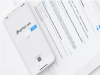
RNA secondary structure prediction using an ensemble of two-dimensional deep neural networks and transfer learning.
Nature communications
The majority of our human genome transcribes into noncoding RNAs with unknown structures and functions. Obtaining functional clues for noncoding RNAs requires accurate base-pairing or secondary-structure prediction. However, the performance of such predictions by current folding-based algorithms has been stagnated for more than a decade. Here, we propose the use of deep contextual learning for base-pair prediction including those noncanonical and non-nested (pseudoknot) base pairs stabilized by tertiary interactions. Since only [Formula: see text]250 nonredundant, high-resolution RNA structures are available for model training, we utilize transfer learning from a model initially trained with a recent high-quality bpRNA dataset of [Formula: see text]10,000 nonredundant RNAs made available through comparative analysis. The resulting method achieves large, statistically significant improvement in predicting all base pairs, noncanonical and non-nested base pairs in particular. The proposed method (SPOT-RNA), with a freely available server and standalone software, should be useful for improving RNA structure modeling, sequence alignment, and functional annotations.
10.1038/s41467-019-13395-9
Prediction of Carbon Dioxide Adsorption via Deep Learning.
Zhang Zihao,Schott Jennifer A,Liu Miaomiao,Chen Hao,Lu Xiuyang,Sumpter Bobby G,Fu Jie,Dai Sheng
Angewandte Chemie (International ed. in English)
Porous carbons with different textural properties exhibit great differences in CO adsorption capacity. It is generally known that narrow micropores contribute to higher CO adsorption capacity. However, it is still unclear what role each variable in the textural properties plays in CO adsorption. Herein, a deep neural network is trained as a generative model to direct the relationship between CO adsorption of porous carbons and corresponding textural properties. The trained neural network is further employed as an implicit model to estimate its ability to predict the CO adsorption capacity of unknown porous carbons. Interestingly, the practical CO adsorption amounts are in good agreement with predicted values using surface area, micropore and mesopore volumes as the input values simultaneously. This unprecedented deep learning neural network (DNN) approach, a type of machine learning algorithm, exhibits great potential to predict gas adsorption and guide the development of next-generation carbons.
10.1002/anie.201812363