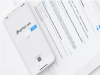
Deep learning in mental health outcome research: a scoping review.
Su Chang,Xu Zhenxing,Pathak Jyotishman,Wang Fei
Translational psychiatry
Mental illnesses, such as depression, are highly prevalent and have been shown to impact an individual's physical health. Recently, artificial intelligence (AI) methods have been introduced to assist mental health providers, including psychiatrists and psychologists, for decision-making based on patients' historical data (e.g., medical records, behavioral data, social media usage, etc.). Deep learning (DL), as one of the most recent generation of AI technologies, has demonstrated superior performance in many real-world applications ranging from computer vision to healthcare. The goal of this study is to review existing research on applications of DL algorithms in mental health outcome research. Specifically, we first briefly overview the state-of-the-art DL techniques. Then we review the literature relevant to DL applications in mental health outcomes. According to the application scenarios, we categorize these relevant articles into four groups: diagnosis and prognosis based on clinical data, analysis of genetics and genomics data for understanding mental health conditions, vocal and visual expression data analysis for disease detection, and estimation of risk of mental illness using social media data. Finally, we discuss challenges in using DL algorithms to improve our understanding of mental health conditions and suggest several promising directions for their applications in improving mental health diagnosis and treatment.
10.1038/s41398-020-0780-3
Development and Validation of an Electronic Health Record-Based Machine Learning Model to Estimate Delirium Risk in Newly Hospitalized Patients Without Known Cognitive Impairment.
Wong Andrew,Young Albert T,Liang April S,Gonzales Ralph,Douglas Vanja C,Hadley Dexter
JAMA network open
Importance:Current methods for identifying hospitalized patients at increased risk of delirium require nurse-administered questionnaires with moderate accuracy. Objective:To develop and validate a machine learning model that predicts incident delirium risk based on electronic health data available on admission. Design, Setting, and Participants:Retrospective cohort study evaluating 5 machine learning algorithms to predict delirium using 796 clinical variables identified by an expert panel as relevant to delirium prediction and consistently available in electronic health records within 24 hours of admission. The training set comprised 14 227 adult patients with non-intensive care unit hospital stays and no delirium on admission who were discharged between January 1, 2016, and August 31, 2017, from UCSF Health, a large academic health institution. The test set comprised 3996 patients with hospital stays who were discharged between August 1, 2017, and November 30, 2017. Exposures:Patient demographic characteristics, diagnoses, nursing records, laboratory results, and medications available in electronic health records during hospitalization. Main Outcomes and Measures:Delirium was defined as a positive Nursing Delirium Screening Scale or Confusion Assessment Method for the Intensive Care Unit score. Models were assessed using the area under the receiver operating characteristic curve (AUC) and compared against the 4-point scoring system AWOL (age >79 years, failure to spell world backward, disorientation to place, and higher nurse-rated illness severity), a validated delirium risk-assessment tool routinely administered in this cohort. Results:The training set included 14 227 patients (5113 [35.9%] aged >64 years; 7335 [51.6%] female; 687 [4.8%] with delirium), and the test set included 3996 patients (1491 [37.3%] aged >64 years; 1966 [49.2%] female; 191 [4.8%] with delirium). In total, the analysis included 18 223 hospital admissions (6604 [36.2%] aged >64 years; 9301 [51.0%] female; 878 [4.8%] with delirium). The AWOL system achieved a baseline AUC of 0.678. The gradient boosting machine model performed best, with an AUC of 0.855. Setting specificity at 90%, the model had a 59.7% (95% CI, 52.4%-66.7%) sensitivity, 23.1% (95% CI, 20.5%-25.9%) positive predictive value, 97.8% (95% CI, 97.4%-98.1%) negative predictive value, and a number needed to screen of 4.8. Penalized logistic regression and random forest models also performed well, with AUCs of 0.854 and 0.848, respectively. Conclusions and Relevance:Machine learning can be used to estimate hospital-acquired delirium risk using electronic health record data available within 24 hours of hospital admission. Such a model may allow more precise targeting of delirium prevention resources without increasing the burden on health care professionals.
10.1001/jamanetworkopen.2018.1018
Adverse Drug Event Detection from Electronic Health Records Using Hierarchical Recurrent Neural Networks with Dual-Level Embedding.
Wunnava Susmitha,Qin Xiao,Kakar Tabassum,Sen Cansu,Rundensteiner Elke A,Kong Xiangnan
Drug safety
INTRODUCTION:Adverse drug event (ADE) detection is a vital step towards effective pharmacovigilance and prevention of future incidents caused by potentially harmful ADEs. The electronic health records (EHRs) of patients in hospitals contain valuable information regarding ADEs and hence are an important source for detecting ADE signals. However, EHR texts tend to be noisy. Yet applying off-the-shelf tools for EHR text preprocessing jeopardizes the subsequent ADE detection performance, which depends on a well tokenized text input. OBJECTIVE:In this paper, we report our experience with the NLP Challenges for Detecting Medication and Adverse Drug Events from Electronic Health Records (MADE1.0), which aims to promote deep innovations on this subject. In particular, we have developed rule-based sentence and word tokenization techniques to deal with the noise in the EHR text. METHODS:We propose a detection methodology by adapting a three-layered, deep learning architecture of (1) recurrent neural network [bi-directional long short-term memory (Bi-LSTM)] for character-level word representation to encode the morphological features of the medical terminology, (2) Bi-LSTM for capturing the contextual information of each word within a sentence, and (3) conditional random fields for the final label prediction by also considering the surrounding words. We experiment with different word embedding methods commonly used in word-level classification tasks and demonstrate the impact of an integrated usage of both domain-specific and general-purpose pre-trained word embedding for detecting ADEs from EHRs. RESULTS:Our system was ranked first for the named entity recognition task in the MADE1.0 challenge, with a micro-averaged F1-score of 0.8290 (official score). CONCLUSION:Our results indicate that the integration of two widely used sequence labeling techniques that complement each other along with dual-level embedding (character level and word level) to represent words in the input layer results in a deep learning architecture that achieves excellent information extraction accuracy for EHR notes.
10.1007/s40264-018-0765-9
Artificial intelligence sepsis prediction algorithm learns to say "I don't know".
NPJ digital medicine
Sepsis is a leading cause of morbidity and mortality worldwide. Early identification of sepsis is important as it allows timely administration of potentially life-saving resuscitation and antimicrobial therapy. We present COMPOSER (COnformal Multidimensional Prediction Of SEpsis Risk), a deep learning model for the early prediction of sepsis, specifically designed to reduce false alarms by detecting unfamiliar patients/situations arising from erroneous data, missingness, distributional shift and data drifts. COMPOSER flags these unfamiliar cases as indeterminate rather than making spurious predictions. Six patient cohorts (515,720 patients) curated from two healthcare systems in the United States across intensive care units (ICU) and emergency departments (ED) were used to train and externally and temporally validate this model. In a sequential prediction setting, COMPOSER achieved a consistently high area under the curve (AUC) (ICU: 0.925-0.953; ED: 0.938-0.945). Out of over 6 million prediction windows roughly 20% and 8% were identified as indeterminate amongst non-septic and septic patients, respectively. COMPOSER provided early warning within a clinically actionable timeframe (ICU: 12.2 [3.2 22.8] and ED: 2.1 [0.8 4.5] hours prior to first antibiotics order) across all six cohorts, thus allowing for identification and prioritization of patients at high risk for sepsis.
10.1038/s41746-021-00504-6
Emerging role of eHealth in the identification of very early inflammatory rheumatic diseases.
Kataria Suchitra,Ravindran Vinod
Best practice & research. Clinical rheumatology
Digital health or eHealth technologies, notably pervasive computing, robotics, big-data, wearable devices, machine learning, and artificial intelligence (AI), have opened unprecedented opportunities as to how the diseases are diagnosed and managed with active patient engagement. Patient-related data have provided insights (real world data) into understanding the disease processes. Advanced analytics have refined these insights further to draw dynamic algorithms aiding clinicians in making more accurate diagnosis with the help of machine learning. AI is another tool, which, although is still in the evolution stage, has the potential to help identify early signs even before the clinical features are apparent. The evolving digital developments pose challenges on allowing access to health-related data for further research but, at the same time, protecting each patient's privacy. This review focuses on the recent technological advances and their applications and highlights the immense potential to enable early diagnosis of rheumatological diseases.
10.1016/j.berh.2019.101429
Digital Medicine in Rheumatology: Challenges and Opportunities.
Venuturupalli R Swamy,Sufka Paul,Bhana Suleman
Rheumatic diseases clinics of North America
The exponential growth in technology has had a significant effect on the practice of medicine and will likely transform the practice of medicine. In this article, the authors review select technologies that are already influencing the practice of rheumatology. Social media Websites such as Twitter are now important sources of information and discussion for health care professionals interested in rheumatology. Virtual reality is an innovative technology with great potential for acute and chronic pain management. The authors also review several low-cost technology alternatives to commonly used tools used in rheumatology.
10.1016/j.rdc.2018.09.010
Digital health technologies: opportunities and challenges in rheumatology.
Solomon Daniel H,Rudin Robert S
Nature reviews. Rheumatology
The past decade in rheumatology has seen tremendous innovation in digital health technologies, including the electronic health record, virtual visits, mobile health, wearable technology, digital therapeutics, artificial intelligence and machine learning. The increased availability of these technologies offers opportunities for improving important aspects of rheumatology, including access, outcomes, adherence and research. However, despite its growth in some areas, particularly with non-health-care consumers, digital health technology has not substantially changed the delivery of rheumatology care. This Review discusses key barriers and opportunities to improve application of digital health technologies in rheumatology. Key topics include smart design, voice enablement and the integration of electronic patient-reported outcomes. Smart design involves active engagement with the end users of the technologies, including patients and clinicians through focus groups, user testing sessions and prototype review. Voice enablement using voice assistants could be critical for enabling patients with hand arthritis to effectively use smartphone apps and might facilitate patient engagement with many technologies. Tracking many rheumatic diseases requires frequent monitoring of patient-reported outcomes. Current practice only collects this information sporadically, and rarely between visits. Digital health technology could enable patient-reported outcomes to inform appropriate timing of face-to-face visits and enable improved application of treat-to-target strategies. However, best practice standards for digital health technologies do not yet exist. To achieve the potential of digital health technology in rheumatology, rheumatology professionals will need to be more engaged upstream in the technology design process and provide leadership to effectively incorporate the new tools into clinical care.
10.1038/s41584-020-0461-x
Peeking into a black box, the fairness and generalizability of a MIMIC-III benchmarking model.
Scientific data
As artificial intelligence (AI) makes continuous progress to improve quality of care for some patients by leveraging ever increasing amounts of digital health data, others are left behind. Empirical evaluation studies are required to keep biased AI models from reinforcing systemic health disparities faced by minority populations through dangerous feedback loops. The aim of this study is to raise broad awareness of the pervasive challenges around bias and fairness in risk prediction models. We performed a case study on a MIMIC-trained benchmarking model using a broadly applicable fairness and generalizability assessment framework. While open-science benchmarks are crucial to overcome many study limitations today, this case study revealed a strong class imbalance problem as well as fairness concerns for Black and publicly insured ICU patients. Therefore, we advocate for the widespread use of comprehensive fairness and performance assessment frameworks to effectively monitor and validate benchmark pipelines built on open data resources.
10.1038/s41597-021-01110-7
Evaluating eligibility criteria of oncology trials using real-world data and AI.
Nature
There is a growing focus on making clinical trials more inclusive but the design of trial eligibility criteria remains challenging. Here we systematically evaluate the effect of different eligibility criteria on cancer trial populations and outcomes with real-world data using the computational framework of Trial Pathfinder. We apply Trial Pathfinder to emulate completed trials of advanced non-small-cell lung cancer using data from a nationwide database of electronic health records comprising 61,094 patients with advanced non-small-cell lung cancer. Our analyses reveal that many common criteria, including exclusions based on several laboratory values, had a minimal effect on the trial hazard ratios. When we used a data-driven approach to broaden restrictive criteria, the pool of eligible patients more than doubled on average and the hazard ratio of the overall survival decreased by an average of 0.05. This suggests that many patients who were not eligible under the original trial criteria could potentially benefit from the treatments. We further support our findings through analyses of other types of cancer and patient-safety data from diverse clinical trials. Our data-driven methodology for evaluating eligibility criteria can facilitate the design of more-inclusive trials while maintaining safeguards for patient safety.
10.1038/s41586-021-03430-5
Studying patterns and predictors of HIV viral suppression using A Big Data approach: a research protocol.
Zhang Jiajia,Olatosi Bankole,Yang Xueying,Weissman Sharon,Li Zhenlong,Hu Jianjun,Li Xiaoming
BMC infectious diseases
BACKGROUND:Given the importance of viral suppression in ending the HIV epidemic in the US and elsewhere, an optimal predictive model of viral status can help clinicians identify those at risk of poor viral control and inform clinical improvements in HIV treatment and care. With an increasing availability of electronic health record (EHR) data and social environmental information, there is a unique opportunity to improve our understanding of the dynamic pattern of viral suppression. Using a statewide cohort of people living with HIV (PLWH) in South Carolina (SC), the overall goal of the proposed research is to examine the dynamic patterns of viral suppression, develop optimal predictive models of various viral suppression indicators, and translate the models to a beta version of service-ready tools for clinical decision support. METHODS:The PLWH cohort will be identified through the SC Enhanced HIV/AIDS Reporting System (eHARS). The SC Office of Revenue and Fiscal Affairs (RFA) will extract longitudinal EHR clinical data of all PLWH in SC from multiple health systems, obtain data from other state agencies, and link the patient-level data with county-level data from multiple publicly available data sources. Using the deidentified data, the proposed study will consist of three operational phases: Phase 1: "Pattern Analysis" to identify the longitudinal dynamics of viral suppression using multiple viral load indicators; Phase 2: "Model Development" to determine the critical predictors of multiple viral load indicators through artificial intelligence (AI)-based modeling accounting for multilevel factors; and Phase 3: "Translational Research" to develop a multifactorial clinical decision system based on a risk prediction model to assist with the identification of the risk of viral failure or viral rebound when patients present at clinical visits. DISCUSSION:With both extensive data integration and data analytics, the proposed research will: (1) improve the understanding of the complex inter-related effects of longitudinal trajectories of HIV viral suppressions and HIV treatment history while taking into consideration multilevel factors; and (2) develop empirical public health approaches to achieve ending the HIV epidemic through translating the risk prediction model to a multifactorial decision system that enables the feasibility of AI-assisted clinical decisions.
10.1186/s12879-022-07047-5
Bagged random causal networks for interventional queries on observational biomedical datasets.
Prosperi Mattia,Guo Yi,Bian Jiang
Journal of biomedical informatics
Learning causal effects from observational data, e.g. estimating the effect of a treatment on survival by data-mining electronic health records (EHRs), can be biased due to unmeasured confounders, mediators, and colliders. When the causal dependencies among features/covariates are expressed in the form of a directed acyclic graph, using do-calculus it is possible to identify one or more adjustment sets for eliminating the bias on a given causal query under certain assumptions. However, prior knowledge of the causal structure might be only partial; algorithms for causal structure discovery often provide ambiguous solutions, and their computational complexity becomes practically intractable when the feature sets grow large. We hypothesize that the estimation of the true causal effect of a causal query on to an outcome can be approximated as an ensemble of lower complexity estimators, namely bagged random causal networks. A bagged random causal network is an ensemble of subnetworks constructed by sampling the feature subspaces (with the query, the outcome, and a random number of other features), drawing conditional dependencies among the features, and inferring the corresponding adjustment sets. The causal effect can be then estimated by any regression function of the outcome by the query paired with the adjustment sets. Through simulations and a real-world clinical dataset (class III malocclusion data), we show that the bagged estimator is -in most cases- consistent with the true causal effect if the structure is known, has a good variance/bias trade-off when the structure is unknown (estimated using heuristics), has lower computational complexity than learning a full network, and outperforms boosted regression. In conclusion, the bagged random causal network is well-suited to estimate query-target causal effects from observational studies on EHR and other high-dimensional biomedical databases.
10.1016/j.jbi.2021.103689
Extracting comprehensive clinical information for breast cancer using deep learning methods.
Zhang Xiaohui,Zhang Yaoyun,Zhang Qin,Ren Yuankai,Qiu Tinglin,Ma Jianhui,Sun Qiang
International journal of medical informatics
OBJECTIVE:Breast cancer is the most common malignant tumor among women. The diagnosis and treatment information of breast cancer patients is abundant in multiple types of clinical fields, including clinicopathological data, genotype and phenotype information, treatment information, and prognosis information. However, current studies are mainly focused on extracting information from one specific type of clinical field. This study defines a comprehensive information model to represent the whole-course clinical information of patients. Furthermore, deep learning approaches are used to extract the concepts and their attributes from clinical breast cancer documents by fine-tuning pretrained Bidirectional Encoder Representations from Transformers (BERT) language models. MATERIALS AND METHODS:The clinical corpus that was used in this study was from one 3A cancer hospital in China, consisting of the encounter notes, operation records, pathology notes, radiology notes, progress notes and discharge summaries of 100 breast cancer patients. Our system consists of two components: a named entity recognition (NER) component and a relation recognition component. For each component, we implemented deep learning-based approaches by fine-tuning BERT, which outperformed other state-of-the-art methods on multiple natural language processing (NLP) tasks. A clinical language model is first pretrained using BERT on a large-scale unlabeled corpus of Chinese clinical text. For NER, the context embeddings that were pretrained using BERT were used as the input features of the Bi-LSTM-CRF (Bidirectional long-short-memory-conditional random fields) model and were fine-tuned using the annotated breast cancer notes. Furthermore, we proposed an approach to fine-tune BERT for relation extraction. It was considered to be a classification problem in which the two entities that were mentioned in the input sentence were replaced with their semantic types. RESULTS:Our best-performing system achieved F1 scores of 93.53% for the NER and 96.73% for the relation extraction. Additional evaluations showed that the deep learning-based approaches that fine-tuned BERT did outperform the traditional Bi-LSTM-CRF and CRF machine learning algorithms in NER and the attention-Bi-LSTM and SVM (support vector machines) algorithms in relation recognition. CONCLUSION:In this study, we developed a deep learning approach that fine-tuned BERT to extract the breast cancer concepts and their attributes. It demonstrated its superior performance compared to traditional machine learning algorithms, thus supporting its uses in broader NER and relation extraction tasks in the medical domain.
10.1016/j.ijmedinf.2019.103985
Classifying social determinants of health from unstructured electronic health records using deep learning-based natural language processing.
Journal of biomedical informatics
OBJECTIVE:Social determinants of health (SDOH) are non-medical factors that can profoundly impact patient health outcomes. However, SDOH are rarely available in structured electronic health record (EHR) data such as diagnosis codes, and more commonly found in unstructured narrative clinical notes. Hence, identifying social context from unstructured EHR data has become increasingly important. Yet, previous work on using natural language processing to automate extraction of SDOH from text (a) usually focuses on an ad hoc selection of SDOH, and (b) does not use the latest advances in deep learning. Our objective was to advance automatic extraction of SDOH from clinical text by (a) systematically creating a set of SDOH based on standard biomedical and psychiatric ontologies, and (b) training state-of-the-art deep neural networks to extract mentions of these SDOH from clinical notes. DESIGN:A retrospective cohort study. SETTING AND PARTICIPANTS:Data were extracted from the Medical Information Mart for Intensive Care (MIMIC-III) database. The corpus comprised 3,504 social related sentences from 2,670 clinical notes. METHODS:We developed a framework for automated classification of multiple SDOH categories. Our dataset comprised narrative clinical notes under the "Social Work" category in the MIMIC-III Clinical Database. Using standard terminologies, SNOMED-CT and DSM-IV, we systematically curated a set of 13 SDOH categories and created annotation guidelines for these. After manually annotating the 3,504 sentences, we developed and tested three deep neural network (DNN) architectures - convolutional neural network (CNN), long short-term memory (LSTM) network, and the Bidirectional Encoder Representations from Transformers (BERT) - for automated detection of eight SDOH categories. We also compared these DNNs to three baselines models: (1) cTAKES, as well as (2) L2-regularized logistic regression and (3) random forests on bags-of-words. Model evaluation metrics included micro- and macro- F1, and area under the receiver operating characteristic curve (AUC). RESULTS:All three DNN models accurately classified all SDOH categories (minimum micro-F1 = 0.632, minimum macro-AUC = 0.854). Compared to the CNN and LSTM, BERT performed best in most key metrics (micro-F1 = 0.690, macro-AUC = 0.907). The BERT model most effectively identified the "occupational" category (F1 = 0.774, AUC = 0.965) and least effectively identified the "non-SDOH" category (F = 0.491, AUC = 0.788). BERT outperformed cTAKES in distinguishing social vs non-social sentences (BERT F1 = 0.87 vs. cTAKES F1 = 0.06), and outperformed logistic regression (micro-F1 = 0.649, macro-AUC = 0.696) and random forest (micro-F1 = 0.502, macro-AUC = 0.523) trained on bag-of-words. CONCLUSIONS:Our study framework with DNN models demonstrated improved performance for efficiently identifying a systematic range of SDOH categories from clinical notes in the EHR. Improved identification of patient SDOH may further improve healthcare outcomes.
10.1016/j.jbi.2021.103984
Identification of asthma control factor in clinical notes using a hybrid deep learning model.
BMC medical informatics and decision making
BACKGROUND:There are significant variabilities in guideline-concordant documentation in asthma care. However, assessing clinician's documentation is not feasible using only structured data but requires labor-intensive chart review of electronic health records (EHRs). A certain guideline element in asthma control factors, such as review inhaler techniques, requires context understanding to correctly capture from EHR free text. METHODS:The study data consist of two sets: (1) manual chart reviewed data-1039 clinical notes of 300 patients with asthma diagnosis, and (2) weakly labeled data (distant supervision)-27,363 clinical notes from 800 patients with asthma diagnosis. A context-aware language model, Bidirectional Encoder Representations from Transformers (BERT) was developed to identify inhaler techniques in EHR free text. Both original BERT and clinical BioBERT (cBERT) were applied with a cost-sensitivity to deal with imbalanced data. The distant supervision using weak labels by rules was also incorporated to augment the training set and alleviate a costly manual labeling process in the development of a deep learning algorithm. A hybrid approach using post-hoc rules was also explored to fix BERT model errors. The performance of BERT with/without distant supervision, hybrid, and rule-based models were compared in precision, recall, F-score, and accuracy. RESULTS:The BERT models on the original data performed similar to a rule-based model in F1-score (0.837, 0.845, and 0.838 for rules, BERT, and cBERT, respectively). The BERT models with distant supervision produced higher performance (0.853 and 0.880 for BERT and cBERT, respectively) than without distant supervision and a rule-based model. The hybrid models performed best in F1-score of 0.877 and 0.904 over the distant supervision on BERT and cBERT. CONCLUSIONS:The proposed BERT models with distant supervision demonstrated its capability to identify inhaler techniques in EHR free text, and outperformed both the rule-based model and BERT models trained on the original data. With a distant supervision approach, we may alleviate costly manual chart review to generate the large training data required in most deep learning-based models. A hybrid model was able to fix BERT model errors and further improve the performance.
10.1186/s12911-021-01633-4
Using Electronic Health Records for Population Health Research: A Review of Methods and Applications.
Casey Joan A,Schwartz Brian S,Stewart Walter F,Adler Nancy E
Annual review of public health
The use and functionality of electronic health records (EHRs) have increased rapidly in the past decade. Although the primary purpose of EHRs is clinical, researchers have used them to conduct epidemiologic investigations, ranging from cross-sectional studies within a given hospital to longitudinal studies on geographically distributed patients. Herein, we describe EHRs, examine their use in population health research, and compare them with traditional epidemiologic methods. We describe diverse research applications that benefit from the large sample sizes and generalizable patient populations afforded by EHRs. These have included reevaluation of prior findings, a range of diseases and subgroups, environmental and social epidemiology, stigmatized conditions, predictive modeling, and evaluation of natural experiments. Although studies using primary data collection methods may have more reliable data and better population retention, EHR-based studies are less expensive and require less time to complete. Future EHR epidemiology with enhanced collection of social/behavior measures, linkage with vital records, and integration of emerging technologies such as personal sensing could improve clinical care and population health.
10.1146/annurev-publhealth-032315-021353
Measures of SES for Electronic Health Record-based Research.
American journal of preventive medicine
INTRODUCTION:Although infrequently recorded in electronic health records (EHRs), measures of SES are essential to describe health inequalities and account for confounding in epidemiologic research. Medical Assistance (i.e., Medicaid) is often used as a surrogate for SES, but correspondence between conventional SES and Medical Assistance has been insufficiently studied. METHODS:Geisinger Clinic EHR data from 2001 to 2014 and a 2014 questionnaire were used to create six SES measures: EHR-derived Medical Assistance and proportion of time under observation on Medical Assistance; educational attainment, income, and marital status; and area-level poverty. Analyzed in 2016-2017, associations of SES measures with obesity, hypertension, type 2 diabetes, chronic rhinosinusitis, fatigue, and migraine headache were assessed using weighted age- and sex-adjusted logistic regression. RESULTS:Among 5,550 participants (interquartile range, 39.6-57.5 years, 65.9% female), 83% never used Medical Assistance. All SES measures were correlated (Spearman's p≤0.4). Medical Assistance was significantly associated with all six health outcomes in adjusted models. For example, the OR for prevalent type 2 diabetes associated with Medical Assistance was 1.7 (95% CI=1.3, 2.2); the OR for high school versus college graduates was 1.7 (95% CI=1.2, 2.5). Medical Assistance was an imperfect proxy for SES: associations between conventional SES measures and health were attenuated <20% after adjustment for Medical Assistance. CONCLUSIONS:Because systematically collected SES measures are rarely available in EHRs and are unlikely to appear soon, researchers can use EHR-based Medical Assistance to describe inequalities. As SES has many domains, researchers who use Medical Assistance to evaluate the association of SES with health should expect substantial unmeasured confounding.
10.1016/j.amepre.2017.10.004
Application of Artificial Intelligence in Community-Based Primary Health Care: Systematic Scoping Review and Critical Appraisal.
Abbasgholizadeh Rahimi Samira,Légaré France,Sharma Gauri,Archambault Patrick,Zomahoun Herve Tchala Vignon,Chandavong Sam,Rheault Nathalie,T Wong Sabrina,Langlois Lyse,Couturier Yves,Salmeron Jose L,Gagnon Marie-Pierre,Légaré Jean
Journal of medical Internet research
BACKGROUND:Research on the integration of artificial intelligence (AI) into community-based primary health care (CBPHC) has highlighted several advantages and disadvantages in practice regarding, for example, facilitating diagnosis and disease management, as well as doubts concerning the unintended harmful effects of this integration. However, there is a lack of evidence about a comprehensive knowledge synthesis that could shed light on AI systems tested or implemented in CBPHC. OBJECTIVE:We intended to identify and evaluate published studies that have tested or implemented AI in CBPHC settings. METHODS:We conducted a systematic scoping review informed by an earlier study and the Joanna Briggs Institute (JBI) scoping review framework and reported the findings according to PRISMA-ScR (Preferred Reporting Items for Systematic Reviews and Meta-Analysis-Scoping Reviews) reporting guidelines. An information specialist performed a comprehensive search from the date of inception until February 2020, in seven bibliographic databases: Cochrane Library, MEDLINE, EMBASE, Web of Science, Cumulative Index to Nursing and Allied Health Literature (CINAHL), ScienceDirect, and IEEE Xplore. The selected studies considered all populations who provide and receive care in CBPHC settings, AI interventions that had been implemented, tested, or both, and assessed outcomes related to patients, health care providers, or CBPHC systems. Risk of bias was assessed using the Prediction Model Risk of Bias Assessment Tool (PROBAST). Two authors independently screened the titles and abstracts of the identified records, read the selected full texts, and extracted data from the included studies using a validated extraction form. Disagreements were resolved by consensus, and if this was not possible, the opinion of a third reviewer was sought. A third reviewer also validated all the extracted data. RESULTS:We retrieved 22,113 documents. After the removal of duplicates, 16,870 documents were screened, and 90 peer-reviewed publications met our inclusion criteria. Machine learning (ML) (41/90, 45%), natural language processing (NLP) (24/90, 27%), and expert systems (17/90, 19%) were the most commonly studied AI interventions. These were primarily implemented for diagnosis, detection, or surveillance purposes. Neural networks (ie, convolutional neural networks and abductive networks) demonstrated the highest accuracy, considering the given database for the given clinical task. The risk of bias in diagnosis or prognosis studies was the lowest in the participant category (4/49, 4%) and the highest in the outcome category (22/49, 45%). CONCLUSIONS:We observed variabilities in reporting the participants, types of AI methods, analyses, and outcomes, and highlighted the large gap in the effective development and implementation of AI in CBPHC. Further studies are needed to efficiently guide the development and implementation of AI interventions in CBPHC settings.
10.2196/29839
Integrating pharmacogenomics into electronic health records with clinical decision support.
Hicks J Kevin,Dunnenberger Henry M,Gumpper Karl F,Haidar Cyrine E,Hoffman James M
American journal of health-system pharmacy : AJHP : official journal of the American Society of Health-System Pharmacists
PURPOSE:Existing pharmacogenomic informatics models, key implementation steps, and emerging resources to facilitate the development of pharmacogenomic clinical decision support (CDS) are described. SUMMARY:Pharmacogenomics is an important component of precision medicine. Informatics, especially CDS in the electronic health record (EHR), is a critical tool for the integration of pharmacogenomics into routine patient care. Effective integration of pharmacogenomic CDS into the EHR can address implementation challenges, including the increasing volume of pharmacogenomic clinical knowledge, the enduring nature of pharmacogenomic test results, and the complexity of interpreting results. Both passive and active CDS provide point-of-care information to clinicians that can guide the systematic use of pharmacogenomics to proactively optimize pharmacotherapy. Key considerations for a successful implementation have been identified; these include clinical workflows, identification of alert triggers, and tools to guide interpretation of results. These considerations, along with emerging resources from the Clinical Pharmacogenetics Implementation Consortium and the National Academy of Medicine, are described. CONCLUSION:The EHR with CDS is essential to curate pharmacogenomic data and disseminate patient-specific information at the point of care. As part of the successful implementation of pharmacogenomics into clinical settings, all relevant clinical recommendations pertaining to gene-drug pairs must be summarized and presented to clinicians in a manner that is seamlessly integrated into the clinical workflow of the EHR. In some situations, ancillary systems and applications outside the EHR may be integrated to augment the capabilities of the EHR.
10.2146/ajhp160030
How the electronic health record will change the future of health care.
Ronquillo Jeremiah Geronimo
The Yale journal of biology and medicine
Genetic testing is expected to play a critical role in patient care in the near future. Advances in genomic research have the potential to impact medicine in very tangible and direct ways, from carrier screening to disease diagnosis and prognosis to targeted treatments and personalized medicine. However, numerous barriers to widespread adoption of genetic testing continue to exist, and health information technology will be a critical means of addressing these challenges. Electronic health records (EHRs) are a digital replacement for the traditional paper-based patient chart designed to improve the quality of patient care. EHRs have become increasingly essential to managing the wealth of existing clinical information that now includes genetic information extracted from the patient genome. The EHR is capable of changing health care in the future by transforming the way physicians use genomic information in the practice of medicine.
Integrated precision medicine: the role of electronic health records in delivering personalized treatment.
Sitapati Amy,Kim Hyeoneui,Berkovich Barbara,Marmor Rebecca,Singh Siddharth,El-Kareh Robert,Clay Brian,Ohno-Machado Lucila
Wiley interdisciplinary reviews. Systems biology and medicine
Precision Medicine involves the delivery of a targeted, personalized treatment for a given patient. By harnessing the power of electronic health records (EHRs), we are increasingly able to practice precision medicine to improve patient outcomes. In this article, we introduce the scientific community at large to important building blocks for personalized treatment, such as terminology standards that are the foundation of the EHR and allow for exchange of health information across systems. We briefly review different types of clinical decision support (CDS) and present the current state of CDS, which is already improving the care patients receive with genetic profile-based tailored recommendations regarding diagnostic and treatment plans. We also report on limitations of current systems, which are slowly beginning to integrate new genomic data into patient records but still present many challenges. Finally, we discuss future directions and how the EHR can evolve to increase the capacity of the healthcare system in delivering Precision Medicine at the point of care. WIREs Syst Biol Med 2017, 9:e1378. doi: 10.1002/wsbm.1378 For further resources related to this article, please visit the WIREs website.
10.1002/wsbm.1378
Improving blood pressure control among adults with CKD and diabetes: provider-focused quality improvement using electronic health records.
Thomas Bernadette
Advances in chronic kidney disease
Current evidence demonstrates poor provider knowledge and compliance to clinical practice guidelines (CPGs) for CKD screening, blood pressure (BP) goals specific to people with diabetes mellitus (DM) and CKD, and underutilization or incorrect drug selection for antihypertensive therapy. This 12-week provider-focused quality improvement project sought to (1) increase primary care provider (PCP) adherence to CPG in the treatment and control of BP among adults with CKD and DM by using electronic health records (EHRs) and patient-level feedback (scorecards); (2) increase PCP delivery of basic CKD patient education by using EHR-based decision support; and (3) assess whether electronic decision support and scorecards changed provider behavior. The project included 46 PCPs, physicians, and nurse practitioners, in a statewide federally qualified health center that operates 12 comprehensive primary care sites in Connecticut. There were 6781 DM visits, among 3137 unique, racially diverse patients. There was a statistically significant increase in CKD screening, diagnosis, and use of angiotensin-converting enzyme inhibitor/angiotensin-receptor blocker. There was a statistically, but not clinically, significant increase in CKD basic education and ancillary service provider use when the provider was aware of the diagnosis or used EHR enhancements. EHR decision support and real-time provider feedback are necessary but not sufficient to improve uptake of CPG and to change PCP behavior.
10.1053/j.ackd.2011.10.005
Identifying Symptom Information in Clinical Notes Using Natural Language Processing.
Nursing research
BACKGROUND:Symptoms are a core concept of nursing interest. Large-scale secondary data reuse of notes in electronic health records (EHRs) has the potential to increase the quantity and quality of symptom research. However, the symptom language used in clinical notes is complex. A need exists for methods designed specifically to identify and study symptom information from EHR notes. OBJECTIVES:We aim to describe a method that combines standardized vocabularies, clinical expertise, and natural language processing to generate comprehensive symptom vocabularies and identify symptom information in EHR notes. We piloted this method with five diverse symptom concepts: constipation, depressed mood, disturbed sleep, fatigue, and palpitations. METHODS:First, we obtained synonym lists for each pilot symptom concept from the Unified Medical Language System. Then, we used two large bodies of text (clinical notes from Columbia University Irving Medical Center and PubMed abstracts containing Medical Subject Headings or key words related to the pilot symptoms) to further expand our initial vocabulary of synonyms for each pilot symptom concept. We used NimbleMiner, an open-source natural language processing tool, to accomplish these tasks and evaluated NimbleMiner symptom identification performance by comparison to a manually annotated set of nurse- and physician-authored common EHR note types. RESULTS:Compared to the baseline Unified Medical Language System synonym lists, we identified up to 11 times more additional synonym words or expressions, including abbreviations, misspellings, and unique multiword combinations, for each symptom concept. Natural language processing system symptom identification performance was excellent. DISCUSSION:Using our comprehensive symptom vocabularies and NimbleMiner to label symptoms in clinical notes produced excellent performance metrics. The ability to extract symptom information from EHR notes in an accurate and scalable manner has the potential to greatly facilitate symptom science research.
10.1097/NNR.0000000000000488
Natural language processing of symptoms documented in free-text narratives of electronic health records: a systematic review.
Koleck Theresa A,Dreisbach Caitlin,Bourne Philip E,Bakken Suzanne
Journal of the American Medical Informatics Association : JAMIA
OBJECTIVE:Natural language processing (NLP) of symptoms from electronic health records (EHRs) could contribute to the advancement of symptom science. We aim to synthesize the literature on the use of NLP to process or analyze symptom information documented in EHR free-text narratives. MATERIALS AND METHODS:Our search of 1964 records from PubMed and EMBASE was narrowed to 27 eligible articles. Data related to the purpose, free-text corpus, patients, symptoms, NLP methodology, evaluation metrics, and quality indicators were extracted for each study. RESULTS:Symptom-related information was presented as a primary outcome in 14 studies. EHR narratives represented various inpatient and outpatient clinical specialties, with general, cardiology, and mental health occurring most frequently. Studies encompassed a wide variety of symptoms, including shortness of breath, pain, nausea, dizziness, disturbed sleep, constipation, and depressed mood. NLP approaches included previously developed NLP tools, classification methods, and manually curated rule-based processing. Only one-third (n = 9) of studies reported patient demographic characteristics. DISCUSSION:NLP is used to extract information from EHR free-text narratives written by a variety of healthcare providers on an expansive range of symptoms across diverse clinical specialties. The current focus of this field is on the development of methods to extract symptom information and the use of symptom information for disease classification tasks rather than the examination of symptoms themselves. CONCLUSION:Future NLP studies should concentrate on the investigation of symptoms and symptom documentation in EHR free-text narratives. Efforts should be undertaken to examine patient characteristics and make symptom-related NLP algorithms or pipelines and vocabularies openly available.
10.1093/jamia/ocy173
PASCLex: A comprehensive post-acute sequelae of COVID-19 (PASC) symptom lexicon derived from electronic health record clinical notes.
Journal of biomedical informatics
OBJECTIVE:To develop a comprehensive post-acute sequelae of COVID-19 (PASC) symptom lexicon (PASCLex) from clinical notes to support PASC symptom identification and research. METHODS:We identified 26,117 COVID-19 positive patients from the Mass General Brigham's electronic health records (EHR) and extracted 328,879 clinical notes from their post-acute infection period (day 51-110 from first positive COVID-19 test). PASCLex incorporated Unified Medical Language System® (UMLS) Metathesaurus concepts and synonyms based on selected semantic types. The MTERMS natural language processing (NLP) tool was used to automatically extract symptoms from a development dataset. The lexicon was iteratively revised with manual chart review, keyword search, concept consolidation, and evaluation of NLP output. We assessed the comprehensiveness of PASCLex and the NLP performance using a validation dataset and reported the symptom prevalence across the entire corpus. RESULTS:PASCLex included 355 symptoms consolidated from 1520 UMLS concepts of 16,466 synonyms. NLP achieved an averaged precision of 0.94 and an estimated recall of 0.84. Symptoms with the highest frequency included pain (43.1%), anxiety (25.8%), depression (24.0%), fatigue (23.4%), joint pain (21.0%), shortness of breath (20.8%), headache (20.0%), nausea and/or vomiting (19.9%), myalgia (19.0%), and gastroesophageal reflux (18.6%). DISCUSSION AND CONCLUSION:PASC symptoms are diverse. A comprehensive lexicon of PASC symptoms can be derived using an ontology-driven, EHR-guided and NLP-assisted approach. By using unstructured data, this approach may improve identification and analysis of patient symptoms in the EHR, and inform prospective study design, preventative care strategies, and therapeutic interventions for patient care.
10.1016/j.jbi.2021.103951
Machine Learning, Natural Language Processing, and the Electronic Health Record: Innovations in Mental Health Services Research.
Edgcomb Juliet Beni,Zima Bonnie
Psychiatric services (Washington, D.C.)
An unprecedented amount of clinical information is now available via electronic health records (EHRs). These massive data sets have stimulated opportunities to adapt computational approaches to track and identify target areas for quality improvement in mental health care. In this column, three key areas of EHR data science are described: EHR phenotyping, natural language processing, and predictive modeling. For each of these computational approaches, case examples are provided to illustrate their role in mental health services research. Together, adaptation of these methods underscores the need for standardization and transparency while recognizing the opportunities and challenges ahead.
10.1176/appi.ps.201800401
Artificial intelligence approaches using natural language processing to advance EHR-based clinical research.
The Journal of allergy and clinical immunology
The wide adoption of electronic health record systems in health care generates big real-world data that open new venues to conduct clinical research. As a large amount of valuable clinical information is locked in clinical narratives, natural language processing techniques as an artificial intelligence approach have been leveraged to extract information from clinical narratives in electronic health records. This capability of natural language processing potentially enables automated chart review for identifying patients with distinctive clinical characteristics in clinical care and reduces methodological heterogeneity in defining phenotype, obscuring biological heterogeneity in research concerning allergy, asthma, and immunology. This brief review discusses the current literature on the secondary use of electronic health record data for clinical research concerning allergy, asthma, and immunology and highlights the potential, challenges, and implications of natural language processing techniques.
10.1016/j.jaci.2019.12.897
Large Health System Databases and Drug Hypersensitivity.
Chiriac Anca Mirela,Macy Eric
The journal of allergy and clinical immunology. In practice
Large health system databases have revolutionized our understanding of the epidemiology of adverse drug reactions and immunologically mediated drug hypersensitivity. Population-based background rates of newly reported drug intolerance with each therapeutic exposure could not have been determined without large health system databases. Large databases have increased our understanding of multiple drug intolerance syndrome. Large health care systems, such as Kaiser Permanente, with a single electronic medical record system that covers all inpatient, outpatient, and pharmacy interactions, are particularly valuable in understanding the population-based incidence of severe and nonsevere adverse drug reactions, the risks of delayed-onset adverse drug reactions, such as those caused by Clostridiodes difficile, which can occur months after antibiotic exposures, and the risks and benefits associated with "allergy" delabeling, specifically penicillin allergy delabeling, which may accrue in the years after the delabeling. There currently are limitations to using electronic data, specifically billing code data, when studying adverse drug reactions. It is critical to audit electronic health records, which have temporally associated the use of a drug and an adverse reaction because of high rates of miscoding or lack of true cause and effect. Pending improvements in drug hypersensitivity coding in International Classification of Diseases, Eleventh Revision may make large databases even more useful.
10.1016/j.jaip.2019.04.014
Drug-Induced Anaphylaxis Documented in Electronic Health Records.
Dhopeshwarkar Neil,Sheikh Aziz,Doan Raymond,Topaz Maxim,Bates David W,Blumenthal Kimberly G,Zhou Li
The journal of allergy and clinical immunology. In practice
BACKGROUND:Although drugs represent a common cause of anaphylaxis, few large studies of drug-induced anaphylaxis have been performed. OBJECTIVE:To describe the epidemiology and validity of reported drug-induced anaphylaxis in the electronic health records (EHRs) of a large United States health care system. METHODS:Using EHR drug allergy data from 1995 to 2013, we determined the population prevalence of anaphylaxis including anaphylaxis prevalence over time, and the most commonly implicated drugs/drug classes reported to cause anaphylaxis. Patient risk factors for drug-induced anaphylaxis were assessed using a logistic regression model. Serum tryptase and allergist visits were used to assess the validity and follow-up of EHR-reported anaphylaxis. RESULTS:Among 1,756,481 patients, 19,836 (1.1%) reported drug-induced anaphylaxis; penicillins (45.9 per 10,000), sulfonamide antibiotics (15.1 per 10,000), and nonsteroidal anti-inflammatory drugs (NSAIDs) (13.0 per 10,000) were most commonly implicated. Patients with white race (odds ratio [OR] 2.38, 95% CI 2.27-2.49), female sex (OR 2.20, 95% CI 2.13-2.28), systemic mastocytosis (OR 4.60, 95% CI 2.66-7.94), Sjögren's syndrome (OR 1.94, 95% CI 1.47-2.56), and asthma (OR 1.50, 95% CI 1.43-1.59) had an increased odds of drug-induced anaphylaxis. Serum tryptase was performed in 135 (<1%) anaphylaxis cases and 1,587 patients (8.0%) saw an allergist for follow-up. CONCLUSIONS:EHR-reported anaphylaxis occurred in approximately 1% of patients, most commonly from penicillins, sulfonamide antibiotics, and NSAIDs. Females, whites, and patients with mastocytosis, Sjögren's syndrome, and asthma had increased odds of reporting drug-induced anaphylaxis. The low observed frequency of tryptase testing and specialist evaluation emphasize the importance of educating providers on anaphylaxis management.
10.1016/j.jaip.2018.06.010
The Next Frontier in Pediatric Cardiology: Artificial Intelligence.
Gaffar Sharib,Gearhart Addison S,Chang Anthony C
Pediatric clinics of North America
Artificial intelligence (AI) in the last decade centered primarily around digitizing and incorporating the large volumes of patient data from electronic health records. AI is now poised to make the next step in health care integration, with precision medicine, imaging support, and development of individual health trends with the popularization of wearable devices. Future clinical pediatric cardiologists will use AI as an adjunct in delivering optimum patient care, with the help of accurate predictive risk calculators, continual health monitoring from wearables, and precision medicine. Physicians must also protect their patients' health information from monetization or exploitation.
10.1016/j.pcl.2020.06.010
Artificial Intelligence in Medical Practice: The Question to the Answer?
Miller D Douglas,Brown Eric W
The American journal of medicine
Computer science advances and ultra-fast computing speeds find artificial intelligence (AI) broadly benefitting modern society-forecasting weather, recognizing faces, detecting fraud, and deciphering genomics. AI's future role in medical practice remains an unanswered question. Machines (computers) learn to detect patterns not decipherable using biostatistics by processing massive datasets (big data) through layered mathematical models (algorithms). Correcting algorithm mistakes (training) adds to AI predictive model confidence. AI is being successfully applied for image analysis in radiology, pathology, and dermatology, with diagnostic speed exceeding, and accuracy paralleling, medical experts. While diagnostic confidence never reaches 100%, combining machines plus physicians reliably enhances system performance. Cognitive programs are impacting medical practice by applying natural language processing to read the rapidly expanding scientific literature and collate years of diverse electronic medical records. In this and other ways, AI may optimize the care trajectory of chronic disease patients, suggest precision therapies for complex illnesses, reduce medical errors, and improve subject enrollment into clinical trials.
10.1016/j.amjmed.2017.10.035
Artificial intelligence in precision medicine in hepatology.
Su Tung-Hung,Wu Chih-Horng,Kao Jia-Horng
Journal of gastroenterology and hepatology
The advancement of investigation tools and electronic health records (EHR) enables a paradigm shift from guideline-specific therapy toward patient-specific precision medicine. The multiparametric and large detailed information necessitates novel analyses to explore the insight of diseases and to aid the diagnosis, monitoring, and outcome prediction. Artificial intelligence (AI), machine learning, and deep learning (DL) provide various models of supervised, or unsupervised algorithms, and sophisticated neural networks to generate predictive models more precisely than conventional ones. The data, application tasks, and algorithms are three key components in AI. Various data formats are available in daily clinical practice of hepatology, including radiological imaging, EHR, liver pathology, data from wearable devices, and multi-omics measurements. The images of abdominal ultrasonography, computed tomography, and magnetic resonance imaging can be used to predict liver fibrosis, cirrhosis, non-alcoholic fatty liver disease (NAFLD), and differentiation of benign tumors from hepatocellular carcinoma (HCC). Using EHR, the AI algorithms help predict the diagnosis and outcomes of liver cirrhosis, HCC, NAFLD, portal hypertension, varices, liver transplantation, and acute liver failure. AI helps to predict severity and patterns of fibrosis, steatosis, activity of NAFLD, and survival of HCC by using pathological data. Despite of these high potentials of AI application, data preparation, collection, quality, labeling, and sampling biases of data are major concerns. The selection, evaluation, and validation of algorithms, as well as real-world application of these AI models, are also challenging. Nevertheless, AI opens the new era of precision medicine in hepatology, which will change our future practice.
10.1111/jgh.15415
Artificial intelligence in medicine and cardiac imaging: harnessing big data and advanced computing to provide personalized medical diagnosis and treatment.
Dilsizian Steven E,Siegel Eliot L
Current cardiology reports
Although advances in information technology in the past decade have come in quantum leaps in nearly every aspect of our lives, they seem to be coming at a slower pace in the field of medicine. However, the implementation of electronic health records (EHR) in hospitals is increasing rapidly, accelerated by the meaningful use initiatives associated with the Center for Medicare & Medicaid Services EHR Incentive Programs. The transition to electronic medical records and availability of patient data has been associated with increases in the volume and complexity of patient information, as well as an increase in medical alerts, with resulting "alert fatigue" and increased expectations for rapid and accurate diagnosis and treatment. Unfortunately, these increased demands on health care providers create greater risk for diagnostic and therapeutic errors. In the near future, artificial intelligence (AI)/machine learning will likely assist physicians with differential diagnosis of disease, treatment options suggestions, and recommendations, and, in the case of medical imaging, with cues in image interpretation. Mining and advanced analysis of "big data" in health care provide the potential not only to perform "in silico" research but also to provide "real time" diagnostic and (potentially) therapeutic recommendations based on empirical data. "On demand" access to high-performance computing and large health care databases will support and sustain our ability to achieve personalized medicine. The IBM Jeopardy! Challenge, which pitted the best all-time human players against the Watson computer, captured the imagination of millions of people across the world and demonstrated the potential to apply AI approaches to a wide variety of subject matter, including medicine. The combination of AI, big data, and massively parallel computing offers the potential to create a revolutionary way of practicing evidence-based, personalized medicine.
10.1007/s11886-013-0441-8
Technological progress in electronic health record system optimization: Systematic review of systematic literature reviews.
International journal of medical informatics
BACKGROUND:The recent, rapid development of digital technologies offers new possibilities for more efficient implementation of electronic health record (EHR) and personal health record (PHR) systems. A growing volume of healthcare data has been the hallmark of this digital transformation. The large healthcare datasets' complexity and their dynamic nature pose various challenges related to processing, analysis, storage, security, privacy, data exchange, and usability. MATERIALS AND METHODS:We performed a systematic review of systematic reviews to assess technological progress in EHR and PHR systems. We searched MEDLINE, Cochrane, Web of Science, and Scopus for systematic literature reviews on technological advancements that support EHR and PHR systems published between January 1, 2010, and October 06, 2020. RESULTS:The searches resulted in a total of 2,448 hits. Of these, we finally selected 23 systematic reviews. Most of the included papers dealt with information extraction tools and natural language processing technology (n = 10), followed by studies that assessed the use of blockchain technology in healthcare (n = 8). Other areas of digital technology research included EHR and PHR systems in austere settings (n = 1), de-identification methods (n = 1), visualization techniques (n = 1), communication tools within EHR and PHR systems (n = 1), and methodologies for defining Clinical Information Models that promoted EHRs and PHRs interoperability (n = 1). CONCLUSIONS:Technological advancements can improve the efficiency in the implementation of EHR and PHR systems in numerous ways. Natural language processing techniques, either rule-based, machine-learning, or deep learning-based, can extract information from clinical narratives and other unstructured data locked in EHRs and PHRs, allowing secondary research (i.e., phenotyping). Moreover, EHRs and PHRs are expected to be the primary beneficiaries of the blockchain technology implementation on Health Information Systems. Governance regulations, lack of trust, poor scalability, security, privacy, low performance, and high cost remain the most critical challenges for implementing these technologies.
10.1016/j.ijmedinf.2021.104507
The HealthChain Blockchain for Electronic Health Records: Development Study.
Xiao Yonggang,Xu Bin,Jiang Wenhao,Wu Yunjun
Journal of medical Internet research
BACKGROUND:Health care professionals are required to maintain accurate health records of patients. Furthermore, these records should be shared across different health care organizations for professionals to have a complete review of medical history and avoid missing important information. Nowadays, health care providers use electronic health records (EHRs) as a key to the implementation of these goals and delivery of quality care. However, there are technical and legal hurdles that prevent the adoption of these systems, such as concerns about performance and privacy issues. OBJECTIVE:This study aimed to build and evaluate an experimental blockchain for EHRs, named HealthChain, which overcomes the disadvantages of traditional EHR systems. METHODS:HealthChain is built based on consortium blockchain technology. Specifically, three organizations, namely hospitals, insurance providers, and governmental agencies, form a consortium that operates under a governance model, which enforces the business logic agreed by all participants. Every peer node hosts an instance of the distributed ledger consisting of EHRs and an instance of chaincode regulating the permissions of participants. Designated orderers establish consensus on the order of EHRs and then disseminate blocks to peers. RESULTS:HealthChain achieves functional and nonfunctional requirements. It can store EHRs in a distributed ledger and share them among different participants. Moreover, it demonstrates superior features, such as privacy preservation, security, and high throughput. These are the main reasons why HealthChain is proposed. CONCLUSIONS:Consortium blockchain technology can help to build new EHR systems and solve the problems that prevent the adoption of traditional systems.
10.2196/13556
Artificial intelligence and multi agent based distributed ledger system for better privacy and security of electronic healthcare records.
Alruwaili Fahad F
PeerJ. Computer science
Background:Application of Artificial Intelligence (AI) and the use of agent-based systems in the healthcare system have attracted various researchers to improve the efficiency and utility in the Electronic Health Records (EHR). Nowadays, one of the most important and creative developments is the integration of AI and Blockchain that is, Distributed Ledger Technology (DLT) to enable better and decentralized governance. Privacy and security is a critical piece in EHR implementation and/or adoption. Health records are updated every time a patient visits a doctor as they contain important information about the health and wellbeing of the patient and describes the history of care received during the past and to date. Therefore, such records are critical to research, hospitals, emergency rooms, healthcare laboratories, and even health insurance providers. Methods:In this article, a platform employing the AI and the use of multi-agent based systems along with the DLT technology for privacy preservation is proposed. The emphasis of security and privacy is highlighted during the process of collecting, managing and distributing EHR data. Results:This article aims to ensure privacy, integrity and security metrics of the electronic health records are met when such copies are not only immutable but also distributed. The findings of this work will help guide the development of further techniques using the combination of AI and multi-agent based systems backed by DLT technology for secure and effective handling EHR data. This proposed architecture uses various AI-based intelligent based agents and blockchain for providing privacy and security in EHR. Future enhancement in this work can be the addition of the biometric based systems for improved security.
10.7717/peerj-cs.323
Deep learning for electronic health records: A comparative review of multiple deep neural architectures.
Ayala Solares Jose Roberto,Diletta Raimondi Francesca Elisa,Zhu Yajie,Rahimian Fatemeh,Canoy Dexter,Tran Jenny,Pinho Gomes Ana Catarina,Payberah Amir H,Zottoli Mariagrazia,Nazarzadeh Milad,Conrad Nathalie,Rahimi Kazem,Salimi-Khorshidi Gholamreza
Journal of biomedical informatics
Despite the recent developments in deep learning models, their applications in clinical decision-support systems have been very limited. Recent digitalisation of health records, however, has provided a great platform for the assessment of the usability of such techniques in healthcare. As a result, the field is starting to see a growing number of research papers that employ deep learning on electronic health records (EHR) for personalised prediction of risks and health trajectories. While this can be a promising trend, vast paper-to-paper variability (from data sources and models they use to the clinical questions they attempt to answer) have hampered the field's ability to simply compare and contrast such models for a given application of interest. Thus, in this paper, we aim to provide a comparative review of the key deep learning architectures that have been applied to EHR data. Furthermore, we also aim to: (1) introduce and use one of the world's largest and most complex linked primary care EHR datasets (i.e., Clinical Practice Research Datalink, or CPRD) as a new asset for training such data-hungry models; (2) provide a guideline for working with EHR data for deep learning; (3) share some of the best practices for assessing the "goodness" of deep-learning models in clinical risk prediction; (4) and propose future research ideas for making deep learning models more suitable for the EHR data. Our results highlight the difficulties of working with highly imbalanced datasets, and show that sequential deep learning architectures such as RNN may be more suitable to deal with the temporal nature of EHR.
10.1016/j.jbi.2019.103337
Deep EHR: A Survey of Recent Advances in Deep Learning Techniques for Electronic Health Record (EHR) Analysis.
IEEE journal of biomedical and health informatics
The past decade has seen an explosion in the amount of digital information stored in electronic health records (EHRs). While primarily designed for archiving patient information and performing administrative healthcare tasks like billing, many researchers have found secondary use of these records for various clinical informatics applications. Over the same period, the machine learning community has seen widespread advances in the field of deep learning. In this review, we survey the current research on applying deep learning to clinical tasks based on EHR data, where we find a variety of deep learning techniques and frameworks being applied to several types of clinical applications including information extraction, representation learning, outcome prediction, phenotyping, and deidentification. We identify several limitations of current research involving topics such as model interpretability, data heterogeneity, and lack of universal benchmarks. We conclude by summarizing the state of the field and identifying avenues of future deep EHR research.
10.1109/JBHI.2017.2767063
Marrying Medical Domain Knowledge With Deep Learning on Electronic Health Records: A Deep Visual Analytics Approach.
Journal of medical Internet research
BACKGROUND:Deep learning models have attracted significant interest from health care researchers during the last few decades. There have been many studies that apply deep learning to medical applications and achieve promising results. However, there are three limitations to the existing models: (1) most clinicians are unable to interpret the results from the existing models, (2) existing models cannot incorporate complicated medical domain knowledge (eg, a disease causes another disease), and (3) most existing models lack visual exploration and interaction. Both the electronic health record (EHR) data set and the deep model results are complex and abstract, which impedes clinicians from exploring and communicating with the model directly. OBJECTIVE:The objective of this study is to develop an interpretable and accurate risk prediction model as well as an interactive clinical prediction system to support EHR data exploration, knowledge graph demonstration, and model interpretation. METHODS:A domain-knowledge-guided recurrent neural network (DG-RNN) model is proposed to predict clinical risks. The model takes medical event sequences as input and incorporates medical domain knowledge by attending to a subgraph of the whole medical knowledge graph. A global pooling operation and a fully connected layer are used to output the clinical outcomes. The middle results and the parameters of the fully connected layer are helpful in identifying which medical events cause clinical risks. DG-Viz is also designed to support EHR data exploration, knowledge graph demonstration, and model interpretation. RESULTS:We conducted both risk prediction experiments and a case study on a real-world data set. A total of 554 patients with heart failure and 1662 control patients without heart failure were selected from the data set. The experimental results show that the proposed DG-RNN outperforms the state-of-the-art approaches by approximately 1.5%. The case study demonstrates how our medical physician collaborator can effectively explore the data and interpret the prediction results using DG-Viz. CONCLUSIONS:In this study, we present DG-Viz, an interactive clinical prediction system, which brings together the power of deep learning (ie, a DG-RNN-based model) and visual analytics to predict clinical risks and visually interpret the EHR prediction results. Experimental results and a case study on heart failure risk prediction tasks demonstrate the effectiveness and usefulness of the DG-Viz system. This study will pave the way for interactive, interpretable, and accurate clinical risk predictions.
10.2196/20645
Deep representation learning of patient data from Electronic Health Records (EHR): A systematic review.
Si Yuqi,Du Jingcheng,Li Zhao,Jiang Xiaoqian,Miller Timothy,Wang Fei,Jim Zheng W,Roberts Kirk
Journal of biomedical informatics
OBJECTIVES:Patient representation learning refers to learning a dense mathematical representation of a patient that encodes meaningful information from Electronic Health Records (EHRs). This is generally performed using advanced deep learning methods. This study presents a systematic review of this field and provides both qualitative and quantitative analyses from a methodological perspective. METHODS:We identified studies developing patient representations from EHRs with deep learning methods from MEDLINE, EMBASE, Scopus, the Association for Computing Machinery (ACM) Digital Library, and the Institute of Electrical and Electronics Engineers (IEEE) Xplore Digital Library. After screening 363 articles, 49 papers were included for a comprehensive data collection. RESULTS:Publications developing patient representations almost doubled each year from 2015 until 2019. We noticed a typical workflow starting with feeding raw data, applying deep learning models, and ending with clinical outcome predictions as evaluations of the learned representations. Specifically, learning representations from structured EHR data was dominant (37 out of 49 studies). Recurrent Neural Networks were widely applied as the deep learning architecture (Long short-term memory: 13 studies, Gated recurrent unit: 11 studies). Learning was mainly performed in a supervised manner (30 studies) optimized with cross-entropy loss. Disease prediction was the most common application and evaluation (31 studies). Benchmark datasets were mostly unavailable (28 studies) due to privacy concerns of EHR data, and code availability was assured in 20 studies. DISCUSSION & CONCLUSION:The existing predictive models mainly focus on the prediction of single diseases, rather than considering the complex mechanisms of patients from a holistic review. We show the importance and feasibility of learning comprehensive representations of patient EHR data through a systematic review. Advances in patient representation learning techniques will be essential for powering patient-level EHR analyses. Future work will still be devoted to leveraging the richness and potential of available EHR data. Reproducibility and transparency of reported results will hopefully improve. Knowledge distillation and advanced learning techniques will be exploited to assist the capability of learning patient representation further.
10.1016/j.jbi.2020.103671
Opportunities and challenges in developing deep learning models using electronic health records data: a systematic review.
Journal of the American Medical Informatics Association : JAMIA
Objective:To conduct a systematic review of deep learning models for electronic health record (EHR) data, and illustrate various deep learning architectures for analyzing different data sources and their target applications. We also highlight ongoing research and identify open challenges in building deep learning models of EHRs. Design/method:We searched PubMed and Google Scholar for papers on deep learning studies using EHR data published between January 1, 2010, and January 31, 2018. We summarize them according to these axes: types of analytics tasks, types of deep learning model architectures, special challenges arising from health data and tasks and their potential solutions, as well as evaluation strategies. Results:We surveyed and analyzed multiple aspects of the 98 articles we found and identified the following analytics tasks: disease detection/classification, sequential prediction of clinical events, concept embedding, data augmentation, and EHR data privacy. We then studied how deep architectures were applied to these tasks. We also discussed some special challenges arising from modeling EHR data and reviewed a few popular approaches. Finally, we summarized how performance evaluations were conducted for each task. Discussion:Despite the early success in using deep learning for health analytics applications, there still exist a number of issues to be addressed. We discuss them in detail including data and label availability, the interpretability and transparency of the model, and ease of deployment.
10.1093/jamia/ocy068
Artificial intelligence in clinical care amidst COVID-19 pandemic: A systematic review.
Computational and structural biotechnology journal
The worldwide health crisis caused by the SARS-Cov-2 virus has resulted in>3 million deaths so far. Improving early screening, diagnosis and prognosis of the disease are critical steps in assisting healthcare professionals to save lives during this pandemic. Since WHO declared the COVID-19 outbreak as a pandemic, several studies have been conducted using Artificial Intelligence techniques to optimize these steps on clinical settings in terms of quality, accuracy and most importantly time. The objective of this study is to conduct a systematic literature review on published and preprint reports of Artificial Intelligence models developed and validated for screening, diagnosis and prognosis of the coronavirus disease 2019. We included 101 studies, published from January 1st, 2020 to December 30th, 2020, that developed AI prediction models which can be applied in the clinical setting. We identified in total 14 models for screening, 38 diagnostic models for detecting COVID-19 and 50 prognostic models for predicting ICU need, ventilator need, mortality risk, severity assessment or hospital length stay. Moreover, 43 studies were based on medical imaging and 58 studies on the use of clinical parameters, laboratory results or demographic features. Several heterogeneous predictors derived from multimodal data were identified. Analysis of these multimodal data, captured from various sources, in terms of prominence for each category of the included studies, was performed. Finally, Risk of Bias (RoB) analysis was also conducted to examine the applicability of the included studies in the clinical setting and assist healthcare providers, guideline developers, and policymakers.
10.1016/j.csbj.2021.05.010
Developing a FHIR-based EHR phenotyping framework: A case study for identification of patients with obesity and multiple comorbidities from discharge summaries.
Journal of biomedical informatics
BACKGROUND:Standards-based clinical data normalization has become a key component of effective data integration and accurate phenotyping for secondary use of electronic healthcare records (EHR) data. HL7 Fast Healthcare Interoperability Resources (FHIR) is an emerging clinical data standard for exchanging electronic healthcare data and has been used in modeling and integrating both structured and unstructured EHR data for a variety of clinical research applications. The overall objective of this study is to develop and evaluate a FHIR-based EHR phenotyping framework for identification of patients with obesity and its multiple comorbidities from semi-structured discharge summaries leveraging a FHIR-based clinical data normalization pipeline (known as NLP2FHIR). METHODS:We implemented a multi-class and multi-label classification system based on the i2b2 Obesity Challenge task to evaluate the FHIR-based EHR phenotyping framework. Two core parts of the framework are: (a) the conversion of discharge summaries into corresponding FHIR resources - Composition, Condition, MedicationStatement, Procedure and FamilyMemberHistory using the NLP2FHIR pipeline, and (b) the implementation of four machine learning algorithms (logistic regression, support vector machine, decision tree, and random forest) to train classifiers to predict disease state of obesity and 15 comorbidities using features extracted from standard FHIR resources and terminology expansions. We used the macro- and micro-averaged precision (P), recall (R), and F1 score (F1) measures to evaluate the classifier performance. We validated the framework using a second obesity dataset extracted from the MIMIC-III database. RESULTS:Using the NLP2FHIR pipeline, 1237 clinical discharge summaries from the 2008 i2b2 obesity challenge dataset were represented as the instances of the FHIR Composition resource consisting of 5677 records with 16 unique section types. After the NLP processing and FHIR modeling, a set of 244,438 FHIR clinical resource instances were generated. As the results of the four machine learning classifiers, the random forest algorithm performed the best with F1-micro(0.9466)/F1-macro(0.7887) and F1-micro(0.9536)/F1-macro(0.6524) for intuitive classification (reflecting medical professionals' judgments) and textual classification (reflecting the judgments based on explicitly reported information of diseases), respectively. The MIMIC-III obesity dataset was successfully integrated for prediction with minimal configuration of the NLP2FHIR pipeline and machine learning models. CONCLUSIONS:The study demonstrated that the FHIR-based EHR phenotyping approach could effectively identify the state of obesity and multiple comorbidities using semi-structured discharge summaries. Our FHIR-based phenotyping approach is a first concrete step towards improving the data aspect of phenotyping portability across EHR systems and enhancing interpretability of the machine learning-based phenotyping algorithms.
10.1016/j.jbi.2019.103310
An AI Approach for Identifying Patients With Cirrhosis.
Journal of clinical gastroenterology
GOAL:The goal of this study was to evaluate an artificial intelligence approach, namely deep learning, on clinical text in electronic health records (EHRs) to identify patients with cirrhosis. BACKGROUND AND AIMS:Accurate identification of cirrhosis in EHR is important for epidemiological, health services, and outcomes research. Currently, such efforts depend on International Classification of Diseases (ICD) codes, with limited success. MATERIALS AND METHODS:We trained several machine learning models using discharge summaries from patients with known cirrhosis from a patient registry and random controls without cirrhosis or its complications based on ICD codes. Models were validated on patients for whom discharge summaries were manually reviewed and used as the gold standard test set. We tested Naive Bayes and Random Forest as baseline models and a deep learning model using word embedding and a convolutional neural network (CNN). RESULTS:The training set included 446 cirrhosis patients and 689 controls, while the gold standard test set included 139 cirrhosis patients and 152 controls. Among the machine learning models, the CNN achieved the highest area under the receiver operating characteristic curve (0.993), with a precision of 0.965 and recall of 0.978, compared with 0.879 and 0.981 for the Naive Bayes and Random Forest, respectively (precision 0.787 and 0.958, and recalls 0.878 and 0.827). The precision by ICD codes for cirrhosis was 0.883 and recall was 0.978. CONCLUSIONS:A CNN model trained on discharge summaries identified cirrhosis patients with high precision and recall. This approach for phenotyping cirrhosis in the EHR may provide a more accurate assessment of disease burden in a variety of studies.
10.1097/MCG.0000000000001586
Impacts of structuring the electronic health record: Results of a systematic literature review from the perspective of secondary use of patient data.
Vuokko Riikka,Mäkelä-Bengs Päivi,Hyppönen Hannele,Lindqvist Minna,Doupi Persephone
International journal of medical informatics
PURPOSE:To explore the impacts that structuring of electronic health records (EHRs) has had from the perspective of secondary use of patient data as reflected in currently published literature. This paper presents the results of a systematic literature review aimed at answering the following questions; (1) what are the common methods of structuring patient data to serve secondary use purposes; (2) what are the common methods of evaluating patient data structuring in the secondary use context, and (3) what impacts or outcomes of EHR structuring have been reported from the secondary use perspective. METHODS:The reported study forms part of a wider systematic literature review on the impacts of EHR structuring methods and evaluations of their impact. The review was based on a 12-step systematic review protocol adapted from the Cochrane methodology. Original articles included in the study were divided into three groups for analysis and reporting based on their use focus: nursing documentation, medical use and secondary use (presented in this paper). The analysis from the perspective of secondary use of data includes 85 original articles from 1975 to 2010 retrieved from 15 bibliographic databases. RESULTS:The implementation of structured EHRs can be roughly divided into applications for documenting patient data at the point of care and application for retrieval of patient data (post hoc structuring). Two thirds of the secondary use articles concern EHR structuring methods which were still under development or in the testing phase. METHODS:of structuring patient data such as codes, terminologies, reference information models, forms or templates and documentation standards were usually applied in combination. Most of the identified benefits of utilizing structured EHR data for secondary use purposes concentrated on information content and quality or on technical quality and reliability, particularly in the case of Natural Language Processing (NLP) studies. A few individual articles evaluated impacts on care processes, productivity and costs, patient safety, care quality or other health impacts. In most articles these endpoints were usually discussed as goals of secondary use and less as evidence-supported impacts, resulting from the use of structured EHR data for secondary purposes. CONCLUSIONS:Further studies and more sound evaluation methods are needed for evidence on how EHRs are utilized for secondary purposes, and how structured documentation methods can serve different users' needs, e.g. administration, statistics and research and development, in parallel to medical use purposes.
10.1016/j.ijmedinf.2016.10.004
Data gaps in electronic health record (EHR) systems: An audit of problem list completeness during the COVID-19 pandemic.
International journal of medical informatics
OBJECTIVE:To evaluate the completeness of diagnosis recording in problem lists in a hospital electronic health record (EHR) system during the COVID-19 pandemic. DESIGN:Retrospective chart review with manual review of free text electronic case notes. SETTING:Major teaching hospital trust in London, one year after the launch of a comprehensive EHR system (Epic), during the first peak of the COVID-19 pandemic in the UK. PARTICIPANTS:516 patients with suspected or confirmed COVID-19. MAIN OUTCOME MEASURES:Percentage of diagnoses already included in the structured problem list. RESULTS:Prior to review, these patients had a combined total of 2841 diagnoses recorded in their EHR problem lists. 1722 additional diagnoses were identified, increasing the mean number of recorded problems per patient from 5.51 to 8.84. The overall percentage of diagnoses originally included in the problem list was 62.3% (2841 / 4563, 95% confidence interval 60.8%, 63.7%). CONCLUSIONS:Diagnoses and other clinical information stored in a structured way in electronic health records is extremely useful for supporting clinical decisions, improving patient care and enabling better research. However, recording of medical diagnoses on the structured problem list for inpatients is incomplete, with almost 40% of important diagnoses mentioned only in the free text notes.
10.1016/j.ijmedinf.2021.104452
The Role of Electronic Health Records in Advancing Genomic Medicine.
Annual review of genomics and human genetics
Recent advances in genomic technology and widespread adoption of electronic health records (EHRs) have accelerated the development of genomic medicine, bringing promising research findings from genome science into clinical practice. Genomic and phenomic data, accrued across large populations through biobanks linked to EHRs, have enabled the study of genetic variation at a phenome-wide scale. Through new quantitative techniques, pleiotropy can be explored with phenome-wide association studies, the occurrence of common complex diseases can be predicted using the cumulative influence of many genetic variants (polygenic risk scores), and undiagnosed Mendelian syndromes can be identified using EHR-based phenotypic signatures (phenotype risk scores). In this review, we trace the role of EHRs from the development of genome-wide analytic techniques to translational efforts to test these new interventions to the clinic. Throughout, we describe the challenges that remain when combining EHRs with genetics to improve clinical care.
10.1146/annurev-genom-121120-125204
Electronic health records and polygenic risk scores for predicting disease risk.
Li Ruowang,Chen Yong,Ritchie Marylyn D,Moore Jason H
Nature reviews. Genetics
Accurate prediction of disease risk based on the genetic make-up of an individual is essential for effective prevention and personalized treatment. Nevertheless, to date, individual genetic variants from genome-wide association studies have achieved only moderate prediction of disease risk. The aggregation of genetic variants under a polygenic model shows promising improvements in prediction accuracies. Increasingly, electronic health records (EHRs) are being linked to patient genetic data in biobanks, which provides new opportunities for developing and applying polygenic risk scores in the clinic, to systematically examine and evaluate patient susceptibilities to disease. However, the heterogeneous nature of EHR data brings forth many practical challenges along every step of designing and implementing risk prediction strategies. In this Review, we present the unique considerations for using genotype and phenotype data from biobank-linked EHRs for polygenic risk prediction.
10.1038/s41576-020-0224-1
Research evidence on strategies enabling integration of electronic health records in the health care systems of low- and middle-income countries: A literature review.
Kumar Manish,Mostafa Javed
The International journal of health planning and management
Integration of electronic health records (EHRs) in the national health care systems of low- and middle-income countries (LMICs) is vital for achieving the United Nations Sustainable Development Goal of ensuring healthy lives and promoting well-being for all people of all ages. National EHR systems are increasing, but mostly in developed countries. Besides, there is limited research evidence on successful strategies for ensuring integration of national EHRs in the health care systems of LMICs. To fill this evidence gap, a comprehensive survey of literature was conducted using scientific electronic databases-PubMed, SCOPUS, Web of Science, and Global Health-and consultations with international experts. The review highlights the lack of evidence on strategies for integrating EHR systems, although there was ample evidence on implementation challenges and relevance of EHRs to vertical disease programs such as HIV. The findings describe the narrow focus of EHR implementation, the prominence of vertical disease programs in EHR adoption, testing of theoretical and conceptual models for EHR implementation and success, and strategies for EHR implementation. The review findings are further amplified through examples of EHR implementation in Sierra Leone, Malawi, and India. Unless evidence-based strategies are identified and applied, integration of national EHRs in the health care systems of LMICs is difficult.
10.1002/hpm.2754
Applying Artificial Intelligence to Gynecologic Oncology: A Review.
Obstetrical & gynecological survey
IMPORTANCE:Artificial intelligence (AI) will play an increasing role in health care. In gynecologic oncology, it can advance tailored screening, precision surgery, and personalized targeted therapies. OBJECTIVE:The aim of this study was to review the role of AI in gynecologic oncology. EVIDENCE ACQUISITION:Artificial intelligence publications in gynecologic oncology were identified by searching "gynecologic oncology AND artificial intelligence" in the PubMed database. A review of the literature was performed on the history of AI, its fundamentals, and current applications as related to diagnosis and treatment of cervical, uterine, and ovarian cancers. RESULTS:A PubMed literature search since the year 2000 showed a significant increase in oncology publications related to AI and oncology. Early studies focused on using AI to interrogate electronic health records in order to improve clinical outcome and facilitate clinical research. In cervical cancer, AI algorithms can enhance image analysis of cytology and visual inspection with acetic acid or colposcopy. In uterine cancers, AI can improve the diagnostic accuracies of radiologic imaging and predictive/prognostic capabilities of clinicopathologic characteristics. Artificial intelligence has also been used to better detect early-stage ovarian cancer and predict surgical outcomes and treatment response. CONCLUSIONS AND RELEVANCE:Artificial intelligence has been shown to enhance diagnosis, refine clinical decision making, and advance personalized therapies in gynecologic cancers. The rapid adoption of AI in gynecologic oncology will depend on overcoming the challenges related to data transparency, quality, and interpretation. Artificial intelligence is rapidly transforming health care. However, many physicians are unaware that this technology is being used in their practices and could benefit from a better understanding of the statistics and computer science behind these algorithms. This review provides a summary of AI, its applicability, and its limitations in gynecologic oncology.
10.1097/OGX.0000000000000902
Use of Machine Learning and Artificial Intelligence Methods in Geriatric Mental Health Research Involving Electronic Health Record or Administrative Claims Data: A Systematic Review.
Frontiers in psychiatry
Electronic health records (EHR) and administrative healthcare data (AHD) are frequently used in geriatric mental health research to answer various health research questions. However, there is an increasing amount and complexity of data available that may lend itself to alternative analytic approaches using machine learning (ML) or artificial intelligence (AI) methods. We performed a systematic review of the current application of ML or AI approaches to the analysis of EHR and AHD in geriatric mental health. We searched MEDLINE, Embase, and PsycINFO to identify potential studies. We included all articles that used ML or AI methods on topics related to geriatric mental health utilizing EHR or AHD data. We assessed study quality either by Prediction model Risk OF Bias ASsessment Tool (PROBAST) or Quality Assessment of Diagnostic Accuracy Studies (QUADAS-2) checklist. We initially identified 391 articles through an electronic database and reference search, and 21 articles met inclusion criteria. Among the selected studies, EHR was the most used data type, and the datasets were mainly structured. A variety of ML and AI methods were used, with prediction or classification being the main application of ML or AI with the random forest as the most common ML technique. Dementia was the most common mental health condition observed. The relative advantages of ML or AI techniques compared to biostatistical methods were generally not assessed. Only in three studies, low risk of bias (ROB) was observed according to all the PROBAST domains but in none according to QUADAS-2 domains. The quality of study reporting could be further improved. There are currently relatively few studies using ML and AI in geriatric mental health research using EHR and AHD methods, although this field is expanding. Aside from dementia, there are few studies of other geriatric mental health conditions. The lack of consistent information in the selected studies precludes precise comparisons between them. Improving the quality of reporting of ML and AI work in the future would help improve research in the field. Other courses of improvement include using common data models to collect/organize data, and common datasets for ML model validation.
10.3389/fpsyt.2021.738466
Applications of Artificial Intelligence to Electronic Health Record Data in Ophthalmology.
Lin Wei-Chun,Chen Jimmy S,Chiang Michael F,Hribar Michelle R
Translational vision science & technology
Widespread adoption of electronic health records (EHRs) has resulted in the collection of massive amounts of clinical data. In ophthalmology in particular, the volume range of data captured in EHR systems has been growing rapidly. Yet making effective secondary use of this EHR data for improving patient care and facilitating clinical decision-making has remained challenging due to the complexity and heterogeneity of these data. Artificial intelligence (AI) techniques present a promising way to analyze these multimodal data sets. While AI techniques have been extensively applied to imaging data, there are a limited number of studies employing AI techniques with clinical data from the EHR. The objective of this review is to provide an overview of different AI methods applied to EHR data in the field of ophthalmology. This literature review highlights that the secondary use of EHR data has focused on glaucoma, diabetic retinopathy, age-related macular degeneration, and cataracts with the use of AI techniques. These techniques have been used to improve ocular disease diagnosis, risk assessment, and progression prediction. Techniques such as supervised machine learning, deep learning, and natural language processing were most commonly used in the articles reviewed.
10.1167/tvst.9.2.13
Artificial Intelligence Techniques That May Be Applied to Primary Care Data to Facilitate Earlier Diagnosis of Cancer: Systematic Review.
Journal of medical Internet research
BACKGROUND:More than 17 million people worldwide, including 360,000 people in the United Kingdom, were diagnosed with cancer in 2018. Cancer prognosis and disease burden are highly dependent on the disease stage at diagnosis. Most people diagnosed with cancer first present in primary care settings, where improved assessment of the (often vague) presenting symptoms of cancer could lead to earlier detection and improved outcomes for patients. There is accumulating evidence that artificial intelligence (AI) can assist clinicians in making better clinical decisions in some areas of health care. OBJECTIVE:This study aimed to systematically review AI techniques that may facilitate earlier diagnosis of cancer and could be applied to primary care electronic health record (EHR) data. The quality of the evidence, the phase of development the AI techniques have reached, the gaps that exist in the evidence, and the potential for use in primary care were evaluated. METHODS:We searched MEDLINE, Embase, SCOPUS, and Web of Science databases from January 01, 2000, to June 11, 2019, and included all studies providing evidence for the accuracy or effectiveness of applying AI techniques for the early detection of cancer, which may be applicable to primary care EHRs. We included all study designs in all settings and languages. These searches were extended through a scoping review of AI-based commercial technologies. The main outcomes assessed were measures of diagnostic accuracy for cancer. RESULTS:We identified 10,456 studies; 16 studies met the inclusion criteria, representing the data of 3,862,910 patients. A total of 13 studies described the initial development and testing of AI algorithms, and 3 studies described the validation of an AI algorithm in independent data sets. One study was based on prospectively collected data; only 3 studies were based on primary care data. We found no data on implementation barriers or cost-effectiveness. Risk of bias assessment highlighted a wide range of study quality. The additional scoping review of commercial AI technologies identified 21 technologies, only 1 meeting our inclusion criteria. Meta-analysis was not undertaken because of the heterogeneity of AI modalities, data set characteristics, and outcome measures. CONCLUSIONS:AI techniques have been applied to EHR-type data to facilitate early diagnosis of cancer, but their use in primary care settings is still at an early stage of maturity. Further evidence is needed on their performance using primary care data, implementation barriers, and cost-effectiveness before widespread adoption into routine primary care clinical practice can be recommended.
10.2196/23483
Artificial Intelligence and Early Detection of Pancreatic Cancer: 2020 Summative Review.
Pancreas
ABSTRACT:Despite considerable research efforts, pancreatic cancer is associated with a dire prognosis and a 5-year survival rate of only 10%. Early symptoms of the disease are mostly nonspecific. The premise of improved survival through early detection is that more individuals will benefit from potentially curative treatment. Artificial intelligence (AI) methodology has emerged as a successful tool for risk stratification and identification in general health care. In response to the maturity of AI, Kenner Family Research Fund conducted the 2020 AI and Early Detection of Pancreatic Cancer Virtual Summit (www.pdac-virtualsummit.org) in conjunction with the American Pancreatic Association, with a focus on the potential of AI to advance early detection efforts in this disease. This comprehensive presummit article was prepared based on information provided by each of the interdisciplinary participants on one of the 5 following topics: Progress, Problems, and Prospects for Early Detection; AI and Machine Learning; AI and Pancreatic Cancer-Current Efforts; Collaborative Opportunities; and Moving Forward-Reflections from Government, Industry, and Advocacy. The outcome from the robust Summit conversations, to be presented in a future white paper, indicate that significant progress must be the result of strategic collaboration among investigators and institutions from multidisciplinary backgrounds, supported by committed funders.
10.1097/MPA.0000000000001762
Early Detection of Pancreatic Cancer: Applying Artificial Intelligence to Electronic Health Records.
Pancreas
ABSTRACT:The potential of artificial intelligence (AI) applied to clinical data from electronic health records (EHRs) to improve early detection for pancreatic and other cancers remains underexplored. The Kenner Family Research Fund, in collaboration with the Cancer Biomarker Research Group at the National Cancer Institute, organized the workshop entitled: "Early Detection of Pancreatic Cancer: Opportunities and Challenges in Utilizing Electronic Health Records (EHR)" in March 2021. The workshop included a select group of panelists with expertise in pancreatic cancer, EHR data mining, and AI-based modeling. This review article reflects the findings from the workshop and assesses the feasibility of AI-based data extraction and modeling applied to EHRs. It highlights the increasing role of data sharing networks and common data models in improving the secondary use of EHR data. Current efforts using EHR data for AI-based modeling to enhance early detection of pancreatic cancer show promise. Specific challenges (biology, limited data, standards, compatibility, legal, quality, AI chasm, incentives) are identified, with mitigation strategies summarized and next steps identified.
10.1097/MPA.0000000000001882
Randomized Controlled Trials of Electronic Health Record Interventions: Design, Conduct, and Reporting Considerations.
Pletcher Mark J,Flaherman Valerie,Najafi Nader,Patel Sajan,Rushakoff Robert J,Hoffman Ari,Robinson Andrew,Cucina Russell J,McCulloch Charles E,Gonzales Ralph,Auerbach Andrew
Annals of internal medicine
Electronic health record (EHR) systems can be configured to deliver novel EHR interventions that influence clinical decision making and to support efficient randomized controlled trials (RCTs) designed to evaluate the effectiveness, safety, and costs of those interventions. In designing RCTs of EHR interventions, one should carefully consider the unit of randomization (for example, patient, encounter, clinician, or clinical unit), balancing concerns about contamination of an intervention across randomization units within clusters (for example, patients within clinical units) against the superior control of measured and unmeasured confounders that comes with randomizing a larger number of units. One should also consider whether the key computational assessment components of the EHR intervention, such as a predictive algorithm used to target a subgroup for decision support, should occur before randomization (so that only 1 subgroup is randomized) or after randomization (including all subgroups). When these components are applied after randomization, one must consider expected heterogeneity in the effect of the differential decision support across subgroups, which has implications for overall impact potential, analytic approach, and sample size planning. Trials of EHR interventions should be reviewed by an institutional review board, but may not require patient-level informed consent when the interventions being tested can be considered minimal risk or quality improvement, and when clinical decision making is supported, rather than controlled, by an EHR intervention. Data and safety monitoring for RCTs of EHR interventions should be conducted to guide institutional pragmatic decision making about implementation and ensure that continuing randomization remains justified. Reporting should follow the CONSORT (Consolidated Standards of Reporting Trials) Statement, with extensions for pragmatic trials and cluster RCTs when applicable, and should include detailed materials to enhance reproducibility.
10.7326/M19-0877
The Longitudinal Epidemiologic Assessment of Diabetes Risk (LEADR): Unique 1.4 M patient Electronic Health Record cohort.
Healthcare (Amsterdam, Netherlands)
BACKGROUND:The Longitudinal Epidemiologic Assessment of Diabetes Risk (LEADR) study uses a novel Electronic Health Record (EHR) data approach as a tool to assess the epidemiology of known and new risk factors for type 2 diabetes mellitus (T2DM) and study how prevention interventions affect progression to and onset of T2DM. We created an electronic cohort of 1.4 million patients having had at least 4 encounters with a healthcare organization for at least 24-months; were aged ≥18 years in 2010; and had no diabetes (i.e., T1DM or T2DM) at cohort entry or in the 12 months following entry. EHR data came from patients at nine healthcare organizations across the U.S. between January 1, 2010-December 31, 2016. RESULTS:Approximately 5.9% of the LEADR cohort (82,922 patients) developed T2DM, providing opportunities to explore longitudinal clinical care, medication use, risk factor trajectories, and diagnoses for these patients, compared with patients similarly matched prior to disease onset. CONCLUSIONS:LEADR represents one of the largest EHR databases to have repurposed EHR data to examine patients' T2DM risk. This paper is first in a series demonstrating this novel approach to studying T2DM. IMPLICATIONS:Chronic conditions that often take years to develop can be studied efficiently using EHR data in a retrospective design. LEVEL OF EVIDENCE:While much is already known about T2DM risk, this EHR's cohort's 160 M data points for 1.4 M people over six years, provides opportunities to investigate new unique risk factors and evaluate research hypotheses where results could modify public health practice for preventing T2DM.
10.1016/j.hjdsi.2020.100458
Using electronic health records to quantify and stratify the severity of type 2 diabetes in primary care in England: rationale and cohort study design.
Zghebi Salwa S,Rutter Martin K,Ashcroft Darren M,Salisbury Chris,Mallen Christian,Chew-Graham Carolyn A,Reeves David,van Marwijk Harm,Qureshi Nadeem,Weng Stephen,Peek Niels,Planner Claire,Nowakowska Magdalena,Mamas Mamas,Kontopantelis Evangelos
BMJ open
INTRODUCTION:The increasing prevalence of type 2 diabetes mellitus (T2DM) presents a significant burden on affected individuals and healthcare systems internationally. There is, however, no agreed validated measure to infer diabetes severity from electronic health records (EHRs). We aim to quantify T2DM severity and validate it using clinical adverse outcomes. METHODS AND ANALYSIS:Primary care data from the Clinical Practice Research Datalink, linked hospitalisation and mortality records between April 2007 and March 2017 for patients with T2DM in England will be used to develop a clinical algorithm to grade T2DM severity. The EHR-based algorithm will incorporate main risk factors (severity domains) for adverse outcomes to stratify T2DM cohorts by baseline and longitudinal severity scores. Provisionally, T2DM severity domains, identified through a systematic review and expert opinion, are: diabetes duration, glycated haemoglobin, microvascular complications, comorbidities and coprescribed treatments. Severity scores will be developed by two approaches: (1) calculating a count score of severity domains; (2) through hierarchical stratification of complications. Regression models estimates will be used to calculate domains weights. Survival analyses for the association between weighted severity scores and future outcomes-cardiovascular events, hospitalisation (diabetes-related, cardiovascular) and mortality (diabetes-related, cardiovascular, all-cause mortality)-will be performed as statistical validation. The proposed EHR-based approach will quantify the T2DM severity for primary care performance management and inform the methodology for measuring severity of other primary care-managed chronic conditions. We anticipate that the developed algorithm will be a practical tool for practitioners, aid clinical management decision-making, inform stratified medicine, support future clinical trials and contribute to more effective service planning and policy-making. ETHICS AND DISSEMINATION:The study protocol was approved by the Independent Scientific Advisory Committee. Some data were presented at the National Institute for Health Research School for Primary Care Research Showcase, September 2017, Oxford, UK and the Diabetes UK Professional Conference March 2018, London, UK. The study findings will be disseminated in relevant academic conferences and peer-reviewed journals.
10.1136/bmjopen-2017-020926
Real world evidence in cardiovascular medicine: ensuring data validity in electronic health record-based studies.
Hernandez-Boussard Tina,Monda Keri L,Crespo Blai Coll,Riskin Dan
Journal of the American Medical Informatics Association : JAMIA
OBJECTIVE:With growing availability of digital health data and technology, health-related studies are increasingly augmented or implemented using real world data (RWD). Recent federal initiatives promote the use of RWD to make clinical assertions that influence regulatory decision-making. Our objective was to determine whether traditional real world evidence (RWE) techniques in cardiovascular medicine achieve accuracy sufficient for credible clinical assertions, also known as "regulatory-grade" RWE. DESIGN:Retrospective observational study using electronic health records (EHR), 2010-2016. METHODS:A predefined set of clinical concepts was extracted from EHR structured (EHR-S) and unstructured (EHR-U) data using traditional query techniques and artificial intelligence (AI) technologies, respectively. Performance was evaluated against manually annotated cohorts using standard metrics. Accuracy was compared to pre-defined criteria for regulatory-grade. Differences in accuracy were compared using Chi-square test. RESULTS:The dataset included 10 840 clinical notes. Individual concept occurrence ranged from 194 for coronary artery bypass graft to 4502 for diabetes mellitus. In EHR-S, average recall and precision were 51.7% and 98.3%, respectively and 95.5% and 95.3% in EHR-U, respectively. For each clinical concept, EHR-S accuracy was below regulatory-grade, while EHR-U met or exceeded criteria, with the exception of medications. CONCLUSIONS:Identifying an appropriate RWE approach is dependent on cohorts studied and accuracy required. In this study, recall varied greatly between EHR-S and EHR-U. Overall, EHR-S did not meet regulatory grade criteria, while EHR-U did. These results suggest that recall should be routinely measured in EHR-based studes intended for regulatory use. Furthermore, advanced data and technologies may be required to achieve regulatory grade results.
10.1093/jamia/ocz119
A novel method for causal structure discovery from EHR data and its application to type-2 diabetes mellitus.
Scientific reports
Modern AI-based clinical decision support models owe their success in part to the very large number of predictors they use. Safe and robust decision support, especially for intervention planning, requires causal, not associative, relationships. Traditional methods of causal discovery, clinical trials and extracting biochemical pathways, are resource intensive and may not scale up to the number and complexity of relationships sufficient for precision treatment planning. Computational causal structure discovery (CSD) from electronic health records (EHR) data can represent a solution, however, current CSD methods fall short on EHR data. This paper presents a CSD method tailored to the EHR data. The application of the proposed methodology was demonstrated on type-2 diabetes mellitus. A large EHR dataset from Mayo Clinic was used as development cohort, and another large dataset from an independent health system, M Health Fairview, as external validation cohort. The proposed method achieved very high recall (.95) and substantially higher precision than the general-purpose methods (.84 versus .29, and .55). The causal relationships extracted from the development and external validation cohorts had a high (81%) overlap. Due to the adaptations to EHR data, the proposed method is more suitable for use in clinical decision support than the general-purpose methods.
10.1038/s41598-021-99990-7
Explainable artificial intelligence model to predict acute critical illness from electronic health records.
Lauritsen Simon Meyer,Kristensen Mads,Olsen Mathias Vassard,Larsen Morten Skaarup,Lauritsen Katrine Meyer,Jørgensen Marianne Johansson,Lange Jeppe,Thiesson Bo
Nature communications
Acute critical illness is often preceded by deterioration of routinely measured clinical parameters, e.g., blood pressure and heart rate. Early clinical prediction is typically based on manually calculated screening metrics that simply weigh these parameters, such as early warning scores (EWS). The predictive performance of EWSs yields a tradeoff between sensitivity and specificity that can lead to negative outcomes for the patient. Previous work on electronic health records (EHR) trained artificial intelligence (AI) systems offers promising results with high levels of predictive performance in relation to the early, real-time prediction of acute critical illness. However, without insight into the complex decisions by such system, clinical translation is hindered. Here, we present an explainable AI early warning score (xAI-EWS) system for early detection of acute critical illness. xAI-EWS potentiates clinical translation by accompanying a prediction with information on the EHR data explaining it.
10.1038/s41467-020-17431-x