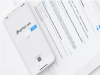
Advances in protein structure prediction and design.
Nature reviews. Molecular cell biology
The prediction of protein three-dimensional structure from amino acid sequence has been a grand challenge problem in computational biophysics for decades, owing to its intrinsic scientific interest and also to the many potential applications for robust protein structure prediction algorithms, from genome interpretation to protein function prediction. More recently, the inverse problem - designing an amino acid sequence that will fold into a specified three-dimensional structure - has attracted growing attention as a potential route to the rational engineering of proteins with functions useful in biotechnology and medicine. Methods for the prediction and design of protein structures have advanced dramatically in the past decade. Increases in computing power and the rapid growth in protein sequence and structure databases have fuelled the development of new data-intensive and computationally demanding approaches for structure prediction. New algorithms for designing protein folds and protein-protein interfaces have been used to engineer novel high-order assemblies and to design from scratch fluorescent proteins with novel or enhanced properties, as well as signalling proteins with therapeutic potential. In this Review, we describe current approaches for protein structure prediction and design and highlight a selection of the successful applications they have enabled.
10.1038/s41580-019-0163-x
Deciphering interaction fingerprints from protein molecular surfaces using geometric deep learning.
Gainza P,Sverrisson F,Monti F,Rodolà E,Boscaini D,Bronstein M M,Correia B E
Nature methods
Predicting interactions between proteins and other biomolecules solely based on structure remains a challenge in biology. A high-level representation of protein structure, the molecular surface, displays patterns of chemical and geometric features that fingerprint a protein's modes of interactions with other biomolecules. We hypothesize that proteins participating in similar interactions may share common fingerprints, independent of their evolutionary history. Fingerprints may be difficult to grasp by visual analysis but could be learned from large-scale datasets. We present MaSIF (molecular surface interaction fingerprinting), a conceptual framework based on a geometric deep learning method to capture fingerprints that are important for specific biomolecular interactions. We showcase MaSIF with three prediction challenges: protein pocket-ligand prediction, protein-protein interaction site prediction and ultrafast scanning of protein surfaces for prediction of protein-protein complexes. We anticipate that our conceptual framework will lead to improvements in our understanding of protein function and design.
10.1038/s41592-019-0666-6
Protein complexes in cells by AI-assisted structural proteomics.
Molecular systems biology
Accurately modeling the structures of proteins and their complexes using artificial intelligence is revolutionizing molecular biology. Experimental data enable a candidate-based approach to systematically model novel protein assemblies. Here, we use a combination of in-cell crosslinking mass spectrometry and co-fractionation mass spectrometry (CoFrac-MS) to identify protein-protein interactions in the model Gram-positive bacterium Bacillus subtilis. We show that crosslinking interactions prior to cell lysis reveals protein interactions that are often lost upon cell lysis. We predict the structures of these protein interactions and others in the SubtiWiki database with AlphaFold-Multimer and, after controlling for the false-positive rate of the predictions, we propose novel structural models of 153 dimeric and 14 trimeric protein assemblies. Crosslinking MS data independently validates the AlphaFold predictions and scoring. We report and validate novel interactors of central cellular machineries that include the ribosome, RNA polymerase, and pyruvate dehydrogenase, assigning function to several uncharacterized proteins. Our approach uncovers protein-protein interactions inside intact cells, provides structural insight into their interaction interfaces, and is applicable to genetically intractable organisms, including pathogenic bacteria.
10.15252/msb.202311544
Higher-order modular regulation of the human proteome.
Molecular systems biology
Operons are transcriptional modules that allow bacteria to adapt to environmental changes by coordinately expressing the relevant set of genes. In humans, biological pathways and their regulation are more complex. If and how human cells coordinate the expression of entire biological processes is unclear. Here, we capture 31 higher-order co-regulation modules, which we term progulons, by help of supervised machine-learning on proteomics data. Progulons consist of dozens to hundreds of proteins that together mediate core cellular functions. They are not restricted to physical interactions or co-localisation. Progulon abundance changes are primarily controlled at the level of protein synthesis and degradation. Implemented as a web app at www.proteomehd.net/progulonFinder, our approach enables the targeted search for progulons of specific cellular processes. We use it to identify a DNA replication progulon and reveal multiple new replication factors, validated by extensive phenotyping of siRNA-induced knockdowns. Progulons provide a new entry point into the molecular understanding of biological processes.
10.15252/msb.20209503
Rapid mass spectrometric conversion of tissue biopsy samples into permanent quantitative digital proteome maps.
Guo Tiannan,Kouvonen Petri,Koh Ching Chiek,Gillet Ludovic C,Wolski Witold E,Röst Hannes L,Rosenberger George,Collins Ben C,Blum Lorenz C,Gillessen Silke,Joerger Markus,Jochum Wolfram,Aebersold Ruedi
Nature medicine
Clinical specimens are each inherently unique, limited and nonrenewable. Small samples such as tissue biopsies are often completely consumed after a limited number of analyses. Here we present a method that enables fast and reproducible conversion of a small amount of tissue (approximating the quantity obtained by a biopsy) into a single, permanent digital file representing the mass spectrometry (MS)-measurable proteome of the sample. The method combines pressure cycling technology (PCT) and sequential window acquisition of all theoretical fragment ion spectra (SWATH)-MS. The resulting proteome maps can be analyzed, re-analyzed, compared and mined in silico to detect and quantify specific proteins across multiple samples. We used this method to process and convert 18 biopsy samples from nine patients with renal cell carcinoma into SWATH-MS fragment ion maps. From these proteome maps we detected and quantified more than 2,000 proteins with a high degree of reproducibility across all samples. The measured proteins clearly distinguished tumorous kidney tissues from healthy tissues and differentiated distinct histomorphological kidney cancer subtypes.
10.1038/nm.3807
Recent advances in mass-spectrometry based proteomics software, tools and databases.
Drug discovery today. Technologies
The field of proteomics immensely depends on data generation and data analysis which are thoroughly supported by software and databases. There has been a massive advancement in mass spectrometry-based proteomics over the last 10 years which has compelled the scientific community to upgrade or develop algorithms, tools, and repository databases in the field of proteomics. Several standalone software, and comprehensive databases have aided the establishment of integrated omics pipeline and meta-analysis workflow which has contributed to understand the disease pathobiology, biomarker discovery and predicting new therapeutic modalities. For shotgun proteomics where Data Dependent Acquisition is performed, several user-friendly software are developed that can analyse the pre-processed data to provide mechanistic insights of the disease. Likewise, in Data Independent Acquisition, pipelines are emerged which can accomplish the task from building the spectral library to identify the therapeutic targets. Furthermore, in the age of big data analysis the implications of machine learning and cloud computing are appending robustness, rapidness and in-depth proteomics data analysis. The current review talks about the recent advancement, and development of software, tools, and database in the field of mass-spectrometry based proteomics.
10.1016/j.ddtec.2021.06.007
Bioinformatics Methods for Mass Spectrometry-Based Proteomics Data Analysis.
Chen Chen,Hou Jie,Tanner John J,Cheng Jianlin
International journal of molecular sciences
Recent advances in mass spectrometry (MS)-based proteomics have enabled tremendous progress in the understanding of cellular mechanisms, disease progression, and the relationship between genotype and phenotype. Though many popular bioinformatics methods in proteomics are derived from other omics studies, novel analysis strategies are required to deal with the unique characteristics of proteomics data. In this review, we discuss the current developments in the bioinformatics methods used in proteomics and how they facilitate the mechanistic understanding of biological processes. We first introduce bioinformatics software and tools designed for mass spectrometry-based protein identification and quantification, and then we review the different statistical and machine learning methods that have been developed to perform comprehensive analysis in proteomics studies. We conclude with a discussion of how quantitative protein data can be used to reconstruct protein interactions and signaling networks.
10.3390/ijms21082873
Simple, efficient and thorough shotgun proteomic analysis with PatternLab V.
Nature protocols
Shotgun proteomics aims to identify and quantify the thousands of proteins in complex mixtures such as cell and tissue lysates and biological fluids. This approach uses liquid chromatography coupled with tandem mass spectrometry and typically generates hundreds of thousands of mass spectra that require specialized computational environments for data analysis. PatternLab for proteomics is a unified computational environment for analyzing shotgun proteomic data. PatternLab V (PLV) is the most comprehensive and crucial update so far, the result of intensive interaction with the proteomics community over several years. All PLV modules have been optimized and its graphical user interface has been completely updated for improved user experience. Major improvements were made to all aspects of the software, ranging from boosting the number of protein identifications to faster extraction of ion chromatograms. PLV provides modules for preparing sequence databases, protein identification, statistical filtering and in-depth result browsing for both labeled and label-free quantitation. The PepExplorer module can even pinpoint de novo sequenced peptides not already present in the database. PLV is of broad applicability and therefore suitable for challenging experimental setups, such as time-course experiments and data handling from unsequenced organisms. PLV interfaces with widely adopted software and community initiatives, e.g., Comet, Skyline, PEAKS and PRIDE. It is freely available at http://www.patternlabforproteomics.org .
10.1038/s41596-022-00690-x
Proteomic differences in hippocampus and cortex of sudden unexplained death in childhood.
Acta neuropathologica
Sudden unexplained death in childhood (SUDC) is death of a child over 1 year of age that is unexplained after review of clinical history, circumstances of death, and complete autopsy with ancillary testing. Multiple etiologies may cause SUDC. SUDC and sudden unexpected death in epilepsy (SUDEP) share clinical and pathological features, suggesting some similarities in mechanism of death and possible abnormalities in hippocampus and cortex. To identify molecular signaling pathways, we performed label-free quantitative mass spectrometry on microdissected frontal cortex, hippocampal dentate gyrus (DG), and cornu ammonis (CA1-3) in SUDC (n = 19) and pediatric control cases (n = 19) with an explained cause of death. At a 5% false discovery rate (FDR), we found differential expression of 660 proteins in frontal cortex, 170 in DG, and 57 in CA1-3. Pathway analysis of altered proteins identified top signaling pathways associated with activated oxidative phosphorylation (p = 6.3 × 10, z = 4.08) and inhibited EIF2 signaling (p = 2.0 × 10, z = - 2.56) in frontal cortex, and activated acute phase response in DG (p = 8.5 × 10, z = 2.65) and CA1-3 (p = 4.7 × 10, z = 2.00). Weighted gene correlation network analysis (WGCNA) of clinical history indicated that SUDC-positive post-mortem virology (n = 4/17) had the most significant module in each brain region, with the top most significant associated with decreased mRNA metabolic processes (p = 2.8 × 10) in frontal cortex. Additional modules were associated with clinical history, including fever within 24 h of death (top: increased mitochondrial fission in DG, p = 1.8 × 10) and febrile seizure history (top: decreased small molecule metabolic processes in frontal cortex, p = 8.8 × 10) in all brain regions, neuropathological hippocampal findings in the DG (top: decreased focal adhesion, p = 1.9 × 10). Overall, cortical and hippocampal protein changes were present in SUDC cases and some correlated with clinical features. Our studies support that proteomic studies of SUDC cohorts can advance our understanding of the pathogenesis of these tragedies and may inform the development of preventive strategies.
10.1007/s00401-022-02414-7
MaxQuant.Live Enables Global Targeting of More Than 25,000 Peptides.
Wichmann Christoph,Meier Florian,Virreira Winter Sebastian,Brunner Andreas-David,Cox Jürgen,Mann Matthias
Molecular & cellular proteomics : MCP
Mass spectrometry (MS)-based proteomics is often performed in a shotgun format, in which as many peptide precursors as possible are selected from full or MS1 scans so that their fragment spectra can be recorded in MS2 scans. Although achieving great proteome depths, shotgun proteomics cannot guarantee that each precursor will be fragmented in each run. In contrast, targeted proteomics aims to reproducibly and sensitively record a restricted number of precursor/fragment combinations in each run, based on prescheduled mass-to-charge and retention time windows. Here we set out to unify these two concepts by a global targeting approach in which an arbitrary number of precursors of interest are detected in real-time, followed by standard fragmentation or advanced peptide-specific analyses. We made use of a fast application programming interface to a quadrupole Orbitrap instrument and real-time recalibration in mass, retention time and intensity dimensions to predict precursor identity. MaxQuant.Live is freely available (www.maxquant.live) and has a graphical user interface to specify many predefined data acquisition strategies. Acquisition speed is as fast as with the vendor software and the power of our approach is demonstrated with the acquisition of breakdown curves for hundreds of precursors of interest. We also uncover precursors that are not even visible in MS1 scans, using elution time prediction based on the auto-adjusted retention time alone. Finally, we successfully recognized and targeted more than 25,000 peptides in single LC-MS runs. Global targeting combines the advantages of two classical approaches in MS-based proteomics, whereas greatly expanding the analytical toolbox. MaxQuant.Live builds on the fast application programming interface of quadrupole Orbitrap mass analyzers to control data acquisition in real-time (freely available at www.maxquant.live). Its graphical user interface enables advanced data acquisition strategies, such as in-depth characterization of peptides of interest. Online recalibration in mass, retention time, and intensity dimensions extends this concept to more than 25,000 peptides per run. Our "global targeting" strategy combines the best of targeted and shotgun approaches.
10.1074/mcp.TIR118.001131
MaxQuant Software for Ion Mobility Enhanced Shotgun Proteomics.
Molecular & cellular proteomics : MCP
Ion mobility can add a dimension to LC-MS based shotgun proteomics which has the potential to boost proteome coverage, quantification accuracy and dynamic range. Required for this is suitable software that extracts the information contained in the four-dimensional (4D) data space spanned by m/z, retention time, ion mobility and signal intensity. Here we describe the ion mobility enhanced MaxQuant software, which utilizes the added data dimension. It offers an end to end computational workflow for the identification and quantification of peptides and proteins in LC-IMS-MS/MS shotgun proteomics data. We apply it to trapped ion mobility spectrometry (TIMS) coupled to a quadrupole time-of-flight (QTOF) analyzer. A highly parallelizable 4D feature detection algorithm extracts peaks which are assembled to isotope patterns. Masses are recalibrated with a non-linear m/z, retention time, ion mobility and signal intensity dependent model, based on peptides from the sample. A new matching between runs (MBR) algorithm that utilizes collisional cross section (CCS) values of MS1 features in the matching process significantly gains specificity from the extra dimension. Prerequisite for using CCS values in MBR is a relative alignment of the ion mobility values between the runs. The missing value problem in protein quantification over many samples is greatly reduced by CCS aware MBR.MS1 level label-free quantification is also implemented which proves to be highly precise and accurate on a benchmark dataset with known ground truth. MaxQuant for LC-IMS-MS/MS is part of the basic MaxQuant release and can be downloaded from http://maxquant.org.
10.1074/mcp.TIR119.001720
MaxQuant and MSstats in Galaxy Enable Reproducible Cloud-Based Analysis of Quantitative Proteomics Experiments for Everyone.
Journal of proteome research
Quantitative mass spectrometry-based proteomics has become a high-throughput technology for the identification and quantification of thousands of proteins in complex biological samples. Two frequently used tools, MaxQuant and MSstats, allow for the analysis of raw data and finding proteins with differential abundance between conditions of interest. To enable accessible and reproducible quantitative proteomics analyses in a cloud environment, we have integrated MaxQuant (including TMTpro 16/18plex), Proteomics Quality Control (PTXQC), MSstats, and MSstatsTMT into the open-source Galaxy framework. This enables the web-based analysis of label-free and isobaric labeling proteomics experiments via Galaxy's graphical user interface on public clouds. MaxQuant and MSstats in Galaxy can be applied in conjunction with thousands of existing Galaxy tools and integrated into standardized, sharable workflows. Galaxy tracks all metadata and intermediate results in analysis histories, which can be shared privately for collaborations or publicly, allowing full reproducibility and transparency of published analysis. To further increase accessibility, we provide detailed hands-on training materials. The integration of MaxQuant and MSstats into the Galaxy framework enables their usage in a reproducible way on accessible large computational infrastructures, hence realizing the foundation for high-throughput proteomics data science for everyone.
10.1021/acs.jproteome.2c00051
Proteomic and transcriptomic profiling of aerial organ development in Arabidopsis.
Mergner Julia,Frejno Martin,Messerer Maxim,Lang Daniel,Samaras Patroklos,Wilhelm Mathias,Mayer Klaus F X,Schwechheimer Claus,Kuster Bernhard
Scientific data
Plant growth and development are regulated by a tightly controlled interplay between cell division, cell expansion and cell differentiation during the entire plant life cycle from seed germination to maturity and seed propagation. To explore some of the underlying molecular mechanisms in more detail, we selected different aerial tissue types of the model plant Arabidopsis thaliana, namely rosette leaf, flower and silique/seed and performed proteomic, phosphoproteomic and transcriptomic analyses of sequential growth stages using tandem mass tag-based mass spectrometry and RNA sequencing. With this exploratory multi-omics dataset, development dynamics of photosynthetic tissues can be investigated from different angles. As expected, we found progressive global expression changes between growth stages for all three omics types and often but not always corresponding expression patterns for individual genes on transcript, protein and phosphorylation site level. The biggest difference between proteomic- and transcriptomic-based expression information could be observed for seed samples. Proteomic and transcriptomic data is available via ProteomeXchange and ArrayExpress with the respective identifiers PXD018814 and E-MTAB-7978.
10.1038/s41597-020-00678-w
Determination of the Time since Deposition of Blood Traces Utilizing a Liquid Chromatography-Mass Spectrometry-Based Proteomics Approach.
Analytical chemistry
Knowledge about when a bloodstain was deposited at a crime scene can be of critical value in forensic investigation. A donor of a genetically identified bloodstain could be linked to a suspected time frame and the crime scene itself. Determination of the time since deposition (TsD) has been extensively studied before but has yet to reach maturity. We therefore conducted a proof-of-principle study to study time- and storage-dependent changes of the proteomes of dried blood stains. A bottom-up proteomics approach was employed, and high-resolution liquid-chromatography-mass-spectrometry (HR-LC-MS) and data-independent acquisition (DIA) were used to analyze samples aged over a 2 month period and two different storage conditions. In multivariate analysis, samples showed distinct clustering according to their TsD in both principal component analysis (PCA) and in partial least square discriminant analysis (PLS DA). The storage condition alters sample aging and yields different separation-driving peptides in hierarchical clustering and in TsD marker peptide selection. Certain peptides and amino acid modifications were identified and further assessed for their applicability in assessing passed TsD. A prediction model based on data resampling (Jackknife) was applied, and prediction values for selected peptide ratios were created. Depending on storage conditions and actual sample age, mean prediction performances ranges in between 70 and 130% for the majority of peptides and time points. This places this study as a first in investigating LC-MS based bottom-up proteomics approaches for TsD determination.
10.1021/acs.analchem.2c01009
Deep Visual Proteomics defines single-cell identity and heterogeneity.
Nature biotechnology
Despite the availabilty of imaging-based and mass-spectrometry-based methods for spatial proteomics, a key challenge remains connecting images with single-cell-resolution protein abundance measurements. Here, we introduce Deep Visual Proteomics (DVP), which combines artificial-intelligence-driven image analysis of cellular phenotypes with automated single-cell or single-nucleus laser microdissection and ultra-high-sensitivity mass spectrometry. DVP links protein abundance to complex cellular or subcellular phenotypes while preserving spatial context. By individually excising nuclei from cell culture, we classified distinct cell states with proteomic profiles defined by known and uncharacterized proteins. In an archived primary melanoma tissue, DVP identified spatially resolved proteome changes as normal melanocytes transition to fully invasive melanoma, revealing pathways that change in a spatial manner as cancer progresses, such as mRNA splicing dysregulation in metastatic vertical growth that coincides with reduced interferon signaling and antigen presentation. The ability of DVP to retain precise spatial proteomic information in the tissue context has implications for the molecular profiling of clinical samples.
10.1038/s41587-022-01302-5
Results of untargeted analysis using the SOMAscan proteomics platform indicates novel associations of circulating proteins with risk of progression to kidney failure in diabetes.
Kidney international
This study applies a large proteomics panel to search for new circulating biomarkers associated with progression to kidney failure in individuals with diabetic kidney disease. Four independent cohorts encompassing 754 individuals with type 1 and type 2 diabetes and early and late diabetic kidney disease were followed to ascertain progression to kidney failure. During ten years of follow-up, 227 of 754 individuals progressed to kidney failure. Using the SOMAscan proteomics platform, we measured baseline concentration of 1129 circulating proteins. In our previous publications, we analyzed 334 of these proteins that were members of specific candidate pathways involved in diabetic kidney disease and found 35 proteins strongly associated with risk of progression to kidney failure. Here, we examined the remaining 795 proteins using an untargeted approach. Of these remaining proteins, 11 were significantly associated with progression to kidney failure. Biological processes previously reported for these proteins were related to neuron development (DLL1, MATN2, NRX1B, KLK8, RTN4R and ROR1) and were implicated in the development of kidney fibrosis (LAYN, DLL1, MAPK11, MATN2, endostatin, and ROR1) in cellular and animal studies. Specific mechanisms that underlie involvement of these proteins in progression of diabetic kidney disease must be further investigated to assess their value as targets for kidney-protective therapies. Using multivariable LASSO regression analysis, five proteins (LAYN, ESAM, DLL1, MAPK11 and endostatin) were found independently associated with risk of progression to kidney failure. Thus, our study identified proteins that may be considered as new candidate prognostic biomarkers to predict risk of progression to kidney failure in diabetic kidney disease. Furthermore, three of these proteins (DLL1, ESAM, and MAPK11) were selected as candidate biomarkers when all SOMAscan results were evaluated.
10.1016/j.kint.2022.04.022
Cracking chromatin with proteomics: From chromatome to histone modifications.
Proteomics
Chromatin is the assembly of genomic DNA and proteins packaged in the nucleus of eukaryotic cells, which together are crucial in regulating a plethora of cellular processes. Histones may be the best known class of protein constituents in chromatin, which are decorated by a range of post-translational modifications to recruit accessory proteins and protein complexes to execute specific functions, ranging from DNA compaction, repair, transcription, and duplication, all in a dynamic fashion and depending on the cellular state. The key role of chromatin in cellular fitness is emphasized by the deregulation of chromatin determinants predisposing to different diseases, including cancer. For this reason, deep investigation of chromatin composition is fundamental to better understand cellular physiology. Proteomic approaches have played a crucial role to understand critical aspects of this complex interplay, benefiting from the ability to identify and quantify proteins and their modifications in an unbiased manner. This review gives an overview of the proteomic approaches that have been developed by combining mass spectrometry-based with tailored biochemical and genetic methods to examine overall protein make-up of chromatin, to characterize chromatin domains, to determine protein interactions, and to decipher the broad spectrum of histone modifications that represent the quintessence of chromatin function.
10.1002/pmic.202100206
A proteomics-MM/PBSA dual approach for the analysis of SARS-CoV-2 main protease substrate peptide specificity.
Peptides
The main protease M of SARS-CoV-2 is a well-studied major drug target. Additionally, it has been linked to this virus' pathogenicity, possibly through off-target effects. It is also an interesting diagnostic target. To obtain more data on possible substrates as well as to assess the enzyme's primary specificity a two-step approach was introduced. First, Terminal Amine Isobaric Labeling of Substrates (TAILS) was employed to identify novel M cleavage sites in a mouse lung proteome library. In a second step, using a structural homology model, the MM/PBSA variant MM/GBSA (Molecular Mechanics Poisson-Boltzmann/Generalized Born Surface Area) free binding energy calculations were carried out to determine relevant interacting amino acids. As a result, 58 unique cleavage sites were detected, including six that displayed glutamine at the P1 position. Furthermore, modeling results indicated that M has a far higher potential promiscuity towards substrates than expected. The combination of proteomics and MM/PBSA modeling analysis can thus be useful for elucidating the specificity of M, and thus open novel perspectives for the development of future peptidomimetic drugs against COVID-19, as well as diagnostic tools.
10.1016/j.peptides.2022.170814
TMT-based quantitative proteomics analysis and potential serum protein biomarkers for systemic lupus erythematosus.
Clinica chimica acta; international journal of clinical chemistry
Systemic lupus erythematosus (SLE) was not only a typical systemic autoimmune disease, but also one of the most challenging heterogeneous diseases for physicians. Currently, the pathogenesis of SLE was unclear, and there were no accurate, universal or easy-to-use diagnostic criteria for assessing SLE activity and predicting SLE severity. Proteins were direct effectors of biological mechanisms, and were closer to clinical phenotypes than the other discovered biomarkers. Moreover, proteins were widely used as biomarkers for clinical diagnosis and mechanism research of many diseases. Herein, we compared the proteins profiles of healthy individuals (HCs) and SLE patients to reveal the pathogenesis and provide evidence for diagnosis and management of persons with SLE. Serum samples were collected from 28 SLE patients and 30 HCs. Tandem mass tag (TMT)-based quantitative proteomics method was used to identify, screen and detect differentially expressed proteins (DEPs) in the collected serum samples. A total of 744 proteins were identified, and 84 of them were considered as DEPs with 71 upregulated and 13 downregulated. Bioinformatics analysis suggested that these DEPs were mainly involved in many biological processes, including immune response, signal transduction, inflammatory response, proteolysis, innate immune response and apoptosis, which were closely related to the pathogenesis of SLE. After comprehensive analysis, serum amyloid A1 (SAA1) and endothelin (CD248) were identified as specific biomarkers for the diagnosis of SLE, and were confirmed by subsequent enzyme-linked immunosorbent assays (ELISA), indicating a high reliability of TMT-based quantitative proteomics results. Areas under the ROC curve (AUC) results confirmed that SAA1 and CD248 combination as early immune diagnosis biomarkers of SLE presented excellent sensitivity and specificity.
10.1016/j.cca.2022.06.031
Protein Adsorption Loss─The Bottleneck of Single-Cell Proteomics.
Journal of proteome research
Single-cell proteomics is a promising field to provide direct yet comprehensive molecular insights into cellular functions without averaging effects. Here, we address a grand technical challenge impeding the maturation of single-cell proteomics─protein adsorption loss (PAL). Even though widely known, there is currently no quantitation on how profoundly and selectively PAL has affected single-cell proteomics. Therefore, the mitigations to this challenge have been generic, and their efficacy was only evaluated by the size of the resolved proteome with no specificity on individual proteins. We use the existing knowledge of PAL, protein expression, and the typical surface area used in single-cell proteomics to discuss the severity of protein loss. We also summarize the current solutions to this challenge and briefly review the available methods to characterize the physical and chemical properties of protein surface adsorption. By citing successful strategies in single-cell genomics for measurement errors in individual transcripts, we pinpoint the urgency to benchmark PAL at the proteome scale with individual protein resolution. Finally, orthogonal single-cell proteomic techniques that have the potential to cross validate PAL are proposed. We hope these efforts can promote the fruition of single-cell proteomics in the near future.
10.1021/acs.jproteome.2c00317
Proteomics and Biochemical Analyses of Secreted Proteins Revealed a Novel Mechanism by Which ADAM12S Regulates the Migration of Gastric Cancer Cells.
Journal of proteome research
Gastric cancer is one of the cancers with the highest morbidity and mortality. Although several therapeutic approaches have been developed to treat this disease, the overall survival rate is still very low due to metastasis, drug resistance, and so forth. Therefore, it is necessary to discover new regulatory molecules and signaling pathways that modulate the metastasis of gastric cancer cells. A Disintegrin And Metalloprotease 12 (ADAM12) was highly expressed in gastric cancer tissues and presented in the patient urine. However, it is unclear whether and how ADAM12 regulates the migration of gastric cancer cells. In this work, we used the secretome protein enrichment with click sugars (SPECS) method to purify the secreted glycosylated proteins and performed quantitative proteomics to identify the secreted proteins that were differentially regulated by ADAM12S, the short and secreted form of ADAM12. Our proteomic and biochemical analyses revealed that ADAM12S upregulated the cell surface glycoprotein CD146, a cell adhesion molecule and melanoma marker, which was dependent on the catalytic residue of ADAM12S. Furthermore, we discovered that the ADAM12S-enhanced migration of gastric cancer cells was, at least partially, mediated by CD146. This work may help to evaluate whether ADAM12 could be a potential therapeutic target for the treatment of gastric cancer patients.
10.1021/acs.jproteome.2c00221
Proteomics analysis of lysine crotonylation and 2-hydroxyisobutyrylation reveals significant features of systemic lupus erythematosus.
Clinical rheumatology
INTRODUCTION/OBJECTIVES:To seek significant features of systemic lupus erythematosus (SLE) by utilizing bioinformatics analysis. METHOD:Liquid chromatography-tandem mass spectrometry (LC-MS/MS) was used to quantify lysine crotonylation (Kcr) and lysine 2-hydroxyisobutyrylation (Khib) in peripheral blood mononuclear cells (PBMCs) of systemic lupus erythematosus (SLE) patients and normal controls. RESULTS:Seventy-six differentially modified proteins (DMPs) dually modified by Kcr and Khib were identified between SLE patients and healthy people. GO enrichment analysis prompted significant enrichment of seventy-six DMPs in MHC class II protein complex binding and leukocyte migration. KEGG pathways were enriched in antigen processing and presentation pathway and leukocyte transendothelial migration pathway. Six DMPs (CLTC, HSPA1B, HSPA8, HSP90AB1, HSPD1, and PDIA3) were identified in antigen processing and presentation pathway, of which HSPA8 was the core protein. Significant changes of Kcr and Khib in HSPA8 may increase ATP hydrolysis and promote antigen binding to MHC II molecule. In leukocyte transendothelial migration pathway, 7 DMPs (ACTN1, ACTN4, EZR, MSN, RAC1, RHOA, and VCL) were identified. MSN was the protein with the most modification sites in this pathway. In amino terminal ferm region of MSN, Kcr and Khib expression change may lead to the adhesion between leukocytes and endothelial cells, which was an important step of leukocyte migration. CONCLUSION:Kcr and Khib may promote the antigen presentation and jointly regulate the tissue damage mediated by leukocyte migration in SLE patients, which may play key roles in the pathogenesis of SLE probably. Key Points • Antigen processing and presentation and leukocyte transendothelial migration may play key roles in the pathogenesis of SLE.
10.1007/s10067-022-06254-4
Heart failure in obesity: insights from proteomics in patients treated with or without weight-loss surgery.
International journal of obesity (2005)
BACKGROUND:Obesity is associated with incident heart failure (HF), but the underlying mechanisms are unclear. METHODS:We performed a nested case-control study within the Swedish-Obese-Subjects study, by identifying 411 cases who developed HF and matched them with respect to age, sex, weight-loss-surgery and length of follow-up with 410 controls who did not develop HF. In analyses corrected for multiple testing, we studied 182 plasma proteins known to be related to cardiovascular disease to investigate whether they could add to the understanding of the processes underlying obesity-related HF. RESULTS:A total of 821 subjects were followed for 16 ± 6 years. Multivariable analysis adjusted for matching variables revealed that 32 proteins were significantly associated with HF. Twelve proteins were related to HF ≥ 80% of the time using a bootstrap resampling approach (false-discovery-rate [FDR] < 0.05): 11 were associated with increased HF-risk: TNFRSF10A*, ST6GAL1, PRCP, MMP12, TIMP1, CCL3, QPCT, ANG, C1QTNF1, SERPINA5 and GAL-9; and one was related to reduced HF-risk: LPL. An further 20 proteins were associated with onset of HF 50-80% of the time using bootstrap resampling (FDR < 0.05). A pathway analysis including all significant 32 proteins suggested that these biomarkers were related to inflammation, matrix remodeling, cardiometabolic hormones and hemostasis. Three proteins, C1QTNF1, FGF-21 and CST3, reflecting dyslipidemia and kidney disease, displayed a higher association with HF in patients who did not undergo weight-loss-surgery and maintained with obesity. CONCLUSION:Pathways associated with HF in obesity include inflammation, matrix remodeling, cardiometabolic hormones and hemostasis; three protein biomarkers predicting HF appeared to be obesity-specific.
10.1038/s41366-022-01194-0
Serum proteomics of severe fever with thrombocytopenia syndrome patients.
Clinical proteomics
BACKGROUND:Dabie bandavirus, also termed as severe fever with thrombocytopenia syndrome virus (SFTSV), was first isolated in China in 2010. At this time, the virus was found to have spread to South Korea, Japan, and other countries. A high case fatality rate is reported for SFTS, ranging from 12-50% within various sources. Several omics for clinical studies among SFTS patients as well as studies of cultured SFTSV have attempted to characterize the relevant molecular biology and epidemiology of the disease. However, a global serum proteomics analysis among SFTS patients has not yet been reported to date. METHODS:In the current study, we evaluated comparative serum proteomics among SFTS patients (eight recovered patients and three deceased patients) with the goal of identifying the protein expression patterns associated with the clinical manifestations of SFTS. RESULTS:The proteomic results in the current study showed that the coagulation factor proteins, protein S and protein C, were statistically significantly downregulated among the deceased patients. Downregulation of the complement system as well as prolonged neutrophil activation were also observed. Additionally, the downstream proteins of tumour necrosis factor alpha, neutrophil-activating cytokine, and interleukin-1β, an inflammatory cytokine, were overexpressed. CONCLUSIONS:Thrombocytopenia and multiple organ failure are the major immediate causes of death among SFTS patients. In this study, serum proteomic changes related to thrombocytopenia, abnormal immune response, and inflammatory activation were documented in SFTS patients. These findings provide useful information for understanding the clinical manifestations of SFTS.
10.1186/s12014-022-09368-8
The Brain Protein Atlas: A conglomerate of proteomics datasets of human neural tissue.
Proteomics
The human brain represents one of the most complex biological structures with significant spatiotemporal molecular plasticity occurring through early development, learning, aging, and disease. While much progress has been made in mapping its transcriptional architecture, more downstream phenotypic readouts are relatively scarce due to limitations with tissue heterogeneity and accessibility, as well as an inability to amplify protein species prior to global -OMICS analysis. To address some of these barriers, our group has recently focused on using mass-spectrometry workflows compatible with small amounts of formalin-fixed paraffin-embedded tissue samples. This has enabled exploration into spatiotemporal proteomic signatures of the brain and disease across otherwise inaccessible neurodevelopmental timepoints and anatomical niches. Given the similar theme and approaches, we introduce an integrated online portal, "The Brain Protein Atlas (BPA)" (www.brainproteinatlas.org), representing a public resource that allows users to access and explore these amalgamated datasets. Specifically, this portal contains a growing set of peer-reviewed mass-spectrometry-based proteomic datasets, including spatiotemporal profiles of human cerebral development, diffuse gliomas, clinically aggressive meningiomas, and a detailed anatomic atlas of glioblastoma. One barrier to entry in mass spectrometry-based proteomics data analysis is the steep learning curve required to extract biologically relevant data. BPA, therefore, includes several built-in analytical tools to generate relevant plots (e.g., volcano plots, heatmaps, boxplots, and scatter plots) and evaluate the spatiotemporal patterns of proteins of interest. Future iterations aim to expand available datasets, including those generated by the community at large, and analytical tools for exploration. Ultimately, BPA aims to improve knowledge dissemination of proteomic information across the neuroscience community in hopes of accelerating the biological understanding of the brain and various maladies.
10.1002/pmic.202200127
Single-cell transcriptomics and cell-specific proteomics reveals molecular signatures of sleep.
Communications biology
Every day, we sleep for a third of the day. Sleep is important for cognition, brain waste clearance, metabolism, and immune responses. The molecular mechanisms governing sleep are largely unknown. Here, we used a combination of single-cell RNA sequencing and cell-type-specific proteomics to interrogate the molecular underpinnings of sleep. Different cell types in three important brain regions for sleep (brainstem, cortex, and hypothalamus) exhibited diverse transcriptional responses to sleep need. Sleep restriction modulates astrocyte-neuron crosstalk and sleep need enhances expression of specific sets of transcription factors in different brain regions. In cortex, we also interrogated the proteome of two major cell types: astrocytes and neurons. Sleep deprivation differentially alters the expression of proteins in astrocytes and neurons. Similarly, phosphoproteomics revealed large shifts in cell-type-specific protein phosphorylation. Our results indicate that sleep need regulates transcriptional, translational, and post-translational responses in a cell-specific manner.
10.1038/s42003-022-03800-3
Exploring direct and indirect targets of current antileishmanial drugs using a novel thermal proteomics profiling approach.
Frontiers in cellular and infection microbiology
Visceral leishmaniasis (VL), caused by , is an oft-fatal neglected tropical disease. In the absence of an effective vaccine, the control of leishmaniasis relies exclusively on chemotherapy. Due to the lack of established molecular/genetic markers denoting parasite resistance, clinical treatment failure is often used as an indicator. Antimony-based drugs have been the standard antileishmanial treatment for more than seven decades, leading to major drug resistance in certain regions. Likewise, drug resistance to miltefosine and amphotericin B continues to spread at alarming rates. In consequence, innovative approaches are needed to accelerate the identification of antimicrobial drug targets and resistance mechanisms. To this end, we have implemented a novel approach based on thermal proteome profiling (TPP) to further characterize the mode of action of antileishmanials antimony, miltefosine and amphotericin B, as well as to better understand the mechanisms of drug resistance deployed by . Proteins become more resistant to heat-induced denaturation when complexed with a ligand. In this way, we used multiplexed quantitative mass spectrometry-based proteomics to monitor the melting profile of thousands of expressed soluble proteins in WT, antimony-resistant, miltefosine-resistant, and amphotericin B-resistant parasites, in the presence (or absence) of the above-mentioned drugs. Bioinformatics analyses were performed, including data normalization, melting profile fitting, and identification of proteins that underwent changes (fold change > 4) caused by complexation with a drug. With this unique approach, we were able to narrow down the regions of the proteome that interact with antimony, miltefosine, and amphotericin B; validating previously-identified and unveiling novel drug targets. Moreover, analyses revealed candidate proteins potentially involved in drug resistance. Interestingly, we detected thermal proximity coaggregation for several proteins belonging to the same metabolic pathway (i.e., tryparedoxin peroxidase and aspartate aminotransferase in proteins exposed to antimony), highlighting the importance of these pathways. Collectively, our results could serve as a jumping-off point for the future development of innovative diagnostic tools for the detection and evaluation of antimicrobial-resistant populations, as well as open the door for new on-target therapies.
10.3389/fcimb.2022.954144
Proteomic Investigation of the Antibacterial Mechanism of Cefiderocol against Escherichia coli.
Microbiology spectrum
This study aimed to investigate the antibacterial mechanism of cefiderocol (CFDC) using data-independent acquisition quantitative proteomics combined with cellular and molecular biological assays. Numerous differentially expressed proteins related to the production of NADH, reduced cofactor flavin adenine dinucleotide (FADH), NADPH and reactive oxygen species (ROS), iron-sulfur cluster binding, and iron ion homeostasis were found to be upregulated by CFDC. Furthermore, parallel reaction monitoring analysis validated these results. Meanwhile, we confirmed that the levels of NADH, ROS, HO, and iron ions were induced by CFDC, and the sensitivity of Escherichia coli to CFDC was inhibited by the antioxidant vitamin C, -acetyl-l-cysteine, and deferoxamine. Moreover, deferoxamine also suppressed the HO stress induced by CFDC. In addition, knockout of the NADH-quinone oxidoreductase genes (, , , , , , , ) in the respiratory chain attenuated the sensitivity of E. coli to CFDC far beyond the effects of cefepime and ceftazidime; in particular, the E. coli BW25113 Δ strain produced 60-fold increases in MIC to CFDC compared to that of the wild-type E. coli BW25113 strain. The present study revealed that CFDC exerts its antibacterial effects by inducing ROS stress by elevating the levels of NADH and iron ions in E. coli. CFDC was the first FDA-approved siderophore cephalosporin antibiotic in 2019 and is known for its Trojan horse tactics and broad antimicrobial activity against Gram-negative bacteria. However, its antibacterial mechanism is not fully understood, and whether it has an impact on iron ion homeostasis remains unknown. To comprehensively reveal the antibacterial mechanisms of CFDC, data-independent acquisition quantitative proteomics combined with cellular and molecular biological assays were performed in this study. The findings will further facilitate our understanding of the antibacterial mechanism of CFDC and may provide a theoretical foundation for controlling CFDC resistance in the future.
10.1128/spectrum.01093-22
Enhanced Competition at the Nano-Bio Interface Enables Comprehensive Characterization of Protein Corona Dynamics and Deep Coverage of Proteomes.
Advanced materials (Deerfield Beach, Fla.)
Introducing engineered nanoparticles (NPs) into a biofluid such as blood plasma leads to the formation of a selective and reproducible protein corona at the particle-protein interface, driven by the relationship between protein-NP affinity and protein abundance. This enables scalable systems that leverage protein-nano interactions to overcome current limitations of deep plasma proteomics in large cohorts. Here the importance of the protein to NP-surface ratio (P/NP) is demonstrated and protein corona formation dynamics are modeled, which determine the competition between proteins for binding. Tuning the P/NP ratio significantly modulates the protein corona composition, enhancing depth and precision of a fully automated NP-based deep proteomic workflow (Proteograph). By increasing the binding competition on engineered NPs, 1.2-1.7× more proteins with 1% false discovery rate are identified on the surface of each NP, and up to 3× more proteins compared to a standard plasma proteomics workflow. Moreover, the data suggest P/NP plays a significant role in determining the in vivo fate of nanomaterials in biomedical applications. Together, the study showcases the importance of P/NP as a key design element for biomaterials and nanomedicine in vivo and as a powerful tuning strategy for accurate, large-scale NP-based deep proteomic studies.
10.1002/adma.202206008
Increasing cell size remodels the proteome and promotes senescence.
Molecular cell
Cell size is tightly controlled in healthy tissues, but it is unclear how deviations in cell size affect cell physiology. To address this, we measured how the cell's proteome changes with increasing cell size. Size-dependent protein concentration changes are widespread and predicted by subcellular localization, size-dependent mRNA concentrations, and protein turnover. As proliferating cells grow larger, concentration changes typically associated with cellular senescence are increasingly pronounced, suggesting that large size may be a cause rather than just a consequence of cell senescence. Consistent with this hypothesis, larger cells are prone to replicative, DNA-damage-induced, and CDK4/6i-induced senescence. Size-dependent changes to the proteome, including those associated with senescence, are not observed when an increase in cell size is accompanied by an increase in ploidy. Together, our findings show how cell size could impact many aspects of cell physiology by remodeling the proteome and provide a rationale for cell size control and polyploidization.
10.1016/j.molcel.2022.07.017
Integration of transcriptomics, proteomics, and metabolomics data to reveal HER2-associated metabolic heterogeneity in gastric cancer with response to immunotherapy and neoadjuvant chemotherapy.
Frontiers in immunology
Background:Currently available prognostic tools and focused therapeutic methods result in unsatisfactory treatment of gastric cancer (GC). A deeper understanding of human epidermal growth factor receptor 2 (HER2)-coexpressed metabolic pathways may offer novel insights into tumour-intrinsic precision medicine. Methods:The integrated multi-omics strategies (including transcriptomics, proteomics and metabolomics) were applied to develop a novel metabolic classifier for gastric cancer. We integrated TCGA-STAD cohort (375 GC samples and 56753 genes) and TCPA-STAD cohort (392 GC samples and 218 proteins), and rated them as transcriptomics and proteomics data, resepectively. 224 matched blood samples of GC patients and healthy individuals were collected to carry out untargeted metabolomics analysis. Results:In this study, pan-cancer analysis highlighted the crucial role of ERBB2 in the immune microenvironment and metabolic remodelling. In addition, the metabolic landscape of GC indicated that alanine, aspartate and glutamate (AAG) metabolism was significantly associated with the prevalence and progression of GC. Weighted metabolite correlation network analysis revealed that glycolysis/gluconeogenesis (GG) and AAG metabolism served as HER2-coexpressed metabolic pathways. Consensus clustering was used to stratify patients with GC into four subtypes with different metabolic characteristics (i.e. quiescent, GG, AAG and mixed subtypes). The GG subtype was characterised by a lower level of ERBB2 expression, a higher proportion of the inflammatory phenotype and the worst prognosis. However, contradictory features were found in the mixed subtype with the best prognosis. The GG and mixed subtypes were found to be highly sensitive to chemotherapy, whereas the quiescent and AAG subtypes were more likely to benefit from immunotherapy. Conclusions:Transcriptomic and proteomic analyses highlighted the close association of HER-2 level with the immune status and metabolic features of patients with GC. Metabolomics analysis highlighted the co-expressed relationship between alanine, aspartate and glutamate and glycolysis/gluconeogenesis metabolisms and HER2 level in GC. The novel integrated multi-omics strategy used in this study may facilitate the development of a more tailored approach to GC therapy.
10.3389/fimmu.2022.951137
Advanced Cell Mapping Visualizations for Single Cell Functional Proteomics Enabling Patient Stratification.
Proteomics
Highly multiplexed single-cell functional proteomics has emerged as one of the next-generation toolkits for a deeper understanding of functional heterogeneity in cell. Different from the conventional population-based bulk and single-cell RNA-Seq assays, the microchip-based proteomics at the single-cell resolution enables a unique identification of highly polyfunctional cell subsets that co-secrete many proteins from live single cells and most importantly correlate with patient response to a therapy. The 32-plex IsoCode chip technology has defined a polyfunctional strength index (PSI) of pre-infusion anti-CD19 chimeric antigen receptor (CAR)-T products, that is significantly associated with patient response to the CAR-T cell therapy. To complement the clinical relevance of the PSI, a comprehensive visualization toolkit of 3D uniform manifold approximation and projection (UMAP) and t-distributed stochastic neighbor embedding (t-SNE) in a proteomic analysis pipeline is developed, providing more advanced analytical algorithms for more intuitive data visualizations. The UMAP and t-SNE of anti-CD19 CAR-T products reveal distinct cytokine profiles between nonresponders and responders and demonstrate a marked upregulation of antitumor-associated cytokine signatures in CAR-T cells from responding patients. Using this powerful while user-friendly analytical tool, the multi-dimensional single-cell data can be dissected from complex immune responses and uncover underlying mechanisms, which can promote correlative biomarker discovery, improved bioprocessing, and personalized treatment development.
10.1002/pmic.201900270
Data-Independent Acquisition Mass Spectrometry-Based Proteomics and Software Tools: A Glimpse in 2020.
Zhang Fangfei,Ge Weigang,Ruan Guan,Cai Xue,Guo Tiannan
Proteomics
This review provides a brief overview of the development of data-independent acquisition (DIA) mass spectrometry-based proteomics and selected DIA data analysis tools. Various DIA acquisition schemes for proteomics are summarized first including Shotgun-CID, DIA, MS , PAcIFIC, AIF, SWATH, MSX, SONAR, WiSIM, BoxCar, Scanning SWATH, diaPASEF, and PulseDIA, as well as the mass spectrometers enabling these methods. Next, the software tools for DIA data analysis are classified into three groups: library-based tools, library-free tools, and statistical validation tools. The approaches are reviewed for generating spectral libraries for six selected library-based DIA data analysis software tools which are tested by the authors, including OpenSWATH, Spectronaut, Skyline, PeakView, DIA-NN, and EncyclopeDIA. An increasing number of library-free DIA data analysis tools are developed including DIA-Umpire, Group-DIA, PECAN, PEAKS, which facilitate identification of novel proteoforms. The authors share their user experience of when to use DIA-MS, and several selected DIA data analysis software tools. Finally, the state of the art DIA mass spectrometry and software tools, and the authors' views of future directions are summarized.
10.1002/pmic.201900276
Moving Profiling Spatial Proteomics Beyond Discrete Classification.
Proteomics
The spatial subcellular proteome is a dynamic environment; one that can be perturbed by molecular cues and regulated by post-translational modifications. Compartmentalization of this environment and management of these biomolecular dynamics allows for an array of ancillary protein functions. Profiling spatial proteomics has proved to be a powerful technique in identifying the primary subcellular localization of proteins. The approach has also been refashioned to study multi-localization and localization dynamics. Here, the analytical approaches that have been applied to spatial proteomics thus far are critiqued, and challenges particularly associated with multi-localization and dynamic relocalization is identified. To meet some of the current limitations in analytical processing, it is suggested that Bayesian modeling has clear benefits over the methods applied to date and should be favored whenever possible. Careful consideration of the limitations and challenges, and development of robust statistical frameworks, will ensure that profiling spatial proteomics remains a valuable technique as its utility is expanded.
10.1002/pmic.201900392
Targeted and Untargeted Proteomics Approaches in Biomarker Development.
Sobsey Constance A,Ibrahim Sahar,Richard Vincent R,Gaspar Vanessa,Mitsa Georgia,Lacasse Vincent,Zahedi René P,Batist Gerald,Borchers Christoph H
Proteomics
An enormous amount of research effort has been devoted to biomarker discovery and validation. With the completion of the human genome, proteomics is now playing an increasing role in this search for new and better biomarkers. Here, what leads to successful biomarker development is reviewed and how these features may be applied in the context of proteomic biomarker research is considered. The "fit-for-purpose" approach to biomarker development suggests that untargeted proteomic approaches may be better suited for early stages of biomarker discovery, while targeted approaches are preferred for validation and implementation. A systematic screening of published biomarker articles using MS-based proteomics reveals that while both targeted and untargeted technologies are used in proteomic biomarker development, most researchers do not combine these approaches. i) The reasons for this discrepancy, (ii) how proteomic technologies can overcome technical challenges that seem to limit their translation into the clinic, and (iii) how MS can improve, complement, or replace existing clinically important assays in the future are discussed.
10.1002/pmic.201900029
pseudoQC: A Regression-Based Simulation Software for Correction and Normalization of Complex Metabolomics and Proteomics Datasets.
Wang Shisheng,Yang Hao
Proteomics
Various types of unwanted and uncontrollable signal variations in MS-based metabolomics and proteomics datasets severely disturb the accuracies of metabolite and protein profiling. Therefore, pooled quality control (QC) samples are often employed in quality management processes, which are indispensable to the success of metabolomics and proteomics experiments, especially in high-throughput cases and long-term projects. However, data consistency and QC sample stability are still difficult to guarantee because of the experimental operation complexity and differences between experimenters. To make things worse, numerous proteomics projects do not take QC samples into consideration at the beginning of experimental design. Herein, a powerful and interactive web-based software, named pseudoQC, is presented to simulate QC sample data for actual metabolomics and proteomics datasets using four different machine learning-based regression methods. The simulated data are used for correction and normalization of the two published datasets, and the obtained results suggest that nonlinear regression methods perform better than linear ones. Additionally, the above software is available as a web-based graphical user interface and can be utilized by scientists without a bioinformatics background. pseudoQC is open-source software and freely available at https://www.omicsolution.org/wukong/pseudoQC/.
10.1002/pmic.201900264
Scalable Data Analysis in Proteomics and Metabolomics Using BioContainers and Workflows Engines.
Proteomics
The recent improvements in mass spectrometry instruments and new analytical methods are increasing the intersection between proteomics and big data science. In addition, bioinformatics analysis is becoming increasingly complex and convoluted, involving multiple algorithms and tools. A wide variety of methods and software tools have been developed for computational proteomics and metabolomics during recent years, and this trend is likely to continue. However, most of the computational proteomics and metabolomics tools are designed as single-tiered software application where the analytics tasks cannot be distributed, limiting the scalability and reproducibility of the data analysis. In this paper the key steps of metabolomics and proteomics data processing, including the main tools and software used to perform the data analysis, are summarized. The combination of software containers with workflows environments for large-scale metabolomics and proteomics analysis is discussed. Finally, a new approach for reproducible and large-scale data analysis based on BioContainers and two of the most popular workflow environments, Galaxy and Nextflow, is introduced to the proteomics and metabolomics communities.
10.1002/pmic.201900147
A field guide to the proteomics of post-translational modifications in DNA repair.
Proteomics
All cells incur DNA damage from exogenous and endogenous sources and possess pathways to detect and repair DNA damage. Post-translational modifications (PTMs), in the past 20 years, have risen to ineluctable importance in the study of the regulation of DNA repair mechanisms. For example, DNA damage response kinases are critical in both the initial sensing of DNA damage as well as in orchestrating downstream activities of DNA repair factors. Mass spectrometry-based proteomics revolutionized the study of the role of PTMs in the DNA damage response and has canonized PTMs as central modulators of nearly all aspects of DNA damage signaling and repair. This review provides a biologist-friendly guide for the mass spectrometry analysis of PTMs in the context of DNA repair and DNA damage responses. We reflect on the current state of proteomics for exploring new mechanisms of PTM-based regulation and outline a roadmap for designing PTM mapping experiments that focus on the DNA repair and DNA damage responses.
10.1002/pmic.202200064
A practical guide to interpreting and generating bottom-up proteomics data visualizations.
Proteomics
Mass-spectrometry based bottom-up proteomics is the main method to analyze proteomes comprehensively and the rapid evolution of instrumentation and data analysis has made the technology widely available. Data visualization is an integral part of the analysis process and it is crucial for the communication of results. This is a major challenge due to the immense complexity of MS data. In this review, we provide an overview of commonly used visualizations, starting with raw data of traditional and novel MS technologies, then basic peptide and protein level analyses, and finally visualization of highly complex datasets and networks. We specifically provide guidance on how to critically interpret and discuss the multitude of different proteomics data visualizations. Furthermore, we highlight Python-based libraries and other open science tools that can be applied for independent and transparent generation of customized visualizations. To further encourage programmatic data visualization, we provide the Python code used to generate all data figures in this review on GitHub (https://github.com/MannLabs/ProteomicsVisualization).
10.1002/pmic.202100103
Targeted proteomics on its way to discovery.
Proteomics
For a long time, targeted and discovery proteomics covered different corners of the detection spectrum, with targeted proteomics focused on small target sets. This changed with the recent advances in highly multiplexed analysis. While discovery proteomics still pushes higher numbers of identified and quantified proteins, the advances in targeted proteomics rose to cover large parts of less complex proteomes or proteomes with low protein detection counts due to dynamic range restrictions, like the blood proteome. These new developments will impact, especially on the field of biomarker discovery and the possibility of using targeted proteomics for diagnostic purposes.
10.1002/pmic.202100330
Proteomics of human biological fluids for biomarker discoveries: technical advances and recent applications.
Expert review of proteomics
INTRODUCTION:Biological fluids are routine samples for diagnostic testing and monitoring. Blood samples are typically measured because of their moderate invasive collection and high information content on health and disease. Several body fluids, such as cerebrospinal fluid (CSF), are also studied and suited to specific pathologies. Over the last two decades, proteomics has quested to identify protein biomarkers but with limited success. Recent technologies and refined pipelines have accelerated the profiling of human biological fluids. AREAS COVERED:We review proteomic technologies for the identification of biomarkers. These are based on antibodies/aptamers arrays or mass spectrometry (MS), but new ones are emerging. Advances in scalability and throughput have allowed to better design studies and cope with the limited sample size that has until now prevailed due to technological constraints. With these enablers, plasma/serum, CSF, saliva, tears, urine, and milk proteomes have been further profiled; we provide a non-exhaustive picture of some recent highlights (mainly covering literature from the last 5 years in the Scopus database) using MS-based proteomics. EXPERT OPINION:While proteomics has been in the shadow of genomics for years, proteomic tools and methodologies have reached certain maturity. They are now better suited to discover innovative and robust biofluid biomarkers.
10.1080/14789450.2022.2070477
Triple extraction method enables high quality mass spectrometry-based proteomics and phospho-proteomics for eventual multi-omics integration studies.
Sanchez-Quiles Virginia,Shi Ming-Jun,Dingli Florent,Krucker Clémentine,Loew Damarys,Bernard-Pierrot Isabelle,Radvanyi François
Proteomics
Large-scale multi-omic analysis allows a thorough understanding of different physiological or pathological conditions, particularly cancer. Here, an extraction method simultaneously yielding DNA, RNA and protein (thereby referred to as "triple extraction", TEx) was tested for its suitability to unbiased, system-wide proteomic investigation. Largely proven efficient for transcriptomic and genomic studies, we aimed at exploring TEx compatibility with mass spectrometry-based proteomics and phospho-proteomics, as compared to a standard urea extraction. TEx is suitable for the shotgun investigation of proteomes, providing similar results as urea-based protocol both at the qualitative and quantitative levels. TEx is likewise compatible with the exploration of phosphorylation events, actually providing a higher number of correctly localized sites than urea, although the nature of extracted modifications appears somewhat distinct between both techniques. These results highlight that the presented protocol is well suited for the examination of the proteome and modified proteome of this bladder cancer cell model, as efficiently as other more widely used workflows for mass spectrometry-based analysis. Potentially applicable to other mammalian cell types and tissues, TEx represents an advantageous strategy for multi-omics on scarce and/or heterogenous samples.
10.1002/pmic.202000303
Pan-cancer proteomic map of 949 human cell lines.
Cancer cell
The proteome provides unique insights into disease biology beyond the genome and transcriptome. A lack of large proteomic datasets has restricted the identification of new cancer biomarkers. Here, proteomes of 949 cancer cell lines across 28 tissue types are analyzed by mass spectrometry. Deploying a workflow to quantify 8,498 proteins, these data capture evidence of cell-type and post-transcriptional modifications. Integrating multi-omics, drug response, and CRISPR-Cas9 gene essentiality screens with a deep learning-based pipeline reveals thousands of protein biomarkers of cancer vulnerabilities that are not significant at the transcript level. The power of the proteome to predict drug response is very similar to that of the transcriptome. Further, random downsampling to only 1,500 proteins has limited impact on predictive power, consistent with protein networks being highly connected and co-regulated. This pan-cancer proteomic map (ProCan-DepMapSanger) is a comprehensive resource available at https://cellmodelpassports.sanger.ac.uk.
10.1016/j.ccell.2022.06.010
Proteomic analysis reveals key differences between squamous cell carcinomas and adenocarcinomas across multiple tissues.
Nature communications
Squamous cell carcinoma (SCC) and adenocarcinoma (AC) are two main histological subtypes of solid cancer; however, SCCs are derived from different organs with similar morphologies, and it is challenging to distinguish the origin of metastatic SCCs. Here we report a deep proteomic analysis of 333 SCCs of 17 organs and 69 ACs of 7 organs. Proteomic comparison between SCCs and ACs identifies distinguishable pivotal pathways and molecules in those pathways play consistent adverse or opposite prognostic roles in ACs and SCCs. A comparison between common and rare SCCs highlights lipid metabolism may reinforce the malignancy of rare SCCs. Proteomic clusters reveal anatomical features, and kinase-transcription factor networks indicate differential SCC characteristics, while immune subtyping reveals diverse tumor microenvironments across and within diagnoses and identified potential druggable targets. Furthermore, tumor-specific proteins provide candidates with differentially diagnostic values. This proteomics architecture represents a public resource for researchers seeking a better understanding of SCCs and ACs.
10.1038/s41467-022-31719-0
Undulating changes in human plasma proteome profiles across the lifespan.
Nature medicine
Aging is a predominant risk factor for several chronic diseases that limit healthspan. Mechanisms of aging are thus increasingly recognized as potential therapeutic targets. Blood from young mice reverses aspects of aging and disease across multiple tissues, which supports a hypothesis that age-related molecular changes in blood could provide new insights into age-related disease biology. We measured 2,925 plasma proteins from 4,263 young adults to nonagenarians (18-95 years old) and developed a new bioinformatics approach that uncovered marked non-linear alterations in the human plasma proteome with age. Waves of changes in the proteome in the fourth, seventh and eighth decades of life reflected distinct biological pathways and revealed differential associations with the genome and proteome of age-related diseases and phenotypic traits. This new approach to the study of aging led to the identification of unexpected signatures and pathways that might offer potential targets for age-related diseases.
10.1038/s41591-019-0673-2
Pan-cancer molecular subtypes revealed by mass-spectrometry-based proteomic characterization of more than 500 human cancers.
Chen Fengju,Chandrashekar Darshan S,Varambally Sooryanarayana,Creighton Chad J
Nature communications
Mass-spectrometry-based proteomic profiling of human cancers has the potential for pan-cancer analyses to identify molecular subtypes and associated pathway features that might be otherwise missed using transcriptomics. Here, we classify 532 cancers, representing six tissue-based types (breast, colon, ovarian, renal, uterine), into ten proteome-based, pan-cancer subtypes that cut across tumor lineages. The proteome-based subtypes are observable in external cancer proteomic datasets surveyed. Gene signatures of oncogenic or metabolic pathways can further distinguish between the subtypes. Two distinct subtypes both involve the immune system, one associated with the adaptive immune response and T-cell activation, and the other associated with the humoral immune response. Two additional subtypes each involve the tumor stroma, one of these including the collagen VI interacting network. Three additional proteome-based subtypes-respectively involving proteins related to Golgi apparatus, hemoglobin complex, and endoplasmic reticulum-were not reflected in previous transcriptomics analyses. A data portal is available at UALCAN website.
10.1038/s41467-019-13528-0
A large peptidome dataset improves HLA class I epitope prediction across most of the human population.
Nature biotechnology
Prediction of HLA epitopes is important for the development of cancer immunotherapies and vaccines. However, current prediction algorithms have limited predictive power, in part because they were not trained on high-quality epitope datasets covering a broad range of HLA alleles. To enable prediction of endogenous HLA class I-associated peptides across a large fraction of the human population, we used mass spectrometry to profile >185,000 peptides eluted from 95 HLA-A, -B, -C and -G mono-allelic cell lines. We identified canonical peptide motifs per HLA allele, unique and shared binding submotifs across alleles and distinct motifs associated with different peptide lengths. By integrating these data with transcript abundance and peptide processing, we developed HLAthena, providing allele-and-length-specific and pan-allele-pan-length prediction models for endogenous peptide presentation. These models predicted endogenous HLA class I-associated ligands with 1.5-fold improvement in positive predictive value compared with existing tools and correctly identified >75% of HLA-bound peptides that were observed experimentally in 11 patient-derived tumor cell lines.
10.1038/s41587-019-0322-9
Unbiased spatial proteomics with single-cell resolution in tissues.
Molecular cell
Mass spectrometry (MS)-based proteomics has become a powerful technology to quantify the entire complement of proteins in cells or tissues. Here, we review challenges and recent advances in the LC-MS-based analysis of minute protein amounts, down to the level of single cells. Application of this technology revealed that single-cell transcriptomes are dominated by stochastic noise due to the very low number of transcripts per cell, whereas the single-cell proteome appears to be complete. The spatial organization of cells in tissues can be studied by emerging technologies, including multiplexed imaging and spatial transcriptomics, which can now be combined with ultra-sensitive proteomics. Combined with high-content imaging, artificial intelligence and single-cell laser microdissection, MS-based proteomics provides an unbiased molecular readout close to the functional level. Potential applications range from basic biological questions to precision medicine.
10.1016/j.molcel.2022.05.022
A knowledge graph to interpret clinical proteomics data.
Nature biotechnology
Implementing precision medicine hinges on the integration of omics data, such as proteomics, into the clinical decision-making process, but the quantity and diversity of biomedical data, and the spread of clinically relevant knowledge across multiple biomedical databases and publications, pose a challenge to data integration. Here we present the Clinical Knowledge Graph (CKG), an open-source platform currently comprising close to 20 million nodes and 220 million relationships that represent relevant experimental data, public databases and literature. The graph structure provides a flexible data model that is easily extendable to new nodes and relationships as new databases become available. The CKG incorporates statistical and machine learning algorithms that accelerate the analysis and interpretation of typical proteomics workflows. Using a set of proof-of-concept biomarker studies, we show how the CKG might augment and enrich proteomics data and help inform clinical decision-making.
10.1038/s41587-021-01145-6
Quantitative Proteomics in Translational Absorption, Distribution, Metabolism, and Excretion and Precision Medicine.
Pharmacological reviews
A reliable translation of in vitro and preclinical data on drug absorption, distribution, metabolism, and excretion (ADME) to humans is important for safe and effective drug development. Precision medicine that is expected to provide the right clinical dose for the right patient at the right time requires a comprehensive understanding of population factors affecting drug disposition and response. Characterization of drug-metabolizing enzymes and transporters for the protein abundance and their interindividual as well as differential tissue and cross-species variabilities is important for translational ADME and precision medicine. This review first provides a brief overview of quantitative proteomics principles including liquid chromatography-tandem mass spectrometry tools, data acquisition approaches, proteomics sample preparation techniques, and quality controls for ensuring rigor and reproducibility in protein quantification data. Then, potential applications of quantitative proteomics in the translation of in vitro and preclinical data as well as prediction of interindividual variability are discussed in detail with tabulated examples. The applications of quantitative proteomics data in physiologically based pharmacokinetic modeling for ADME prediction are discussed with representative case examples. Finally, various considerations for reliable quantitative proteomics analysis for translational ADME and precision medicine and the future directions are discussed. SIGNIFICANCE STATEMENT: Quantitative proteomics analysis of drug-metabolizing enzymes and transporters in humans and preclinical species provides key physiological information that assists in the translation of in vitro and preclinical data to humans. This review provides the principles and applications of quantitative proteomics in characterizing in vitro, ex vivo, and preclinical models for translational research and interindividual variability prediction. Integration of these data into physiologically based pharmacokinetic modeling is proving to be critical for safe, effective, timely, and cost-effective drug development.
10.1124/pharmrev.121.000449
Deep Learning in Proteomics.
Proteomics
Proteomics, the study of all the proteins in biological systems, is becoming a data-rich science. Protein sequences and structures are comprehensively catalogued in online databases. With recent advancements in tandem mass spectrometry (MS) technology, protein expression and post-translational modifications (PTMs) can be studied in a variety of biological systems at the global scale. Sophisticated computational algorithms are needed to translate the vast amount of data into novel biological insights. Deep learning automatically extracts data representations at high levels of abstraction from data, and it thrives in data-rich scientific research domains. Here, a comprehensive overview of deep learning applications in proteomics, including retention time prediction, MS/MS spectrum prediction, de novo peptide sequencing, PTM prediction, major histocompatibility complex-peptide binding prediction, and protein structure prediction, is provided. Limitations and the future directions of deep learning in proteomics are also discussed. This review will provide readers an overview of deep learning and how it can be used to analyze proteomics data.
10.1002/pmic.201900335
An image-based multi-label human protein subcellular localization predictor (iLocator) reveals protein mislocalizations in cancer tissues.
Xu Ying-Ying,Yang Fan,Zhang Yang,Shen Hong-Bin
Bioinformatics (Oxford, England)
MOTIVATION:Human cells are organized into compartments of different biochemical cellular processes. Having proteins appear at the right time to the correct locations in the cellular compartments is required to conduct their functions in normal cells, whereas mislocalization of proteins can result in pathological diseases, including cancer. RESULTS:To reveal the cancer-related protein mislocalizations, we developed an image-based multi-label subcellular location predictor, iLocator, which covers seven cellular localizations. The iLocator incorporates both global and local image descriptors and generates predictions by using an ensemble multi-label classifier. The algorithm has the ability to treat both single- and multiple-location proteins. We first trained and tested iLocator on 3240 normal human tissue images that have known subcellular location information from the human protein atlas. The iLocator was then used to generate protein localization predictions for 3696 protein images from seven cancer tissues that have no location annotations in the human protein atlas. By comparing the output data from normal and cancer tissues, we detected eight potential cancer biomarker proteins that have significant localization differences with P-value < 0.01. AVAILABILITY:http://www.csbio.sjtu.edu.cn/bioinf/iLocator/
10.1093/bioinformatics/btt320
New Opportunities and Challenges of Smart Polymers in Post-Translational Modification Proteomics.
Qing Guangyan,Lu Qi,Xiong Yuting,Zhang Lei,Wang Hongxi,Li Xiuling,Liang Xinmiao,Sun Taolei
Advanced materials (Deerfield Beach, Fla.)
Protein post-translational modifications (PTMs), which denote covalent additions of various functional groups (e.g., phosphate, glycan, methyl, or ubiquitin) to proteins, significantly increase protein complexity and diversity. PTMs play crucial roles in the regulation of protein functions and numerous cellular processes. However, in a living organism, native PTM proteins are typically present at substoichiometric levels, considerably impeding mass-spectrometry-based analyses and identification. Over the past decade, the demand for in-depth PTM proteomics studies has spawned a variety of selective affinity materials capable of capturing trace amounts of PTM peptides from highly complex biosamples. However, novel design ideas or strategies are urgently required for fulfilling the increasingly complex and accurate requirements of PTM proteomics analysis, which can hardly be met by using conventional enrichment materials. Considering two typical types of protein PTMs, phosphorylation and glycosylation, an overview of polymeric enrichment materials is provided here, with an emphasis on the superiority of smart-polymer-based materials that can function in intelligent modes. Moreover, some smart separation materials are introduced to demonstrate the enticing prospects and the challenges of smart polymers applied in PTM proteomics.
10.1002/adma.201604670
Microfluidic-Mass Spectrometry Interfaces for Translational Proteomics.
Pedde R Daniel,Li Huiyan,Borchers Christoph H,Akbari Mohsen
Trends in biotechnology
Interfacing mass spectrometry (MS) with microfluidic chips (μchip-MS) holds considerable potential to transform a clinician's toolbox, providing translatable methods for the early detection, diagnosis, monitoring, and treatment of noncommunicable diseases by streamlining and integrating laborious sample preparation workflows on high-throughput, user-friendly platforms. Overcoming the limitations of competitive immunoassays - currently the gold standard in clinical proteomics - μchip-MS can provide unprecedented access to complex proteomic assays having high sensitivity and specificity, but without the labor, costs, and complexities associated with conventional MS sample processing. This review surveys recent μchip-MS systems for clinical applications and examines their emerging role in streamlining the development and translation of MS-based proteomic assays by alleviating many of the challenges that currently inhibit widespread clinical adoption.
10.1016/j.tibtech.2017.06.006
Detecting Cardiovascular Protein-Protein Interactions by Proximity Proteomics.
Circulation research
Rapidly changing and transient protein-protein interactions regulate dynamic cellular processes in the cardiovascular system. Traditional methods, including affinity purification and mass spectrometry, have revealed many macromolecular complexes in cardiomyocytes and the vasculature. Yet these methods often fail to identify in vivo or transient protein-protein interactions. To capture these interactions in living cells and animals with subsequent mass spectrometry identification, enzyme-catalyzed proximity labeling techniques have been developed in the past decade. Although the application of this methodology to cardiovascular research is still in its infancy, the field is developing rapidly, and the promise is substantial. In this review, we outline important concepts and discuss how proximity proteomics has been applied to study physiological and pathophysiological processes relevant to the cardiovascular system.
10.1161/CIRCRESAHA.121.319810
A proteomics sample metadata representation for multiomics integration and big data analysis.
Nature communications
The amount of public proteomics data is rapidly increasing but there is no standardized format to describe the sample metadata and their relationship with the dataset files in a way that fully supports their understanding or reanalysis. Here we propose to develop the transcriptomics data format MAGE-TAB into a standard representation for proteomics sample metadata. We implement MAGE-TAB-Proteomics in a crowdsourcing project to manually curate over 200 public datasets. We also describe tools and libraries to validate and submit sample metadata-related information to the PRIDE repository. We expect that these developments will improve the reproducibility and facilitate the reanalysis and integration of public proteomics datasets.
10.1038/s41467-021-26111-3
Single-Cell Proteomics.
Vistain Luke F,Tay Savaş
Trends in biochemical sciences
The inability to make broad, minimally biased measurements of a cell's proteome stands as a major bottleneck for understanding how gene expression translates into cellular phenotype. Unlike sequencing for nucleic acids, there is no dominant method for making single-cell proteomic measurements. Instead, methods typically focus on either absolute quantification of a small number of proteins or highly multiplexed protein measurements. Advances in microfluidics and output encoding have led to major improvements in both aspects. Here, we review the most recent progress that has enabled hundreds of protein measurements and ultrahigh-sensitivity quantification. We also highlight emerging technologies such as single-cell mass spectrometry that may enable unbiased measurement of cellular proteomes.
10.1016/j.tibs.2021.01.013
High-throughput proteomics and AI for cancer biomarker discovery.
Xiao Qi,Zhang Fangfei,Xu Luang,Yue Liang,Kon Oi Lian,Zhu Yi,Guo Tiannan
Advanced drug delivery reviews
Biomarkers are assayed to assess biological and pathological status. Recent advances in high-throughput proteomic technology provide opportunities for developing next generation biomarkers for clinical practice aided by artificial intelligence (AI) based techniques. We summarize the advances and limitations of cancer biomarkers based on genomic and transcriptomic analysis, as well as classical antibody-based methodologies. Then we review recent progresses in mass spectrometry (MS)-based proteomics in terms of sample preparation, peptide fractionation by liquid chromatography (LC) and mass spectrometric data acquisition. We highlight applications of AI techniques in high-throughput clinical studies as compared with clinical decisions based on singular features. This review sets out our approach for discovering clinical biomarkers in studies using proteomic big data technology conjoined with computational and statistical methods.
10.1016/j.addr.2021.113844
Paving the way to single-molecule protein sequencing.
Restrepo-Pérez Laura,Joo Chirlmin,Dekker Cees
Nature nanotechnology
Proteins are major building blocks of life. The protein content of a cell and an organism provides key information for the understanding of biological processes and disease. Despite the importance of protein analysis, only a handful of techniques are available to determine protein sequences, and these methods face limitations, for example, requiring a sizable amount of sample. Single-molecule techniques would revolutionize proteomics research, providing ultimate sensitivity for the detection of low-abundance proteins and the realization of single-cell proteomics. In recent years, novel single-molecule protein sequencing schemes that use fluorescence, tunnelling currents and nanopores have been proposed. Here, we present a review of these approaches, together with the first experimental efforts towards their realization. We discuss their advantages and drawbacks, and present our perspective on the development of single-molecule protein sequencing techniques.
10.1038/s41565-018-0236-6
Spatial proteomics: a powerful discovery tool for cell biology.
Lundberg Emma,Borner Georg H H
Nature reviews. Molecular cell biology
Protein subcellular localization is tightly controlled and intimately linked to protein function in health and disease. Capturing the spatial proteome - that is, the localizations of proteins and their dynamics at the subcellular level - is therefore essential for a complete understanding of cell biology. Owing to substantial advances in microscopy, mass spectrometry and machine learning applications for data analysis, the field is now mature for proteome-wide investigations of spatial cellular regulation. Studies of the human proteome have begun to reveal a complex architecture, including single-cell variations, dynamic protein translocations, changing interaction networks and proteins localizing to multiple compartments. Furthermore, several studies have successfully harnessed the power of comparative spatial proteomics as a discovery tool to unravel disease mechanisms. We are at the beginning of an era in which spatial proteomics finally integrates with cell biology and medical research, thereby paving the way for unbiased systems-level insights into cellular processes. Here, we discuss current methods for spatial proteomics using imaging or mass spectrometry and specifically highlight global comparative applications. The aim of this Review is to survey the state of the field and also to encourage more cell biologists to apply spatial proteomics approaches.
10.1038/s41580-018-0094-y
A Golden Age for Working with Public Proteomics Data.
Martens Lennart,Vizcaíno Juan Antonio
Trends in biochemical sciences
Data sharing in mass spectrometry (MS)-based proteomics is becoming a common scientific practice, as is now common in the case of other, more mature 'omics' disciplines like genomics and transcriptomics. We want to highlight that this situation, unprecedented in the field, opens a plethora of opportunities for data scientists. First, we explain in some detail some of the work already achieved, such as systematic reanalysis efforts. We also explain existing applications of public proteomics data, such as proteogenomics and the creation of spectral libraries and spectral archives. Finally, we discuss the main existing challenges and mention the first attempts to combine public proteomics data with other types of omics data sets.
10.1016/j.tibs.2017.01.001
Integrating Networks and Proteomics: Moving Forward.
Goh Wilson Wen Bin,Wong Limsoon
Trends in biotechnology
Networks can resolve many analytical problems in proteomics, including incomplete coverage and inconsistency. Despite high expectations, network-related research in proteomics has experienced only modest growth. In practice, most current research examines non-quantitative usages, for example determining physical interactions among proteins or contextualizing a differential protein list, rather than addressing practical quantitative usages, for example predicting missing proteins or making sample-class predictions. Moreover, many applications are irreproducible and are not widely adopted owing to a lack of common standards, particularly evaluation criteria and gold-standard datasets. A concerted drive towards quantitative applications and convergence towards common standards is essential for 'network-based proteomics' to realize its development potential and make meaningful contributions to clinical applications.
10.1016/j.tibtech.2016.05.015
From Molecules to Mechanisms: Functional Proteomics and Its Application to Renal Tubule Physiology.
Rinschen Markus M,Limbutara Kavee,Knepper Mark A,Payne D Michael,Pisitkun Trairak
Physiological reviews
Classical physiological studies using electrophysiological, biophysical, biochemical, and molecular techniques have created a detailed picture of molecular transport, bioenergetics, contractility and movement, and growth, as well as the regulation of these processes by external stimuli in cells and organisms. Newer systems biology approaches are beginning to provide deeper and broader understanding of these complex biological processes and their dynamic responses to a variety of environmental cues. In the past decade, advances in mass spectrometry-based proteomic technologies have provided invaluable tools to further elucidate these complex cellular processes, thereby confirming, complementing, and advancing common views of physiology. As one notable example, the application of proteomics to study the regulation of kidney function has yielded novel insights into the chemical and physical processes that tightly control body fluids, electrolytes, and metabolites to provide optimal microenvironments for various cellular and organ functions. Here, we systematically review, summarize, and discuss the most significant key findings from functional proteomic studies in renal epithelial physiology. We also identify further improvements in technological and bioinformatics methods that will be essential to advance precision medicine in nephrology.
10.1152/physrev.00057.2017