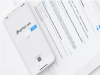
[Updates and interpretation on NCCN clinical practice guidelines for gastric cancer 2017 version 5].
Qiu Haibo,Zhou Zhiwei
Zhonghua wei chang wai ke za zhi = Chinese journal of gastrointestinal surgery
The National Comprehensive Cancer Network (NCCN) issued the clinical practice guidelines for gastric cancer 2017 edition version 5, which has been fully updated for the treatment of gastric cancer, including systematic treatment, surgery and radiotherapy. This article review and summarize the updated NCCN clinical practice guidelines for gastric cancer in 2017 and try to interpret it. (1)Biomarkers: mismatch repair defect (dMMR) or high microsatellite instability (MSI-H), programmed death ligand 1 (PD-L1) and tumor Epstein Barr virus (EBV) status should be considered for patients with gastric cancer. (2)Treatment of advanced gastric cancer: the major update is the application of immunotherapy (Pembrolizumab, Nivolumab combined with Ipilimumab). (3)Adjuvant therapy after D2 resection and perioperative treatment: the guidelines recommended Capecitabine combined with Oxaliplatin as adjuvant therapy after radical operation, updated from category 2A to 1; although the 2017 edition of the NCCN guidelines have not yet been adopted, Docetaxel-based FLOT scheme has certain potential in adjuvant therapy for gastric cancer. (4) Radiotherapy: a more detailed definition of radiotherapy for gastric cancer in different locations, especially in high-risk lymphatic drainage areas, was updated. (5) Genetic risk assessment: the guidelines recommended genetic screening for gastric cancer, including hereditary diffuse gastric cancer (HDGC), Lynch syndrome, juvenile polyposis (JPS), Peutz-Jephers syndrome (PJS) and familial adenomatous polyposis (FAP). The NCCN guidelines continue to update based on new evidences, which is the embodiment of precision medicine in the treatment of gastric cancer. The biggest change in the 2017 gastric cancer guidelines is the updates of immunotherapy, which also suggests that the direction of the gastric cancer treatment began to turn to immunotherapy.
The Chinese Society of Clinical Oncology (CSCO): clinical guidelines for the diagnosis and treatment of gastric cancer.
Wang Feng-Hua,Shen Lin,Li Jin,Zhou Zhi-Wei,Liang Han,Zhang Xiao-Tian,Tang Lei,Xin Yan,Jin Jing,Zhang Yu-Jing,Yuan Xiang-Lin,Liu Tian-Shu,Li Guo-Xin,Wu Qi,Xu Hui-Mian,Ji Jia-Fu,Li Yuan-Fang,Wang Xin,Yu Shan,Liu Hao,Guan Wen-Long,Xu Rui-Hua
Cancer communications (London, England)
China is one of the countries with the highest incidence of gastric cancer. There are differences in epidemiological characteristics, clinicopathological features, tumor biological characteristics, treatment patterns, and drug selection between gastric cancer patients from the Eastern and Western countries. Non-Chinese guidelines cannot specifically reflect the diagnosis and treatment characteristics for the Chinese gastric cancer patients. The Chinese Society of Clinical Oncology (CSCO) arranged for a panel of senior experts specializing in all sub-specialties of gastric cancer to compile, discuss, and revise the guidelines on the diagnosis and treatment of gastric cancer based on the findings of evidence-based medicine in China and abroad. By referring to the opinions of industry experts, taking into account of regional differences, giving full consideration to the accessibility of diagnosis and treatment resources, these experts have conducted experts' consensus judgement on relevant evidence and made various grades of recommendations for the clinical diagnosis and treatment of gastric cancer to reflect the value of cancer treatment and meeting health economic indexes. This guideline uses tables and is complemented by explanatory and descriptive notes covering the diagnosis, comprehensive treatment, and follow-up visits for gastric cancer.
10.1186/s40880-019-0349-9
Guidelines for endoscopic submucosal dissection and endoscopic mucosal resection for early gastric cancer.
Ono Hiroyuki,Yao Kenshi,Fujishiro Mitsuhiro,Oda Ichiro,Nimura Satoshi,Yahagi Naohisa,Iishi Hiroyasu,Oka Masashi,Ajioka Yoichi,Ichinose Masao,Matsui Toshiyuki
Digestive endoscopy : official journal of the Japan Gastroenterological Endoscopy Society
In response to the rapid and wide acceptance and use of endoscopic treatments for early gastric cancer, the Japan Gastroenterological Endoscopy Society (JGES), in collaboration with the Japanese Gastric Cancer Association (JGCA), has produced 'Guidelines for ESD and EMR for Early Gastric Cancer', as a set of basic guidelines in accordance with the principles of evidence-based medicine. These Guidelines cover the present state of knowledge and are divided into the following seven categories: Indications, Preoperative diagnosis, Techniques, Evaluation of curability, Complications, Long-term postoperative surveillance, and Histology. Twenty-three statements were finally accepted as guidelines, and the majority of these were obtained from descriptive studies with lower evidence levels. A number of statements had to be created by consensus (the lowest evidence level), as evidence levels remain low for many specific areas in this field.
10.1111/den.12518
A Lesion-Based Convolutional Neural Network Improves Endoscopic Detection and Depth Prediction of Early Gastric Cancer.
Yoon Hong Jin,Kim Seunghyup,Kim Jie-Hyun,Keum Ji-Soo,Oh Sang-Il,Jo Junik,Chun Jaeyoung,Youn Young Hoon,Park Hyojin,Kwon In Gyu,Choi Seung Ho,Noh Sung Hoon
Journal of clinical medicine
In early gastric cancer (EGC), tumor invasion depth is an important factor for determining the treatment method. However, as endoscopic ultrasonography has limitations when measuring the exact depth in a clinical setting as endoscopists often depend on gross findings and personal experience. The present study aimed to develop a model optimized for EGC detection and depth prediction, and we investigated factors affecting artificial intelligence (AI) diagnosis. We employed a visual geometry group(VGG)-16 model for the classification of endoscopic images as EGC (T1a or T1b) or non-EGC. To induce the model to activate EGC regions during training, we proposed a novel loss function that simultaneously measured classification and localization errors. We experimented with 11,539 endoscopic images (896 T1a-EGC, 809 T1b-EGC, and 9834 non-EGC). The areas under the curves of receiver operating characteristic curves for EGC detection and depth prediction were 0.981 and 0.851, respectively. Among the factors affecting AI prediction of tumor depth, only histologic differentiation was significantly associated, where undifferentiated-type histology exhibited a lower AI accuracy. Thus, the lesion-based model is an appropriate training method for AI in EGC. However, further improvements and validation are required, especially for undifferentiated-type histology.
10.3390/jcm8091310
Highly accurate artificial intelligence systems to predict the invasion depth of gastric cancer: efficacy of conventional white-light imaging, nonmagnifying narrow-band imaging, and indigo-carmine dye contrast imaging.
Gastrointestinal endoscopy
BACKGROUND AND AIMS:Diagnosing the invasion depth of gastric cancer (GC) is necessary to determine the optimal method of treatment. Although the efficacy of evaluating macroscopic features and EUS has been reported, there is a need for more accurate and objective methods. The primary aim of this study was to test the efficacy of novel artificial intelligence (AI) systems in predicting the invasion depth of GC. METHODS:A total of 16,557 images from 1084 cases of GC for which endoscopic resection or surgery was performed between January 2013 and June 2019 were extracted. Cases were randomly assigned to training and test datasets at a ratio of 4:1. Through transfer learning leveraging a convolutional neural network architecture, ResNet50, 3 independent AI systems were developed. Each system was trained to predict the invasion depth of GC using conventional white-light imaging (WLI), nonmagnifying narrow-band imaging (NBI), and indigo-carmine dye contrast imaging (Indigo). RESULTS:The area under the curve of the WLI AI system was .9590. The lesion-based sensitivity, specificity, accuracy, positive predictive value, and negative predictive value of the WLI AI system were 84.4%, 99.4%, 94.5%, 98.5%, and 92.9%, respectively. The lesion-based accuracies of the WLI, NBI, and Indigo AI systems were 94.5%, 94.3%, and 95.5%, respectively, with no significant difference. CONCLUSIONS:These new AI systems trained with multiple images from different angles and distances could predict the invasion depth of GC with high accuracy. The lesion-based accuracy of the WLI, NBI, and Indigo AI systems was not significantly different.
10.1016/j.gie.2020.06.047
Application of convolutional neural network in the diagnosis of the invasion depth of gastric cancer based on conventional endoscopy.
Gastrointestinal endoscopy
BACKGROUND AND AIMS:According to guidelines, endoscopic resection should only be performed for patients whose early gastric cancer invasion depth is within the mucosa or submucosa of the stomach regardless of lymph node involvement. The accurate prediction of invasion depth based on endoscopic images is crucial for screening patients for endoscopic resection. We constructed a convolutional neural network computer-aided detection (CNN-CAD) system based on endoscopic images to determine invasion depth and screen patients for endoscopic resection. METHODS:Endoscopic images of gastric cancer tumors were obtained from the Endoscopy Center of Zhongshan Hospital. An artificial intelligence-based CNN-CAD system was developed through transfer learning leveraging a state-of-the-art pretrained CNN architecture, ResNet50. A total of 790 images served as a development dataset and another 203 images as a test dataset. We used the CNN-CAD system to determine the invasion depth of gastric cancer and evaluated the system's classification accuracy by calculating its sensitivity, specificity, and area under the receiver operating characteristic curve. RESULTS:The area under the receiver operating characteristic curve for the CNN-CAD system was .94 (95% confidence interval [CI], .90-.97). At a threshold value of .5, sensitivity was 76.47%, and specificity 95.56%. Overall accuracy was 89.16%. Positive and negative predictive values were 89.66% and 88.97%, respectively. The CNN-CAD system achieved significantly higher accuracy (by 17.25%; 95% CI, 11.63-22.59) and specificity (by 32.21%; 95% CI, 26.78-37.44) than human endoscopists. CONCLUSIONS:We constructed a CNN-CAD system to determine the invasion depth of gastric cancer with high accuracy and specificity. This system distinguished early gastric cancer from deeper submucosal invasion and minimized overestimation of invasion depth, which could reduce unnecessary gastrectomy.
10.1016/j.gie.2018.11.011