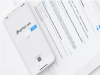
Screening for cardiac contractile dysfunction using an artificial intelligence-enabled electrocardiogram.
Nature medicine
Asymptomatic left ventricular dysfunction (ALVD) is present in 3-6% of the general population, is associated with reduced quality of life and longevity, and is treatable when found. An inexpensive, noninvasive screening tool for ALVD in the doctor's office is not available. We tested the hypothesis that application of artificial intelligence (AI) to the electrocardiogram (ECG), a routine method of measuring the heart's electrical activity, could identify ALVD. Using paired 12-lead ECG and echocardiogram data, including the left ventricular ejection fraction (a measure of contractile function), from 44,959 patients at the Mayo Clinic, we trained a convolutional neural network to identify patients with ventricular dysfunction, defined as ejection fraction ≤35%, using the ECG data alone. When tested on an independent set of 52,870 patients, the network model yielded values for the area under the curve, sensitivity, specificity, and accuracy of 0.93, 86.3%, 85.7%, and 85.7%, respectively. In patients without ventricular dysfunction, those with a positive AI screen were at 4 times the risk (hazard ratio, 4.1; 95% confidence interval, 3.3 to 5.0) of developing future ventricular dysfunction compared with those with a negative screen. Application of AI to the ECG-a ubiquitous, low-cost test-permits the ECG to serve as a powerful screening tool in asymptomatic individuals to identify ALVD.
10.1038/s41591-018-0240-2
Artificial intelligence algorithm for predicting cardiac arrest using electrocardiography.
Kwon Joon-Myoung,Kim Kyung-Hee,Jeon Ki-Hyun,Lee Soo Youn,Park Jinsik,Oh Byung-Hee
Scandinavian journal of trauma, resuscitation and emergency medicine
BACKGROUND:In-hospital cardiac arrest is a major burden in health care. Although several track-and-trigger systems are used to predict cardiac arrest, they often have unsatisfactory performances. We hypothesized that a deep-learning-based artificial intelligence algorithm (DLA) could effectively predict cardiac arrest using electrocardiography (ECG). We developed and validated a DLA for predicting cardiac arrest using ECG. METHODS:We conducted a retrospective study that included 47,505 ECGs of 25,672 adult patients admitted to two hospitals, who underwent at least one ECG from October 2016 to September 2019. The endpoint was occurrence of cardiac arrest within 24 h from ECG. Using subgroup analyses in patients who were initially classified as non-event, we confirmed the delayed occurrence of cardiac arrest and unexpected intensive care unit transfer over 14 days. RESULTS:We used 32,294 ECGs of 10,461 patients and 4483 ECGs of 4483 patients from a hospital were used as development and internal validation data, respectively. Additionally, 10,728 ECGs of 10,728 patients from another hospital were used as external validation data, which confirmed the robustness of the developed DLA. During internal and external validation, the areas under the receiver operating characteristic curves of the DLA in predicting cardiac arrest within 24 h were 0.913 and 0.948, respectively. The high risk group of the DLA showed a significantly higher hazard for delayed cardiac arrest (5.74% vs. 0.33%, P < 0.001) and unexpected intensive care unit transfer (4.23% vs. 0.82%, P < 0.001). A sensitivity map of the DLA displayed the ECG regions used to predict cardiac arrest, with the DLA focused most on the QRS complex. CONCLUSIONS:Our DLA successfully predicted cardiac arrest using diverse formats of ECG. The results indicate that cardiac arrest could be screened and predicted not only with a conventional 12-lead ECG, but also with a single-lead ECG using a wearable device that employs our DLA.
10.1186/s13049-020-00791-0
Performance of a convolutional neural network derived from an ECG database in recognizing myocardial infarction.
Scientific reports
Artificial intelligence (AI) is developing rapidly in the medical technology field, particularly in image analysis. ECG-diagnosis is an image analysis in the sense that cardiologists assess the waveforms presented in a 2-dimensional image. We hypothesized that an AI using a convolutional neural network (CNN) may also recognize ECG images and patterns accurately. We used the PTB ECG database consisting of 289 ECGs including 148 myocardial infarction (MI) cases to develop a CNN to recognize MI in ECG. Our CNN model, equipped with 6-layer architecture, was trained with training-set ECGs. After that, our CNN and 10 physicians are tested with test-set ECGs and compared their MI recognition capability in metrics F1 (harmonic mean of precision and recall) and accuracy. The F1 and accuracy by our CNN were significantly higher (83 ± 4%, 81 ± 4%) as compared to physicians (70 ± 7%, 67 ± 7%, P < 0.0001, respectively). Furthermore, elimination of Goldberger-leads or ECG image compression up to quarter resolution did not significantly decrease the recognition capability. Deep learning with a simple CNN for image analysis may achieve a comparable capability to physicians in recognizing MI on ECG. Further investigation is warranted for the use of AI in ECG image assessment.
10.1038/s41598-020-65105-x
Artificial intelligence for early prediction of pulmonary hypertension using electrocardiography.
Kwon Joon-Myoung,Kim Kyung-Hee,Medina-Inojosa Jose,Jeon Ki-Hyun,Park Jinsik,Oh Byung-Hee
The Journal of heart and lung transplantation : the official publication of the International Society for Heart Transplantation
BACKGROUND:Screening and early diagnosis of pulmonary hypertension (PH) are critical for managing progression and preventing associated mortality; however, there are no tools for this purpose. We developed and validated an artificial intelligence (AI) algorithm for predicting PH using electrocardiography (ECG). METHODS:This historical cohort study included data from consecutive patients from 2 hospitals. The patients in one hospital were divided into derivation (56,670 ECGs from 24,202 patients) and internal validation (3,174 ECGs from 3,174 patients) datasets, whereas the patients in the other hospital were included in only an external validation (10,865 ECGs from 10,865 patients) dataset. An AI algorithm based on an ensemble neural network was developed using 12-lead ECG signal and demographic information from the derivation dataset. The end-point was the diagnosis of PH. In addition, the interpretable AI algorithm identified which region had the most significant effect on decision making using a sensitivity map. RESULTS:During the internal and external validation, the area under the receiver operating characteristic curve of the AI algorithm for detecting PH was 0.859 and 0.902, respectively. In the 2,939 individuals without PH at initial echocardiography, those patients that the AI defined as having a higher risk had a significantly higher chance of developing PH than those in the low-risk group (31.5% vs 5.9%, p < 0.001) during the follow-up period. The sensitivity map showed that the AI algorithm focused on the S-wave, P-wave, and T-wave for each patient by QRS complex characteristics. CONCLUSIONS:The AI algorithm demonstrated high accuracy for PH prediction using 12-lead and single-lead ECGs.
10.1016/j.healun.2020.04.009
Comparing the performance of artificial intelligence and conventional diagnosis criteria for detecting left ventricular hypertrophy using electrocardiography.
Kwon Joon-Myoung,Jeon Ki-Hyun,Kim Hyue Mee,Kim Min Jeong,Lim Sung Min,Kim Kyung-Hee,Song Pil Sang,Park Jinsik,Choi Rak Kyeong,Oh Byung-Hee
Europace : European pacing, arrhythmias, and cardiac electrophysiology : journal of the working groups on cardiac pacing, arrhythmias, and cardiac cellular electrophysiology of the European Society of Cardiology
AIMS:Although left ventricular hypertrophy (LVH) has a high incidence and clinical importance, the conventional diagnosis criteria for detecting LVH using electrocardiography (ECG) has not been satisfied. We aimed to develop an artificial intelligence (AI) algorithm for detecting LVH. METHODS AND RESULTS:This retrospective cohort study involved the review of 21 286 patients who were admitted to two hospitals between October 2016 and July 2018 and underwent 12-lead ECG and echocardiography within 4 weeks. The patients in one hospital were divided into a derivation and internal validation dataset, while the patients in the other hospital were included in only an external validation dataset. An AI algorithm based on an ensemble neural network (ENN) combining convolutional and deep neural network was developed using the derivation dataset. And we visualized the ECG area that the AI algorithm used to make the decision. The area under the receiver operating characteristic curve of the AI algorithm based on ENN was 0.880 (95% confidence interval 0.877-0.883) and 0.868 (0.865-0.871) during the internal and external validations. These results significantly outperformed the cardiologist's clinical assessment with Romhilt-Estes point system and Cornell voltage criteria, Sokolov-Lyon criteria, and interpretation of ECG machine. At the same specificity, the AI algorithm based on ENN achieved 159.9%, 177.7%, and 143.8% higher sensitivities than those of the cardiologist's assessment, Sokolov-Lyon criteria, and interpretation of ECG machine. CONCLUSION:An AI algorithm based on ENN was highly able to detect LVH and outperformed cardiologists, conventional methods, and other machine learning techniques.
10.1093/europace/euz324
Artificial Intelligence-Enabled ECG: a Modern Lens on an Old Technology.
Kashou Anthony H,May Adam M,Noseworthy Peter A
Current cardiology reports
PURPOSE OF REVIEW:To (i) review the concept of artificial intelligence (AI); (ii) summarize recent developments in artificial intelligence-enabled electrocardiogram (AI-ECG); (iii) address notable inherent limitations and challenges of AI-ECG; and (iv) discuss the future direction of the field. RECENT FINDINGS:Advancements in machine learning and computing methods have led to application of AI-ECG and potential new applications to patient care. Further study is needed to verify previous findings in diverse populations as well as begin to confront the limitations needed for clinical implementation. Nearly one century after the Nobel Prize was awarded to Willem Einthoven for demonstrating that an electrocardiogram (ECG) could record the electrical signature of the heart, the ECG remains one of the most important diagnostic tests in modern medicine. We now stand at the edge of true ECG innovation. Simultaneous advancements in computing power, wireless technology, digitized data availability, and machine learning have led to the birth of AI-ECG algorithms with novel capabilities and real potential for clinical application. AI has the potential to improve diagnostic accuracy and efficiency by providing fully automated, unbiased, and unambiguous ECG analysis along with promising new findings that may unlock new value in the ECG. These breakthroughs may cause a paradigm shift in clinical workflow as well as patient monitoring and management.
10.1007/s11886-020-01317-x
Artificial intelligence for detecting mitral regurgitation using electrocardiography.
Kwon Joon-Myoung,Kim Kyung-Hee,Akkus Zeynettin,Jeon Ki-Hyun,Park Jinsik,Oh Byung-Hee
Journal of electrocardiology
BACKGROUND:Screening and early diagnosis of mitral regurgitation (MR) are crucial for preventing irreversible progression of MR. In this study, we developed and validated an artificial intelligence (AI) algorithm for detecting MR using electrocardiography (ECG). METHODS:This retrospective cohort study included data from two hospital. An AI algorithm was trained using 56,670 ECGs from 24,202 patients. Internal validation of the algorithm was performed with 3174 ECGs of 3174 patients from one hospital, while external validation was performed with 10,865 ECGs of 10,865 patients from another hospital. The endpoint was the diagnosis of significant MR, moderate to severe, confirmed by echocardiography. We used 500 Hz ECG raw data as predictive variables. Additionally, we showed regions of ECG that have the most significant impact on the decision-making of the AI algorithm using a sensitivity map. RESULTS:During the internal and external validation, the area under the receiver operating characteristic curve of the AI algorithm using a 12-lead ECG for detecting MR was 0.816 and 0.877, respectively, while that using a single-lead ECG was 0.758 and 0.850, respectively. In the 3157 non-MR individuals, those patients that the AI defined as high risk had a significantly higher chance of development of MR than the low risk group (13.9% vs. 2.6%, p < 0.001) during the follow-up period. The sensitivity map showed the AI algorithm focused on the P-wave and T-wave for MR patients and QRS complex for non-MR patients. CONCLUSIONS:The proposed AI algorithm demonstrated promising results for MR detecting using 12-lead and single-lead ECGs.
10.1016/j.jelectrocard.2020.02.008
Artificial Intelligence ECG to Detect Left Ventricular Dysfunction in COVID-19: A Case Series.
Mayo Clinic proceedings
Coronavirus disease 2019 (COVID-19) can result in deterioration of cardiac function, which is associated with high mortality. A simple point-of-care diagnostic test to screen for ventricular dysfunction would be clinically useful to guide management. We sought to review the clinical experience with an artificial intelligence electrocardiogram (AI ECG) to screen for ventricular dysfunction in patients with documented COVID-19. We examined all patients in the Mayo Clinic system who underwent clinically indicated electrocardiography and echocardiography within 2 weeks following a positive COVID-19 test and had permitted use of their data for research were included. Of the 27 patients who met the inclusion criteria, one had a history of normal ventricular function who developed COVID-19 myocarditis with rapid clinical decline. The initial AI ECG in this patient indicated normal ventricular function. Repeat AI ECG showed a probability of ejection fraction (EF) less than or equal to 40% of 90.2%, corroborated with an echocardiographic EF of 35%. One other patient had a pre-existing EF less than or equal to 40%, accurately detected by the algorithm before and after COVID-19 diagnosis, and another was found to have a low EF by AI ECG and echocardiography with the COVID-19 diagnosis. The area under the curve for detection of EF less than or equal to 40% was 0.95. This case series suggests that the AI ECG, previously shown to detect ventricular dysfunction in a large general population, may be useful as a screening tool for the detection of cardiac dysfunction in patients with COVID-19.
10.1016/j.mayocp.2020.09.020
Precision Medicine and Artificial Intelligence: A Pilot Study on Deep Learning for Hypoglycemic Events Detection based on ECG.
Scientific reports
Tracking the fluctuations in blood glucose levels is important for healthy subjects and crucial diabetic patients. Tight glucose monitoring reduces the risk of hypoglycemia, which can result in a series of complications, especially in diabetic patients, such as confusion, irritability, seizure and can even be fatal in specific conditions. Hypoglycemia affects the electrophysiology of the heart. However, due to strong inter-subject heterogeneity, previous studies based on a cohort of subjects failed to deploy electrocardiogram (ECG)-based hypoglycemic detection systems reliably. The current study used personalised medicine approach and Artificial Intelligence (AI) to automatically detect nocturnal hypoglycemia using a few heartbeats of raw ECG signal recorded with non-invasive, wearable devices, in healthy individuals, monitored 24 hours for 14 consecutive days. Additionally, we present a visualisation method enabling clinicians to visualise which part of the ECG signal (e.g., T-wave, ST-interval) is significantly associated with the hypoglycemic event in each subject, overcoming the intelligibility problem of deep-learning methods. These results advance the feasibility of a real-time, non-invasive hypoglycemia alarming system using short excerpts of ECG signal.
10.1038/s41598-019-56927-5
Artificial Intelligence Algorithm for Screening Heart Failure with Reduced Ejection Fraction Using Electrocardiography.
Cho Jinwoo,Lee ByeongTak,Kwon Joon-Myoung,Lee Yeha,Park Hyunho,Oh Byung-Hee,Jeon Ki-Hyun,Park Jinsik,Kim Kyung-Hee
ASAIO journal (American Society for Artificial Internal Organs : 1992)
Although heart failure with reduced ejection fraction (HFrEF) is a common clinical syndrome and can be modified by the administration of appropriate medical therapy, there is no adequate tool available to perform reliable, economical, early-stage screening. To meet this need, we developed an interpretable artificial intelligence (AI) algorithm for HFrEF screening using electrocardiography (ECG) and validated its performance. This retrospective cohort study included two hospitals. An AI algorithm based on a convolutional neural network was developed using 39,371 ECG results from 17,127 patients. The internal validation included 3,470 ECGs from 2,908 patients. Furthermore, we conducted external validation using 4,362 ECGs from 4,176 patients from another hospital to verify the applicability of the algorithm across different centers. The end-point was to detect HFrEF, defined as an ejection fraction <40%. We also visualized the regions in 12 lead ECG that affected HFrEF detection in the AI algorithm and compared this to the previously documented literature. During the internal and external validation, the areas under the curves of the AI algorithm using a 12 lead ECG for detecting HFrEF were 0.913 (95% confidence interval, 0.902-0.925) and 0.961 (0.951-0.971), respectively, and the areas under the curves of the AI algorithm using a single-lead ECG were 0.874 (0.859-0.890) and 0.929 (0.911-0.946), respectively. The deep learning-based AI algorithm performed HFrEF detection well using not only a 12 lead but also a single-lead ECG. These results suggest that HFrEF can be screened not only using a 12 lead ECG, as is typical of a conventional ECG machine, but also with a single-lead ECG performed by a wearable device employing the AI algorithm, thereby preventing irreversible disease progression and mortality.
10.1097/MAT.0000000000001218
Artificial Intelligence-Electrocardiography to Predict Incident Atrial Fibrillation: A Population-Based Study.
Christopoulos Georgios,Graff-Radford Jonathan,Lopez Camden L,Yao Xiaoxi,Attia Zachi I,Rabinstein Alejandro A,Petersen Ronald C,Knopman David S,Mielke Michelle M,Kremers Walter,Vemuri Prashanthi,Siontis Konstantinos C,Friedman Paul A,Noseworthy Peter A
Circulation. Arrhythmia and electrophysiology
BACKGROUND:An artificial intelligence (AI) algorithm applied to electrocardiography during sinus rhythm has recently been shown to detect concurrent episodic atrial fibrillation (AF). We sought to characterize the value of AI-enabled electrocardiography (AI-ECG) as a predictor of future AF and assess its performance compared with the CHARGE-AF score (Cohorts for Aging and Research in Genomic Epidemiology-AF) in a population-based sample. METHODS:We calculated the probability of AF using AI-ECG, among participants in the population-based Mayo Clinic Study of Aging who had no history of AF at the time of the baseline study visit. Cox proportional hazards models were fit to assess the independent prognostic value and interaction between AI-ECG AF model output and CHARGE-AF score. C statistics were calculated for AI-ECG AF model output, CHARGE-AF score, and combined AI-ECG and CHARGE-AF score. RESULTS:A total of 1936 participants with median age 75.8 (interquartile range, 70.4-81.8) years and median CHARGE-AF score 14.0 (IQR, 13.2-14.7) were included in the analysis. Participants with AI-ECG AF model output of >0.5 at the baseline visit had cumulative incidence of AF 21.5% at 2 years and 52.2% at 10 years. When included in the same model, both AI-ECG AF model output (hazard ratio, 1.76 per SD after logit transformation [95% CI, 1.51-2.04]) and CHARGE-AF score (hazard ratio, 1.90 per SD [95% CI, 1.58-2.28]) independently predicted future AF without significant interaction (=0.54). C statistics were 0.69 (95% CI, 0.66-0.72) for AI-ECG AF model output, 0.69 (95% CI, 0.66-0.71) for CHARGE-AF, and 0.72 (95% CI, 0.69-0.75) for combined AI-ECG and CHARGE-AF score. CONCLUSIONS:In the present study, both the AI-ECG AF model output and CHARGE-AF score independently predicted incident AF. The AI-ECG may offer a means to assess risk with a single test and without requiring manual or automated clinical data abstraction.
10.1161/CIRCEP.120.009355
ECG AI-Guided Screening for Low Ejection Fraction (EAGLE): Rationale and design of a pragmatic cluster randomized trial.
Yao Xiaoxi,McCoy Rozalina G,Friedman Paul A,Shah Nilay D,Barry Barbara A,Behnken Emma M,Inselman Jonathan W,Attia Zachi I,Noseworthy Peter A
American heart journal
BACKGROUND:A deep learning algorithm to detect low ejection fraction (EF) using routine 12-lead electrocardiogram (ECG) has recently been developed and validated. The algorithm was incorporated into the electronic health record (EHR) to automatically screen for low EF, encouraging clinicians to obtain a confirmatory transthoracic echocardiogram (TTE) for previously undiagnosed patients, thereby facilitating early diagnosis and treatment. OBJECTIVES:To prospectively evaluate a novel artificial intelligence (AI) screening tool for detecting low EF in primary care practices. DESIGN:The EAGLE trial is a pragmatic two-arm cluster randomized trial (NCT04000087) that will randomize >100 clinical teams (i.e., clusters) to either intervention (access to the new AI screening tool) or control (usual care) at 48 primary care practices across Minnesota and Wisconsin. The trial is expected to involve approximately 400 clinicians and 20,000 patients. The primary endpoint is newly discovered EF ≤50%. Eligible patients will include adults who undergo ECG for any reason and have not been previously diagnosed with low EF. Data will be pulled from the EHR, and no contact will be made with patients. A positive deviance qualitative study and a post-implementation survey will be conducted among select clinicians to identify facilitators and barriers to using the new screening report. SUMMARY:This trial will examine the effectiveness of the AI-enabled ECG for detection of asymptomatic low EF in routine primary care practices and will be among the first to prospectively evaluate the value of AI in real-world practice. Its findings will inform future implementation strategies for the translation of other AI-enabled algorithms.
10.1016/j.ahj.2019.10.007
An artificial intelligence-enabled ECG algorithm for the identification of patients with atrial fibrillation during sinus rhythm: a retrospective analysis of outcome prediction.
Attia Zachi I,Noseworthy Peter A,Lopez-Jimenez Francisco,Asirvatham Samuel J,Deshmukh Abhishek J,Gersh Bernard J,Carter Rickey E,Yao Xiaoxi,Rabinstein Alejandro A,Erickson Brad J,Kapa Suraj,Friedman Paul A
Lancet (London, England)
BACKGROUND:Atrial fibrillation is frequently asymptomatic and thus underdetected but is associated with stroke, heart failure, and death. Existing screening methods require prolonged monitoring and are limited by cost and low yield. We aimed to develop a rapid, inexpensive, point-of-care means of identifying patients with atrial fibrillation using machine learning. METHODS:We developed an artificial intelligence (AI)-enabled electrocardiograph (ECG) using a convolutional neural network to detect the electrocardiographic signature of atrial fibrillation present during normal sinus rhythm using standard 10-second, 12-lead ECGs. We included all patients aged 18 years or older with at least one digital, normal sinus rhythm, standard 10-second, 12-lead ECG acquired in the supine position at the Mayo Clinic ECG laboratory between Dec 31, 1993, and July 21, 2017, with rhythm labels validated by trained personnel under cardiologist supervision. We classified patients with at least one ECG with a rhythm of atrial fibrillation or atrial flutter as positive for atrial fibrillation. We allocated ECGs to the training, internal validation, and testing datasets in a 7:1:2 ratio. We calculated the area under the curve (AUC) of the receiver operatoring characteristic curve for the internal validation dataset to select a probability threshold, which we applied to the testing dataset. We evaluated model performance on the testing dataset by calculating the AUC and the accuracy, sensitivity, specificity, and F1 score with two-sided 95% CIs. FINDINGS:We included 180 922 patients with 649 931 normal sinus rhythm ECGs for analysis: 454 789 ECGs recorded from 126 526 patients in the training dataset, 64 340 ECGs from 18 116 patients in the internal validation dataset, and 130 802 ECGs from 36 280 patients in the testing dataset. 3051 (8·4%) patients in the testing dataset had verified atrial fibrillation before the normal sinus rhythm ECG tested by the model. A single AI-enabled ECG identified atrial fibrillation with an AUC of 0·87 (95% CI 0·86-0·88), sensitivity of 79·0% (77·5-80·4), specificity of 79·5% (79·0-79·9), F1 score of 39·2% (38·1-40·3), and overall accuracy of 79·4% (79·0-79·9). Including all ECGs acquired during the first month of each patient's window of interest (ie, the study start date or 31 days before the first recorded atrial fibrillation ECG) increased the AUC to 0·90 (0·90-0·91), sensitivity to 82·3% (80·9-83·6), specificity to 83·4% (83·0-83·8), F1 score to 45·4% (44·2-46·5), and overall accuracy to 83·3% (83·0-83·7). INTERPRETATION:An AI-enabled ECG acquired during normal sinus rhythm permits identification at point of care of individuals with atrial fibrillation. FUNDING:None.
10.1016/S0140-6736(19)31721-0