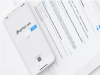
EEG Emotion Recognition Applied to the Effect Analysis of Music on Emotion Changes in Psychological Healthcare.
International journal of environmental research and public health
Music therapy is increasingly being used to promote physical health. Emotion semantic recognition is more objective and provides direct awareness of the real emotional state based on electroencephalogram (EEG) signals. Therefore, we proposed a music therapy method to carry out emotion semantic matching between the EEG signal and music audio signal, which can improve the reliability of emotional judgments, and, furthermore, deeply mine the potential influence correlations between music and emotions. Our proposed EER model (EEG-based Emotion Recognition Model) could identify 20 types of emotions based on 32 EEG channels, and the average recognition accuracy was above 90% and 80%, respectively. Our proposed music-based emotion classification model (MEC model) could classify eight typical emotion types of music based on nine music feature combinations, and the average classification accuracy was above 90%. In addition, the semantic mapping was analyzed according to the influence of different music types on emotional changes from different perspectives based on the two models, and the results showed that the joy type of music video could improve fear, disgust, mania, and trust emotions into surprise or intimacy emotions, while the sad type of music video could reduce intimacy to the fear emotion.
10.3390/ijerph20010378
Microstate analysis in infancy.
Infant behavior & development
BACKGROUND:Microstate analysis is an emerging method for investigating global brain connections using electroencephalography (EEG). Microstates have been colloquially referred to as the "atom of thought," meaning that from these underlying networks comes coordinated neural processing and cognition. The present study examined microstates at 6-, 8-, and 10-months of age. It was hypothesized that infants would demonstrate distinct microstates comparable to those identified in adults that also parallel resting-state networks using fMRI. An additional exploratory aim was to examine the relationship between microstates and temperament, assessed via parent reports, to further demonstrate microstate analysis as a viable tool for examining the relationship between neural networks, cognitive processes as well as emotional expression embodied in temperament attributes. METHODS:The microstates analysis was performed with infant EEG data when the infant was either 6- (n = 12), 8- (n = 16), or 10-months (n = 6) old. The resting-state task involved watching a 1-minute video segment of Baby Einstein while listening to the accompanying music. Parents completed the IBQ-R to assess infant temperament. RESULTS:Four microstate topographies were extracted. Microstate 1 had an isolated posterior activation; Microstate 2 had a symmetric occipital to prefrontal orientation; Microstate 3 had a left occipital to right frontal orientation; and Microstate 4 had a right occipital to left frontal orientation. At 10-months old, Microstate 3, thought to reflect auditory/language processing, became activated more often, for longer periods of time, covering significantly more time across the task and was more likely to be transitioned into. This finding is interpreted as consistent with language acquisition and phonological processing that emerges around 10-months. Microstate topographies and parameters were also correlated with differing temperament broadband and narrowband scales on the IBQ-R. CONCLUSION:Three microstates emerged that appear comparable to underlying networks identified in adult and infant microstate literature and fMRI studies. Each of the temperament domains was related to specific microstates and their parameters. These networks also correspond with auditory and visual processing as well as the default mode network found in prior research and can lead to new investigations examining differences across stimulus presentations to further explain how infants begin to recognize, respond to, and engage with the world around them.
10.1016/j.infbeh.2022.101785
A Large Finer-grained Affective Computing EEG Dataset.
Scientific data
Affective computing based on electroencephalogram (EEG) has gained increasing attention for its objectivity in measuring emotional states. While positive emotions play a crucial role in various real-world applications, such as human-computer interactions, the state-of-the-art EEG datasets have primarily focused on negative emotions, with less consideration given to positive emotions. Meanwhile, these datasets usually have a relatively small sample size, limiting exploration of the important issue of cross-subject affective computing. The proposed Finer-grained Affective Computing EEG Dataset (FACED) aimed to address these issues by recording 32-channel EEG signals from 123 subjects. During the experiment, subjects watched 28 emotion-elicitation video clips covering nine emotion categories (amusement, inspiration, joy, tenderness; anger, fear, disgust, sadness, and neutral emotion), providing a fine-grained and balanced categorization on both the positive and negative sides of emotion. The validation results show that emotion categories can be effectively recognized based on EEG signals at both the intra-subject and the cross-subject levels. The FACED dataset is expected to contribute to developing EEG-based affective computing algorithms for real-world applications.
10.1038/s41597-023-02650-w
Decoding Emotions From EEG Responses Elicited by Videos Using Machine Learning Techniques on Two Datasets.
Annual International Conference of the IEEE Engineering in Medicine and Biology Society. IEEE Engineering in Medicine and Biology Society. Annual International Conference
In recent times, we have seen extensive research in the field of EEG-based emotion identification. The majority of solutions suggested by current literature use sophisticated deep learning techniques for the identification of human emotions. These models are very complex and need huge resources to implement. Hence, in this work, a method for human emotion recognition is proposed which is based on much simpler architecture. For that, two publicly available datasets SEED and DEAP are used to perform experiments. First, the EEG signals of the two datasets are segmented into epochs of 1second duration. The epochs are also decomposed into different brain rhythms. The features computation is performed in two different ways, one is directly from the epochs and the other way is from the brain rhythms obtained after the decomposition of the epochs. Several features and their combination are examined with different classifiers. For the DEAP dataset baseline features are also utilised. It is observed that the support vector machine (SVM) has shown the best performance for the DEAP dataset when baseline feature correction and epoch decomposition are implemented together. The best achieved average accuracy is 96.50% and 96.71% for high versus low valence classes and high versus low arousal classes, respectively. For the SEED dataset, the best average accuracy of 86.89% is achieved using the multilayer perceptron (MLP) with 2 hidden layers.Clinical relevance- This work can be further explored to develop an automated mental health monitor which can assist doctors in their primary screening.
10.1109/EMBC40787.2023.10341106
Game induced emotion analysis using electroencephalography.
Computers in biology and medicine
Organizations vie to develop insights into the psychological aspects of consumer decision-making to enhance their products accordingly. Understanding how emotions and personality traits influence the choices we make is an integral part of product design. In this paper, we have employed machine learning algorithms to profile discrete emotions, in response to video games stimuli, based on features extracted from recorded electroencephalography (EEG) and to understand certain characteristics of personality. Four video games from different genres have been used for emotion elicitation and players' EEG signals are recorded. EEG being a non-stationary, non-linear and extremely noisy signal has been cleaned using a Savitzky-Golay filter which is found to be suitable for single-channel EEG devices. Seven out of sixteen features from time, frequency and time-frequency domains have been selected using Random Forest and used to classify emotions. Support Vector Machine, k-Nearest Neighbour and Gradient Boosted Trees classifiers have been used where the highest classification accuracy 82.26% is achieved with Boosted Trees classifier. Our findings propagate that for a single-channel EEG device, only four discrete emotions (happy, bored, relaxed, stressed) can be classified where two emotions happy and bored achieved the highest individual accuracy of 88.89% and 85.29% respectively with the Gradient Boosted Trees Classifier. In this study, we have also identified personality traits, extroversion and neuroticism influence players' perception of video games. The results indicate that players with low extroversion prefer relatively slow and strategy games as compared to highly extroverted. It has also been identified that puzzle and racing games are well-liked irrespective of the levels of the two personality traits.
10.1016/j.compbiomed.2022.105441
EEG microstate correlates of emotion dynamics and stimulation content during video watching.
Cerebral cortex (New York, N.Y. : 1991)
INTRODUCTION:EEG microstates have been widely adopted to understand the complex and dynamic-changing process in dynamic brain systems, but how microstates are temporally modulated by emotion dynamics is still unclear. An investigation of EEG microstates under video-evoking emotion dynamics modulation would provide a novel insight into the understanding of temporal dynamics of functional brain networks. METHODS:In the present study, we postulate that emotional states dynamically modulate the microstate patterns, and perform an in-depth investigation between EEG microstates and emotion dynamics under a video-watching task. By mapping from subjective-experienced emotion states and objective-presented stimulation content to EEG microstates, we gauge the comprehensive associations among microstates, emotions, and multimedia stimulation. RESULTS:The results show that emotion dynamics could be well revealed by four EEG microstates (MS1, MS2, MS3, and MS4), where MS3 and MS4 are found to be highly correlated to different emotion states (emotion task effect and level effect) and the affective information involved in the multimedia content (visual and audio). CONCLUSION:In this work, we reveal the microstate patterns related to emotion dynamics from sensory and stimulation dimensions, which deepens the understanding of the neural representation under emotion dynamics modulation and will be beneficial for the future study of brain dynamic systems.
10.1093/cercor/bhac082
An Investigation of Olfactory-Enhanced Video on EEG-Based Emotion Recognition.
IEEE transactions on neural systems and rehabilitation engineering : a publication of the IEEE Engineering in Medicine and Biology Society
Collecting emotional physiological signals is significant in building affective Human-Computer Interactions (HCI). However, how to evoke subjects' emotions efficiently in EEG-related emotional experiments is still a challenge. In this work, we developed a novel experimental paradigm that allows odors dynamically participate in different stages of video-evoked emotions, to investigate the efficiency of olfactory-enhanced videos in inducing subjects' emotions; According to the period that the odors participated in, the stimuli were divided into four patterns, i.e., the olfactory-enhanced video in early/later stimulus periods (OVEP/OVLP), and the traditional videos in early/later stimulus periods (TVEP/TVLP). The differential entropy (DE) feature and four classifiers were employed to test the efficiency of emotion recognition. The best average accuracies of the OVEP, OVLP, TVEP, and TVLP were 50.54%, 51.49%, 40.22%, and 57.55%, respectively. The experimental results indicated that the OVEP significantly outperformed the TVEP on classification performance, while there was no significant difference between the OVLP and TVLP. Besides, olfactory-enhanced videos achieved higher efficiency in evoking negative emotions than traditional videos. Moreover, we found that the neural patterns in response to emotions under different stimulus methods were stable, and for Fp1, FP2, and F7, there existed significant differences in whether adopt the odors.
10.1109/TNSRE.2023.3253866
The EEG microstate representation of discrete emotions.
International journal of psychophysiology : official journal of the International Organization of Psychophysiology
Understanding how human emotions are represented in our brain is a central question in the field of affective neuroscience. While previous studies have mainly adopted a modular and static perspective on the neural representation of emotions, emerging research suggests that emotions may rely on a distributed and dynamic representation. The present study aimed to explore the EEG microstate representations for nine discrete emotions (Anger, Disgust, Fear, Sadness, Neutral, Amusement, Inspiration, Joy and Tenderness). Seventy-eight participants were recruited to watch emotion eliciting videos with their EEGs recorded. Multivariate analysis revealed that different emotions had distinct EEG microstate features. By using the EEG microstate features in the Neutral condition as the reference, the coverage of C, duration of C and occurrence of B were found to be the top-contributing microstate features for the discrete positive and negative emotions. The emotions of Disgust, Fear and Joy were found to be most effectively represented by EEG microstate. The present study provided the first piece of evidence of EEG microstate representation for discrete emotions, highlighting a whole-brain, dynamical representation of human emotions.
10.1016/j.ijpsycho.2023.02.002
Infants of depressed mothers exhibit atypical frontal brain activity: a replication and extension of previous findings.
Dawson G,Frey K,Panagiotides H,Osterling J,Hessl D
Journal of child psychology and psychiatry, and allied disciplines
The left frontal brain region is specialized for expression of positive emotions (e.g. joy) whereas the right frontal region is specialized for negative emotions (e.g. sadness). Depressed adults have been found to exhibit reduced left frontal electroencephalographic activity. In this study, baseline frontal and parietal EEG activity was measured in 13-15-month-old infants of depressed and nondepressed mothers who were of middle income with no other major psychiatric problems. Compared to infants of nondepressed mothers, infants of depressed mothers exhibited reduced left frontal EEG activity. Infants of mothers with major depression exhibited lower levels of left frontal EEG activity than those of mothers with subthreshold depression.
Brain responses for the subconscious recognition of faces.
Hoshiyama Minoru,Kakigi Ryusuke,Watanabe Shoko,Miki Kensaku,Takeshima Yasuyuki
Neuroscience research
We investigated the event-related responses following subthreshold and suprathreshold stimulation with facial and non-facial figures using magnetoencephalography (MEG) and EEG recordings to clarify the physiological nature of subconscious perception. Event-related magnetic fields and potentials were recorded from the right hemisphere in eight healthy subjects. Three types of stimulus, i.e., facial image (Face), letters of the alphabet (Letters) and random patterns of dots (Dots), with different presentation periods, subthreshold (16 ms), intermediate (32 ms) and suprathreshold (48 ms) were visually presented in a random order. A psychological discrimination task using the same stimuli was also employed. Clear MEG and EEG responses were recorded for all the stimuli, but the amplitude of the responses was largest for Face and smallest for Dots even in the subthreshold stimulation. The equivalent current dipoles (ECDs) for Face were located around the fusiform gyrus, although the correlation coefficients for ECDs were low under subthreshold and intermediate conditions. The ECDs for Letters and Dots were not estimated with reliable correlation coefficients. The results from the psychological task correlated with the dominancy of face recognition. Face perception was processed differently in the subthreshold condition as well as suprathreshold condition. The subconscious recognition of face might be processed around the fusiform gyrus.
Hyperkinetic disorder in the ICD-10: EEG evidence for a definitional widening?
Clarke A R,Barry R J,McCarthy R,Selikowitz M
European child & adolescent psychiatry
This study investigated EEG differences between children with Hyperkinetic Disorder (HKD), HKD sub-threshold attention deficit (HKDsub), and control children, in order to determine from an EEG perspective whether children with HKDsub represent a valid clinical disorder. Twenty-four boys were included in each of the three age-matched groups. The HKD group had greater total power and absolute delta and theta, more relative theta, and less relative alpha and beta than the control group. The HKDsub group had EEG profiles which were different from both control children and children with HKD, with the HKDsub group having EEG results generally between the HKD and control group. Additionally, a number of topographic differences were found in the frontal regions which suggest that the two HKD groups have independent EEG components. These results support the inclusion of a diagnostic category of attention deficit in future editions of the ICD.
10.1007/s00787-003-0315-5
An Electroencephalography Connectomic Profile of Posttraumatic Stress Disorder.
Toll Russell T,Wu Wei,Naparstek Sharon,Zhang Yu,Narayan Manjari,Patenaude Brian,De Los Angeles Carlo,Sarhadi Kasra,Anicetti Nicole,Longwell Parker,Shpigel Emmanuel,Wright Rachael,Newman Jennifer,Gonzalez Bryan,Hart Roland,Mann Silas,Abu-Amara Duna,Sarhadi Kamron,Cornelssen Carena,Marmar Charles,Etkin Amit
The American journal of psychiatry
OBJECTIVE:The authors sought to identify brain regions whose frequency-specific, orthogonalized resting-state EEG power envelope connectivity differs between combat veterans with posttraumatic stress disorder (PTSD) and healthy combat-exposed veterans, and to determine the behavioral correlates of connectomic differences. METHODS:The authors first conducted a connectivity method validation study in healthy control subjects (N=36). They then conducted a two-site case-control study of veterans with and without PTSD who were deployed to Iraq and/or Afghanistan. Healthy individuals (N=95) and those meeting full or subthreshold criteria for PTSD (N=106) underwent 64-channel resting EEG (eyes open and closed), which was then source-localized and orthogonalized to mitigate effects of volume conduction. Correlation coefficients between band-limited source-space power envelopes of different regions of interest were then calculated and corrected for multiple comparisons. Post hoc correlations of connectomic abnormalities with clinical features and performance on cognitive tasks were conducted to investigate the relevance of the dysconnectivity findings. RESULTS:Seventy-four brain region connections were significantly reduced in PTSD (all in the eyes-open condition and predominantly using the theta carrier frequency). Underconnectivity of the orbital and anterior middle frontal gyri were most prominent. Performance differences in the digit span task mapped onto connectivity between 25 of the 74 brain region pairs, including within-network connections in the dorsal attention, frontoparietal control, and ventral attention networks. CONCLUSIONS:Robust PTSD-related abnormalities were evident in theta-band source-space orthogonalized power envelope connectivity, which furthermore related to cognitive deficits in these patients. These findings establish a clinically relevant connectomic profile of PTSD using a tool that facilitates the lower-cost clinical translation of network connectivity research.
10.1176/appi.ajp.2019.18080911