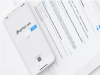
Radiomic analysis of contrast-enhanced CT predicts microvascular invasion and outcome in hepatocellular carcinoma.
Xu Xun,Zhang Hai-Long,Liu Qiu-Ping,Sun Shu-Wen,Zhang Jing,Zhu Fei-Peng,Yang Guang,Yan Xu,Zhang Yu-Dong,Liu Xi-Sheng
Journal of hepatology
BACKGROUND & AIMS:Microvascular invasion (MVI) impairs surgical outcomes in patients with hepatocellular carcinoma (HCC). As there is no single highly reliable factor to preoperatively predict MVI, we developed a computational approach integrating large-scale clinical and imaging modalities, especially radiomic features from contrast-enhanced CT, to predict MVI and clinical outcomes in patients with HCC. METHODS:In total, 495 surgically resected patients were retrospectively included. MVI-related radiomic scores (R-scores) were built from 7,260 radiomic features in 6 target volumes. Six R-scores, 15 clinical factors, and 12 radiographic scores were integrated into a predictive model, the radiographic-radiomic (RR) model, with multivariate logistic regression. RESULTS:Radiomics related to tumor size and intratumoral heterogeneity were the top-ranked MVI predicting features. The related R-scores showed significant differences according to MVI status (p <0.001). Regression analysis identified 8 MVI risk factors, including 5 radiographic features and an R-score. The R-score (odds ratio [OR] 2.34) was less important than tumor capsule (OR 5.12), tumor margin (OR4.20), and peritumoral enhancement (OR 3.03). The RR model using these predictors achieved an area under the curve (AUC) of 0.909 in training/validation and 0.889 in the test set. Progression-free survival (PFS) and overall survival (OS) were significantly different between the RR-predicted MVI-absent and MVI-present groups (median PFS: 49.5 vs. 12.9 months; median OS: 76.3 vs. 47.3 months). RR-computed MVI probability, histologic MVI, tumor size, and Edmondson-Steiner grade were independently associated with disease-specific recurrence and mortality. CONCLUSIONS:The computational approach, integrating large-scale clinico-radiologic and radiomic features, demonstrates good performance for predicting MVI and clinical outcomes. However, radiomics with current CT imaging analysis protocols do not provide statistically significant added value to radiographic scores. LAY SUMMARY:The most effective treatment for hepatocellular carcinoma (HCC) is surgical removal of the tumor but often recurrence occurs, partly due to the presence of microvascular invasion (MVI). Lacking a single highly reliable factor able to preoperatively predict MVI, we developed a computational approach to predict MVI and the long-term clinical outcome of patients with HCC. In particular, the added value of radiomics, a newly emerging form of radiography, was comprehensively investigated. This computational method can enhance the communication with the patient about the likely success of the treatment and guide clinical management, with the aim of finding drugs that reduce the risk of recurrence.
10.1016/j.jhep.2019.02.023
Predicting the risk of locoregional recurrence after early breast cancer: an external validation of the Dutch INFLUENCE-nomogram with clinical cancer registry data from Germany.
Voelkel Vinzenz,Draeger Teresa,Groothuis-Oudshoorn Catharina G M,de Munck Linda,Hueting Tom,Gerken Michael,Klinkhammer-Schalke Monika,Lavric Miha,Siesling Sabine
Journal of cancer research and clinical oncology
PURPOSE:Follow-up after breast cancer treatment aims for an early detection of locoregional breast cancer recurrences (LRR) to improve the patients' outcome. By estimating individual's 5-year recurrence-risks, the Dutch INFLUENCE-nomogram can assist health professionals and patients in developing personalized risk-based follow-up pathways. The objective of this study is to validate the prediction tool on non-Dutch patients. MATERIAL AND METHODS:Data for this external validation derive from a large clinical cancer registry in southern Germany, covering a population of 1.1 million. Patients with curative resection of early-stage breast cancer, diagnosed between 2000 and 2012, were included in the analysis (n = 6520). For each of them, an individual LRR-risk was estimated by the INFLUENCE-nomogram. Its predictive ability was tested by comparing estimated and observed LRR-probabilities using the Hosmer-Lemeshow goodness-of-fit test and C-statistics. RESULTS:In the German validation-cohort, 2.8% of the patients developed an LRR within 5 years after primary surgery (n = 184). While the INFLUENCE-nomogram generally underestimates the actual LRR-risk of the German patients (p < 0.001), its discriminative ability is comparable to the one observed in the original Dutch modeling-cohort (C-statistic German validation-cohort: 0.73, CI 0.69-0.77 vs. C-statistic Dutch modeling-cohort: 0.71, CI 0.69-0.73). Similar results were obtained in most of the subgroup analyses stratified by age, type of surgery and intrinsic biological subtypes. CONCLUSION:The outcomes of this external validation underline the generalizability of the INFLUENCE-nomogram beyond the Dutch population. The model performance could be enhanced in future by incorporating additional risk factors for LRR.
10.1007/s00432-019-02904-4
Prognostic models for breast cancer: a systematic review.
Phung Minh Tung,Tin Tin Sandar,Elwood J Mark
BMC cancer
BACKGROUND:Breast cancer is the most common cancer in women worldwide, with a great diversity in outcomes among individual patients. The ability to accurately predict a breast cancer outcome is important to patients, physicians, researchers, and policy makers. Many models have been developed and tested in different settings. We systematically reviewed the prognostic models developed and/or validated for patients with breast cancer. METHODS:We conducted a systematic search in four electronic databases and some oncology websites, and a manual search in the bibliographies of the included studies. We identified original studies that were published prior to 1st January 2017, and presented the development and/or validation of models based mainly on clinico-pathological factors to predict mortality and/or recurrence in female breast cancer patients. RESULTS:From the 96 articles selected from 4095 citations found, we identified 58 models, which predicted mortality (n = 28), recurrence (n = 23), or both (n = 7). The most frequently used predictors were nodal status (n = 49), tumour size (n = 42), tumour grade (n = 29), age at diagnosis (n = 24), and oestrogen receptor status (n = 21). Models were developed in Europe (n = 25), Asia (n = 13), North America (n = 12), and Australia (n = 1) between 1982 and 2016. Models were validated in the development cohorts (n = 43) and/or independent populations (n = 17), by comparing the predicted outcomes with the observed outcomes (n = 55) and/or with the outcomes estimated by other models (n = 32), or the outcomes estimated by individual prognostic factors (n = 8). The most commonly used methods were: Cox proportional hazards regression for model development (n = 32); the absolute differences between the predicted and observed outcomes (n = 30) for calibration; and C-index/AUC (n = 44) for discrimination. Overall, the models performed well in the development cohorts but less accurately in some independent populations, particularly in patients with high risk and young and elderly patients. An exception is the Nottingham Prognostic Index, which retains its predicting ability in most independent populations. CONCLUSIONS:Many prognostic models have been developed for breast cancer, but only a few have been validated widely in different settings. Importantly, their performance was suboptimal in independent populations, particularly in patients with high risk and in young and elderly patients.
10.1186/s12885-019-5442-6
Triple-negative breast cancer: Pretreatment magnetic resonance imaging features and clinicopathological factors associated with recurrence.
Lee Youn Joo,Youn In Kyung,Kim Sung Hun,Kang Bong Joo,Park Woo-Chan,Lee Ahwon
Magnetic resonance imaging
PURPOSE:We aimed to investigate the magnetic resonance imaging (MRI) features and clinicopathologic factors with recurrence of triple-negative breast cancer (TNBC). PATIENTS AND METHODS:We identified 281 patients with 288 surgically confirmed TNBC lesions who underwent pretreatment MRI between 2009 and 2015. The presence of intratumoral high signal on T2-weighted images, high-signal rim on diffusion-weighted images (DWI), and rim enhancement on the dynamic contrast-enhanced MRI and clinicopathological data were collected. Cox proportional analysis was performed. RESULTS:Of the 288 lesions, 36 (12.5%) recurred after a median follow-up of 18 months (range, 3.6-68.3 months). Rim enhancement (hazard ratio [HR] = 3.15; 95% confidence interval [CI] = 1.01, 9.88; p = .048), and lymphovascular invasion (HR = 2.73, 95% CI = 1.20, 6.23; p = .016) were independently associated with disease recurrence. While fibroglandular volume, background parenchymal enhancement, intratumoral T2 high signal, and high-signal rim on DWI, were not found to be risk factors for recurrence. CONCLUSION:Pretreatment MRI features may help predict a high risk of recurrence in patients with TNBC.
10.1016/j.mri.2019.10.001
Prediction of Breast Cancer Recurrence Using a Deep Convolutional Neural Network Without Region-of-Interest Labeling.
Frontiers in oncology
PURPOSE:The present study aimed to assign a risk score for breast cancer recurrence based on pathological whole slide images (WSIs) using a deep learning model. METHODS:A total of 233 WSIs from 138 breast cancer patients were assigned either a low-risk or a high-risk score based on a 70-gene signature. These images were processed into patches of 512x512 pixels by the PyHIST tool and underwent color normalization using the Macenko method. Afterward, out of focus and pixelated patches were removed using the Laplacian algorithm. Finally, the remaining patches (n=294,562) were split into 3 parts for model training (50%), validation (7%) and testing (43%). We used 6 pretrained models for transfer learning and evaluated their performance using accuracy, precision, recall, F1 score, confusion matrix, and AUC. Additionally, to demonstrate the robustness of the final model and its generalization capacity, the testing set was used for model evaluation. Finally, the GRAD-CAM algorithm was used for model visualization. RESULTS:Six models, namely VGG16, ResNet50, ResNet101, Inception_ResNet, EfficientB5, and Xception, achieved high performance in the validation set with an overall accuracy of 0.84, 0.85, 0.83, 0.84, 0.87, and 0.91, respectively. We selected Xception for assessment of the testing set, and this model achieved an overall accuracy of 0.87 with a patch-wise approach and 0.90 and 1.00 with a patient-wise approach for high-risk and low-risk groups, respectively. CONCLUSIONS:Our study demonstrated the feasibility and high performance of artificial intelligence models trained without region-of-interest labeling for predicting cancer recurrence based on a 70-gene signature risk score.
10.3389/fonc.2021.734015
A multi-institutional prediction model to estimate the risk of recurrence and mortality after mastectomy for T1-2N1 breast cancer.
Cancer
BACKGROUND:Post-mastectomy radiation therapy (PMRT) in women with pathologic stage T1-2N1M0 breast cancer is controversial. METHODS:Data from five North American institutions including women undergoing mastectomy without neoadjuvant therapy with pT1-2N1M0 breast cancer treated from 2006 to 2015 were pooled for analysis. Competing-risks regression was performed to identify factors associated with locoregional recurrence (LRR), distant metastasis (DM), overall recurrence (OR), and breast cancer mortality (BCM). RESULTS:A total of 3532 patients were included for analysis with a median follow-up time among survivors of 6.8 years (interquartile range [IQR], 4.5-9.5 years). The 2154 (61%) patients who received PMRT had significantly more adverse risk factors than those patients not receiving PMRT: younger age, larger tumors, more positive lymph nodes, lymphovascular invasion, extracapsular extension, and positive margins (p < .05 for all). On competing risk regression analysis, receipt of PMRT was significantly associated with a decreased risk of LRR (hazard ratio [HR], 0.21; 95% confidence interval [CI], 0.14-0.31; p < .001) and OR (HR, 0.76; 95% CI, 0.62-0.94; p = .011). Model performance metrics for each end point showed good discrimination and calibration. An online prediction model to estimate predicted risks for each outcome based on individual patient and tumor characteristics was created from the model. CONCLUSIONS:In a large multi-institutional cohort of patients, PMRT for T1-2N1 breast cancer was associated with a significant reduction in locoregional and overall recurrence after accounting for known prognostic factors. An online calculator was developed to aid in personalized decision-making regarding PMRT in this population.
10.1002/cncr.34352
Prediction of HER2-positive breast cancer recurrence and metastasis risk from histopathological images and clinical information via multimodal deep learning.
Yang Jialiang,Ju Jie,Guo Lei,Ji Binbin,Shi Shufang,Yang Zixuan,Gao Songlin,Yuan Xu,Tian Geng,Liang Yuebin,Yuan Peng
Computational and structural biotechnology journal
HER2-positive breast cancer is a highly heterogeneous tumor, and about 30% of patients still suffer from recurrence and metastasis after trastuzumab targeted therapy. Predicting individual prognosis is of great significance for the further development of precise therapy. With the continuous development of computer technology, more and more attention has been paid to computer-aided diagnosis and prognosis prediction based on Hematoxylin and Eosin (H&E) pathological images, which are available for all breast cancer patients undergone surgical treatment. In this study, we first enrolled 127 HER2-positive breast cancer patients with known recurrence and metastasis status from Cancer Hospital of the Chinese Academy of Medical Sciences. We then proposed a novel multimodal deep learning method integrating whole slide H&E images (WSIs) and clinical information to accurately assess the risk of relapse and metastasis in patients with HER2-positive breast cancer. Specifically, we obtained the whole H&E staining images from the surgical specimens of breast cancer patients, and these images were adjusted to size 512 × 512 pixels. The deep convolutional neural network (CNN) was applied to these images to retrieve image features, which were combined with the clinical data. Based on the combined features. After that, a novel multimodal model was constructed for predicting the prognosis of each patient. The model achieved an area under curve (AUC) of 0.76 in the two-fold cross-validation (CV). To further evaluate the performance of our model, we downloaded the data of all 123 HER2-positive breast cancer patients with available H&E image and known recurrence and metastasis status in The Cancer Genome Atlas (TCGA), which was severed as an independent testing data. Despite the huge differences in race and experimental strategies, our model achieved an AUC of 0.72 in the TCGA samples. As a conclusion, H&E images, in conjunction with clinical information and advanced deep learning models, could be used to evaluate the risk of relapse and metastasis in patients with HER2-positive breast cancer.
10.1016/j.csbj.2021.12.028