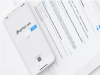
Prognosis and improved outcomes in major depression: a review.
Kraus Christoph,Kadriu Bashkim,Lanzenberger Rupert,Zarate Carlos A,Kasper Siegfried
Translational psychiatry
Treatment outcomes for major depressive disorder (MDD) need to be improved. Presently, no clinically relevant tools have been established for stratifying subgroups or predicting outcomes. This literature review sought to investigate factors closely linked to outcome and summarize existing and novel strategies for improvement. The results show that early recognition and treatment are crucial, as duration of untreated depression correlates with worse outcomes. Early improvement is associated with response and remission, while comorbidities prolong course of illness. Potential biomarkers have been explored, including hippocampal volumes, neuronal activity of the anterior cingulate cortex, and levels of brain-derived neurotrophic factor (BDNF) and central and peripheral inflammatory markers (e.g., translocator protein (TSPO), interleukin-6 (IL-6), C-reactive protein (CRP), tumor necrosis factor alpha (TNFα)). However, their integration into routine clinical care has not yet been fully elucidated, and more research is needed in this regard. Genetic findings suggest that testing for CYP450 isoenzyme activity may improve treatment outcomes. Strategies such as managing risk factors, improving clinical trial methodology, and designing structured step-by-step treatments are also beneficial. Finally, drawing on existing guidelines, we outline a sequential treatment optimization paradigm for selecting first-, second-, and third-line treatments for acute and chronically ill patients. Well-established treatments such as electroconvulsive therapy (ECT) are clinically relevant for treatment-resistant populations, and novel transcranial stimulation methods such as theta-burst stimulation (TBS) and magnetic seizure therapy (MST) have shown promising results. Novel rapid-acting antidepressants, such as ketamine, may also constitute a paradigm shift in treatment optimization for MDD.
10.1038/s41398-019-0460-3
Ensemble Learning for Early-Response Prediction of Antidepressant Treatment in Major Depressive Disorder.
Pei Cong,Sun Yurong,Zhu Jinlong,Wang Xinyi,Zhang Yujie,Zhang Shuqiang,Yao Zhijian,Lu Qing
Journal of magnetic resonance imaging : JMRI
BACKGROUND:In order to reduce unsuccessful treatment trials for depression, neuroimaging and genetic information can be considered as biomarkers. Together with machine-learning methods, prediction models have proved to be valuable for baseline prediction. PURPOSE:To propose an ensemble learning modeling framework that integrates imaging and genetic information for individualized baseline prediction of early-stage treatment response of antidepressants in major depressive disorder (MDD). STUDY TYPE:Prospective. SUBJECTS:In all, 98 inpatients with MDD. FIELD STRENGTH/SEQUENCE:3.0T MRI and gradient-echo echo-planar imaging sequence. ASSESSMENT:Participants were divided into responders and nonresponders based on reducing rates of HDRS-6 after early-stage treatment of 2 weeks. Fourteen brain regions of interest were selected according to previous studies. An ensemble learning modeling framework was used to integrate imaging data and genetic data. STATISTICAL TESTS:Support vector machine (SVM) with linear kernel was utilized to integrate multimode information and then to construct the prediction model. Leave-one-out cross-validation (LOOCV) was used to evaluate the performance. The position characteristics obtained through SVM-RFE (recursive feature elimination) algorithm and LOOCV was considered to compare each feature's relative importance for the prediction model. RESULTS:Compared with the single-level prediction model, the ensemble learning prediction model showed improvement in prediction performance (accuracy from 0.61 to 0.86 with imaging data and genetic data). Integrated with 14 priori brain regions, the region of interest (ROI) map ensemble learning prediction model can achieve a performance that is analogous with the model with information from whole-brain regions (both with accuracy of 0.81). The integration of genetic features further improved the sensitivity of prediction (sensitivity from 0.78 to 0.87 under the ensemble learning framework). DATA CONCLUSION:Our ensemble learning prediction model demonstrated significant advantages in interpretability and information integration. The findings may provide more assistance for clinical treatment selection in MDD at the individual level. LEVEL OF EVIDENCE:1 Technical Efficacy: Stage 2 J. Magn. Reson. Imaging 2020;52:161-171.
10.1002/jmri.27029