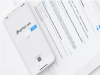
Meta-analysis of Calibration, Discrimination, and Stratum-Specific Likelihood Ratios for the CRB-65 Score.
Ebell Mark H,Walsh Mary E,Fahey Tom,Kearney Maggie,Marchello Christian
Journal of general internal medicine
BACKGROUND:The CRB-65 score is recommended as a decision support tool to help identify patients with community-acquired pneumonia (CAP) who can safely be treated as outpatients. OBJECTIVE:To perform an updated meta-analysis of the accuracy, discrimination, and calibration of the CRB-65 score using a novel approach to calculation of stratum-specific likelihood ratios. DESIGN:Meta-analysis of accuracy, discrimination, and calibration. METHODS:We searched PubMed, Google, previous systematic reviews, and reference lists of included studies. Data was abstracted and quality assessed in parallel by two investigators. The quality assessment used an adaptation of the TRIPOD and PROBAST criteria. Measures of discrimination, calibration, and stratum-specific likelihood ratios are reported. KEY RESULTS:Twenty-nine studies met our inclusion criteria and provided usable data. Most studies were set in Europe, none in North America, and 12 were judged to be at low risk of bias. The pooled estimate of area under the receiver operating characteristic curve was 0.74 (95% CI 0.71-0.77) for all studies. Calibration was good although there was significant heterogeneity; the pooled estimate of the ratio of observed to expected mortality for all studies was 1.04 (95% CI 0.91-1.19). The corresponding values for studies at low risk of bias where patients could be treated as outpatients or inpatients were 0.76 (0.70-0.81) and 0.88 (0.69-1.13). Summary estimates of stratum-specific likelihood ratios for all studies were 0.19 for the low-risk group, 1.1 for the moderate-risk group, and 4.5 for the high-risk group, and 0.13, 1.3, and 5.6 for studies at low risk of bias where patients could be treated as outpatients or inpatients. CONCLUSIONS:The CRB-65 is useful for identifying low-risk patients for outpatient therapy. Given a 4% overall mortality risk, patients classified as low risk by the CRB-65 had an outpatient mortality risk of no more than 0.5%.
10.1007/s11606-019-04869-z
Prediction models for diagnosis and prognosis of covid-19: systematic review and critical appraisal
BMJ (Clinical research ed.)
OBJECTIVE:To review and appraise the validity and usefulness of published and preprint reports of prediction models for diagnosing coronavirus disease 2019 (covid-19) in patients with suspected infection, for prognosis of patients with covid-19, and for detecting people in the general population at increased risk of covid-19 infection or being admitted to hospital with the disease. DESIGN:Living systematic review and critical appraisal by the COVID-PRECISE (Precise Risk Estimation to optimise covid-19 Care for Infected or Suspected patients in diverse sEttings) group. DATA SOURCES:PubMed and Embase through Ovid, up to 1 July 2020, supplemented with arXiv, medRxiv, and bioRxiv up to 5 May 2020. STUDY SELECTION:Studies that developed or validated a multivariable covid-19 related prediction model. DATA EXTRACTION:At least two authors independently extracted data using the CHARMS (critical appraisal and data extraction for systematic reviews of prediction modelling studies) checklist; risk of bias was assessed using PROBAST (prediction model risk of bias assessment tool). RESULTS:37 421 titles were screened, and 169 studies describing 232 prediction models were included. The review identified seven models for identifying people at risk in the general population; 118 diagnostic models for detecting covid-19 (75 were based on medical imaging, 10 to diagnose disease severity); and 107 prognostic models for predicting mortality risk, progression to severe disease, intensive care unit admission, ventilation, intubation, or length of hospital stay. The most frequent types of predictors included in the covid-19 prediction models are vital signs, age, comorbidities, and image features. Flu-like symptoms are frequently predictive in diagnostic models, while sex, C reactive protein, and lymphocyte counts are frequent prognostic factors. Reported C index estimates from the strongest form of validation available per model ranged from 0.71 to 0.99 in prediction models for the general population, from 0.65 to more than 0.99 in diagnostic models, and from 0.54 to 0.99 in prognostic models. All models were rated at high or unclear risk of bias, mostly because of non-representative selection of control patients, exclusion of patients who had not experienced the event of interest by the end of the study, high risk of model overfitting, and unclear reporting. Many models did not include a description of the target population (n=27, 12%) or care setting (n=75, 32%), and only 11 (5%) were externally validated by a calibration plot. The Jehi diagnostic model and the 4C mortality score were identified as promising models. CONCLUSION:Prediction models for covid-19 are quickly entering the academic literature to support medical decision making at a time when they are urgently needed. This review indicates that almost all pubished prediction models are poorly reported, and at high risk of bias such that their reported predictive performance is probably optimistic. However, we have identified two (one diagnostic and one prognostic) promising models that should soon be validated in multiple cohorts, preferably through collaborative efforts and data sharing to also allow an investigation of the stability and heterogeneity in their performance across populations and settings. Details on all reviewed models are publicly available at https://www.covprecise.org/. Methodological guidance as provided in this paper should be followed because unreliable predictions could cause more harm than benefit in guiding clinical decisions. Finally, prediction model authors should adhere to the TRIPOD (transparent reporting of a multivariable prediction model for individual prognosis or diagnosis) reporting guideline. SYSTEMATIC REVIEW REGISTRATION:Protocol https://osf.io/ehc47/, registration https://osf.io/wy245. READERS' NOTE:This article is a living systematic review that will be updated to reflect emerging evidence. Updates may occur for up to two years from the date of original publication. This version is update 3 of the original article published on 7 April 2020 (BMJ 2020;369:m1328). Previous updates can be found as data supplements (https://www.bmj.com/content/369/bmj.m1328/related#datasupp). When citing this paper please consider adding the update number and date of access for clarity.
10.1136/bmj.m1328
FINDRISC in Latin America: a systematic review of diagnosis and prognosis models.
BMJ open diabetes research & care
This review aimed to assess whether the FINDRISC, a risk score for type 2 diabetes mellitus (T2DM), has been externally validated in Latin America and the Caribbean (LAC). We conducted a systematic review following the CHARMS (CHecklist for critical Appraisal and data extraction for systematic Reviews of prediction Modelling Studies) framework. Reports were included if they validated or re-estimated the FINDRISC in population-based samples, health facilities or administrative data. Reports were excluded if they only studied patients or at-risk individuals. The search was conducted in Medline, Embase, Global Health, Scopus and LILACS. Risk of bias was assessed with the PROBAST (Prediction model Risk of Bias ASsessment Tool) tool. From 1582 titles and abstracts, 4 (n=7502) reports were included for qualitative summary. All reports were from South America; there were slightly more women, and the mean age ranged from 29.5 to 49.7 years. Undiagnosed T2DM prevalence ranged from 2.6% to 5.1%. None of the studies conducted an independent external validation of the FINDRISC; conversely, they used the same (or very similar) predictors to fit a new model. None of the studies reported calibration metrics. The area under the receiver operating curve was consistently above 65.0%. All studies had high risk of bias. There has not been any external validation of the FINDRISC model in LAC. Selected reports re-estimated the FINDRISC, although they have several methodological limitations. There is a need for big data to develop-or improve-T2DM diagnostic and prognostic models in LAC. This could benefit T2DM screening and early diagnosis.
10.1136/bmjdrc-2019-001169
Prognostic models for predicting incident or recurrent atrial fibrillation: protocol for a systematic review.
Systematic reviews
BACKGROUND:Atrial fibrillation (AF) is the arrhythmia most commonly diagnosed in clinical practice. It is associated with significant morbidity and mortality. Prevalence of AF and complications of AF, estimated by hospitalisations, have increased dramatically in the last decade. Being able to predict AF would allow tailoring of management strategies and a focus on primary or secondary prevention. Models predicting recurrent AF would have particular clinical use for the selection of rhythm control therapy. There are existing prognostic models which combine several predictors or risk factors to generate an individualised estimate of risk of AF. The aim of this systematic review is to summarise and compare model performance measures and predictive accuracy across different models and populations at risk of developing incident or recurrent AF. METHODS:Methods tailored to systematic reviews of prognostic models will be used for study identification, risk of bias assessment and synthesis. Studies will be eligible for inclusion where they report an internally or externally validated model. The quality of studies reporting a prognostic model will be assessed using the Prediction Study Risk Of Bias Assessment Tool (PROBAST). Studies will be narratively described and included variables and predictive accuracy compared across different models and populations. Meta-analysis of model performance measures for models validated in similar populations will be considered where possible. DISCUSSION:To the best of our knowledge, this will be the first systematic review to collate evidence from all studies reporting on validated prognostic models, or on the impact of such models, in any population at risk of incident or recurrent AF. The review may identify models which are suitable for impact assessment in clinical practice. Should gaps in the evidence be identified, research recommendations relating to model development, validation or impact assessment will be made. Findings will be considered in the context of any models already used in clinical practice, and the extent to which these have been validated. SYSTEMATIC REVIEW REGISTRATION:PROSPERO ( CRD42018111649 ).
10.1186/s13643-019-1128-z
Prognostic models for outcome prediction in patients with chronic obstructive pulmonary disease: systematic review and critical appraisal.
Bellou Vanesa,Belbasis Lazaros,Konstantinidis Athanasios K,Tzoulaki Ioanna,Evangelou Evangelos
BMJ (Clinical research ed.)
OBJECTIVE:To map and assess prognostic models for outcome prediction in patients with chronic obstructive pulmonary disease (COPD). DESIGN:Systematic review. DATA SOURCES:PubMed until November 2018 and hand searched references from eligible articles. ELIGIBILITY CRITERIA FOR STUDY SELECTION:Studies developing, validating, or updating a prediction model in COPD patients and focusing on any potential clinical outcome. RESULTS:The systematic search yielded 228 eligible articles, describing the development of 408 prognostic models, the external validation of 38 models, and the validation of 20 prognostic models derived for diseases other than COPD. The 408 prognostic models were developed in three clinical settings: outpatients (n=239; 59%), patients admitted to hospital (n=155; 38%), and patients attending the emergency department (n=14; 3%). Among the 408 prognostic models, the most prevalent endpoints were mortality (n=209; 51%), risk for acute exacerbation of COPD (n=42; 10%), and risk for readmission after the index hospital admission (n=36; 9%). Overall, the most commonly used predictors were age (n=166; 41%), forced expiratory volume in one second (n=85; 21%), sex (n=74; 18%), body mass index (n=66; 16%), and smoking (n=65; 16%). Of the 408 prognostic models, 100 (25%) were internally validated and 91 (23%) examined the calibration of the developed model. For 286 (70%) models a model presentation was not available, and only 56 (14%) models were presented through the full equation. Model discrimination using the C statistic was available for 311 (76%) models. 38 models were externally validated, but in only 12 of these was the validation performed by a fully independent team. Only seven prognostic models with an overall low risk of bias according to PROBAST were identified. These models were ADO, B-AE-D, B-AE-D-C, extended ADO, updated ADO, updated BODE, and a model developed by Bertens et al. A meta-analysis of C statistics was performed for 12 prognostic models, and the summary estimates ranged from 0.611 to 0.769. CONCLUSIONS:This study constitutes a detailed mapping and assessment of the prognostic models for outcome prediction in COPD patients. The findings indicate several methodological pitfalls in their development and a low rate of external validation. Future research should focus on the improvement of existing models through update and external validation, as well as the assessment of the safety, clinical effectiveness, and cost effectiveness of the application of these prognostic models in clinical practice through impact studies. SYSTEMATIC REVIEW REGISTRATION:PROSPERO CRD42017069247.
10.1136/bmj.l5358
Risk Prediction Models for Kidney Cancer: A Systematic Review.
European urology focus
CONTEXT:Early detection of kidney cancer improves survival; however, low prevalence means that population-wide screening may be inefficient. Stratification of the population into risk categories could allow for the introduction of a screening programme tailored to individuals. OBJECTIVE:This review will identify and compare published models that predict the risk of developing kidney cancer in the general population. EVIDENCE ACQUISITION:A search identified primary research reporting or validating models predicting the risk of kidney cancer in Medline and EMBASE. After screening identified studies for inclusion, we extracted data onto a standardised form. The risk models were classified using the Transparent Reporting of a multivariable prediction model for Individual Prognosis Or Diagnosis (TRIPOD) guidelines and evaluated using the PROBAST assessment tool. EVIDENCE SYNTHESIS:The search identified 15 281 articles. Sixty-two satisfied the inclusion criteria; performance measures were provided for 11 models. Some models predicted the risk of prevalent undiagnosed disease and others future incident disease. Six of the models had been validated, two using external populations. The most commonly included risk factors were age, smoking status, and body mass index. Most of the models had acceptable-to-good discrimination (area under the receiver-operating curve >0.7) in development and validation. Many models also had high specificity; however, several had low sensitivity. The highest performance was seen for the models using only biomarkers to detect kidney cancer; however, these were developed and validated in small case-control studies. CONCLUSIONS:We identified a small number of risk models that could be used to stratify the population according to the risk of kidney cancer. Most exhibit reasonable discrimination, but a few have been validated externally in population-based studies. PATIENT SUMMARY:In this review, we looked at mathematical models predicting the likelihood of an individual developing kidney cancer. We found several suitable models, using a range of risk factors (such as age and smoking) to predict the risk for individuals. Most of the models identified require further testing in the general population to confirm their usefulness.
10.1016/j.euf.2020.06.024
Artificial intelligence versus clinicians: systematic review of design, reporting standards, and claims of deep learning studies.
BMJ (Clinical research ed.)
OBJECTIVE:To systematically examine the design, reporting standards, risk of bias, and claims of studies comparing the performance of diagnostic deep learning algorithms for medical imaging with that of expert clinicians. DESIGN:Systematic review. DATA SOURCES:Medline, Embase, Cochrane Central Register of Controlled Trials, and the World Health Organization trial registry from 2010 to June 2019. ELIGIBILITY CRITERIA FOR SELECTING STUDIES:Randomised trial registrations and non-randomised studies comparing the performance of a deep learning algorithm in medical imaging with a contemporary group of one or more expert clinicians. Medical imaging has seen a growing interest in deep learning research. The main distinguishing feature of convolutional neural networks (CNNs) in deep learning is that when CNNs are fed with raw data, they develop their own representations needed for pattern recognition. The algorithm learns for itself the features of an image that are important for classification rather than being told by humans which features to use. The selected studies aimed to use medical imaging for predicting absolute risk of existing disease or classification into diagnostic groups (eg, disease or non-disease). For example, raw chest radiographs tagged with a label such as pneumothorax or no pneumothorax and the CNN learning which pixel patterns suggest pneumothorax. REVIEW METHODS:Adherence to reporting standards was assessed by using CONSORT (consolidated standards of reporting trials) for randomised studies and TRIPOD (transparent reporting of a multivariable prediction model for individual prognosis or diagnosis) for non-randomised studies. Risk of bias was assessed by using the Cochrane risk of bias tool for randomised studies and PROBAST (prediction model risk of bias assessment tool) for non-randomised studies. RESULTS:Only 10 records were found for deep learning randomised clinical trials, two of which have been published (with low risk of bias, except for lack of blinding, and high adherence to reporting standards) and eight are ongoing. Of 81 non-randomised clinical trials identified, only nine were prospective and just six were tested in a real world clinical setting. The median number of experts in the comparator group was only four (interquartile range 2-9). Full access to all datasets and code was severely limited (unavailable in 95% and 93% of studies, respectively). The overall risk of bias was high in 58 of 81 studies and adherence to reporting standards was suboptimal (<50% adherence for 12 of 29 TRIPOD items). 61 of 81 studies stated in their abstract that performance of artificial intelligence was at least comparable to (or better than) that of clinicians. Only 31 of 81 studies (38%) stated that further prospective studies or trials were required. CONCLUSIONS:Few prospective deep learning studies and randomised trials exist in medical imaging. Most non-randomised trials are not prospective, are at high risk of bias, and deviate from existing reporting standards. Data and code availability are lacking in most studies, and human comparator groups are often small. Future studies should diminish risk of bias, enhance real world clinical relevance, improve reporting and transparency, and appropriately temper conclusions. STUDY REGISTRATION:PROSPERO CRD42019123605.
10.1136/bmj.m689
Gene Expression Profiling Tests for Early-Stage Invasive Breast Cancer: A Health Technology Assessment.
Ontario health technology assessment series
BACKGROUND:Breast cancer is a disease in which cells in the breast grow out of control. They often form a tumour that may be seen on an x-ray or felt as a lump.Gene expression profiling (GEP) tests are intended to help predict the risk of metastasis (spread of the cancer to other parts of the body) and to identify people who will most likely benefit from chemotherapy. We conducted a health technology assessment of four GEP tests (EndoPredict, MammaPrint, Oncotype DX, and Prosigna) for people with early-stage invasive breast cancer, which included an evaluation of effectiveness, safety, cost effectiveness, the budget impact of publicly funding GEP tests, and patient preferences and values. METHODS:We performed a systematic literature search of the clinical evidence. We assessed the risk of bias of each included study using either the Cochrane Risk of Bias tool, Prediction model Risk Of Bias ASsessment Tool (PROBAST), or Risk of Bias Assessment tool for Non-randomized Studies (RoBANS), depending on the type of study and outcome of interest, and the quality of the body of evidence according to the Grading of Recommendations Assessment, Development, and Evaluation (GRADE) Working Group criteria. We also performed a literature survey of the quantitative evidence of preferences and values of patients and providers for GEP tests.We performed an economic evidence review to identify published studies assessing the cost-effectiveness of each of the four GEP tests compared with usual care or with one another for people with early-stage invasive breast cancer. We adapted a decision-analytic model to compare the costs and outcomes of care that includes a GEP test with usual care without a GEP test over a lifetime horizon. We also estimated the budget impact of publicly funding GEP tests to be conducted in Ontario, compared with funding tests conducted through the out-of-country program and compared with no funding of tests in any location.To contextualize the potential value of GEP tests, we spoke with people who have been diagnosed with early-stage invasive breast cancer. RESULTS:We included 68 studies in the clinical evidence review. Within the lymph-node-negative (LN-) population, GEP tests can prognosticate the risk of distant recurrence (GRADE: Moderate) and may predict chemotherapy benefit (GRADE: Low). The evidence for prognostic and predictive ability (ability to indicate the risk of an outcome and ability to predict who will benefit from chemotherapy, respectively) was lower for the lymph-node-positive (LN+) population (GRADE: Very Low to Low). GEP tests may also lead to changes in treatment (GRADE: Low) and generally may increase physician confidence in treatment recommendations (GRADE: Low).Our economic evidence review showed that GEP tests are generally cost-effective compared with usual care.Our primary economic evaluation showed that all GEP test strategies were more effective (led to more quality-adjusted life-years [QALYs]) than usual care and can be considered cost-effective below a willingness-to-pay of $20,000 per QALY gained. There was some uncertainty in our results. At a willingness-to-pay of $50,000 per QALY gained, the probability of each test being cost-effective compared to usual care was 63.0%, 89.2%, 89.2%, and 100% for EndoPredict, MammaPrint, Oncotype DX, and Prosigna, respectively.Sensitivity analyses showed our results were robust to variation in subgroups considered (i.e., LN+ and premenopausal), discount rates, age, and utilities. However, cost parameter assumptions did influence our results. Our scenario analysis comparing tests showed Oncotype DX was likely cost-effective compared with MammaPrint, and Prosigna was likely cost-effective compared with EndoPredict. When the GEP tests were compared with a clinical tool, the cost-effectiveness of the tests varied. Assuming a higher uptake of GEP tests, we estimated the budget impact to publicly fund GEP tests in Ontario would be between $1.29 million (Year 1) and $2.22 million (Year 5) compared to the current scenario of publicly funded GEP tests through the out-of-country program.Gene expression profiling tests are valued by patients and physicians for the additional information they provide for treatment decision-making. Patients are satisfied with what they learn from GEP tests and feel GEP tests can help reduce decisional uncertainty and anxiety. CONCLUSIONS:Gene expression profiling tests can likely prognosticate the risk of distant recurrence and some tests may also predict chemotherapy benefit. In people with breast cancer that is ER+, LN-, and human epidermal growth factor receptor 2 (HER2)-negative, GEP tests are likely cost-effective compared with no testing. The GEP tests are also likely cost-effective in LN+ and premenopausal people. Compared with funding GEP tests through the out-of-country program, publicly funding GEP tests in Ontario would cost an additional $1 million to $2 million annually, assuming a higher uptake of tests. GEP tests are valued by both patients and physicians for chemotherapy treatment decision-making.
A systematic review of methodology used in the development of prediction models for future asthma exacerbation.
Bridge Joshua,Blakey John D,Bonnett Laura J
BMC medical research methodology
BACKGROUND:Clinical prediction models are widely used to guide medical advice and therapeutic interventions. Asthma is one of the most common chronic diseases globally and is characterised by acute deteriorations. These exacerbations are largely preventable, so there is interest in using clinical prediction models in this area. The objective of this review was to identify studies which have developed such models, determine whether consistent and appropriate methodology was used and whether statistically reliable prognostic models exist. METHODS:We searched online databases MEDLINE (1948 onwards), CINAHL Plus (1937 onwards), The Cochrane Library, Web of Science (1898 onwards) and ClinicalTrials.gov, using index terms relating to asthma and prognosis. Data was extracted and assessment of quality was based on GRADE and an early version of PROBAST (Prediction study Risk of Bias Assessment Tool). A meta-analysis of the discrimination and calibration measures was carried out to determine overall performance across models. RESULTS:Ten unique prognostic models were identified. GRADE identified moderate risk of bias in two of the studies, but more detailed quality assessment via PROBAST highlighted that most models were developed using highly selected and small datasets, incompletely recorded predictors and outcomes, and incomplete methodology. None of the identified models modelled recurrent exacerbations, instead favouring either presence/absence of an event, or time to first or specified event. Preferred methodologies were logistic regression and Cox proportional hazards regression. The overall pooled c-statistic was 0.77 (95% confidence interval 0.73 to 0.80), though individually some models performed no better than chance. The meta-analysis had an I value of 99.75% indicating a high amount of heterogeneity between studies. The majority of studies were small and did not include internal or external validation, therefore the individual performance measures are likely to be optimistic. CONCLUSIONS:Current prognostic models for asthma exacerbations are heterogeneous in methodology, but reported c-statistics suggest a clinically useful model could be created. Studies were consistent in lacking robust validation and in not modelling serial events. Further research is required with respect to incorporating recurrent events, and to externally validate tools in large representative populations to demonstrate the generalizability of published results.
10.1186/s12874-020-0913-7
A critical appraisal of the clinical applicability and risk of bias of the predictive models for mortality and recurrence in patients with oropharyngeal cancer: Systematic review.
Head & neck
The use of predictive models is becoming widespread. However, these models should be developed appropriately (CHecklist for critical Appraisal and data extraction for systematic Reviews of prediction Modeling Studies [CHARMS] and Prediction model Risk Of Bias ASsessment Tool [PROBAST] statements). Concerning mortality/recurrence in oropharyngeal cancer, we are not aware of any systematic reviews of the predictive models. We carried out a systematic review of the MEDLINE/EMBASE databases of those predictive models. In these models, we analyzed the 11 domains of the CHARMS statement and the risk of bias and applicability, using the PROBAST tool. Six papers were finally included in the systematic review and all of them presented high risk of bias and several limitations in the statistical analysis. The applicability was satisfactory in five out of six studies. None of the models could be considered ready for use in clinical practice.
10.1002/hed.26025
[Introduction of the Prediction model Risk Of Bias ASsessment Tool: a tool to assess risk of bias and applicability of prediction model studies].
Chen R,Wang S F,Zhou J C,Sun F,Wei W W,Zhan S Y
Zhonghua liu xing bing xue za zhi = Zhonghua liuxingbingxue zazhi
This paper introduceds the tool named as "Prediction model Risk Of Bias ASsessment Tool" (PROBAST) to assess the risk of bias and applicability in prediction model studies and the relevant items and steps of assessment. PROBAST is organized into four domains including participants, predictors, outcome and analysis. These domains contain a total of 20 signaling questions to facilitate structured judgment of risk of bias occurring in study design, conduct or analysis. Through comprehensive judgment, the risk of bias and applicability of original study is categorized as high, low or unclear. PROBAST enables a focused and transparent approach to assessing the risk of bias of studies that develop, validate, or update prediction models for individualized predictions. Although PROBAST was designed for systematic reviews, it can be also used more generally in critical appraisal of prediction model studies.
10.3760/cma.j.cn112338-20190805-00580
Examining Bias and Reporting in Oral Health Prediction Modeling Studies.
Du M,Haag D,Song Y,Lynch J,Mittinty M
Journal of dental research
Recent efforts to improve the reliability and efficiency of scientific research have caught the attention of researchers conducting prediction modeling studies (PMSs). Use of prediction models in oral health has become more common over the past decades for predicting the risk of diseases and treatment outcomes. Risk of bias and insufficient reporting present challenges to the reproducibility and implementation of these models. A recent tool for bias assessment and a reporting guideline-PROBAST (Prediction Model Risk of Bias Assessment Tool) and TRIPOD (Transparent Reporting of a Multivariable Prediction Model for Individual Prognosis or Diagnosis)-have been proposed to guide researchers in the development and reporting of PMSs, but their application has been limited. Following the standards proposed in these tools and a systematic review approach, a literature search was carried out in PubMed to identify oral health PMSs published in dental, epidemiologic, and biostatistical journals. Risk of bias and transparency of reporting were assessed with PROBAST and TRIPOD. Among 2,881 papers identified, 34 studies containing 58 models were included. The most investigated outcomes were periodontal diseases (42%) and oral cancers (30%). Seventy-five percent of the studies were susceptible to at least 4 of 20 sources of bias, including measurement error in predictors ( = 12) and/or outcome ( = 7), omitting samples with missing data ( = 10), selecting variables based on univariate analyses ( = 9), overfitting ( = 13), and lack of model performance assessment ( = 24). Based on TRIPOD, at least 5 of 31 items were inadequately reported in 95% of the studies. These items included sampling approaches ( = 15), participant eligibility criteria ( = 6), and model-building procedures ( = 16). There was a general lack of transparent reporting and identification of bias across the studies. Application of the recommendations proposed in PROBAST and TRIPOD can benefit future research and improve the reproducibility and applicability of prediction models in oral health.
10.1177/0022034520903725
PROBAST: A Tool to Assess the Risk of Bias and Applicability of Prediction Model Studies.
Annals of internal medicine
Clinical prediction models combine multiple predictors to estimate risk for the presence of a particular condition (diagnostic models) or the occurrence of a certain event in the future (prognostic models). PROBAST (Prediction model Risk Of Bias ASsessment Tool), a tool for assessing the risk of bias (ROB) and applicability of diagnostic and prognostic prediction model studies, was developed by a steering group that considered existing ROB tools and reporting guidelines. The tool was informed by a Delphi procedure involving 38 experts and was refined through piloting. PROBAST is organized into the following 4 domains: participants, predictors, outcome, and analysis. These domains contain a total of 20 signaling questions to facilitate structured judgment of ROB, which was defined to occur when shortcomings in study design, conduct, or analysis lead to systematically distorted estimates of model predictive performance. PROBAST enables a focused and transparent approach to assessing the ROB and applicability of studies that develop, validate, or update prediction models for individualized predictions. Although PROBAST was designed for systematic reviews, it can be used more generally in critical appraisal of prediction model studies. Potential users include organizations supporting decision making, researchers and clinicians who are interested in evidence-based medicine or involved in guideline development, journal editors, and manuscript reviewers.
10.7326/M18-1376