC invasion depth. The anthropopathic approach demonstrates the potential to outperform deep learning architecture in practice.
10.14309/ctg.0000000000000606
Effect of an artificial intelligence-assisted system on endoscopic diagnosis of superficial oesophageal squamous cell carcinoma and precancerous lesions: a multicentre, tandem, double-blind, randomised controlled trial.
The lancet. Gastroenterology & hepatology
BACKGROUND:Despite the usefulness of white light endoscopy (WLE) and non-magnified narrow-band imaging (NBI) for screening for superficial oesophageal squamous cell carcinoma and precancerous lesions, these lesions might be missed due to their subtle features and interpretation variations among endoscopists. Our team has developed an artificial intelligence (AI) system to detect superficial oesophageal squamous cell carcinoma and precancerous lesions using WLE and non-magnified NBI. We aimed to evaluate the auxiliary diagnostic performance of the AI system in a real clinical setting. METHODS:We did a multicentre, tandem, double-blind, randomised controlled trial at 12 hospitals in China. Eligible patients were aged 18 years or older and underwent sedated upper gastrointestinal endoscopy for screening, investigation of gastrointestinal symptoms, or surveillance. Patients were randomly assigned (1:1) to either the AI-first group or the routine-first group using a computerised random number generator. Patients, pathologists, and statistical analysts were masked to group assignment, whereas endoscopists and research assistants were not. The same endoscopist at each centre did tandem upper gastrointestinal endoscopy for each eligible patient on the same day. In the AI-first group, the endoscopist did the first examination with the assistance of the AI system and the second examination without it. In the routine-first group, the order of examinations was reversed. The primary outcome was the miss rate of superficial oesophageal squamous cell carcinoma and precancerous lesions, calculated on a per-lesion and per-patient basis. All analyses were done on a per-protocol basis. This trial is registered with the Chinese Clinical Trial Registry (ChiCTR2100052116) and is completed. FINDINGS:Between Oct 19, 2021, and June 8, 2022, 5934 patients were randomly assigned to the AI-first group and 5912 to the routine-first group, of whom 5865 and 5850 were eligible for analysis. Per-lesion miss rates were 1·7% (2/118; 95% CI 0·0-4·0) in the AI-first group versus 6·7% (6/90; 1·5-11·8) in the routine-first group (risk ratio 0·25, 95% CI 0·06-1·08; p=0·079). Per-patient miss rates were 1·9% (2/106; 0·0-4·5) in AI-first group versus 5·1% (4/79; 0·2-9·9) in the routine-first group (0·37, 0·08-1·71; p=0·40). Bleeding after biopsy of oesophageal lesions was observed in 13 (0·2%) patients in the AI-first group and 11 (0·2%) patients in the routine-first group. No serious adverse events were reported by patients in either group. INTERPRETATION:The observed effect of AI-assisted endoscopy on the per-lesion and per-patient miss rates of superficial oesophageal squamous cell carcinoma and precancerous lesions under WLE and non-magnified NBI was consistent with substantial benefit through to a neutral or small negative effect. The effectiveness and cost-benefit of this AI system in real-world clinical settings remain to be further assessed. FUNDING:National Natural Science Foundation of China, 1·3·5 project for disciplines of excellence, West China Hospital, Sichuan University, and Chengdu Science and Technology Project. TRANSLATION:For the Chinese translation of the abstract see Supplementary Materials section.
10.1016/S2468-1253(23)00276-5
Endoscopic prediction of submucosal invasion in Barrett's cancer with the use of artificial intelligence: a pilot study.
Endoscopy
BACKGROUND:The accurate differentiation between T1a and T1b Barrett's-related cancer has both therapeutic and prognostic implications but is challenging even for experienced physicians. We trained an artificial intelligence (AI) system on the basis of deep artificial neural networks (deep learning) to differentiate between T1a and T1b Barrett's cancer on white-light images. METHODS:Endoscopic images from three tertiary care centers in Germany were collected retrospectively. A deep learning system was trained and tested using the principles of cross validation. A total of 230 white-light endoscopic images (108 T1a and 122 T1b) were evaluated using the AI system. For comparison, the images were also classified by experts specialized in endoscopic diagnosis and treatment of Barrett's cancer. RESULTS:The sensitivity, specificity, F1 score, and accuracy of the AI system in the differentiation between T1a and T1b cancer lesions was 0.77, 0.64, 0.74, and 0.71, respectively. There was no statistically significant difference between the performance of the AI system and that of experts, who showed sensitivity, specificity, F1, and accuracy of 0.63, 0.78, 0.67, and 0.70, respectively. CONCLUSION:This pilot study demonstrates the first multicenter application of an AI-based system in the prediction of submucosal invasion in endoscopic images of Barrett's cancer. AI scored equally to international experts in the field, but more work is necessary to improve the system and apply it to video sequences and real-life settings. Nevertheless, the correct prediction of submucosal invasion in Barrett's cancer remains challenging for both experts and AI.
10.1055/a-1311-8570
A novel CT-based radiomics model for predicting response and prognosis of chemoradiotherapy in esophageal squamous cell carcinoma.
Scientific reports
No clinically relevant biomarker has been identified for predicting the response of esophageal squamous cell carcinoma (ESCC) to chemoradiotherapy (CRT). Herein, we established a CT-based radiomics model with artificial intelligence (AI) to predict the response and prognosis of CRT in ESCC. A total of 44 ESCC patients (stage I-IV) were enrolled in this study; training (n = 27) and validation (n = 17) cohorts. First, we extracted a total of 476 radiomics features from three-dimensional CT images of cancer lesions in training cohort, selected 110 features associated with the CRT response by ROC analysis (AUC ≥ 0.7) and identified 12 independent features, excluding correlated features by Pearson's correlation analysis (r ≥ 0.7). Based on the 12 features, we constructed 5 prediction models of different machine learning algorithms (Random Forest (RF), Ridge Regression, Naive Bayes, Support Vector Machine, and Artificial Neural Network models). Among those, the RF model showed the highest AUC in the training cohort (0.99 [95%CI 0.86-1.00]) as well as in the validation cohort (0.92 [95%CI 0.71-0.99]) to predict the CRT response. Additionally, Kaplan-Meyer analysis of the validation cohort and all the patient data showed significantly longer progression-free and overall survival in the high-prediction score group compared with the low-prediction score group in the RF model. Univariate and multivariate analyses revealed that the radiomics prediction score and lymph node metastasis were independent prognostic biomarkers for CRT of ESCC. In conclusion, we have developed a CT-based radiomics model using AI, which may have the potential to predict the CRT response as well as the prognosis for ESCC patients with non-invasiveness and cost-effectiveness.
10.1038/s41598-024-52418-4
Artificial intelligence for the detection of esophageal and esophagogastric junctional adenocarcinoma.
Iwagami Hiroyoshi,Ishihara Ryu,Aoyama Kazuharu,Fukuda Hiromu,Shimamoto Yusaku,Kono Mitsuhiro,Nakahira Hiroko,Matsuura Noriko,Shichijo Satoki,Kanesaka Takashi,Kanzaki Hiromitsu,Ishii Tatsuya,Nakatani Yasuki,Tada Tomohiro
Journal of gastroenterology and hepatology
BACKGROUND AND AIM:Conventional endoscopy for the early detection of esophageal and esophagogastric junctional adenocarcinoma (E/J cancer) is limited because early lesions are asymptomatic, and the associated changes in the mucosa are subtle. There are no reports on artificial intelligence (AI) diagnosis for E/J cancer from Asian countries. Therefore, we aimed to develop a computerized image analysis system using deep learning for the detection of E/J cancers. METHODS:A total of 1172 images from 166 pathologically proven superficial E/J cancer cases and 2271 images of normal mucosa in esophagogastric junctional from 219 cases were used as the training image data. A total of 232 images from 36 cancer cases and 43 non-cancerous cases were used as the validation test data. The same validation test data were diagnosed by 15 board-certified specialists (experts). RESULTS:The sensitivity, specificity, and accuracy of the AI system were 94%, 42%, and 66%, respectively, and that of the experts were 88%, 43%, and 63%, respectively. The sensitivity of the AI system was favorable, while its specificity for non-cancerous lesions was similar to that of the experts. Interobserver agreement among the experts for detecting superficial E/J was fair (Fleiss' kappa = 0.26, z = 20.4, P < 0.001). CONCLUSIONS:Our AI system achieved high sensitivity and acceptable specificity for the detection of E/J cancers and may be a good supporting tool for the screening of E/J cancers.
10.1111/jgh.15136
Artificial Intelligence for Detecting and Delineating Margins of Early ESCC Under WLI Endoscopy.
Clinical and translational gastroenterology
INTRODUCTION:Conventional white light imaging (WLI) endoscopy is the most common screening technique used for detecting early esophageal squamous cell carcinoma (ESCC). Nevertheless, it is difficult to detect and delineate margins of early ESCC using WLI endoscopy. This study aimed to develop an artificial intelligence (AI) model to detect and delineate margins of early ESCC under WLI endoscopy. METHODS:A total of 13,083 WLI images from 1,239 patients were used to train and test the AI model. To evaluate the detection performance of the model, 1,479 images and 563 images were used as internal and external validation data sets, respectively. For assessing the delineation performance of the model, 1,114 images and 211 images were used as internal and external validation data sets, respectively. In addition, 216 images were used to compare the delineation performance between the model and endoscopists. RESULTS:The model showed an accuracy of 85.7% and 84.5% in detecting lesions in internal and external validation, respectively. For delineating margins, the model achieved an accuracy of 93.4% and 95.7% in the internal and external validation, respectively, under an overlap ratio of 0.60. The accuracy of the model, senior endoscopists, and expert endoscopists in delineating margins were 98.1%, 78.6%, and 95.3%, respectively. The proposed model achieved similar delineating performance compared with that of expert endoscopists but superior to senior endoscopists. DISCUSSION:We successfully developed an AI model, which can be used to accurately detect early ESCC and delineate the margins of the lesions under WLI endoscopy.
10.14309/ctg.0000000000000433
Utilizing artificial intelligence in endoscopy: a clinician's guide.
Expert review of gastroenterology & hepatology
INTRODUCTION:Artificial intelligence (AI) that surpasses human ability in image recognition is expected to be applied in the field of gastrointestinal endoscopes. Accordingly, its research and development (R &D) is being actively conducted. With the development of endoscopic diagnosis, there is a shortage of specialists who can perform high-precision endoscopy. We will examine whether AI with excellent image recognition ability can overcome this problem. AREAS COVERED:Since 2016, papers on artificial intelligence using convolutional neural network (CNN in other word Deep Learning) have been published. CNN is generally capable of more accurate detection and classification than conventional machine learning. This is a review of papers using CNN in the gastrointestinal endoscopy area, along with the reasons why AI is required in clinical practice. We divided this review into four parts: stomach, esophagus, large intestine, and capsule endoscope (small intestine). EXPERT OPINION:Potential applications for the AI include colorectal polyp detection and differentiation, gastric and esophageal cancer detection, and lesion detection in capsule endoscopy. The accuracy of endoscopic diagnosis will increase if the AI and endoscopist perform the endoscopy together.
10.1080/17474124.2020.1779058
Diagnosis using deep-learning artificial intelligence based on the endocytoscopic observation of the esophagus.
Kumagai Youichi,Takubo Kaiyo,Kawada Kenro,Aoyama Kazuharu,Endo Yuma,Ozawa Tsuyoshi,Hirasawa Toshiaki,Yoshio Toshiyuki,Ishihara Soichiro,Fujishiro Mitsuhiro,Tamaru Jun-Ichi,Mochiki Erito,Ishida Hideyuki,Tada Tomohiro
Esophagus : official journal of the Japan Esophageal Society
BACKGROUND AND AIMS:The endocytoscopic system (ECS) helps in virtual realization of histology and can aid in confirming histological diagnosis in vivo. We propose replacing biopsy-based histology for esophageal squamous cell carcinoma (ESCC) by using the ECS. We applied deep-learning artificial intelligence (AI) to analyse ECS images of the esophagus to determine whether AI can support endoscopists for the replacement of biopsy-based histology. METHODS:A convolutional neural network-based AI was constructed based on GoogLeNet and trained using 4715 ECS images of the esophagus (1141 malignant and 3574 non-malignant images). To evaluate the diagnostic accuracy of the AI, an independent test set of 1520 ECS images, collected from 55 consecutive patients (27 ESCCs and 28 benign esophageal lesions) were examined. RESULTS:On the basis of the receiver-operating characteristic curve analysis, the areas under the curve of the total images, higher magnification pictures, and lower magnification pictures were 0.85, 0.90, and 0.72, respectively. The AI correctly diagnosed 25 of the 27 ESCC cases, with an overall sensitivity of 92.6%. Twenty-five of the 28 non-cancerous lesions were diagnosed as non-malignant, with a specificity of 89.3% and an overall accuracy of 90.9%. Two cases of malignant lesions, misdiagnosed as non-malignant by the AI, were correctly diagnosed as malignant by the endoscopist. Among the 3 cases of non-cancerous lesions diagnosed as malignant by the AI, 2 were of radiation-related esophagitis and one was of gastroesophageal reflux disease. CONCLUSION:AI is expected to support endoscopists in diagnosing ESCC based on ECS images without biopsy-based histological reference.
10.1007/s10388-018-0651-7
Feasibility and Accuracy of Artificial Intelligence-Assisted Sponge Cytology for Community-Based Esophageal Squamous Cell Carcinoma Screening in China.
Gao Ye,Xin Lei,Feng Ya-Dong,Yao Bin,Lin Han,Sun Chang,An Wei,Li Zhao-Shen,Shi Rui-Hua,Wang Luo-Wei
The American journal of gastroenterology
INTRODUCTION:Screening is the pivotal strategy to relieve the burden of esophageal squamous cell carcinoma (ESCC) in high-risk areas. The cost, invasiveness, and accessibility of esophagogastroduodenoscopy (EGD) necessitate the development of preliminary screening methods. METHODS:Residents aged 40-85 years were recruited in a high-risk area of ESCC. Esophageal cells were collected using an approved novel capsule sponge, and cytology slides were scanned by a trained artificial intelligence (AI) system before cytologists provided confirmation. Atypical squamous cell or more severe diagnosis was defined as positive cytology. AI-based abnormal cell counts were also reported. EGD was performed subsequently with biopsy as needed. Diagnostic accuracy, adverse events, and acceptability of cytology testing were assessed. Esophageal high-grade lesions (ESCC and high-grade intraepithelial neoplasia) were the primary target lesions. RESULTS:In total, 1,844 participants were enrolled, and 20 (1.1%) high-grade lesions were confirmed by endoscopic biopsy. The AI-assisted cytologist-confirmed cytology showed good diagnostic accuracy, with a sensitivity of 90.0% (95% confidence interval [CI], 76.9%-100.0%), specificity of 93.7% (95% CI, 92.6%-94.8%), and positive predictive value of 13.5% (95% CI, 7.70%-19.3%) for detecting high-grade lesions. The area under the receiver operation characteristics curve was 0.926 (95% CI, 0.850-1.000) and 0.949 (95% CI, 0.890-1.000) for AI-assisted cytologist-confirmed cytology and AI-based abnormal cell count, respectively. The numbers of EGD could be reduced by 92.5% (from 99.2 to 7.4 to detect 1 high-grade lesion) if only cytology-positive participants were referred to endoscopy. No serious adverse events were documented during the cell collection process, and 96.1% participants reported this process as acceptable. DISCUSSION:The AI-assisted sponge cytology is feasible, safe, and acceptable for ESCC screening in community, with high accuracy for detecting esophageal squamous high-grade lesions.
10.14309/ajg.0000000000001499
Artificial intelligence assisted cytological detection for early esophageal squamous epithelial lesions by using low-grade squamous intraepithelial lesion as diagnostic threshold.
Cancer medicine
BACKGROUND:Manual cytological diagnosis for early esophageal squamous cell carcinoma (early ESCC) and high-grade intraepithelial neoplasia (HGIN) is unsatisfactory. Herein, we have introduced an artificial intelligence (AI)-assisted cytological diagnosis for such lesions. METHODS:Low-grade squamous intraepithelial lesion or worse was set as the diagnostic threshold for AI-assisted diagnosis. The performance of AI-assisted diagnosis was evaluated and compared to that of manual diagnosis. Feasibility in large-scale screening was also assessed. RESULTS:AI-assisted diagnosis for abnormal cells was superior to manual reading by presenting a higher efficiency for each slide (50.9 ± 0.8 s vs 236.8 ± 3.9 s, p = 1.52 × 10 ) and a better interobserver agreement (93.27% [95% CI, 92.76%-93.74%] vs 65.29% [95% CI, 64.35%-66.22%], p = 1.03 × 10 ). AI-assisted detection showed a higher diagnostic accuracy (96.89% [92.38%-98.57%] vs 72.54% [65.85%-78.35%], p = 1.42 × 10 ), sensitivity (99.35% [95.92%-99.97%] vs 68.39% [60.36%-75.48%], p = 7.11 × 10 ), and negative predictive value (NPV) (97.06% [82.95%-99.85%] vs 40.96% [30.46%-52.31%], p = 1.42 × 10 ). Specificity and positive predictive value (PPV) were not significantly differed. AI-assisted diagnosis demonstrated a smaller proportion of participants of interest (3.73%, [79/2117] vs.12.84% [272/2117], p = 1.59 × 10 ), a higher consistence between cytology and endoscopy (40.51% [32/79] vs. 12.13% [33/272], p = 1.54 × 10 8), specificity (97.74% [96.98%-98.32%] vs 88.52% [87.05%-89.84%], p = 3.19 × 10 ), and PPV (40.51% [29.79%-52.15%] vs 12.13% [8.61%-16.75%], p = 1.54 × 10 ) in community-based screening. Sensitivity and NPV were not significantly differed. AI-assisted diagnosis as primary screening significantly reduced average cost for detecting positive cases. CONCLUSION:Our study provides a novel cytological method for detecting and screening early ESCC and HGIN.
10.1002/cam4.4984
A new artificial intelligence system successfully detects and localises early neoplasia in Barrett's esophagus by using convolutional neural networks.
United European gastroenterology journal
BACKGROUND AND AIMS:Seattle protocol biopsies for Barrett's Esophagus (BE) surveillance are labour intensive with low compliance. Dysplasia detection rates vary, leading to missed lesions. This can potentially be offset with computer aided detection. We have developed convolutional neural networks (CNNs) to identify areas of dysplasia and where to target biopsy. METHODS:119 Videos were collected in high-definition white light and optical chromoendoscopy with i-scan (Pentax Hoya, Japan) imaging in patients with dysplastic and non-dysplastic BE (NDBE). We trained an indirectly supervised CNN to classify images as dysplastic/non-dysplastic using whole video annotations to minimise selection bias and maximise accuracy. The CNN was trained using 148,936 video frames (31 dysplastic patients, 31 NDBE, two normal esophagus), validated on 25,161 images from 11 patient videos and tested on 264 iscan-1 images from 28 dysplastic and 16 NDBE patients which included expert delineations. To localise targeted biopsies/delineations, a second directly supervised CNN was generated based on expert delineations of 94 dysplastic images from 30 patients. This was tested on 86 i-scan one images from 28 dysplastic patients. FINDINGS:The indirectly supervised CNN achieved a per image sensitivity in the test set of 91%, specificity 79%, area under receiver operator curve of 93% to detect dysplasia. Per-lesion sensitivity was 100%. Mean assessment speed was 48 frames per second (fps). 97% of targeted biopsy predictions matched expert and histological assessment at 56 fps. The artificial intelligence system performed better than six endoscopists. INTERPRETATION:Our CNNs classify and localise dysplastic Barrett's Esophagus potentially supporting endoscopists during surveillance.
10.1002/ueg2.12233
Artificial intelligence in upper GI endoscopy - current status, challenges and future promise.
Yu Honggang,Singh Rajvinder,Shin Seon Ho,Ho Khek Yu
Journal of gastroenterology and hepatology
White-light endoscopy with biopsy is the current gold standard modality for detecting and diagnosing upper gastrointestinal (GI) pathology. However, missed lesions remain a challenge. To overcome interobserver variability and learning curve issues, artificial intelligence (AI) has recently been introduced to assist endoscopists in the detection and diagnosis of upper GI neoplasia. In contrast to AI in colonoscopy, current AI studies for upper GI endoscopy are smaller pilot studies. Researchers currently lack large volume, well-annotated, high-quality datasets in gastric cancer, dysplasia in Barrett's esophagus and early esophageal squamous cell cancer. This review will look at the latest studies of AI in upper GI endoscopy, discuss some of the challenges facing researchers, and predict what the future may hold in this rapidly changing field.
10.1111/jgh.15354
Alterations to the Esophageal Microbiome Associated with Progression from Barrett's Esophagus to Esophageal Adenocarcinoma.
Snider Erik J,Compres Griselda,Freedberg Daniel E,Khiabanian Hossein,Nobel Yael R,Stump Stephania,Uhlemann Anne-Catrin,Lightdale Charles J,Abrams Julian A
Cancer epidemiology, biomarkers & prevention : a publication of the American Association for Cancer Research, cosponsored by the American Society of Preventive Oncology
BACKGROUND:The incidence of esophageal adenocarcinoma has risen dramatically over the past half century, and the underlying reasons are incompletely understood. Broad shifts to the upper gastrointestinal microbiome may be partly responsible. The goal of this study was to describe alterations in the esophageal microbiome that occur with progression from Barrett's esophagus to esophageal adenocarcinoma. METHODS:A case-control study was performed of patients with and without Barrett's esophagus who were scheduled to undergo upper endoscopy. Demographic, clinical, and dietary intake data were collected, and esophageal brushings were collected during the endoscopy. 16S rRNA gene sequencing was performed to characterize the microbiome. RESULTS:A total of 45 patients were enrolled and included in the analyses [16 controls; 14 Barrett's esophagus without dysplasia (NDBE); 6 low-grade dysplasia (LGD); 5 high-grade dysplasia (HGD); and 4 esophageal adenocarcinoma]. There was no difference in alpha diversity between non-Barrett's esophagus and Barrett's esophagus, but there was evidence of decreased diversity in patients with esophageal adenocarcinoma as assessed by Simpson index. There was an apparent shift in composition at the transition from LGD to HGD, and patients with HGD and esophageal adenocarcinoma had decreased Firmicutes and increased Proteobacteria. In addition, patients with HGD or esophageal adenocarcinoma had increased and and reduced . In the study population, patients taking proton pump inhibitors had increased and decreased Gram-negative bacteria overall. CONCLUSIONS:Shifts in the Barrett's esophagus-associated microbiome were observed in patients with HGD and esophageal adenocarcinoma, with increases in certain potentially pathogenic bacteria. IMPACT:The microbiome may play a role in esophageal carcinogenesis.
10.1158/1055-9965.EPI-19-0008
Advancements in Barrett's esophagus detection: The role of artificial intelligence and its implications.
World journal of gastroenterology
Artificial intelligence (AI) is making significant strides in revolutionizing the detection of Barrett's esophagus (BE), a precursor to esophageal adenocarcinoma. In the research article by Tsai , researchers utilized endoscopic images to train an AI model, challenging the traditional distinction between endoscopic and histological BE. This approach yielded remarkable results, with the AI system achieving an accuracy of 94.37%, sensitivity of 94.29%, and specificity of 94.44%. The study's extensive dataset enhances the AI model's practicality, offering valuable support to endoscopists by minimizing unnecessary biopsies. However, questions about the applicability to different endoscopic systems remain. The study underscores the potential of AI in BE detection while highlighting the need for further research to assess its adaptability to diverse clinical settings.
10.3748/wjg.v30.i11.1494
Endoscopic detection and differentiation of esophageal lesions using a deep neural network.
Ohmori Masayasu,Ishihara Ryu,Aoyama Kazuharu,Nakagawa Kentaro,Iwagami Hiroyoshi,Matsuura Noriko,Shichijo Satoki,Yamamoto Katsumi,Nagaike Koji,Nakahara Masanori,Inoue Takuya,Aoi Kenji,Okada Hiroyuki,Tada Tomohiro
Gastrointestinal endoscopy
BACKGROUND AND AIMS:Diagnosing esophageal squamous cell carcinoma (SCC) depends on individual physician expertise and may be subject to interobserver variability. Therefore, we developed a computerized image-analysis system to detect and differentiate esophageal SCC. METHODS:A total of 9591 nonmagnified endoscopy (non-ME) and 7844 ME images of pathologically confirmed superficial esophageal SCCs and 1692 non-ME and 3435 ME images from noncancerous lesions or normal esophagus were used as training image data. Validation was performed using 255 non-ME white-light images, 268 non-ME narrow-band images/blue-laser images, and 204 ME narrow-band images/blue-laser images from 135 patients. The same validation test data were diagnosed by 15 board-certified specialists (experienced endoscopists). RESULTS:Regarding diagnosis by non-ME with narrow-band imaging/blue-laser imaging, the sensitivity, specificity, and accuracy were 100%, 63%, and 77%, respectively, for the artificial intelligence (AI) system and 92%, 69%, and 78%, respectively, for the experienced endoscopists. Regarding diagnosis by non-ME with white-light imaging, the sensitivity, specificity, and accuracy were 90%, 76%, and 81%, respectively, for the AI system and 87%, 67%, and 75%, respectively, for the experienced endoscopists. Regarding diagnosis by ME, the sensitivity, specificity, and accuracy were 98%, 56%, and 77%, respectively, for the AI system and 83%, 70%, and 76%, respectively, for the experienced endoscopists. There was no significant difference in the diagnostic performance between the AI system and the experienced endoscopists. CONCLUSIONS:Our AI system showed high sensitivity for detecting SCC by non-ME and high accuracy for differentiating SCC from noncancerous lesions by ME.
10.1016/j.gie.2019.09.034
Artificial intelligence for cancer detection of the upper gastrointestinal tract.
Suzuki Hideo,Yoshitaka Tokai,Yoshio Toshiyuki,Tada Tomohiro
Digestive endoscopy : official journal of the Japan Gastroenterological Endoscopy Society
In recent years, artificial intelligence (AI) has been found to be useful to physicians in the field of image recognition due to three elements: deep learning (that is, CNN, convolutional neural network), a high-performance computer, and a large amount of digitized data. In the field of gastrointestinal endoscopy, Japanese endoscopists have produced the world's first achievements of CNN-based AI system for detecting gastric and esophageal cancers. This study reviews papers on CNN-based AI for gastrointestinal cancers, and discusses the future of this technology in clinical practice. Employing AI-based endoscopes would enable early cancer detection. The better diagnostic abilities of AI technology may be beneficial in early gastrointestinal cancers in which endoscopists have variable diagnostic abilities and accuracy. AI coupled with the expertise of endoscopists would increase the accuracy of endoscopic diagnosis.
10.1111/den.13897
Predictive Marker: HER2 in Esophageal Adenocarcinoma.
Subasinghe Duminda,Acott Nathan,Kumarasinghe M Priyanthi
Methods in molecular biology (Clifton, N.J.)
HER2 positivity is based on the fundamental principle of amplification of the human epidermal growth factor receptor 2 (HER2) gene resulting in overexpression of the protein products . Arising from that a "HER2-positive cancer" is one that shows HER2 gene amplification and resultant protein expression as demonstrated by in situ hybridization and immunohistochemistry, respectively. Testing of the HER2 status is crucial to ensure selection of the correct patient who may benefit from target therapy for esophageal adenocarcinoma. Accurate testing is dependent on several pre-analytical and analytical factors including sample selection, laboratory techniques, and accurate interpretation of HER2 test results.
10.1007/978-1-4939-7734-5_11
Usefulness of an artificial intelligence system for the detection of esophageal squamous cell carcinoma evaluated with videos simulating overlooking situation.
Waki Kotaro,Ishihara Ryu,Kato Yusuke,Shoji Ayaka,Inoue Takahiro,Matsueda Katsunori,Miyake Muneaki,Shimamoto Yusaku,Fukuda Hiromu,Matsuura Noriko,Ono Yoichiro,Yao Kenshi,Hashimoto Satoru,Terai Shuji,Ohmori Masayasu,Tanaka Kyosuke,Kato Motohiko,Shono Takashi,Miyamoto Hideaki,Tanaka Yasuhito,Tada Tomohiro
Digestive endoscopy : official journal of the Japan Gastroenterological Endoscopy Society
OBJECTIVES:Artificial intelligence (AI) systems have shown favorable performance in the detection of esophageal squamous cell carcinoma (ESCC). However, previous studies were limited by the quality of their validation methods. In this study, we evaluated the performance of an AI system with videos simulating situations in which ESCC has been overlooked. METHODS:We used 17,336 images from 1376 superficial ESCCs and 1461 images from 196 noncancerous and normal esophagi to construct the AI system. To record validation videos, the endoscope was passed through the esophagus at a constant speed without focusing on the lesion to simulate situations in which ESCC has been missed. Validation videos were evaluated by the AI system and 21 endoscopists. RESULTS:We prepared 100 video datasets, including 50 superficial ESCCs, 22 noncancerous lesions, and 28 normal esophagi. The AI system had sensitivity of 85.7% (54 of 63 ESCCs) and specificity of 40%. Initial evaluation by endoscopists conducted with plain video (without AI support) had average sensitivity of 75.0% (47.3 of 63 ESCC) and specificity of 91.4%. Subsequent evaluation by endoscopists was conducted with AI assistance, which improved their sensitivity to 77.7% (P = 0.00696) without changing their specificity (91.6%, P = 0.756). CONCLUSIONS:Our AI system had high sensitivity for the detection of ESCC. As a support tool, the system has the potential to enhance detection of ESCC without reducing specificity. (UMIN000039645).
10.1111/den.13934
Real-time artificial intelligence for endoscopic diagnosis of early esophageal squamous cell cancer (with video).
Yang Xiao-Xiao,Li Zhen,Shao Xue-Jun,Ji Rui,Qu Jun-Yan,Zheng Meng-Qi,Sun Yi-Ning,Zhou Ru-Chen,You Hang,Li Li-Xiang,Feng Jian,Yang Xiao-Yun,Li Yan-Qing,Zuo Xiu-Li
Digestive endoscopy : official journal of the Japan Gastroenterological Endoscopy Society
BACKGROUND AND AIMS:Endoscopic diagnosis of early esophageal squamous cell cancer (ESCC) is complicated and dependent on operators' experience. This study aimed to develop an artificial intelligence (AI) model for automatic diagnosis of early ESCC. METHODS:Non-magnifying and magnifying endoscopic images of normal/noncancerous lesions, early ESCC, and advanced esophageal cancer (AEC) were retrospectively obtained from Qilu Hospital of Shandong University. A total of 10,988 images from 5075 cases were chosen for training and validation. Another 2309 images from 1055 cases were collected for testing. One hundred and four real-time videos were also collected to evaluate the diagnostic performance of the AI model. The diagnostic performance of the AI model was compared with endoscopists by magnifying images and the assistant efficiency of the AI model for novices was evaluated. RESULTS:The AI diagnosis for non-magnifying images showed a per-patient accuracy, sensitivity, and specificity of 99.5%, 100%, 99.5% for white light imaging, and 97.0%, 97.2%, 96.4% for optical enhancement/iodine straining images. Regarding diagnosis for magnifying images, the per-patient accuracy, sensitivity, and specificity were 88.1%, 90.9%, and 85.0%. The diagnostic accuracy of the AI model was similar to experts (84.5%, P = 0.205) and superior to novices (68.5%, P = 0.005). The diagnostic performance of novices was significantly improved by AI assistance. When it comes to the diagnosis for real-time videos, the AI model showed acceptable performance as well. CONCLUSIONS:The AI model could accurately recognize early ESCC among noncancerous mucosa and AEC. It could be a potential assistant for endoscopists, especially for novices.
10.1111/den.13908
Ability of artificial intelligence to detect T1 esophageal squamous cell carcinoma from endoscopic videos and the effects of real-time assistance.
Shiroma Sho,Yoshio Toshiyuki,Kato Yusuke,Horie Yoshimasa,Namikawa Ken,Tokai Yoshitaka,Yoshimizu Shoichi,Yoshizawa Natsuko,Horiuchi Yusuke,Ishiyama Akiyoshi,Hirasawa Toshiaki,Tsuchida Tomohiro,Akazawa Naoki,Akiyama Junichi,Tada Tomohiro,Fujisaki Junko
Scientific reports
Diagnosis using artificial intelligence (AI) with deep learning could be useful in endoscopic examinations. We investigated the ability of AI to detect superficial esophageal squamous cell carcinoma (ESCC) from esophagogastroduodenoscopy (EGD) videos. We retrospectively collected 8428 EGD images of esophageal cancer to develop a convolutional neural network through deep learning. We evaluated the detection accuracy of the AI diagnosing system compared with that of 18 endoscopists. We used 144 EGD videos for the two validation sets. First, we used 64 EGD observation videos of ESCCs using both white light imaging (WLI) and narrow-band imaging (NBI). We then evaluated the system using 80 EGD videos from 40 patients (20 with superficial ESCC and 20 with non-ESCC). In the first set, the AI system correctly diagnosed 100% ESCCs. In the second set, it correctly detected 85% (17/20) ESCCs. Of these, 75% (15/20) and 55% (11/22) were detected by WLI and NBI, respectively, and the positive predictive value was 36.7%. The endoscopists correctly detected 45% (25-70%) ESCCs. With AI real-time assistance, the sensitivities of the endoscopists were significantly improved without AI assistance (p < 0.05). AI can detect superficial ESCCs from EGD videos with high sensitivity and the sensitivity of the endoscopist was improved with AI real-time support.
10.1038/s41598-021-87405-6
Application of artificial intelligence using convolutional neural networks in determining the invasion depth of esophageal squamous cell carcinoma.
Tokai Yoshitaka,Yoshio Toshiyuki,Aoyama Kazuharu,Horie Yoshimasa,Yoshimizu Shoichi,Horiuchi Yusuke,Ishiyama Akiyoshi,Tsuchida Tomohiro,Hirasawa Toshiaki,Sakakibara Yuko,Yamada Takuya,Yamaguchi Shinjiro,Fujisaki Junko,Tada Tomohiro
Esophagus : official journal of the Japan Esophageal Society
OBJECTIVES:In Japan, endoscopic resection (ER) is often used to treat esophageal squamous cell carcinoma (ESCC) when invasion depths are diagnosed as EP-SM1, whereas ESCC cases deeper than SM2 are treated by surgical operation or chemoradiotherapy. Therefore, it is crucial to determine the invasion depth of ESCC via preoperative endoscopic examination. Recently, rapid progress in the utilization of artificial intelligence (AI) with deep learning in medical fields has been achieved. In this study, we demonstrate the diagnostic ability of AI to measure ESCC invasion depth. METHODS:We retrospectively collected 1751 training images of ESCC at the Cancer Institute Hospital, Japan. We developed an AI-diagnostic system of convolutional neural networks using deep learning techniques with these images. Subsequently, 291 test images were prepared and reviewed by the AI-diagnostic system and 13 board-certified endoscopists to evaluate the diagnostic accuracy. RESULTS:The AI-diagnostic system detected 95.5% (279/291) of the ESCC in test images in 10 s, analyzed the 279 images and correctly estimated the invasion depth of ESCC with a sensitivity of 84.1% and accuracy of 80.9% in 6 s. The accuracy score of this system exceeded those of 12 out of 13 board-certified endoscopists, and its area under the curve (AUC) was greater than the AUCs of all endoscopists. CONCLUSIONS:The AI-diagnostic system demonstrated a higher diagnostic accuracy for ESCC invasion depth than those of endoscopists and, therefore, can be potentially used in ESCC diagnostics.
10.1007/s10388-020-00716-x
Comparison of performances of artificial intelligence versus expert endoscopists for real-time assisted diagnosis of esophageal squamous cell carcinoma (with video).
Fukuda Hiromu,Ishihara Ryu,Kato Yusuke,Matsunaga Takashi,Nishida Tsutomu,Yamada Takuya,Ogiyama Hideharu,Horie Mai,Kinoshita Kazuo,Tada Tomohiro
Gastrointestinal endoscopy
BACKGROUND AND AIMS:Narrow-band imaging (NBI) is currently regarded as the standard modality for diagnosing esophageal squamous cell carcinoma (SCC). We developed a computerized image-analysis system for diagnosing esophageal SCC by NBI and estimated its performance with video images. METHODS:Altogether, 23,746 images from 1544 pathologically proven superficial esophageal SCCs and 4587 images from 458 noncancerous and normal tissue were used to construct an artificial intelligence (AI) system. Five- to 9-second video clips from 144 patients captured by NBI or blue-light imaging were used as the validation dataset. These video images were diagnosed by the AI system and 13 board-certified specialists (experts). RESULTS:The diagnostic process was divided into 2 parts: detection (identify suspicious lesions) and characterization (differentiate cancer from noncancer). The sensitivities, specificities, and accuracies for the detection of SCC were, respectively, 91%, 51%, and 63% for the AI system and 79%, 72%, and 75% for the experts. The sensitivity of the AI system was significantly higher than that of the experts, but its specificity was significantly lower. Sensitivities, specificities, and accuracy for the characterization of SCC were, respectively, 86%, 89%, and 88% for the AI system and 74%, 76%, and 75% for the experts. The receiver operating characteristic curve showed that the AI system had significantly better diagnostic performance than the experts. CONCLUSIONS:Our AI system showed significantly higher sensitivity for detecting SCC and higher accuracy for characterizing SCC from noncancerous tissue than endoscopic experts.
10.1016/j.gie.2020.05.043
Targeted Therapies for Advanced Oesophagogastric Cancer: Recent Progress and Future Directions.
Drugs
The genomic landscape of oesophagogastric (OG) cancer is highly complex. The recent elucidation of some of the pathways involved has suggested a number of novel targets for therapy. This therapy is urgently required as with conventional chemotherapy regimens patients with advanced OG cancer still have a median overall survival of under a year. This review outlines the rationale for the current treatment of OG cancer with chemotherapy and describes both previously conducted and ongoing clinical trials of novel agents in this area. The targets and associated treatments discussed include HER-2, EGFR, VEGF, c-Met, FGFR-2, PI3K, mTOR andIGF-1. To date only two targeted treatments, trastuzumab and ramucirumab, have become part of the treatment paradigm for OG cancer, partly due to difficulties in defining predictive biomarkers in this disease. However, there are a number of promising drugs in the pipeline and this article seeks to describe these and other potential novel approaches including targeting DNA repair deficiencies and the immune system.
10.1007/s40265-015-0510-y
Real-time detection of the recurrent laryngeal nerve in thoracoscopic esophagectomy using artificial intelligence.
Surgical endoscopy
BACKGROUND:Artificial intelligence (AI) has been largely investigated in the field of surgery, particularly in quality assurance. However, AI-guided navigation during surgery has not yet been put into practice because a sufficient level of performance has not been reached. We aimed to develop deep learning-based AI image processing software to identify the location of the recurrent laryngeal nerve during thoracoscopic esophagectomy and determine whether the incidence of recurrent laryngeal nerve paralysis is reduced using this software. METHODS:More than 3000 images extracted from 20 thoracoscopic esophagectomy videos and 40 images extracted from 8 thoracoscopic esophagectomy videos were annotated for identification of the recurrent laryngeal nerve. The Dice coefficient was used to assess the detection performance of the model and that of surgeons (specialized esophageal surgeons and certified general gastrointestinal surgeons). The performance was compared using a test set. RESULTS:The average Dice coefficient of the AI model was 0.58. This was not significantly different from the Dice coefficient of the group of specialized esophageal surgeons (P = 0.26); however, it was significantly higher than that of the group of certified general gastrointestinal surgeons (P = 0.019). CONCLUSIONS:Our software's performance in identification of the recurrent laryngeal nerve was superior to that of general surgeons and almost reached that of specialized surgeons. Our software provides real-time identification and will be useful for thoracoscopic esophagectomy after further developments.
10.1007/s00464-022-09268-w
Real-time automated diagnosis of precancerous lesions and early esophageal squamous cell carcinoma using a deep learning model (with videos).
Guo LinJie,Xiao Xiao,Wu ChunCheng,Zeng Xianhui,Zhang Yuhang,Du Jiang,Bai Shuai,Xie Jia,Zhang Zhiwei,Li Yuhong,Wang Xuedan,Cheung Onpan,Sharma Malay,Liu Jingjia,Hu Bing
Gastrointestinal endoscopy
BACKGROUND AND AIMS:We developed a system for computer-assisted diagnosis (CAD) for real-time automated diagnosis of precancerous lesions and early esophageal squamous cell carcinomas (ESCCs) to assist the diagnosis of esophageal cancer. METHODS:A total of 6473 narrow-band imaging (NBI) images, including precancerous lesions, early ESCCs, and noncancerous lesions, were used to train the CAD system. We validated the CAD system using both endoscopic images and video datasets. The receiver operating characteristic curve of the CAD system was generated based on image datasets. An artificial intelligence probability heat map was generated for each input of endoscopic images. The yellow color indicated high possibility of cancerous lesion, and the blue color indicated noncancerous lesions on the probability heat map. When the CAD system detected any precancerous lesion or early ESCCs, the lesion of interest was masked with color. RESULTS:The image datasets contained 1480 malignant NBI images from 59 consecutive cancerous cases (sensitivity, 98.04%) and 5191 noncancerous NBI images from 2004 cases (specificity, 95.03%). The area under curve was 0.989. The video datasets of precancerous lesions or early ESCCs included 27 nonmagnifying videos (per-frame sensitivity 60.8%, per-lesion sensitivity, 100%) and 20 magnifying videos (per-frame sensitivity 96.1%, per-lesion sensitivity, 100%). Unaltered full-range normal esophagus videos included 33 videos (per-frame specificity 99.9%, per-case specificity, 90.9%). CONCLUSIONS:A deep learning model demonstrated high sensitivity and specificity for both endoscopic images and video datasets. The real-time CAD system has a promising potential in the near future to assist endoscopists in diagnosing precancerous lesions and ESCCs.
10.1016/j.gie.2019.08.018
Diagnostic Performance of Artificial Intelligence-Centred Systems in the Diagnosis and Postoperative Surveillance of Upper Gastrointestinal Malignancies Using Computed Tomography Imaging: A Systematic Review and Meta-Analysis of Diagnostic Accuracy.
Chidambaram Swathikan,Sounderajah Viknesh,Maynard Nick,Markar Sheraz R
Annals of surgical oncology
BACKGROUND:Upper gastrointestinal cancers are aggressive malignancies with poor prognosis, even following multimodality therapy. As such, they require timely and accurate diagnostic and surveillance strategies; however, such radiological workflows necessitate considerable expertise and resource to maintain. In order to lessen the workload upon already stretched health systems, there has been increasing focus on the development and use of artificial intelligence (AI)-centred diagnostic systems. This systematic review summarizes the clinical applicability and diagnostic performance of AI-centred systems in the diagnosis and surveillance of esophagogastric cancers. METHODS:A systematic review was performed using the MEDLINE, EMBASE, Cochrane Review, and Scopus databases. Articles on the use of AI and radiomics for the diagnosis and surveillance of patients with esophageal cancer were evaluated, and quality assessment of studies was performed using the QUADAS-2 tool. A meta-analysis was performed to assess the diagnostic accuracy of sequencing methodologies. RESULTS:Thirty-six studies that described the use of AI were included in the qualitative synthesis and six studies involving 1352 patients were included in the quantitative analysis. Of these six studies, four studies assessed the utility of AI in gastric cancer diagnosis, one study assessed its utility for diagnosing esophageal cancer, and one study assessed its utility for surveillance. The pooled sensitivity and specificity were 73.4% (64.6-80.7) and 89.7% (82.7-94.1), respectively. CONCLUSIONS:AI systems have shown promise in diagnosing and monitoring esophageal and gastric cancer, particularly when combined with existing diagnostic methods. Further work is needed to further develop systems of greater accuracy and greater consideration of the clinical workflows that they aim to integrate within.
10.1245/s10434-021-10882-6
Artificial Intelligence in Upper Gastrointestinal Endoscopy.
Digestive diseases (Basel, Switzerland)
BACKGROUND:Over the past decade, several artificial intelligence (AI) systems are developed to assist in endoscopic assessment of (pre-)cancerous lesions of the gastrointestinal (GI) tract. In this review, we aimed to provide an overview of the possible indications of AI technology in upper GI endoscopy and hypothesize about potential challenges for its use in clinical practice. SUMMARY:Application of AI in upper GI endoscopy has been investigated for several indications: (1) detection, characterization, and delineation of esophageal and gastric cancer (GC) and their premalignant conditions; (2) prediction of tumor invasion; and (3) detection of Helicobacter pylori. AI systems show promising results with an accuracy of up to 99% for the detection of superficial and advanced upper GI cancers. AI outperformed trainee and experienced endoscopists for the detection of esophageal lesions and atrophic gastritis. For GC, AI outperformed mid-level and trainee endoscopists but not expert endoscopists. KEY MESSAGES:Application of artificial intelligence (AI) in upper gastrointestinal endoscopy may improve early diagnosis of esophageal and gastric cancer and may enable endoscopists to better identify patients eligible for endoscopic resection. The benefit of AI on the quality of upper endoscopy still needs to be demonstrated, while prospective trials are needed to confirm accuracy and feasibility during real-time daily endoscopy.
10.1159/000518232
Mutational signatures in esophageal adenocarcinoma define etiologically distinct subgroups with therapeutic relevance.
Nature genetics
Esophageal adenocarcinoma (EAC) has a poor outcome, and targeted therapy trials have thus far been disappointing owing to a lack of robust stratification methods. Whole-genome sequencing (WGS) analysis of 129 cases demonstrated that this is a heterogeneous cancer dominated by copy number alterations with frequent large-scale rearrangements. Co-amplification of receptor tyrosine kinases (RTKs) and/or downstream mitogenic activation is almost ubiquitous; thus tailored combination RTK inhibitor (RTKi) therapy might be required, as we demonstrate in vitro. However, mutational signatures showed three distinct molecular subtypes with potential therapeutic relevance, which we verified in an independent cohort (n = 87): (i) enrichment for BRCA signature with prevalent defects in the homologous recombination pathway; (ii) dominant T>G mutational pattern associated with a high mutational load and neoantigen burden; and (iii) C>A/T mutational pattern with evidence of an aging imprint. These subtypes could be ascertained using a clinically applicable sequencing strategy (low coverage) as a basis for therapy selection.
10.1038/ng.3659
Classification for invasion depth of esophageal squamous cell carcinoma using a deep neural network compared with experienced endoscopists.
Nakagawa Kentaro,Ishihara Ryu,Aoyama Kazuharu,Ohmori Masayasu,Nakahira Hiroko,Matsuura Noriko,Shichijo Satoki,Nishida Tsutomu,Yamada Takuya,Yamaguchi Shinjiro,Ogiyama Hideharu,Egawa Satoshi,Kishida Osamu,Tada Tomohiro
Gastrointestinal endoscopy
BACKGROUND AND AIMS:Cancer invasion depth is a critical factor affecting the choice of treatment in patients with superficial squamous cell carcinoma (SCC). However, the diagnosis of invasion depth is currently subjective and liable to interobserver variability. METHODS:We developed a deep learning-based artificial intelligence (AI) system based on Single Shot MultiBox Detector architecture for the assessment of superficial esophageal SCC. We obtained endoscopic images from patients with superficial esophageal SCC at our facility between December 2005 and December 2016. RESULTS:After excluding poor-quality images, 8660 non-magnified endoscopic (non-ME) and 5678 ME images from 804 superficial esophageal SCCs with pathologic proof of cancer invasion depth were used as the training dataset, and 405 non-ME images and 509 ME images from 155 patients were selected for the validation set. Our system showed a sensitivity of 90.1%, specificity of 95.8%, positive predictive value of 99.2%, negative predictive value of 63.9%, and an accuracy of 91.0% for differentiating pathologic mucosal and submucosal microinvasive (SM1) cancers from submucosal deep invasive (SM2/3) cancers. Cancer invasion depth was diagnosed by 16 experienced endoscopists using the same validation set, with an overall sensitivity of 89.8%, specificity of 88.3%, positive predictive value of 97.9%, negative predictive value of 65.5%, and an accuracy of 89.6%. CONCLUSIONS:This newly developed AI system showed favorable performance for diagnosing invasion depth in patients with superficial esophageal SCC, with comparable performance to experienced endoscopists.
10.1016/j.gie.2019.04.245
Artificial intelligence diagnostic system predicts multiple Lugol-voiding lesions in the esophagus and patients at high risk for esophageal squamous cell carcinoma.
Ikenoyama Yohei,Yoshio Toshiyuki,Tokura Junki,Naito Sakiko,Namikawa Ken,Tokai Yoshitaka,Yoshimizu Shoichi,Horiuchi Yusuke,Ishiyama Akiyoshi,Hirasawa Toshiaki,Tsuchida Tomohiro,Katayama Naoyuki,Tada Tomohiro,Fujisaki Junko
Endoscopy
BACKGROUND:It is known that an esophagus with multiple Lugol-voiding lesions (LVLs) after iodine staining is high risk for esophageal cancer; however, it is preferable to identify high-risk cases without staining because iodine causes discomfort and prolongs examination times. This study assessed the capability of an artificial intelligence (AI) system to predict multiple LVLs from images that had not been stained with iodine as well as patients at high risk for esophageal cancer. METHODS:We constructed the AI system by preparing a training set of 6634 images from white-light and narrow-band imaging in 595 patients before they underwent endoscopic examination with iodine staining. Diagnostic performance was evaluated on an independent validation dataset (667 images from 72 patients) and compared with that of 10 experienced endoscopists. RESULTS:The sensitivity, specificity, and accuracy of the AI system to predict multiple LVLs were 84.4 %, 70.0 %, and 76.4 %, respectively, compared with 46.9 %, 77.5 %, and 63.9 %, respectively, for the endoscopists. The AI system had significantly higher sensitivity than 9/10 experienced endoscopists. We also identified six endoscopic findings that were significantly more frequent in patients with multiple LVLs; however, the AI system had greater sensitivity than these findings for the prediction of multiple LVLs. Moreover, patients with AI-predicted multiple LVLs had significantly more cancers in the esophagus and head and neck than patients without predicted multiple LVLs. CONCLUSION:The AI system could predict multiple LVLs with high sensitivity from images without iodine staining. The system could enable endoscopists to apply iodine staining more judiciously.
10.1055/a-1334-4053
Utility of an artificial intelligence system for classification of esophageal lesions when simulating its clinical use.
Scientific reports
Previous reports have shown favorable performance of artificial intelligence (AI) systems for diagnosing esophageal squamous cell carcinoma (ESCC) compared with endoscopists. However, these findings don't reflect performance in clinical situations, as endoscopists classify lesions based on both magnified and non-magnified videos, while AI systems often use only a few magnified narrow band imaging (NBI) still images. We evaluated the performance of the AI system in simulated clinical situations. We used 25,048 images from 1433 superficial ESCC and 4746 images from 410 noncancerous esophagi to construct our AI system. For the validation dataset, we took NBI videos of suspected superficial ESCCs. The AI system diagnosis used one magnified still image taken from each video, while 19 endoscopists used whole videos. We used 147 videos and still images including 83 superficial ESCC and 64 non-ESCC lesions. The accuracy, sensitivity and specificity for the classification of ESCC were, respectively, 80.9% [95% CI 73.6-87.0], 85.5% [76.1-92.3], and 75.0% [62.6-85.0] for the AI system and 69.2% [66.4-72.1], 67.5% [61.4-73.6], and 71.5% [61.9-81.0] for the endoscopists. The AI system correctly classified all ESCCs invading the muscularis mucosa or submucosa and 96.8% of lesions ≥ 20 mm, whereas even the experts diagnosed some of them as non-ESCCs. Our AI system showed higher accuracy for classifying ESCC and non-ESCC than endoscopists. It may provide valuable diagnostic support to endoscopists.
10.1038/s41598-022-10739-2
Real-time assessment of video images for esophageal squamous cell carcinoma invasion depth using artificial intelligence.
Journal of gastroenterology
BACKGROUND:Although optimal treatment of superficial esophageal squamous cell carcinoma (SCC) requires accurate evaluation of cancer invasion depth, the current process is rather subjective and may vary by observer. We, therefore, aimed to develop an AI system to calculate cancer invasion depth. METHODS:We gathered and selected 23,977 images (6857 WLI and 17,120 NBI/BLI images) of pathologically proven superficial esophageal SCC from endoscopic videos and still images of superficial esophageal SCC taken in our facility, to use as a learning dataset. We annotated the images with information [such as magnified endoscopy (ME) or non-ME, pEP-LPM, pMM, pSM1, and pSM2-3 cancers] based on pathologic diagnosis of the resected specimens. We created a model using a convolutional neural network. Performance of the AI system was compared with that of invited experts who used the same validation video set, independent of the learning dataset. RESULTS:Accuracy, sensitivity, and specificity with non-magnified endoscopy (ME) were 87%, 50%, and 99% for the AI system and 85%, 45%, 97% for the experts. Accuracy, sensitivity, and specificity with ME were 89%, 71%, and 95% for the AI system and 84%, 42%, 97% for the experts. CONCLUSIONS:Most diagnostic parameters were higher when done by the AI system than by the experts. These results suggest that our AI system could potentially provide useful support during endoscopies.
10.1007/s00535-020-01716-5
Evaluation of Endoscopic Response Using Deep Neural Network in Esophageal Cancer Patients Who Received Neoadjuvant Chemotherapy.
Annals of surgical oncology
BACKGROUND:We previously reported that endoscopic response evaluation can preoperatively predict the prognosis and distribution of residual tumors after neoadjuvant chemotherapy (NAC). In this study, we developed artificial intelligence (AI)-guided endoscopic response evaluation using a deep neural network to discriminate endoscopic responders (ERs) in patients with esophageal squamous cell carcinoma (ESCC) after NAC. METHOD:Surgically resectable ESCC patients who underwent esophagectomy following NAC were retrospectively analyzed in this study. Endoscopic images of the tumors were analyzed using a deep neural network. The model was validated with a test data set using 10 newly collected ERs and 10 newly collected non-ER images. The sensitivity, specificity, positive predictive value (PPV), and negative predictive value (NPV) of the endoscopic response evaluation by AI and endoscopists were calculated and compared. RESULTS:Of 193 patients, 40 (21%) were diagnosed as ERs. The median sensitivity, specificity, PPV, and NPV values for ER detection in 10 models were 60%, 100%, 100%, and 71%, respectively. Similarly, the median values by the endoscopist were 80%, 80%, 81%, and 81%, respectively. CONCLUSION:This proof-of-concept study using a deep learning algorithm demonstrated that the constructed AI-guided endoscopic response evaluation after NAC could identify ER with high specificity and PPV. It would appropriately guide an individualized treatment strategy that includes an organ preservation approach in ESCC patients.
10.1245/s10434-023-13140-z
A single-center prospective study evaluating the usefulness of artificial intelligence for the diagnosis of esophageal squamous cell carcinoma in a real-time setting.
BMC gastroenterology
BACKGROUND:Several pre-clinical studies have reported the usefulness of artificial intelligence (AI) systems in the diagnosis of esophageal squamous cell carcinoma (ESCC). We conducted this study to evaluate the usefulness of an AI system for real-time diagnosis of ESCC in a clinical setting. METHODS:This study followed a single-center prospective single-arm non-inferiority design. Patients at high risk for ESCC were recruited and real-time diagnosis by the AI system was compared with that of endoscopists for lesions suspected to be ESCC. The primary outcomes were the diagnostic accuracy of the AI system and endoscopists. The secondary outcomes were sensitivity, specificity, positive predictive value (PPV), negative predictive value (NPV), and adverse events. RESULTS:A total of 237 lesions were evaluated. The accuracy, sensitivity, and specificity of the AI system were 80.6%, 68.2%, and 83.4%, respectively. The accuracy, sensitivity, and specificity of endoscopists were 85.7%, 61.4%, and 91.2%, respectively. The difference between the accuracy of the AI system and that of the endoscopists was - 5.1%, and the lower limit of the 90% confidence interval was less than the non-inferiority margin. CONCLUSIONS:The non-inferiority of the AI system in comparison with endoscopists in the real-time diagnosis of ESCC in a clinical setting was not proven. TRIAL REGISTRATION:Japan Registry of Clinical Trials (jRCTs052200015, 18/05/2020).
10.1186/s12876-023-02788-2
Artificial intelligence for tumour tissue detection and histological regression grading in oesophageal adenocarcinomas: a retrospective algorithm development and validation study.
The Lancet. Digital health
BACKGROUND:Oesophageal adenocarcinoma and adenocarcinoma of the oesophagogastric junction are among the most common malignant epithelial tumours. Most patients receive neoadjuvant therapy before complete tumour resection. Histological assessment after resection includes identification of residual tumour tissue and areas of regressive tumour, data which are used to calculate a clinically relevant regression score. We developed an artificial intelligence (AI) algorithm for tumour tissue detection and tumour regression grading in surgical specimens from patients with oesophageal adenocarcinoma or adenocarcinoma of the oesophagogastric junction. METHODS:We used one training cohort and four independent test cohorts to develop, train, and validate a deep learning tool. The material consisted of histological slides from surgically resected specimens from patients with oesophageal adenocarcinoma and adenocarcinoma of the oesophagogastric junction from three pathology institutes (two in Germany, one in Austria) and oesophageal cancer cohort of The Cancer Genome Atlas (TCGA). All slides were from neoadjuvantly treated patients except for those from the TCGA cohort, who were neoadjuvant-therapy naive. Data from training cohort and test cohort cases were extensively manually annotated for 11 tissue classes. A convolutional neural network was trained on the data using a supervised principle. First, the tool was formally validated using manually annotated test datasets. Next, tumour regression grading was assessed in a retrospective cohort of post-neoadjuvant therapy surgical specimens. The grading of the algorithm was compared with that of a group of 12 board-certified pathologists from one department. To further validate the tool, three pathologists processed whole resection cases with and without AI assistance. FINDINGS:Of the four test cohorts, one included 22 manually annotated histological slides (n=20 patients), one included 62 sides (n=15), one included 214 slides (n=69), and the final one included 22 manually annotated histological slides (n=22). In the independent test cohorts the AI tool had high patch-level accuracy for identifying both tumour and regression tissue. When we validated the concordance of the AI tool against analyses by a group of pathologists (n=12), agreement was 63·6% (quadratic kappa 0·749; p<0·0001) at case level. The AI-based regression grading triggered true reclassification of resected tumour slides in seven cases (including six cases who had small tumour regions that were initially missed by pathologists). Use of the AI tool by three pathologists increased interobserver agreement and substantially reduced diagnostic time per case compared with working without AI assistance. INTERPRETATION:Use of our AI tool in the diagnostics of oesophageal adenocarcinoma resection specimens by pathologists increased diagnostic accuracy, interobserver concordance, and significantly reduced assessment time. Prospective validation of the tool is required. FUNDING:North Rhine-Westphalia state, Federal Ministry of Education and Research of Germany, and the Wilhelm Sander Foundation.
10.1016/S2589-7500(23)00027-4
Efficiency of endoscopic artificial intelligence in the diagnosis of early esophageal cancer.
Thoracic cancer
BACKGROUND:The accuracy of artificial intelligence (AI) and experts in diagnosing early esophageal cancer (EC) and its infiltration depth was summarized and analyzed, thus identifying the advantages of AI over traditional manual diagnosis, with a view to more accurately assisting doctors in evaluating the patients' conditions and improving their cure and survival rates. METHODS:The PubMed, EMBASE, Cochrane, Google, and CNKI databases were searched for relevant literature related to AI diagnosis of early EC and its invasion depth published before August 2023. Summary analysis of pooled sensitivity, specificity, summary receiver operating characteristics (SROC) and area under the curve (AUC) of AI in diagnosing early EC were performed, and Review Manager and Stata were adopted for data analysis. RESULTS:A total of 19 studies were enrolled with a low to moderate total risk of bias. The pooled sensitivity of AI for diagnosing early EC was markedly higher than that of novices and comparable to that of endoscopists. Moreover, AI predicted early EC with markedly higher AUCs than novices and experts (0.93 vs. 0.74 vs. 0.89). In addition, pooled sensitivity and specificity in the diagnosis of invasion depth in early EC were higher than that of experts, with AUCs of 0.97 and 0.92, respectively. CONCLUSION:AI-assistance can diagnose early EC and its infiltration depth more accurately, which can help in its early intervention and the customization of personalized treatment plans. Therefore, AI systems have great potential in the early diagnosis of EC.
10.1111/1759-7714.15261
Artificial intelligence-assisted endoscopic detection of esophageal neoplasia in early stage: The next step?
Liu Yong
World journal of gastroenterology
Esophageal cancer (EC) is a common malignant tumor of the digestive tract and originates from the epithelium of the esophageal mucosa. It has been confirmed that early EC lesions can be cured by endoscopic therapy, and the curative effect is equivalent to that of surgical operation. Upper gastrointestinal endoscopy is still the gold standard for EC diagnosis. The accuracy of endoscopic examination results largely depends on the professional level of the examiner. Artificial intelligence (AI) has been applied in the screening of early EC and has shown advantages; notably, it is more accurate than less-experienced endoscopists. This paper reviews the application of AI in the field of endoscopic detection of early EC, including squamous cell carcinoma and adenocarcinoma, and describes the relevant progress. Although up to now most of the studies evaluating the clinical application of AI in early EC endoscopic detection are focused on still images, AI-assisted real-time detection based on live-stream video may be the next step.
10.3748/wjg.v27.i14.1392
Convolutional neural network-based artificial intelligence for the diagnosis of early esophageal cancer based on endoscopic images: A meta-analysis.
Saudi journal of gastroenterology : official journal of the Saudi Gastroenterology Association
Background:Early screening and treatment of esophageal cancer (EC) is particularly important for the survival and prognosis of patients. However, early EC is difficult to diagnose by a routine endoscopic examination. Therefore, convolutional neural network (CNN)-based artificial intelligence (AI) has become a very promising method in the diagnosis of early EC using endoscopic images. The aim of this study was to evaluate the diagnostic performance of CNN-based AI for detecting early EC based on endoscopic images. Methods:A comprehensive search was performed to identify relevant English articles concerning CNN-based AI in the diagnosis of early EC based on endoscopic images (from the date of database establishment to April 2022). The pooled sensitivity (SEN), pooled specificity (SPE), positive likelihood ratio (LR+), negative likelihood ratio (LR-), diagnostic odds ratio (DOR) with 95% confidence interval (CI), summary receiver operating characteristic (SROC) curve, and area under the curve (AUC) for the accuracy of CNN-based AI in the diagnosis of early EC based on endoscopic images were calculated. We used the I test to assess heterogeneity and investigated the source of heterogeneity by performing meta-regression analysis. Publication bias was assessed using Deeks' funnel plot asymmetry test. Results:Seven studies met the eligibility criteria. The SEN and SPE were 0.90 (95% confidence interval [CI]: 0.82-0.94) and 0.91 (95% CI: 0.79-0.96), respectively. The LR+ of the malignant ultrasonic features was 9.8 (95% CI: 3.8-24.8) and the LR- was 0.11 (95% CI: 0.06-0.21), revealing that CNN-based AI exhibited an excellent ability to confirm or exclude early EC on endoscopic images. Additionally, SROC curves showed that the AUC of the CNN-based AI in the diagnosis of early EC based on endoscopic images was 0.95 (95% CI: 0.93-0.97), demonstrating that CNN-based AI has good diagnostic value for early EC based on endoscopic images. Conclusions:Based on our meta-analysis, CNN-based AI is an excellent diagnostic tool with high sensitivity, specificity, and AUC in the diagnosis of early EC based on endoscopic images.
10.4103/sjg.sjg_178_22
Performance of radiomics-based artificial intelligence systems in the diagnosis and prediction of treatment response and survival in esophageal cancer: a systematic review and meta-analysis of diagnostic accuracy.
Diseases of the esophagus : official journal of the International Society for Diseases of the Esophagus
Radiomics can interpret radiological images with more detail and in less time compared to the human eye. Some challenges in managing esophageal cancer can be addressed by incorporating radiomics into image interpretation, treatment planning, and predicting response and survival. This systematic review and meta-analysis provides a summary of the evidence of radiomics in esophageal cancer. The systematic review was carried out using Pubmed, MEDLINE, and Ovid EMBASE databases-articles describing radiomics in esophageal cancer were included. A meta-analysis was also performed; 50 studies were included. For the assessment of treatment response using 18F-FDG PET/computed tomography (CT) scans, seven studies (443 patients) were included in the meta-analysis. The pooled sensitivity and specificity were 86.5% (81.1-90.6) and 87.1% (78.0-92.8). For the assessment of treatment response using CT scans, five studies (625 patients) were included in the meta-analysis, with a pooled sensitivity and specificity of 86.7% (81.4-90.7) and 76.1% (69.9-81.4). The remaining 37 studies formed the qualitative review, discussing radiomics in diagnosis, radiotherapy planning, and survival prediction. This review explores the wide-ranging possibilities of radiomics in esophageal cancer management. The sensitivities of 18F-FDG PET/CT scans and CT scans are comparable, but 18F-FDG PET/CT scans have improved specificity for AI-based prediction of treatment response. Models integrating clinical and radiomic features facilitate diagnosis and survival prediction. More research is required into comparing models and conducting large-scale studies to build a robust evidence base.
10.1093/dote/doad034
Recent advances in early esophageal cancer: diagnosis and treatment based on endoscopy.
Yang Hang,Hu Bing
Postgraduate medicine
Esophageal cancer (EC) often cannot be discovered in time because of its asymptomatic or symptom-atypical characteristics in early stage. The risk and probability of lymph node metastasis and distant metastasis increase correspondingly as the cancer aggressively invades deeper layers. Treatment regimens may be shifted to surgery and chemoradiotherapy (CRT) from endoscopic eradication therapy (EET) with poor quality of life and prognosis. It is imperative to identify dysplasia and EC early and enable early curative endoscopic treatments. Newer methods have been attempted in the clinical setting to achieve early detection at a more microscopic and precise level. Newer imaging techniques and artificial intelligence (AI) technology have been involved in targeted biopsies and will gradually unveil the visualization of pathology in the future. Early detection and diagnosis are the prerequisite to choose personal and precise treatment regimens. EET has also been undergoing development and improvement to benefit more patients as the first option or the firstly chosen alternative therapy, when compared with esophagectomy. More clinical studies are needed to provide more possibilities for EET.
10.1080/00325481.2021.1934495
Diagnostic outcomes of esophageal cancer by artificial intelligence using convolutional neural networks.
Horie Yoshimasa,Yoshio Toshiyuki,Aoyama Kazuharu,Yoshimizu Shoichi,Horiuchi Yusuke,Ishiyama Akiyoshi,Hirasawa Toshiaki,Tsuchida Tomohiro,Ozawa Tsuyoshi,Ishihara Soichiro,Kumagai Youichi,Fujishiro Mitsuhiro,Maetani Iruru,Fujisaki Junko,Tada Tomohiro
Gastrointestinal endoscopy
BACKGROUND AND AIMS:The prognosis of esophageal cancer is relatively poor. Patients are usually diagnosed at an advanced stage when it is often too late for effective treatment. Recently, artificial intelligence (AI) using deep learning has made remarkable progress in medicine. However, there are no reports on its application for diagnosing esophageal cancer. Here, we demonstrate the diagnostic ability of AI to detect esophageal cancer including squamous cell carcinoma and adenocarcinoma. METHODS:We retrospectively collected 8428 training images of esophageal cancer from 384 patients at the Cancer Institute Hospital, Japan. Using these, we developed deep learning through convolutional neural networks (CNNs). We also prepared 1118 test images for 47 patients with 49 esophageal cancers and 50 patients without esophageal cancer to evaluate the diagnostic accuracy. RESULTS:The CNN took 27 seconds to analyze 1118 test images and correctly detected esophageal cancer cases with a sensitivity of 98%. CNN could detect all 7 small cancer lesions less than 10 mm in size. Although the positive predictive value for each image was 40%, misdiagnosing shadows and normal structures led to a negative predictive value of 95%. The CNN could distinguish superficial esophageal cancer from advanced cancer with an accuracy of 98%. CONCLUSIONS:The constructed CNN system for detecting esophageal cancer can analyze stored endoscopic images in a short time with high sensitivity. However, more training would lead to higher diagnostic accuracy. This system can facilitate early detection in practice, leading to a better prognosis in the near future.
10.1016/j.gie.2018.07.037
The role of artificial intelligence in the endoscopic diagnosis of esophageal cancer: a systematic review and meta-analysis.
Diseases of the esophagus : official journal of the International Society for Diseases of the Esophagus
Early detection of esophageal cancer is limited by accurate endoscopic diagnosis of subtle macroscopic lesions. Endoscopic interpretation is subject to expertise, diagnostic skill, and thus human error. Artificial intelligence (AI) in endoscopy is increasingly bridging this gap. This systematic review and meta-analysis consolidate the evidence on the use of AI in the endoscopic diagnosis of esophageal cancer. The systematic review was carried out using Pubmed, MEDLINE and Ovid EMBASE databases and articles on the role of AI in the endoscopic diagnosis of esophageal cancer management were included. A meta-analysis was also performed. Fourteen studies (1590 patients) assessed the use of AI in endoscopic diagnosis of esophageal squamous cell carcinoma-the pooled sensitivity and specificity were 91.2% (84.3-95.2%) and 80% (64.3-89.9%). Nine studies (478 patients) assessed AI capabilities of diagnosing esophageal adenocarcinoma with the pooled sensitivity and specificity of 93.1% (86.8-96.4) and 86.9% (81.7-90.7). The remaining studies formed the qualitative summary. AI technology, as an adjunct to endoscopy, can assist in accurate, early detection of esophageal malignancy. It has shown superior results to endoscopists alone in identifying early cancer and assessing depth of tumor invasion, with the added benefit of not requiring a specialized skill set. Despite promising results, the application in real-time endoscopy is limited, and further multicenter trials are required to accurately assess its use in routine practice.
10.1093/dote/doad048
Artificial intelligence technique in detection of early esophageal cancer.
Huang Lu-Ming,Yang Wen-Juan,Huang Zhi-Yin,Tang Cheng-Wei,Li Jing
World journal of gastroenterology
Due to the rapid progression and poor prognosis of esophageal cancer (EC), the early detection and diagnosis of early EC are of great value for the prognosis improvement of patients. However, the endoscopic detection of early EC, especially Barrett's dysplasia or squamous epithelial dysplasia, is difficult. Therefore, the requirement for more efficient methods of detection and characterization of early EC has led to intensive research in the field of artificial intelligence (AI). Deep learning (DL) has brought about breakthroughs in processing images, videos, and other aspects, whereas convolutional neural networks (CNNs) have shone lights on detection of endoscopic images and videos. Many studies on CNNs in endoscopic analysis of early EC demonstrate excellent performance including sensitivity and specificity and progress gradually from image analysis for classification to real-time detection of early esophageal neoplasia. When AI technique comes to the pathological diagnosis, borderline lesions that are difficult to determine may become easier than before. In gene diagnosis, due to the lack of tissue specificity of gene diagnostic markers, they can only be used as supplementary measures at present. In predicting the risk of cancer, there is still a lack of prospective clinical research to confirm the accuracy of the risk stratification model.
10.3748/wjg.v26.i39.5959
Artificial intelligence-assisted esophageal cancer management: Now and future.
Zhang Yu-Hang,Guo Lin-Jie,Yuan Xiang-Lei,Hu Bing
World journal of gastroenterology
Esophageal cancer poses diagnostic, therapeutic and economic burdens in high-risk regions. Artificial intelligence (AI) has been developed for diagnosis and outcome prediction using various features, including clinicopathologic, radiologic, and genetic variables, which can achieve inspiring results. One of the most recent tasks of AI is to use state-of-the-art deep learning technique to detect both early esophageal squamous cell carcinoma and esophageal adenocarcinoma in Barrett's esophagus. In this review, we aim to provide a comprehensive overview of the ways in which AI may help physicians diagnose advanced cancer and make clinical decisions based on predicted outcomes, and combine the endoscopic images to detect precancerous lesions or early cancer. Pertinent studies conducted in recent two years have surged in numbers, with large datasets and external validation from multi-centers, and have partly achieved intriguing results of expert's performance of AI in real time. Improved pre-trained computer-aided diagnosis algorithms in the future studies with larger training and external validation datasets, aiming at real-time video processing, are imperative to produce a diagnostic efficacy similar to or even superior to experienced endoscopists. Meanwhile, supervised randomized controlled trials in real clinical practice are highly essential for a solid conclusion, which meets patient-centered satisfaction. Notably, ethical and legal issues regarding the black-box nature of computer algorithms should be addressed, for both clinicians and regulators.
10.3748/wjg.v26.i35.5256
Artificial Intelligence and Deep Learning for Upper Gastrointestinal Neoplasia.
Gastroenterology
Upper gastrointestinal (GI) neoplasia account for 35% of GI cancers and 1.5 million cancer-related deaths every year. Despite its efficacy in preventing cancer mortality, diagnostic upper GI endoscopy is affected by a substantial miss rate of neoplastic lesions due to failure to recognize a visible lesion or imperfect navigation. This may be offset by the real-time application of artificial intelligence (AI) for detection (computer-aided detection [CADe]) and characterization (computer-aided diagnosis [CADx]) of upper GI neoplasia. Stand-alone performance of CADe for esophageal squamous cell neoplasia, Barrett's esophagus-related neoplasia, and gastric cancer showed promising accuracy, sensitivity ranging between 83% and 93%. However, incorporation of CADe/CADx in clinical practice depends on several factors, such as possible bias in the training or validation phases of these algorithms, its interaction with human endoscopists, and clinical implications of false-positive results. The aim of this review is to guide the clinician across the multiple steps of AI development in clinical practice.
10.1053/j.gastro.2021.11.040