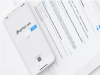
Design and Implementation of a Machine Learning Based EEG Processor for Accurate Estimation of Depth of Anesthesia.
Saadeh Wala,Khan Fatima Hameed,Altaf Muhammad Awais Bin
IEEE transactions on biomedical circuits and systems
Accurate monitoring of the depth of anesthesia (DoA) is essential for intraoperative and postoperative patient's health. Commercially available electroencephalograph (EEG)-based DoA monitors are recommended only for certain anesthetic drugs and specific age-group patients. This paper presents a machine learning classification processor for accurate DoA estimation irrespective of the patient's age and anesthetic drug. The classification is solely based on six features extracted from EEG signal, i.e., spectral edge frequency (SEF), beta ratio, and four bands of spectral energy (FBSE). A machine learning fine decision tree classifier is adopted to achieve a four-class DoA classification (deep, moderate, and light DoA versus awake state). The feature selection and the classification processor are optimized to achieve the highest classification accuracy for the state of moderate anesthesia required for the surgical operations. The proposed 256-point fast Fourier transform accelerator is implemented to realize SEF, beta ratio, and FBSE that enables minimal latency and high accuracy feature extraction. The proposed DoA processor is implemented using a 65 nm CMOS technology and experimentally verified using field programming gate array (FPGA) based on the EEG recordings of 75 patients undergoing elective surgery with different types of anesthetic agents. The processor achieves an average accuracy of 92.2% for all DoA states, with a latency of 1s The 0.09 mm DoA processor consumes 140nJ/classification.
10.1109/TBCAS.2019.2921875
Fuzzy logic based anaesthesia monitoring systems for the detection of absolute hypovolaemia.
Mansoor Baig Mirza,Gholamhosseini Hamid,Harrison Michael J
Computers in biology and medicine
Anaesthesia monitoring involves critical diagnostic tasks carried out amongst lots of distractions. Computers are capable of handling large amounts of data at high speed and therefore decision support systems and expert systems are now capable of processing many signals simultaneously in real time. We have developed two fuzzy logic based anaesthesia monitoring systems; a real time smart anaesthesia alarm system (RT-SAAM) and fuzzy logic monitoring system-2 (FLMS-2), an updated version of FLMS for the detection of absolute hypovolaemia. This paper presents the design aspects of these two systems which employ fuzzy logic techniques to detect absolute hypovolaemia, and compares their performances in terms of usability and acceptability. The interpretation of these two systems of absolute hypovolaemia was compared with clinicians' assessments using Kappa analysis, RT-SAAM K=0.62, FLMS-2 K=0.75; an improvement in performance by FLMS-2.
10.1016/j.compbiomed.2013.01.023
[Particle swarm optimization fuzzy modeling and closed-loop anaesthesia control based on cerebral state index].
Tang Jingtian,Cao Yang,Xiao Jiaying,Guo Qulian
Sheng wu yi xue gong cheng xue za zhi = Journal of biomedical engineering = Shengwu yixue gongchengxue zazhi
Due to individual differences of the depth of anaesthesia (DOA) controlled objects, the drawbacks of monitoring index, the traditional PID controller of anesthesia depth could not meet the demands of nonlinear control. However, the adjustments of the rules of DOA fuzzy control often rely on personal experience and, therefore, it could not achieve the satisfactory control effects. The present research established a fuzzy closed-loop control system which takes the cerebral state index (CSI) value as a feedback controlled variable, and it also adopts the particle swarm optimization (PSO) to optimize the fuzzy control rule and membership functions between the change of CSI and propofol infusion rate. The system sets the CSI targets at 40 and 30 through the system simulation, and it also adds some Gaussian noise to imitate clinical disturbance. Experimental results indicated that this system could reach the set CSI point accurately, rapidly and stably, with no obvious perturbation in the presence of noise. The fuzzy controller based on CSI which has been optimized by PSO has better stability and robustness in the DOA closed loop control system.
Relative wave energy-based adaptive neuro-fuzzy inference system for estimation of the depth of anaesthesia.
Benzy V K,Jasmin E A,Koshy Rachel Cherian,Amal Frank,Indiradevi K P
Journal of integrative neuroscience
The advancement in medical research and intelligent modeling techniques has lead to the developments in anaesthesia management. The present study is targeted to estimate the depth of anaesthesia using cognitive signal processing and intelligent modeling techniques. The neurophysiological signal that reflects cognitive state of anaesthetic drugs is the electroencephalogram signal. The information available on electroencephalogram signals during anaesthesia are drawn by extracting relative wave energy features from the anaesthetic electroencephalogram signals. Discrete wavelet transform is used to decomposes the electroencephalogram signals into four levels and then relative wave energy is computed from approximate and detail coefficients of sub-band signals. Relative wave energy is extracted to find out the degree of importance of different electroencephalogram frequency bands associated with different anaesthetic phases awake, induction, maintenance and recovery. The Kruskal-Wallis statistical test is applied on the relative wave energy features to check the discriminating capability of relative wave energy features as awake, light anaesthesia, moderate anaesthesia and deep anaesthesia. A novel depth of anaesthesia index is generated by implementing a Adaptive neuro-fuzzy inference system based fuzzy c-means clustering algorithm which uses relative wave energy features as inputs. Finally, the generated depth of anaesthesia index is compared with a commercially available depth of anaesthesia monitor Bispectral index.
10.31083/JIN-170039
Monitoring the Depth of Anesthesia Using a New Adaptive Neurofuzzy System.
Shalbaf Ahmad,Saffar Mohsen,Sleigh Jamie W,Shalbaf Reza
IEEE journal of biomedical and health informatics
Accurate and noninvasive monitoring of the depth of anesthesia (DoA) is highly desirable. Since the anesthetic drugs act mainly on the central nervous system, the analysis of brain activity using electroencephalogram (EEG) is very useful. This paper proposes a novel automated method for assessing the DoA using EEG. First, 11 features including spectral, fractal, and entropy are extracted from EEG signal and then, by applying an algorithm according to exhaustive search of all subsets of features, a combination of the best features (Beta-index, sample entropy, shannon permutation entropy, and detrended fluctuation analysis) is selected. Accordingly, we feed these extracted features to a new neurofuzzy classification algorithm, adaptive neurofuzzy inference system with linguistic hedges (ANFIS-LH). This structure can successfully model systems with nonlinear relationships between input and output, and also classify overlapped classes accurately. ANFIS-LH, which is based on modified classical fuzzy rules, reduces the effects of the insignificant features in input space, which causes overlapping and modifies the output layer structure. The presented method classifies EEG data into awake, light, general, and deep states during anesthesia with sevoflurane in 17 patients. Its accuracy is 92% compared to a commercial monitoring system (response entropy index) successfully. Moreover, this method reaches the classification accuracy of 93% to categorize EEG signal to awake and general anesthesia states by another database of propofol and volatile anesthesia in 50 patients. To sum up, this method is potentially applicable to a new real-time monitoring system to help the anesthesiologist with continuous assessment of DoA quickly and accurately.
10.1109/JBHI.2017.2709841
The use of multi-criteria decision making models in evaluating anesthesia method options in circumcision surgery.
Hancerliogullari Gulsah,Hancerliogullari Kadir Oymen,Koksalmis Emrah
BMC medical informatics and decision making
BACKGROUND:Determining the most suitable anesthesia method for circumcision surgery plays a fundamental role in pediatric surgery. This study is aimed to present pediatric surgeons' perspective on the relative importance of the criteria for selecting anesthesia method for circumcision surgery by utilizing the multi-criteria decision making methods. METHODS:Fuzzy set theory offers a useful tool for transforming linguistic terms into numerical assessments. Since the evaluation of anesthesia methods requires linguistic terms, we utilize the fuzzy Analytic Hierarchy Process (AHP) and fuzzy Technique for Order Preference by Similarity to Ideal Solution (TOPSIS). Both mathematical decision-making methods are originated from individual judgements for qualitative factors utilizing the pair-wise comparison matrix. Our model uses four main criteria, eight sub-criteria as well as three alternatives. To assess the relative priorities, an online questionnaire was completed by three experts, pediatric surgeons, who had experience with circumcision surgery. RESULTS:Discussion of the results with the experts indicates that time-related factors are the most important criteria, followed by psychology, convenience and duration. Moreover, general anesthesia with penile block for circumcision surgery is the preferred choice of anesthesia compared to general anesthesia without penile block, which has a greater priority compared to local anesthesia under the discussed main-criteria and sub-criteria. CONCLUSIONS:The results presented in this study highlight the need to integrate surgeons' criteria into the decision making process for selecting anesthesia methods. This is the first study in which multi-criteria decision making tools, specifically fuzzy AHP and fuzzy TOPSIS, are used to evaluate anesthesia methods for a pediatric surgical procedure.
10.1186/s12911-017-0409-5
Calculations of consciousness: electroencephalography analyses to determine anesthetic depth.
Eagleman Sarah L,Drover David R
Current opinion in anaesthesiology
PURPOSE OF REVIEW:Electroencephalography (EEG) was introduced into anesthesia practice in the 1990s as a tool to titrate anesthetic depth. However, limitations in current analysis techniques have called into question whether these techniques improve standard of care, or instead call for improved, more ubiquitously applicable measures to assess anesthetic transitions and depth. This review highlights emerging analytical approaches and techniques from neuroscience research that have the potential to better capture anesthetic transitions to provide better measurements of anesthetic depth. RECENT FINDINGS:Since the introduction of electroencephalography, neuroscientists, engineers, mathematicians, and clinicians have all been developing new ways of analyzing continuous electrical signals. Collaborations between these fields have proliferated several analytical techniques that demonstrate how anesthetics affect brain dynamics and conscious transitions. Here, we review techniques in the following categories: network science, integration and information, nonlinear dynamics, and artificial intelligence. SUMMARY:Up-and-coming techniques have the potential to better clinically define and characterize altered consciousness time points. Such new techniques used alongside traditional measures have the potential to improve depth of anesthesia measurements and enhance an understanding of how the brain is affected by anesthetic agents. However, new measures will be needed to be tested for robustness in real-world environments and on diverse experimental protocols.
10.1097/ACO.0000000000000618
Automatic anesthesia depth staging using entropy measures and relative power of electroencephalogram frequency bands.
Jahanseir Mercedeh,Setarehdan Seyed Kamaledin,Momenzadeh Sirous
Australasian physical & engineering sciences in medicine
Many of the surgeries performed under general anesthesia are aided by electroencephalogram (EEG) monitoring. With increased focus on detecting the anesthesia states of patients in the course of surgery, more attention has been paid to analyzing the power spectra and entropy measures of EEG signal during anesthesia. In this paper, by using the relative power of EEG frequency bands and the EEG entropy measures, a new method for detecting the depth of anesthesia states has been presented based on the least squares support vector machine (LS-SVM) classifiers. EEG signals were recorded from 20 patients before, during and after general anesthesia in the operating room at a sampling rate of 200 Hz. Then, 12 features were extracted from each EEG segment, 10 s in length, which are used for anesthesia state monitoring. No significant difference was observed (p > 0.05) between these features and the bispectral index (BIS), which is the commonly used measure of anesthetic effect. The used LS-SVM classifier based method is able to identify the anesthesia states with an accuracy of 80% with reference to the BIS index. Since the underlying equation of the utilized LS-SVM is linear, the computational time of the algorithm is not significant and therefore it can be used for online application in operation rooms.
10.1007/s13246-018-0688-x
Auditory evoked potentials for the assessment of depth of anaesthesia: different configurations of artefact detection algorithms.
Luecke Daniela,Stockmanns Gudrun,Gallinat Michael,Kochs Eberhard F,Schneider Gerhard
Biomedizinische Technik. Biomedical engineering
Monitoring the depth of anaesthesia has become an important research topic in the field of biosignal processing. Auditory evoked potentials (AEPs) have been shown to be a promising tool for this purpose. Signals recorded in the noisy environment of an operating theatre are often contaminated by artefacts. Thus, artefact detection and elimination in the underlying electroencephalogram (EEG) are mandatory before AEP extraction. Determination of a suitable artefact detection configuration based on EEG data from a clinical study is described. Artefact detection algorithms and an AEP extraction procedure encompassing the artefact detection results are presented. Different configurations of artefact detection algorithms are evaluated using an AEP verification procedure and support vector machines to determine a suitable configuration for the assessment of depth of anaesthesia using AEPs.
10.1515/BMT.2007.017
Use of Multiple EEG Features and Artificial Neural Network to Monitor the Depth of Anesthesia.
Gu Yue,Liang Zhenhu,Hagihira Satoshi
Sensors (Basel, Switzerland)
The electroencephalogram (EEG) can reflect brain activity and contains abundant information of different anesthetic states of the brain. It has been widely used for monitoring depth of anesthesia (DoA). In this study, we propose a method that combines multiple EEG-based features with artificial neural network (ANN) to assess the DoA. Multiple EEG-based features can express the states of the brain more comprehensively during anesthesia. First, four parameters including permutation entropy, 95% spectral edge frequency, BetaRatio and SynchFastSlow were extracted from the EEG signal. Then, the four parameters were set as the inputs to an ANN which used bispectral index (BIS) as the reference output. 16 patient datasets during propofol anesthesia were used to evaluate this method. The results indicated that the accuracies of detecting each state were 86.4% (awake), 73.6% (light anesthesia), 84.4% (general anesthesia), and 14% (deep anesthesia). The correlation coefficient between BIS and the index of this method was 0.892 ( p < 0.001 ). The results showed that the proposed method could well distinguish between awake and other anesthesia states. This method is promising and feasible for a monitoring system to assess the DoA.
10.3390/s19112499
Artificial intelligence research in anesthesia and intensive care.
Rennels G D,Miller P L
Journal of clinical monitoring
This article describes several research directions exploring the application of artificial intelligence techniques in anesthesia and intensive care. Artificial intelligence can be loosely defined as the discipline of designing computer systems that exhibit "intelligent" behavior. This article first introduces artificial intelligence and computer science research and discusses why medicine has proved to be a challenging domain for applying artificial intelligence techniques. A discussion of the central research themes that arise in medical artificial intelligence, many of which are common to different projects and to different medical settings, is followed by a description of specific research projects that apply artificial intelligence techniques in anesthesiology, ventilatory management, and cardiovascular management. Finally, further comments are made on the current state of the field.
10.1007/bf01617327
Automated EEG preprocessing during anaesthesia: new aspects using artificial neural networks.
Jeleazcov C,Egner S,Bremer F,Schwilden H
Biomedizinische Technik. Biomedical engineering
The computer-aided detection of artefacts became an essential task with increasing automation of quantitative electroencephalogram (EEG) analysis during anaesthesiological applications. The different algorithms published so far required individual manual adjustment or have been based on limited decision criteria. In this study, we developed an artificial neural networks-(ANN-)aided method for automated detection of artefacts and EEG suppression periods. 72 hr EEG recorded before, during and after anaesthesia with propofol have been evaluated. Selected parameterized patterns of 0.25 s length were used to train the ANN (22 input, 8 hidden and 4 output neurons) with error back propagation. The detection performance of the ANN-aided method was tested with processing epochs between 1 to10 s. Related to examiner EEG evaluation, the average detection performance of the method was 72% sensitivity and 80% specificity for artefacts and 90% sensitivity and 92% specificity for EEG suppression. The improvement in signal-to-noise ratio with automated artefact processing was 1.39 times for the spectral edge frequency 95 (SEF95) and 1.89 times for the approximate entropy (ApEn). We conclude that ANN-aided preprocessing provide an useful tool for automated EEG evaluation in anaesthesiological applications.
10.1515/BMT.2004.025
Critical incident reports concerning anaesthetic equipment: analysis of the UK National Reporting and Learning System (NRLS) data from 2006-2008*.
Cassidy C J,Smith A,Arnot-Smith J
Anaesthesia
Anaesthetic equipment plays a central role in anaesthetic practice but brings the potential for malfunction or misuse. We aimed to explore the national picture by reviewing patient safety incidents relating to anaesthetic equipment from the National Reporting and Learning System for England and Wales between 2006 and 2008. We searched the database using the system's own classification and by scrutinising the free text of relevant incidents. There were 1029 relevant incidents. Of these, 410 (39.8%) concerned patient monitoring, most commonly screen failure during anaesthesia, failure of one modality or failure to transfer data automatically from anaesthetic room to operating theatre. Problems relating to ventilators made up 185 (17.9%) of the reports. Sudden failures during anaesthesia accounted for 142 (13.8%) of these, with a further 10 cases (0.9%) where malfunction caused a sustained or increasing positive pressure in the patient's airway. Leaks made up 99 (9.6%) of incidents and 53 (5.2%) of incidents arose from the use of infusion pumps. Most (89%) of the incidents caused no patient harm; only 30 (2.9%) were judged to have led to moderate or severe harm. Although equipment was often faulty, user error or unfamiliarity also played a part. A large variety of causes led to a relatively small number of clinical scenarios, that anaesthetists should be ready, both individually and organisationally, to manage even when the cause is not apparent. We make recommendations for enhancing patient safety with respect to equipment. You can respond to this article at http://www.anaesthesiacorrespondence.com.
10.1111/j.1365-2044.2011.06826.x
Interval type-2 fuzzy neural network controller for a multivariable anesthesia system based on a hardware-in-the-loop simulation.
El-Nagar Ahmad M,El-Bardini Mohammad
Artificial intelligence in medicine
OBJECTIVE:This manuscript describes the use of a hardware-in-the-loop simulation to simulate the control of a multivariable anesthesia system based on an interval type-2 fuzzy neural network (IT2FNN) controller. METHODS AND MATERIALS:The IT2FNN controller consists of an interval type-2 fuzzy linguistic process as the antecedent part and an interval neural network as the consequent part. It has been proposed that the IT2FNN controller can be used for the control of a multivariable anesthesia system to minimize the effects of surgical stimulation and to overcome the uncertainty problem introduced by the large inter-individual variability of the patient parameters. The parameters of the IT2FNN controller were trained online using a back-propagation algorithm. RESULTS:Three experimental cases are presented. All of the experimental results show good performance for the proposed controller over a wide range of patient parameters. Additionally, the results show better performance than the type-1 fuzzy neural network (T1FNN) controller under the effect of surgical stimulation. The response of the proposed controller has a smaller settling time and a smaller overshoot compared with the T1FNN controller and the adaptive interval type-2 fuzzy logic controller (AIT2FLC). The values of the performance indices for the proposed controller are lower than those obtained for the T1FNN controller and the AIT2FLC. CONCLUSION:The IT2FNN controller is superior to the T1FNN controller for the handling of uncertain information due to the structure of type-2 fuzzy logic systems (FLSs), which are able to model and minimize the numerical and linguistic uncertainties associated with the inputs and outputs of the FLSs.
10.1016/j.artmed.2014.03.002
Design and implementation of a control system reflecting the level of analgesia during general anesthesia.
Janda Matthias,Schubert Agnes,Bajorat Jörn,Hofmockel Rainer,Nöldge-Schomburg Gabriele F E,Lampe Bernhard P,Simanski Olaf
Biomedizinische Technik. Biomedical engineering
INTRODUCTION:Measuring and ensuring an adequate level of analgesia in patients are of increasing interest in the area of automated drug delivery during general anesthesia. Therefore, the aim of this investigation was to develop a control system that may reflect the intraoperative analgesia value. Our hypothesis was that a feedback controller could be applied in clinical practice safely and at an adequate quality of analgesia. The purpose of this study was to evaluate the practical feasibility of such a system in a clinical setting. METHODS:The control system for the level of analgesia described in this paper relies on a parameter combination of heart rate variability (HRV), heart rate (HR), and blood pressure (mean arterial pressure, MAP), which serve as input variables for an expert system. For this fuzzy system, the experience of the participating anesthesiologists was translated into a set of fuzzy rules. In a pilot trial, the control system for automated titration of remifentanil, a short-acting opioid, was tested combined with a closed-loop propofol infusion system for hypnosis. Ten adult patients (4 women, 6 men), aged 22-52 years (median, 45 years; range, 29-49 years), with an American Society of Anesthesiologists physical status class I or II and who were scheduled for elective trauma surgery in a supine position were enrolled in this prospective trial. The precision of the system was calculated using internationally defined performance parameters. RESULTS:There was no human intervention necessary during the computer-controlled administration of propofol and remifentanil, and operating conditions were satisfactory in all patients. All patients assessed the quality of anesthesia as "good" to "very good". Median performance error, median absolute performance error, and wobble for HR and MAP during maintenance of anesthesia were -8.98 (5.32), 10.08 (4.17), and 2.68 (1.29) and -4.51 (12.73), 13.63 (2.27), and 3.90 (2.08) [mean (SD)], respectively. CONCLUSION:The control system, reflecting the level of analgesia during general anesthesia designed and evaluated in this study, allows for a clinically practical, nearly fully automated infusion of an opioid during medium-length surgical procedures with acceptable technical requirements and an adequate precision.
10.1515/bmt-2012-0090
Artificial intelligence applications in the intensive care unit.
Hanson C W,Marshall B E
Critical care medicine
OBJECTIVE:To review the history and current applications of artificial intelligence in the intensive care unit. DATA SOURCES:The MEDLINE database, bibliographies of selected articles, and current texts on the subject. STUDY SELECTION:The studies that were selected for review used artificial intelligence tools for a variety of intensive care applications, including direct patient care and retrospective database analysis. DATA EXTRACTION:All literature relevant to the topic was reviewed. DATA SYNTHESIS:Although some of the earliest artificial intelligence (AI) applications were medically oriented, AI has not been widely accepted in medicine. Despite this, patient demographic, clinical, and billing data are increasingly available in an electronic format and therefore susceptible to analysis by intelligent software. Individual AI tools are specifically suited to different tasks, such as waveform analysis or device control. CONCLUSIONS:The intensive care environment is particularly suited to the implementation of AI tools because of the wealth of available data and the inherent opportunities for increased efficiency in inpatient care. A variety of new AI tools have become available in recent years that can function as intelligent assistants to clinicians, constantly monitoring electronic data streams for important trends, or adjusting the settings of bedside devices. The integration of these tools into the intensive care unit can be expected to reduce costs and improve patient outcomes.
10.1097/00003246-200102000-00038
An Adaptive Monitoring Scheme for Automatic Control of Anaesthesia in dynamic surgical environments based on Bispectral Index and Blood Pressure.
Yu Yu-Ning,Doctor Faiyaz,Fan Shou-Zen,Shieh Jiann-Shing
Journal of medical systems
During surgical procedures, bispectral index (BIS) is a well-known measure used to determine the patient's depth of anesthesia (DOA). However, BIS readings can be subject to interference from many factors during surgery, and other parameters such as blood pressure (BP) and heart rate (HR) can provide more stable indicators. However, anesthesiologist still consider BIS as a primary measure to determine if the patient is correctly anaesthetized while relaying on the other physiological parameters to monitor and ensure the patient's status is maintained. The automatic control of administering anesthesia using intelligent control systems has been the subject of recent research in order to alleviate the burden on the anesthetist to manually adjust drug dosage in response physiological changes for sustaining DOA. A system proposed for the automatic control of anesthesia based on type-2 Self Organizing Fuzzy Logic Controllers (T2-SOFLCs) has been shown to be effective in the control of DOA under simulated scenarios while contending with uncertainties due to signal noise and dynamic changes in pharmacodynamics (PD) and pharmacokinetic (PK) effects of the drug on the body. This study considers both BIS and BP as part of an adaptive automatic control scheme, which can adjust to the monitoring of either parameter in response to changes in the availability and reliability of BIS signals during surgery. The simulation of different control schemes using BIS data obtained during real surgical procedures to emulate noise and interference factors have been conducted. The use of either or both combined parameters for controlling the delivery Propofol to maintain safe target set points for DOA are evaluated. The results show that combing BIS and BP based on the proposed adaptive control scheme can ensure the target set points and the correct amount of drug in the body is maintained even with the intermittent loss of BIS signal that could otherwise disrupt an automated control system.
10.1007/s10916-018-0933-6
Performance analysis of extracted rule-base multivariable type-2 self-organizing fuzzy logic controller applied to anesthesia.
BioMed research international
We compare type-1 and type-2 self-organizing fuzzy logic controller (SOFLC) using expert initialized and pretrained extracted rule-bases applied to automatic control of anaesthesia during surgery. We perform experimental simulations using a nonfixed patient model and signal noise to account for environmental and patient drug interaction uncertainties. The simulations evaluate the performance of the SOFLCs in their ability to control anesthetic delivery rates for maintaining desired physiological set points for muscle relaxation and blood pressure during a multistage surgical procedure. The performances of the SOFLCs are evaluated by measuring the steady state errors and control stabilities which indicate the accuracy and precision of control task. Two sets of comparisons based on using expert derived and extracted rule-bases are implemented as Wilcoxon signed-rank tests. Results indicate that type-2 SOFLCs outperform type-1 SOFLC while handling the various sources of uncertainties. SOFLCs using the extracted rules are also shown to outperform those using expert derived rules in terms of improved control stability.
10.1155/2014/379090
Closed-loop anesthesia.
LE Guen Morgan,Liu Ngai,Chazot Thierry,Fischler Marc
Minerva anestesiologica
Automated anesthesia which may offer to the physician time to control hemodynamic and to supervise neurological outcome and which may offer to the patient safety and quality was until recently consider as a holy grail. But this field of research is now increasing in every component of general anesthesia (hypnosis, nociception, neuromuscular blockade) and literature describes some successful algorithms - single or multi closed-loop controller. The aim of these devices is to control a predefined target and to continuously titrate anesthetics whatever the patients' co morbidities and surgical events to reach this target. Literature contains many randomized trials comparing manual and automated anesthesia and shows feasibility and safety of this system. Automation could quickly concern other aspects of anesthesia as fluid management and this review proposes an overview of closed-loop systems in anesthesia.
Adaptive fuzzy modeling of the hypnotic process in anesthesia.
Marrero A,Méndez J A,Reboso J A,Martín I,Calvo J L
Journal of clinical monitoring and computing
This paper addresses the problem of patient model synthesis in anesthesia. Recent advanced drug infusion mechanisms use a patient model to establish the proper drug dose. However, due to the inherent complexity and variability of the patient dynamics, difficulty obtaining a good model is high. In this paper, a method based on fuzzy logic and genetic algorithms is proposed as an alternative to standard compartmental models. The model uses a Mamdani type fuzzy inference system developed in a two-step procedure. First, an offline model is obtained using information from real patients. Then, an adaptive strategy that uses genetic algorithms is implemented. The validation of the modeling technique was done using real data obtained from real patients in the operating room. Results show that the proposed method based on artificial intelligence appears to be an improved alternative to existing compartmental methodologies.
10.1007/s10877-016-9868-y
Augmented intelligence in pediatric anesthesia and pediatric critical care.
Görges Matthias,Ansermino J Mark
Current opinion in anaesthesiology
PURPOSE OF REVIEW:Acute care technologies, including novel monitoring devices, big data, increased computing capabilities, machine-learning algorithms and automation, are converging. This enables the application of augmented intelligence for improved outcome predictions, clinical decision-making, and offers unprecedented opportunities to improve patient outcomes, reduce costs, and improve clinician workflow. This article briefly explores recent work in the areas of automation, artificial intelligence and outcome prediction models in pediatric anesthesia and pediatric critical care. RECENT FINDINGS:Recent years have yielded little published research into pediatric physiological closed loop control (a type of automation) beyond studies focused on glycemic control for type 1 diabetes. However, there has been a greater range of research in augmented decision-making, leveraging artificial intelligence and machine-learning techniques, in particular, for pediatric ICU outcome prediction. SUMMARY:Most studies focusing on artificial intelligence demonstrate good performance on prediction or classification, whether they use traditional statistical tools or novel machine-learning approaches. Yet the challenges of implementation, user acceptance, ethics and regulation cannot be underestimated. Areas in which there is easy access to routinely labeled data and robust outcomes, such as those collected through national networks and quality improvement programs, are likely to be at the forefront of the adoption of these advances.
10.1097/ACO.0000000000000845
Artificial Intelligence Technologies for Coping with Alarm Fatigue in Hospital Environments Because of Sensory Overload: Algorithm Development and Validation.
Fernandes Chrystinne Oliveira,Miles Simon,Lucena Carlos José Pereira De,Cowan Donald
Journal of medical Internet research
BACKGROUND:Informed estimates claim that 80% to 99% of alarms set off in hospital units are false or clinically insignificant, representing a cacophony of sounds that do not present a real danger to patients. These false alarms can lead to an alert overload that causes a health care provider to miss important events that could be harmful or even life-threatening. As health care units become more dependent on monitoring devices for patient care purposes, the alarm fatigue issue has to be addressed as a major concern for the health care team as well as to enhance patient safety. OBJECTIVE:The main goal of this paper was to propose a feasible solution for the alarm fatigue problem by using an automatic reasoning mechanism to decide how to notify members of the health care team. The aim was to reduce the number of notifications sent by determining whether or not to group a set of alarms that occur over a short period of time to deliver them together, without compromising patient safety. METHODS:This paper describes: (1) a model for supporting reasoning algorithms that decide how to notify caregivers to avoid alarm fatigue; (2) an architecture for health systems that support patient monitoring and notification capabilities; and (3) a reasoning algorithm that specifies how to notify caregivers by deciding whether to aggregate a group of alarms to avoid alarm fatigue. RESULTS:Experiments were used to demonstrate that providing a reasoning system can reduce the notifications received by the caregivers by up to 99.3% (582/586) of the total alarms generated. Our experiments were evaluated through the use of a dataset comprising patient monitoring data and vital signs recorded during 32 surgical cases where patients underwent anesthesia at the Royal Adelaide Hospital. We present the results of our algorithm by using graphs we generated using the R language, where we show whether the algorithm decided to deliver an alarm immediately or after a delay. CONCLUSIONS:The experimental results strongly suggest that this reasoning algorithm is a useful strategy for avoiding alarm fatigue. Although we evaluated our algorithm in an experimental environment, we tried to reproduce the context of a clinical environment by using real-world patient data. Our future work is to reproduce the evaluation study based on more realistic clinical conditions by increasing the number of patients, monitoring parameters, and types of alarm.
10.2196/15406
Anesthesiology, automation, and artificial intelligence.
Proceedings (Baylor University. Medical Center)
There have been many attempts to incorporate automation into the practice of anesthesiology, though none have been successful. Fundamentally, these failures are due to the underlying complexity of anesthesia practice and the inability of rule-based feedback loops to fully master it. Recent innovations in artificial intelligence, especially machine learning, may usher in a new era of automation across many industries, including anesthesiology. It would be wise to consider the implications of such potential changes before they have been fully realized.
10.1080/08998280.2017.1391036
Artificial intelligence in mitral valve analysis.
Jeganathan Jelliffe,Knio Ziyad,Amador Yannis,Hai Ting,Khamooshian Arash,Matyal Robina,Khabbaz Kamal R,Mahmood Feroze
Annals of cardiac anaesthesia
BACKGROUND:Echocardiographic analysis of mitral valve (MV) has become essential for diagnosis and management of patients with MV disease. Currently, the various software used for MV analysis require manual input and are prone to interobserver variability in the measurements. AIM:The aim of this study is to determine the interobserver variability in an automated software that uses artificial intelligence for MV analysis. SETTINGS AND DESIGN:Retrospective analysis of intraoperative three-dimensional transesophageal echocardiography data acquired from four patients with normal MV undergoing coronary artery bypass graft surgery in a tertiary hospital. MATERIALS AND METHODS:Echocardiographic data were analyzed using the eSie Valve Software (Siemens Healthcare, Mountain View, CA, USA). Three examiners analyzed three end-systolic (ES) frames from each of the four patients. A total of 36 ES frames were analyzed and included in the study. STATISTICAL ANALYSIS:A multiple mixed-effects ANOVA model was constructed to determine if the examiner, the patient, and the loop had a significant effect on the average value of each parameter. A Bonferroni correction was used to correct for multiple comparisons, and P = 0.0083 was considered to be significant. RESULTS:Examiners did not have an effect on any of the six parameters tested. Patient and loop had an effect on the average parameter value for each of the six parameters as expected (P < 0.0083 for both). CONCLUSION:We were able to conclude that using automated analysis, it is possible to obtain results with good reproducibility, which only requires minimal user intervention.
10.4103/aca.ACA_243_16
Artificial Intelligence: A New Tool in Operating Room Management. Role of Machine Learning Models in Operating Room Optimization.
Bellini Valentina,Guzzon Marco,Bigliardi Barbara,Mordonini Monica,Filippelli Serena,Bignami Elena
Journal of medical systems
We conducted a systematic review of literature to better understand the role of new technologies in the perioperative period; in particular we focus on the administrative and managerial Operating Room (OR) perspective. Studies conducted on adult (≥ 18 years) patients between 2015 and February 2019 were deemed eligible. A total of 19 papers were included. Our review suggests that the use of Machine Learning (ML) in the field of OR organization has many potentials. Predictions of the surgical case duration were obtain with a good performance; their use could therefore allow a more precise scheduling, limiting waste of resources. ML is able to support even more complex models, which can coordinate multiple spaces simultaneously, as in the case of the post-anesthesia care unit and operating rooms. Types of Artificial Intelligence could also be used to limit another organizational problem, which has important economic repercussions: cancellation. Random Forest has proven effective in identifing surgeries with high risks of cancellation, allowing to plan preventive measures to reduce the cancellation rate accordingly. In conclusion, although data in literature are still limited, we believe that ML has great potential in the field of OR organization; however, further studies are needed to assess the effective role of these new technologies in the perioperative medicine.
10.1007/s10916-019-1512-1
Artificial intelligence: a key to relieve China's insufficient and unequally-distributed medical resources.
Kong Xiangyi,Ai Bolun,Kong Yiming,Su Lijuan,Ning Yunzhou,Howard Newton,Gong Shun,Li Chen,Wang Jie,Lee Wan-Ting,Wang Jing,Kong Yanguo,Wang Jingping,Fang Yi
American journal of translational research
In this manuscript, we firstly reviewed the challenges faced by China in its health care reform. Though Chinese governments have made tremendous efforts, problems like the difficulties and high expense in medical care and the nervous doctor-patient relationship have been reported a lot, whose key problem is the insufficiency of high-quality medical resource and the supply-demand imbalance. Presently, it's almost old news: artificial intelligence will overturn the existing medical model. Artificial intelligence technology will transform the medical sector and trigger an estimated $147 billion market during the next 20 years. We hereby pointed out the strengths of medical artificial intelligence and its potentials to relieve China's insufficient and unequally-distributed medical resources. Also, we analyzed China's advantages in developing medical AI due to its huge medical big data and China government's powerful promotion policy. Finally, we put forward some challenges for China to practice this.
Artificial intelligence, machine learning and the pediatric airway.
Matava Clyde,Pankiv Evelina,Ahumada Luis,Weingarten Benjamin,Simpao Allan
Paediatric anaesthesia
Artificial intelligence and machine learning are rapidly expanding fields with increasing relevance in anesthesia and, in particular, airway management. The ability of artificial intelligence and machine learning algorithms to recognize patterns from large volumes of complex data makes them attractive for use in pediatric anesthesia airway management. The purpose of this review is to introduce artificial intelligence, machine learning, and deep learning to the pediatric anesthesiologist. Current evidence and developments in artificial intelligence, machine learning, and deep learning relevant to pediatric airway management are presented. We critically assess the current evidence on the use of artificial intelligence and machine learning in the assessment, diagnosis, monitoring, procedure assistance, and predicting outcomes during pediatric airway management. Further, we discuss the limitations of these technologies and offer areas for focused research that may bring pediatric airway management anesthesiology into the era of artificial intelligence and machine learning.
10.1111/pan.13792
Artificial Intelligence in Anesthesiology: Current Techniques, Clinical Applications, and Limitations.
Hashimoto Daniel A,Witkowski Elan,Gao Lei,Meireles Ozanan,Rosman Guy
Anesthesiology
Artificial intelligence has been advancing in fields including anesthesiology. This scoping review of the intersection of artificial intelligence and anesthesia research identified and summarized six themes of applications of artificial intelligence in anesthesiology: (1) depth of anesthesia monitoring, (2) control of anesthesia, (3) event and risk prediction, (4) ultrasound guidance, (5) pain management, and (6) operating room logistics. Based on papers identified in the review, several topics within artificial intelligence were described and summarized: (1) machine learning (including supervised, unsupervised, and reinforcement learning), (2) techniques in artificial intelligence (e.g., classical machine learning, neural networks and deep learning, Bayesian methods), and (3) major applied fields in artificial intelligence.The implications of artificial intelligence for the practicing anesthesiologist are discussed as are its limitations and the role of clinicians in further developing artificial intelligence for use in clinical care. Artificial intelligence has the potential to impact the practice of anesthesiology in aspects ranging from perioperative support to critical care delivery to outpatient pain management.
10.1097/ALN.0000000000002960