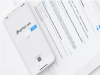
An artificial intelligence-driven agent for real-time head-and-neck IMRT plan generation using conditional generative adversarial network (cGAN).
Li Xinyi,Wang Chunhao,Sheng Yang,Zhang Jiahan,Wang Wentao,Yin Fang-Fang,Wu Qiuwen,Wu Q Jackie,Ge Yaorong
Medical physics
PURPOSE:To develop an artificial intelligence (AI) agent for fully automated rapid head-and-neck intensity-modulated radiation therapy (IMRT) plan generation without time-consuming dose-volume-based inverse planning. METHODS:This AI agent was trained via implementing a conditional generative adversarial network (cGAN) architecture. The generator, PyraNet, is a novel deep learning network that implements 28 classic ResNet blocks in pyramid-like concatenations. The discriminator is a customized four-layer DenseNet. The AI agent first generates multiple customized two-dimensional projections at nine template beam angles from a patient's three-dimensional computed tomography (CT) volume and structures. These projections are then stacked as four-dimensional inputs of PyraNet, from which nine radiation fluence maps of the corresponding template beam angles are generated simultaneously. Finally, the predicted fluence maps are automatically postprocessed by Gaussian deconvolution operations and imported into a commercial treatment planning system (TPS) for plan integrity check and visualization. The AI agent was built and tested upon 231 oropharyngeal IMRT plans from a TPS plan library. 200/16/15 plans were assigned for training/validation/testing, respectively. Only the primary plans in the sequential boost regime were studied. All plans were normalized to 44 Gy prescription (2 Gy/fx). A customized Harr wavelet loss was adopted for fluence map comparison during the training of the PyraNet. For test cases, isodose distributions in AI plans and TPS plans were qualitatively evaluated for overall dose distributions. Key dosimetric metrics were compared by Wilcoxon signed-rank tests with a significance level of 0.05. RESULTS:All 15 AI plans were successfully generated. Isodose gradients outside of PTV in AI plans were comparable to those of the TPS plans. After PTV coverage normalization, D of left parotid (D = 23.1 ± 2.4 Gy; D = 23.1 ± 2.0 Gy), right parotid (D = 23.8 ± 3.0 Gy; D = 23.9 ± 2.3 Gy), and oral cavity (D = 24.7 ± 6.0 Gy; D = 23.9 ± 4.3 Gy) in the AI plans and the TPS plans were comparable without statistical significance. AI plans achieved comparable results for maximum dose at 0.01cc of brainstem (D = 15.0 ± 2.1 Gy; D = 15.5 ± 2.7 Gy) and cord + 5mm (D = 27.5 ± 2.3 Gy; D = 25.8 ± 1.9 Gy) without clinically relevant differences, but body D results (D = 121.1 ± 3.9 Gy; D = 109.0 ± 0.9 Gy) were higher than the TPS plan results. The AI agent needed ~3 s for predicting fluence maps of an IMRT plan. CONCLUSIONS:With rapid and fully automated execution, the developed AI agent can generate complex head-and-neck IMRT plans with acceptable dosimetry quality. This approach holds great potential for clinical applications in preplanning decision-making and real-time planning.
10.1002/mp.14770
Evaluation of auto-segmentation accuracy of cloud-based artificial intelligence and atlas-based models.
Urago Yuka,Okamoto Hiroyuki,Kaneda Tomoya,Murakami Naoya,Kashihara Tairo,Takemori Mihiro,Nakayama Hiroki,Iijima Kotaro,Chiba Takahito,Kuwahara Junichi,Katsuta Shouichi,Nakamura Satoshi,Chang Weishan,Saitoh Hidetoshi,Igaki Hiroshi
Radiation oncology (London, England)
BACKGROUND:Contour delineation, a crucial process in radiation oncology, is time-consuming and inaccurate due to inter-observer variation has been a critical issue in this process. An atlas-based automatic segmentation was developed to improve the delineation efficiency and reduce inter-observer variation. Additionally, automated segmentation using artificial intelligence (AI) has recently become available. In this study, auto-segmentations by atlas- and AI-based models for Organs at Risk (OAR) in patients with prostate and head and neck cancer were performed and delineation accuracies were evaluated. METHODS:Twenty-one patients with prostate cancer and 30 patients with head and neck cancer were evaluated. MIM Maestro was used to apply the atlas-based segmentation. MIM Contour ProtégéAI was used to apply the AI-based segmentation. Three similarity indices, the Dice similarity coefficient (DSC), Hausdorff distance (HD), and mean distance to agreement (MDA), were evaluated and compared with manual delineations. In addition, radiation oncologists visually evaluated the delineation accuracies. RESULTS:Among patients with prostate cancer, the AI-based model demonstrated higher accuracy than the atlas-based on DSC, HD, and MDA for the bladder and rectum. Upon visual evaluation, some errors were observed in the atlas-based delineations when the boundary between the small bowel or the seminal vesicle and the bladder was unclear. For patients with head and neck cancer, no significant differences were observed between the two models for almost all OARs, except small delineations such as the optic chiasm and optic nerve. The DSC tended to be lower when the HD and the MDA were smaller in small volume delineations. CONCLUSIONS:In terms of efficiency, the processing time for head and neck cancers was much shorter than manual delineation. While quantitative evaluation with AI-based segmentation was significantly more accurate than atlas-based for prostate cancer, there was no significant difference for head and neck cancer. According to the results of visual evaluation, less necessity of manual correction in AI-based segmentation indicates that the segmentation efficiency of AI-based model is higher than that of atlas-based model. The effectiveness of the AI-based model can be expected to improve the segmentation efficiency and to significantly shorten the delineation time.
10.1186/s13014-021-01896-1