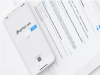
A continual prediction model for inpatient acute kidney injury.
Kate Rohit J,Pearce Noah,Mazumdar Debesh,Nilakantan Vani
Computers in biology and medicine
Acute kidney injury (AKI) commonly occurs in hospitalized patients and can lead to serious medical complications. But it is preventable and potentially reversible with early diagnosis and management. Therefore, several machine learning based predictive models have been built to predict AKI in advance from electronic health records (EHR) data. These models to predict inpatient AKI were always built to make predictions at a particular time, for example, 24 or 48 h from admission. However, hospital stays can be several days long and AKI can develop any time within a few hours. To optimally predict AKI before it develops at any time during a hospital stay, we present a novel framework in which AKI is continually predicted automatically from EHR data over the entire hospital stay. The continual model predicts AKI every time a patient's AKI-relevant variable changes in the EHR. Thus, the model not only is independent of a particular time for making predictions, it can also leverage the latest values of all the AKI-relevant patient variables for making predictions. A method to comprehensively evaluate the overall performance of a continual prediction model is also introduced, and we experimentally show using a large dataset of hospital stays that the continual prediction model out-performs all one-time prediction models in predicting AKI.
10.1016/j.compbiomed.2019.103580
Pathologic Exploration of the Axillary Soft Tissue Microenvironment and Its Impact on Axillary Management and Breast Cancer Outcomes.
Journal of clinical oncology : official journal of the American Society of Clinical Oncology
PURPOSE:Axillary soft tissue (AXT) involvement with tumor cells extending beyond the positive lymph node (LN+) and extracapsular extension (ECE) has been overlooked in breast pathology specimen analysis. MATERIALS AND METHODS:We analyzed 2,162 LN+ patients, dividing them into four groups on the basis of axillary pathology: (1) LN+ only, (2) LN+ and ECE only, (3) LN+ and AXT without ECE, and (4) LN+ with both AXT and ECE. The primary end points were 10-year locoregional failure (LRF), the 10-year axillary failure, and 10-year distant metastasis rates. Multivariable Cox models, accounting for clinical factors, were fitted using the entire cohort, and subgroups analyses were conducted. RESULTS:The median follow-up was 9.4 years. The 10-year distant metastasis incidence was 42% for LN + AXT + ECE, 23% for both LN + AXT and LN + ECE only, and 13% for LN+ only. The 10-year axillary failure rates were 4.5% for LN + AXT + ECE, 4.6% for LN + AXT, 0.8% for LN + ECE only, and 1.6% for LN+ only. The 10-year LRF rates were 14% for LN + AXT + ECE, 10% for LN + AXT, 5.7% for LN + ECE only, and 6.2% for LN+ only. Multivariable analysis revealed that AXT was significantly associated with distant metastasis (hazard ratio [HR], 1.6; < .001), locoregional failure (HR, 2.3; < .001), and axillary failure (HR, 3.3; = .003). Subgroup analyses showed that regional LN radiation (RLNR) improved locoregional tumor outcomes with AXT, ECE, or both (HR, 0.5; = .03). Delivering ≤50 Gy to the axilla in the presence of AXT/ECE increased axillary failure (HR, 3.0; = .04). Moreover, when delivering RLNR, axillary LN dissection could be de-escalated to sentinel node biopsy even in the presence of features such as AXT or ECE without significantly increasing any failure outcome: (HR, 1.0; = .92) for LRF, (HR, 1.1; = .94) axillary failure, and (HR, 0.4; = .01) distant metastasis. CONCLUSION:Routine reporting of axillary tissue involvement, beyond LNs and ECE, is crucial in predicting breast cancer outcomes. Ruling out the presence of AXT is imperative before any form of axillary de-escalation, especially RLNR omission.
10.1200/JCO.23.01009
Development and validation of a risk prediction model of preterm birth for women with preterm labour symptoms (the QUIDS study): A prospective cohort study and individual participant data meta-analysis.
Stock Sarah J,Horne Margaret,Bruijn Merel,White Helen,Boyd Kathleen A,Heggie Robert,Wotherspoon Lisa,Aucott Lorna,Morris Rachel K,Dorling Jon,Jackson Lesley,Chandiramani Manju,David Anna L,Khalil Asma,Shennan Andrew,van Baaren Gert-Jan,Hodgetts-Morton Victoria,Lavender Tina,Schuit Ewoud,Harper-Clarke Susan,Mol Ben W,Riley Richard D,Norman Jane E,Norrie John
PLoS medicine
BACKGROUND:Timely interventions in women presenting with preterm labour can substantially improve health outcomes for preterm babies. However, establishing such a diagnosis is very challenging, as signs and symptoms of preterm labour are common and can be nonspecific. We aimed to develop and externally validate a risk prediction model using concentration of vaginal fluid fetal fibronectin (quantitative fFN), in combination with clinical risk factors, for the prediction of spontaneous preterm birth and assessed its cost-effectiveness. METHODS AND FINDINGS:Pregnant women included in the analyses were 22+0 to 34+6 weeks gestation with signs and symptoms of preterm labour. The primary outcome was spontaneous preterm birth within 7 days of quantitative fFN test. The risk prediction model was developed and internally validated in an individual participant data (IPD) meta-analysis of 5 European prospective cohort studies (2009 to 2016; 1,783 women; mean age 29.7 years; median BMI 24.8 kg/m2; 67.6% White; 11.7% smokers; 51.8% nulliparous; 10.4% with multiple pregnancy; 139 [7.8%] with spontaneous preterm birth within 7 days). The model was then externally validated in a prospective cohort study in 26 United Kingdom centres (2016 to 2018; 2,924 women; mean age 28.2 years; median BMI 25.4 kg/m2; 88.2% White; 21% smokers; 35.2% nulliparous; 3.5% with multiple pregnancy; 85 [2.9%] with spontaneous preterm birth within 7 days). The developed risk prediction model for spontaneous preterm birth within 7 days included quantitative fFN, current smoking, not White ethnicity, nulliparity, and multiple pregnancy. After internal validation, the optimism adjusted area under the curve was 0.89 (95% CI 0.86 to 0.92), and the optimism adjusted Nagelkerke R2 was 35% (95% CI 33% to 37%). On external validation in the prospective UK cohort population, the area under the curve was 0.89 (95% CI 0.84 to 0.94), and Nagelkerke R2 of 36% (95% CI: 34% to 38%). Recalibration of the model's intercept was required to ensure overall calibration-in-the-large. A calibration curve suggested close agreement between predicted and observed risks in the range of predictions 0% to 10%, but some miscalibration (underprediction) at higher risks (slope 1.24 (95% CI 1.23 to 1.26)). Despite any miscalibration, the net benefit of the model was higher than "treat all" or "treat none" strategies for thresholds up to about 15% risk. The economic analysis found the prognostic model was cost effective, compared to using qualitative fFN, at a threshold for hospital admission and treatment of ≥2% risk of preterm birth within 7 days. Study limitations include the limited number of participants who are not White and levels of missing data for certain variables in the development dataset. CONCLUSIONS:In this study, we found that a risk prediction model including vaginal fFN concentration and clinical risk factors showed promising performance in the prediction of spontaneous preterm birth within 7 days of test and has potential to inform management decisions for women with threatened preterm labour. Further evaluation of the risk prediction model in clinical practice is required to determine whether the risk prediction model improves clinical outcomes if used in practice. TRIAL REGISTRATION:The study was approved by the West of Scotland Research Ethics Committee (16/WS/0068). The study was registered with ISRCTN Registry (ISRCTN 41598423) and NIHR Portfolio (CPMS: 31277).
10.1371/journal.pmed.1003686
Prediction Model for Screening Patients at Risk of Malnutrition After Gastric Cancer Surgery.
Park Ji-Hyeon,Kim Eunjung,Seol Eun-Mi,Kong Seong-Ho,Park Do Joong,Yang Han-Kwang,Choi Jong-Ho,Park Shin-Hoo,Choe Hwi-Nyeong,Kweon Meera,Park Jiwon,Choi Yunhee,Lee Hyuk-Joon
Annals of surgical oncology
BACKGROUND:Malnutrition after gastrectomy is associated with a poor prognosis; however, no accurate model for predicting post-gastrectomy malnutrition exists. Hence, we conducted a retrospective study to develop a prediction model identifying gastric cancer patients at high risk of malnutrition after gastrectomy. METHOD:Gastric cancer patients who underwent curative gastrectomy with more than one weight measurement during a 3-year follow-up period were included. Malnutrition was defined as body mass index (BMI) < 18.5 kg/m according to the European Society of Clinical Nutrition and Metabolism diagnostic criteria. BMI-loss pattern was analyzed using a group-based trajectory model. A prediction model for malnutrition 6 months after gastrectomy was developed based on significant risk factors, and then validated. RESULTS:Overall, 1421 patients were examined. The BMI-loss trajectory model showed significant BMI loss at 6 months after gastrectomy. Severe BMI loss (mean 21.5%; n = 109) was significantly associated with the elderly, female sex, higher preoperative BMI, advanced cancer stage, open surgery, total gastrectomy, Roux-en-Y reconstruction, chemotherapy, and postoperative complications (all p < 0.05). Malnutrition 6 months after gastrectomy was observed in 152 (11.9%) of 1281 patients. Preoperative BMI, sex, and type of operation were included in the final prediction model as predictive factors (p < 0.05). The C-index of the developmental set and bootstrap validation of the prediction model was 0.91 (95% confidence interval 0.89-0.94) and 0.91, respectively. CONCLUSION:The prediction model for the risk of malnutrition 6 months after gastrectomy was accurately developed, with three independent risk factors: low preoperative BMI, female sex, and total or proximal gastrectomy.
10.1245/s10434-020-09559-3
Prediction of recurrent venous thrombosis in all patients with a first venous thrombotic event: The Leiden Thrombosis Recurrence Risk Prediction model (L-TRRiP).
Timp Jasmijn F,Braekkan Sigrid K,Lijfering Willem M,van Hylckama Vlieg Astrid,Hansen John-Bjarne,Rosendaal Frits R,le Cessie Saskia,Cannegieter Suzanne C
PLoS medicine
BACKGROUND:Recurrent venous thromboembolism (VTE) is common. Current guidelines suggest that patients with unprovoked VTE should continue anticoagulants unless they have a high bleeding risk, whereas all others can stop. Prediction models may refine this dichotomous distinction, but existing models apply only to patients with unprovoked first thrombosis. We aimed to develop a prediction model for all patients with first VTE, either provoked or unprovoked. METHODS AND FINDINGS:Data were used from two population-based cohorts of patients with first VTE from the Netherlands (Multiple Environment and Genetic Assessment of Risk Factors for Venous Thrombosis [MEGA] follow-up study, performed from 1994 to 2009; model derivation; n = 3,750) and from Norway (Tromsø study, performed from 1999 to 2016; model validation; n = 663). Four versions of a VTE prediction model were developed: model A (clinical, laboratory, and genetic variables), model B (clinical variables and fewer laboratory markers), model C (clinical and genetic factors), and model D (clinical variables only). The outcome measure was recurrent VTE. To determine the discriminatory power, Harrell's C-statistic was calculated. A prognostic score was assessed for each patient. Kaplan-Meier plots for the observed recurrence risks were created in quintiles of the prognostic scores. For each patient, the 2-year predicted recurrence risk was calculated. Models C and D were validated in the Tromsø study. During 19,201 person-years of follow-up (median duration 5.7 years) in the MEGA study, 507 recurrences occurred. Model A had the highest predictive capability, with a C-statistic of 0.73 (95% CI 0.71-0.76). The discriminative performance was somewhat lower in the other models, with C-statistics of 0.72 for model B, 0.70 for model C, and 0.69 for model D. Internal validation showed a minimal degree of optimism bias. Models C and D were externally validated, with C-statistics of 0.64 (95% CI 0.62-0.66) and 0.65 (95% CI 0.63-0.66), respectively. According to model C, in 2,592 patients with provoked first events, 367 (15%) patients had a predicted 2-year risk of >10%, whereas in 1,082 patients whose first event was unprovoked, 484 (45%) had a predicted 2-year risk of <10%. A limitation of both cohorts is that laboratory measurements were missing in a substantial proportion of patients, which therefore were imputed. CONCLUSIONS:The prediction model we propose applies to patients with provoked or unprovoked first VTE-except for patients with (a history of) cancer-allows refined risk stratification, and is easily usable. For optimal individualized treatment, a management study in which bleeding risks are also taken into account is necessary.
10.1371/journal.pmed.1002883
Normal tissue adjacent to tumor expression profile analysis developed and validated a prognostic model based on Hippo-related genes in hepatocellular carcinoma.
Pan Qingbo,Qin Fanbo,Yuan Hanyu,He Baoning,Yang Ni,Zhang Yitong,Ren Hong,Zeng Yi
Cancer medicine
BACKGROUND:Hepatocellular carcinoma (HCC) is the most common malignant disease worldwide. Although the diagnosis and treatment of HCC have greatly improved in the recent years, there is still a lack of accurate methods to predict the prognosis of patients. Evidence has shown that Hippo signaling in tissues adjacent to HCC plays a significant role in HCC development. In the present study, we aimed to construct a model based on the expression of Hippo-related genes (HRGs) in tissues adjacent to HCC to predict the prognosis of HCC patients. METHODS:Gene expression data of paired normal tissues adjacent to HCC (PNTAH) and clinical information were obtained from Gene Expression Omnibus (GEO) and The Cancer Genome Atlas (TCGA) databases. The HRG signature was constructed using four canonical Hippo-related pathways. Univariate Cox regression analysis was used to screen survival-related HRGs. LASSO and multivariate Cox regression analyses were used to construct the prognostic model. The true and false positive rates of the model were confirmed using receiver operating characteristic (ROC) analysis. RESULTS:The prognostic model was constructed based on the expression levels of five HRGs (NF2, MYC, BIRC3, CSNK1E, and MINK1) in PNTAH. The mortality rate of HCC patients increased as the risk score determined by the model increased. Furthermore, the risk score was found to be an independent risk factor for the survival of patients. ROC analysis showed that the prognostic model had a better predictive value than the other conventional clinical parameters. Moreover, the reliability of the prognostic model was confirmed in TCGA-LIHC cohort. A nomogram was generated to predict patient survival. An exploration of the predictive value of the model in HCC tissues indicated that the model is PNTAH-specific. CONCLUSIONS:We developed and validated a prognostic model based on the expression levels of five HRGs in PNTAH, and this model should be helpful in predicting the prognosis of patients with HCC.
10.1002/cam4.3890
Risk Factors of Subsequent Primary Melanomas in Austria.
JAMA dermatology
Importance:Information on risk factors of subsequent melanomas would be helpful to identify patients at risk after the diagnosis of their first melanomas. Objective:To determine risk factors of subsequent melanomas. Design, Setting, and Participants:In this retrospective case-control study, 1648 participants with histologically verified cutaneous melanoma diagnosed from January 1, 1968, though March 16, 2015, were recruited from a tertiary referral center as part of the Molecular Markers of Melanoma study. CDKN2A was sequenced in 514 and MC1R in 953 participants. Data were analyzed from March 7, 2008, through March 25, 2015. Main Outcomes and Measures:Phenotypic traits and internal and external risk factors for the development of a second, third, or fourth melanoma. Results:In total, 1648 patients (53.6% men; mean [SD] age, 54 [15] years) were enrolled, including 1349 with single and 299 with multiple primary melanoma. Mean (SD) age at recruitment was 57 (15) years for the single-melanoma and 62 (14) years for the multiple-melanoma groups. From the internal risk factors, family history (odds ratio [OR], 1.76; 95% CI, 1.22-2.55; P = .006), CDKN2A high-risk mutations (OR, 4.03; 95% CI, 1.28-12.70; P = .02), and high numbers of nevi as a phenotypic risk factor (ORs, 2.23 [95% CI, 1.56-3.28, P < .001] for 20-30 smaller nevi and 2.56 [95% CI, 1.50-4.36; P = .003] for 20-30 larger nevi) were significantly associated with the risk of developing a subsequent primary melanoma using multivariate logistic regression analysis. Nonmelanoma skin cancer (OR, 2.57; 95% CI, 1.84-3.58; P < .001) and signs of actinic skin damage, particularly on the back (ORs, 1.91 [95% CI, 1.12-3.25; P = .04] for freckling and 1.92 [95% CI, 1.29-3.08; P = .007] for solar lentigines), additionally increased risk of a subsequent melanoma. All those factors were also associated with an earlier development of the second melanoma. Patients with 3 melanomas developed their second melanoma earlier than patients with only 2 melanomas (mean [SD] age, 55 [15] years for those with 2 primary melanomas; 52 [15] years for those with 3 primary melanomas). Time spent outdoors, solarium use, outdoor occupation, and hair color had no significant associations in these models. Conclusions and Relevance:According to the results of this study, internal factors (family history and genetic variants), number of nevi, and actinic damage on the back are more relevant for the development of subsequent melanomas than skin phototype or hair color. Patients with many nevi were younger at the time of the diagnosis of their first melanoma. This finding could help to identify persons at increased risk of developing multiple primary melanomas.
10.1001/jamadermatol.2018.4645
Survival prediction model of children with diffuse intrinsic pontine glioma based on clinical and radiological criteria.
Jansen Marc H,Veldhuijzen van Zanten Sophie E,Sanchez Aliaga Esther,Heymans Martijn W,Warmuth-Metz Monika,Hargrave Darren,van der Hoeven Erica J,Gidding Corrie E,de Bont Eveline S,Eshghi Omid S,Reddingius Roel,Peeters Cacha M,Schouten-van Meeteren Antoinette Y N,Gooskens Rob H J,Granzen Bernd,Paardekooper Gabriel M,Janssens Geert O,Noske David P,Barkhof Frederik,Kramm Christof M,Vandertop W Peter,Kaspers Gertjan J,van Vuurden Dannis G
Neuro-oncology
BACKGROUND:Although diffuse intrinsic pontine glioma (DIPG) carries the worst prognosis of all pediatric brain tumors, studies on prognostic factors in DIPG are sparse. To control for confounding variables in DIPG studies, which generally include relatively small patient numbers, a survival prediction tool is needed. METHODS:A multicenter retrospective cohort study was performed in the Netherlands, the UK, and Germany with central review of clinical data and MRI scans of children with DIPG. Cox proportional hazards with backward regression was used to select prognostic variables (P < .05) to predict the accumulated 12-month risk of death. These predictors were transformed into a practical risk score. The model's performance was validated by bootstrapping techniques. RESULTS:A total of 316 patients were included. The median overall survival was 10 months. Multivariate Cox analysis yielded 5 prognostic variables of which the coefficients were included in the risk score. Age ≤3 years, longer symptom duration at diagnosis, and use of oral and intravenous chemotherapy were favorable predictors, while ring enhancement on MRI at diagnosis was an unfavorable predictor. With increasing risk score categories, overall survival decreased significantly. The model can distinguish between patients with very short, average, and increased overall survival (medians of 7.0, 9.7, and 13.7 mo, respectively). The area under the receiver operating characteristic curve was 0.68. CONCLUSIONS:We developed a DIPG survival prediction tool that can be used to predict the outcome of patients and for stratification in trials. Validation of the model is needed in a prospective cohort.
10.1093/neuonc/nou104
Deficiencies of cardiovascular risk prediction models for type 1 diabetes.
Zgibor Janice C,Piatt Gretchen A,Ruppert Kristine,Orchard Trevor J,Roberts Mark S
Diabetes care
OBJECTIVE:Cardiovascular risk prediction models are available for the general population (Framingham) and for type 2 diabetes (U.K. Prospective Diabetes Study [UKPDS] Risk Engine) but may not be appropriate in type 1 diabetes, as risk factors including younger age at diabetes onset and presence of diabetes complications are not considered. Therefore, our objective was to examine the accuracy of Framingham and UKPDS models for predicting coronary heart disease (CHD) in a type 1 diabetic cohort. RESEARCH DESIGN AND METHODS:Ten-year follow-up data from the Pittsburgh Epidemiology of Diabetes Complications (EDC) study, a prospective cohort study of 658 subjects with childhood-onset type 1 diabetes diagnosed between 1950 and 1980 first seen in 1986-1988, were analyzed. EDC study data were used to calculate the 10-year probability of CHD (fatal CHD, nonfatal myocardial infarction, or Q-waves) applying to the Framingham and UKPDS equations. RESULTS:Mean age at CHD onset was 39 years. When fatal/nonfatal myocardial infarction and CHD death were modeled, both the UKPDS and Framingham models showed significant lack of calibration (P < 0.0001) but moderate discrimination (0.76 UKPDS, 0.77 Framingham men, and 0.88 Framingham women). Both the UKPDS and Framingham models underestimated probability of events in highest risk deciles. CONCLUSIONS:Currently available CHD models poorly predict events in type 1 diabetes. Future research should focus on determining the risk factors accounting for the lack of fit and developing prediction models specific to this high-risk group.
10.2337/dc06-0290
Development and Validation of a Sudden Cardiac Death Prediction Model for the General Population.
Circulation
BACKGROUND:Most sudden cardiac death (SCD) events occur in the general population among persons who do not have any prior history of clinical heart disease. We sought to develop a predictive model of SCD among US adults. METHODS:We evaluated a series of demographic, clinical, laboratory, electrocardiographic, and echocardiographic measures in participants in the ARIC study (Atherosclerosis Risk in Communities) (n=13 677) and the CHS (Cardiovascular Health Study) (n=4207) who were free of baseline cardiovascular disease. Our initial objective was to derive a SCD prediction model using the ARIC cohort and validate it in CHS. Independent risk factors for SCD were first identified in the ARIC cohort to derive a 10-year risk model of SCD. We compared the prediction of SCD with non-SCD and all-cause mortality in both the derivation and validation cohorts. Furthermore, we evaluated whether the SCD prediction equation was better at predicting SCD than the 2013 American College of Cardiology/American Heart Association Cardiovascular Disease Pooled Cohort risk equation. RESULTS:There were a total of 345 adjudicated SCD events in our analyses, and the 12 independent risk factors in the ARIC study included age, male sex, black race, current smoking, systolic blood pressure, use of antihypertensive medication, diabetes mellitus, serum potassium, serum albumin, high-density lipoprotein, estimated glomerular filtration rate, and QTc interval. During a 10-year follow-up period, a model combining these risk factors showed good to excellent discrimination for SCD risk (c-statistic 0.820 in ARIC and 0.745 in CHS). The SCD prediction model was slightly better in predicting SCD than the 2013 American College of Cardiology/American Heart Association Pooled Cohort risk equations (c-statistic 0.808 in ARIC and 0.743 in CHS). Only the SCD prediction model, however, demonstrated similar and accurate prediction for SCD using both the original, uncalibrated score and the recalibrated equation. Finally, in the echocardiographic subcohort, a left ventricular ejection fraction <50% was present in only 1.1% of participants and did not enhance SCD prediction. CONCLUSIONS:Our study is the first to derive and validate a generalizable risk score that provides well-calibrated, absolute risk estimates across different risk strata in an adult population of white and black participants without a clinical diagnosis of cardiovascular disease.
10.1161/CIRCULATIONAHA.116.023042
A novel clinical risk prediction model for sudden cardiac death in hypertrophic cardiomyopathy (HCM risk-SCD).
O'Mahony Constantinos,Jichi Fatima,Pavlou Menelaos,Monserrat Lorenzo,Anastasakis Aristides,Rapezzi Claudio,Biagini Elena,Gimeno Juan Ramon,Limongelli Giuseppe,McKenna William J,Omar Rumana Z,Elliott Perry M,
European heart journal
AIMS:Hypertrophic cardiomyopathy (HCM) is a leading cause of sudden cardiac death (SCD) in young adults. Current risk algorithms provide only a crude estimate of risk and fail to account for the different effect size of individual risk factors. The aim of this study was to develop and validate a new SCD risk prediction model that provides individualized risk estimates. METHODS AND RESULTS:The prognostic model was derived from a retrospective, multi-centre longitudinal cohort study. The model was developed from the entire data set using the Cox proportional hazards model and internally validated using bootstrapping. The cohort consisted of 3675 consecutive patients from six centres. During a follow-up period of 24 313 patient-years (median 5.7 years), 198 patients (5%) died suddenly or had an appropriate implantable cardioverter defibrillator (ICD) shock. Of eight pre-specified predictors, age, maximal left ventricular wall thickness, left atrial diameter, left ventricular outflow tract gradient, family history of SCD, non-sustained ventricular tachycardia, and unexplained syncope were associated with SCD/appropriate ICD shock at the 15% significance level. These predictors were included in the final model to estimate individual probabilities of SCD at 5 years. The calibration slope was 0.91 (95% CI: 0.74, 1.08), C-index was 0.70 (95% CI: 0.68, 0.72), and D-statistic was 1.07 (95% CI: 0.81, 1.32). For every 16 ICDs implanted in patients with ≥4% 5-year SCD risk, potentially 1 patient will be saved from SCD at 5 years. A second model with the data set split into independent development and validation cohorts had very similar estimates of coefficients and performance when externally validated. CONCLUSION:This is the first validated SCD risk prediction model for patients with HCM and provides accurate individualized estimates for the probability of SCD using readily collected clinical parameters.
10.1093/eurheartj/eht439
A Validated Model for Sudden Cardiac Death Risk Prediction in Pediatric Hypertrophic Cardiomyopathy.
Circulation
BACKGROUND:Hypertrophic cardiomyopathy is the leading cause of sudden cardiac death (SCD) in children and young adults. Our objective was to develop and validate a SCD risk prediction model in pediatric hypertrophic cardiomyopathy to guide SCD prevention strategies. METHODS:In an international multicenter observational cohort study, phenotype-positive patients with isolated hypertrophic cardiomyopathy <18 years of age at diagnosis were eligible. The primary outcome variable was the time from diagnosis to a composite of SCD events at 5-year follow-up: SCD, resuscitated sudden cardiac arrest, and aborted SCD, that is, appropriate shock following primary prevention implantable cardioverter defibrillators. Competing risk models with cause-specific hazard regression were used to identify and quantify clinical and genetic factors associated with SCD. The cause-specific regression model was implemented using boosting, and tuned with 10 repeated 4-fold cross-validations. The final model was fitted using all data with the tuned hyperparameter value that maximizes the c-statistic, and its performance was characterized by using the c-statistic for competing risk models. The final model was validated in an independent external cohort (SHaRe [Sarcomeric Human Cardiomyopathy Registry], n=285). RESULTS:Overall, 572 patients met eligibility criteria with 2855 patient-years of follow-up. The 5-year cumulative proportion of SCD events was 9.1% (14 SCD, 25 resuscitated sudden cardiac arrests, and 14 aborted SCD). Risk predictors included age at diagnosis, documented nonsustained ventricular tachycardia, unexplained syncope, septal diameter -score, left ventricular posterior wall diameter score, left atrial diameter score, peak left ventricular outflow tract gradient, and presence of a pathogenic variant. Unlike in adults, left ventricular outflow tract gradient had an inverse association, and family history of SCD had no association with SCD. Clinical and clinical/genetic models were developed to predict 5-year freedom from SCD. Both models adequately discriminated between patients with and without SCD events with a c-statistic of 0.75 and 0.76, respectively, and demonstrated good agreement between predicted and observed events in the primary and validation cohorts (validation c-statistic 0.71 and 0.72, respectively). CONCLUSION:Our study provides a validated SCD risk prediction model with >70% prediction accuracy and incorporates risk factors that are unique to pediatric hypertrophic cardiomyopathy. An individualized risk prediction model has the potential to improve the application of clinical practice guidelines and shared decision making for implantable cardioverter defibrillator insertion. Registration: URL: https://www.clinicaltrials.gov; Unique identifier: NCT0403679.
10.1161/CIRCULATIONAHA.120.047235
Diet Quality Scores and Prediction of All-Cause, Cardiovascular and Cancer Mortality in a Pan-European Cohort Study.
PloS one
Scores of overall diet quality have received increasing attention in relation to disease aetiology; however, their value in risk prediction has been little examined. The objective was to assess and compare the association and predictive performance of 10 diet quality scores on 10-year risk of all-cause, CVD and cancer mortality in 451,256 healthy participants to the European Prospective Investigation into Cancer and Nutrition, followed-up for a median of 12.8y. All dietary scores studied showed significant inverse associations with all outcomes. The range of HRs (95% CI) in the top vs. lowest quartile of dietary scores in a composite model including non-invasive factors (age, sex, smoking, body mass index, education, physical activity and study centre) was 0.75 (0.72-0.79) to 0.88 (0.84-0.92) for all-cause, 0.76 (0.69-0.83) to 0.84 (0.76-0.92) for CVD and 0.78 (0.73-0.83) to 0.91 (0.85-0.97) for cancer mortality. Models with dietary scores alone showed low discrimination, but composite models also including age, sex and other non-invasive factors showed good discrimination and calibration, which varied little between different diet scores examined. Mean C-statistic of full models was 0.73, 0.80 and 0.71 for all-cause, CVD and cancer mortality. Dietary scores have poor predictive performance for 10-year mortality risk when used in isolation but display good predictive ability in combination with other non-invasive common risk factors.
10.1371/journal.pone.0159025
Development and validation of improved algorithms for the assessment of global cardiovascular risk in women: the Reynolds Risk Score.
Ridker Paul M,Buring Julie E,Rifai Nader,Cook Nancy R
JAMA
CONTEXT:Despite improved understanding of atherothrombosis, cardiovascular prediction algorithms for women have largely relied on traditional risk factors. OBJECTIVE:To develop and validate cardiovascular risk algorithms for women based on a large panel of traditional and novel risk factors. DESIGN, SETTING, AND PARTICIPANTS:Thirty-five factors were assessed among 24 558 initially healthy US women 45 years or older who were followed up for a median of 10.2 years (through March 2004) for incident cardiovascular events (an adjudicated composite of myocardial infarction, ischemic stroke, coronary revascularization, and cardiovascular death). We used data among a random two thirds (derivation cohort, n = 16 400) to develop new risk algorithms that were then tested to compare observed and predicted outcomes in the remaining one third of women (validation cohort, n = 8158). MAIN OUTCOME MEASURE:Minimization of the Bayes Information Criterion was used in the derivation cohort to develop the best-fitting parsimonious prediction models. In the validation cohort, we compared predicted vs actual 10-year cardiovascular event rates when the new algorithms were compared with models based on covariates included in the Adult Treatment Panel III risk score. RESULTS:In the derivation cohort, a best-fitting model (model A) and a clinically simplified model (model B, the Reynolds Risk Score) had lower Bayes Information Criterion scores than models based on covariates used in Adult Treatment Panel III. In the validation cohort, all measures of fit, discrimination, and calibration were improved when either model A or B was used. For example, among participants without diabetes with estimated 10-year risks according to the Adult Treatment Panel III of 5% to less than 10% (n = 603) or 10% to less than 20% (n = 156), model A reclassified 379 (50%) into higher- or lower-risk categories that in each instance more accurately matched actual event rates. Similar effects were achieved for clinically simplified model B limited to age, systolic blood pressure, hemoglobin A(1c) if diabetic, smoking, total and high-density lipoprotein cholesterol, high-sensitivity C-reactive protein, and parental history of myocardial infarction before age 60 years. Neither new algorithm provided substantive information about women at very low risk based on the published Adult Treatment Panel III score. CONCLUSION:We developed, validated, and demonstrated highly improved accuracy of 2 clinical algorithms for global cardiovascular risk prediction that reclassified 40% to 50% of women at intermediate risk into higher- or lower-risk categories.
10.1001/jama.297.6.611
Development and validation of a patient self-assessment score for diabetes risk.
Annals of internal medicine
BACKGROUND:National guidelines disagree on who should be screened for undiagnosed diabetes. No existing diabetes risk score is highly generalizable or widely followed. OBJECTIVE:To develop a new diabetes screening score and compare it with other available screening instruments (Centers for Disease Control and Prevention, American Diabetes Association, and U.S. Preventive Services Task Force guidelines; 2 American Diabetes Association risk questionnaires; and the Rotterdam model). DESIGN:Cross-sectional data. SETTING:NHANES (National Health and Nutrition Examination Survey) 1999 to 2004 for model development and 2005 to 2006, plus a combined cohort of 2 community studies, ARIC (Atherosclerosis Risk in Communities) Study and CHS (Cardiovascular Health Study), for validation. PARTICIPANTS:U.S. adults aged 20 years or older. MEASUREMENTS:A risk-scoring algorithm for undiagnosed diabetes, defined as fasting plasma glucose level of 7.0 mmol/L (126 mg/dL) or greater without known diabetes, was developed in the development data set. Logistic regression was used to determine which participant characteristics were independently associated with undiagnosed diabetes. The new algorithm and other methods were evaluated by standard diagnostic and feasibility measures. RESULTS:Age, sex, family history of diabetes, history of hypertension, obesity, and physical activity were associated with undiagnosed diabetes. In NHANES (ARIC/CHS), the cut-point of 5 or more points selected 35% (40%) of persons for diabetes screening and yielded a sensitivity of 79% (72%), specificity of 67% (62%), positive predictive value of 10% (10%), and positive likelihood ratio of 2.39 (1.89). In contrast, the comparison scores yielded a sensitivity of 44% to 100%, specificity of 10% to 73%, positive predictive value of 5% to 8%, and positive likelihood ratio of 1.11 to 1.98. LIMITATION:Data during pregnancy were not available. CONCLUSION:This easy-to-implement diabetes screening score seems to demonstrate improvements over existing methods. Studies are needed to evaluate it in diverse populations in real-world settings. PRIMARY FUNDING SOURCE:Clinical and Translational Science Center at Weill Cornell Medical College.
10.7326/0003-4819-151-11-200912010-00005
A clinical prediction model to identify patients at high risk of death in the emergency department.
Coslovsky Michael,Takala Jukka,Exadaktylos Aristomenis K,Martinolli Luca,Merz Tobias M
Intensive care medicine
PURPOSE:Rapid assessment and intervention is important for the prognosis of acutely ill patients admitted to the emergency department (ED). The aim of this study was to prospectively develop and validate a model predicting the risk of in-hospital death based on all available information available at the time of ED admission and to compare its discriminative performance with a non-systematic risk estimate by the triaging first health-care provider. METHODS:Prospective cohort analysis based on a multivariable logistic regression for the probability of death. RESULTS:A total of 8,607 consecutive admissions of 7,680 patients admitted to the ED of a tertiary care hospital were analysed. Most frequent APACHE II diagnostic categories at the time of admission were neurological (2,052, 24%), trauma (1,522, 18%), infection categories [1,328, 15%; including sepsis (357, 4.1%), severe sepsis (249, 2.9%), septic shock (27, 0.3%)], cardiovascular (1,022, 12%), gastrointestinal (848, 10%) and respiratory (449, 5%). The predictors of the final model were age, prolonged capillary refill time, blood pressure, mechanical ventilation, oxygen saturation index, Glasgow coma score and APACHE II diagnostic category. The model showed good discriminative ability, with an area under the receiver operating characteristic curve of 0.92 and good internal validity. The model performed significantly better than non-systematic triaging of the patient. CONCLUSIONS:The use of the prediction model can facilitate the identification of ED patients with higher mortality risk. The model performs better than a non-systematic assessment and may facilitate more rapid identification and commencement of treatment of patients at risk of an unfavourable outcome.
10.1007/s00134-015-3737-x
Prognostic and Prediction Tools in Bladder Cancer: A Comprehensive Review of the Literature.
Kluth Luis A,Black Peter C,Bochner Bernard H,Catto James,Lerner Seth P,Stenzl Arnulf,Sylvester Richard,Vickers Andrew J,Xylinas Evanguelos,Shariat Shahrokh F
European urology
CONTEXT:This review focuses on risk assessment and prediction tools for bladder cancer (BCa). OBJECTIVE:To review the current knowledge on risk assessment and prediction tools to enhance clinical decision making and counseling of patients with BCa. EVIDENCE ACQUISITION:A literature search in English was performed using PubMed in July 2013. Relevant risk assessment and prediction tools for BCa were selected. More than 1600 publications were retrieved. Special attention was given to studies that investigated the clinical benefit of a prediction tool. EVIDENCE SYNTHESIS:Most prediction tools for BCa focus on the prediction of disease recurrence and progression in non-muscle-invasive bladder cancer or disease recurrence and survival after radical cystectomy. Although these tools are helpful, recent prediction tools aim to address a specific clinical problem, such as the prediction of organ-confined disease and lymph node metastasis to help identify patients who might benefit from neoadjuvant chemotherapy. Although a large number of prediction tools have been reported in recent years, many of them lack external validation. Few studies have investigated the clinical utility of any given model as measured by its ability to improve clinical decision making. There is a need for novel biomarkers to improve the accuracy and utility of prediction tools for BCa. CONCLUSIONS:Decision tools hold the promise of facilitating the shared decision process, potentially improving clinical outcomes for BCa patients. Prediction models need external validation and assessment of clinical utility before they can be incorporated into routine clinical care. PATIENT SUMMARY:We looked at models that aim to predict outcomes for patients with bladder cancer (BCa). We found a large number of prediction models that hold the promise of facilitating treatment decisions for patients with BCa. However, many models are missing confirmation in a different patient cohort, and only a few studies have tested the clinical utility of any given model as measured by its ability to improve clinical decision making.
10.1016/j.eururo.2015.01.032
Development and validation of risk prediction equations to estimate survival in patients with colorectal cancer: cohort study.
BMJ (Clinical research ed.)
To develop and externally validate risk prediction equations to estimate absolute and conditional survival in patients with colorectal cancer. Cohort study. General practices in England providing data for the QResearch database linked to the national cancer registry. 44 145 patients aged 15-99 with colorectal cancer from 947 practices to derive the equations. The equations were validated in 15 214 patients with colorectal cancer from 305 different QResearch practices and 437 821 patients with colorectal cancer from the national cancer registry. The primary outcome was all cause mortality and secondary outcome was colorectal cancer mortality. Cause specific hazards models were used to predict risks of colorectal cancer mortality and other cause mortality accounting for competing risks, and these risk estimates were combined to obtain risks of all cause mortality. Separate equations were derived for men and women. Several variables were tested: age, ethnicity, deprivation score, cancer stage, cancer grade, surgery, chemotherapy, radiotherapy, smoking status, alcohol consumption, body mass index, family history of bowel cancer, anaemia, liver function test result, comorbidities, use of statins, use of aspirin, clinical values for anaemia, and platelet count. Measures of calibration and discrimination were determined in both validation cohorts at 1, 5, and 10 years. The final models included the following variables in men and women: age, deprivation score, cancer stage, cancer grade, smoking status, colorectal surgery, chemotherapy, family history of bowel cancer, raised platelet count, abnormal liver function, cardiovascular disease, diabetes, chronic renal disease, chronic obstructive pulmonary disease, prescribed aspirin at diagnosis, and prescribed statins at diagnosis. Improved survival in women was associated with younger age, earlier stage of cancer, well or moderately differentiated cancer grade, colorectal cancer surgery (adjusted hazard ratio 0.50), family history of bowel cancer (0.62), and prescriptions for statins (0.77) and aspirin (0.83) at diagnosis, with comparable results for men. The risk equations were well calibrated, with predicted risks closely matching observed risks. Discrimination was good in men and women in both validation cohorts. For example, the five year survival equations on the QResearch validation cohort explained 45.3% of the variation in time to colorectal cancer death for women, the D statistic was 1.86, and Harrell's C statistic was 0.80 (both measures of discrimination, indicating that the scores are able to distinguish between people with different levels of risk). The corresponding results for all cause mortality were 42.6%, 1.77, and 0.79. Risk prediction equations were developed and validated to estimate overall and conditional survival of patients with colorectal cancer accounting for an individual's clinical and demographic characteristics. These equations can provide more individualised accurate information for patients with colorectal cancer to inform decision making and follow-up.
10.1136/bmj.j2497
Development and validation of risk prediction algorithms to estimate future risk of common cancers in men and women: prospective cohort study.
BMJ open
OBJECTIVE:To derive and validate a set of clinical risk prediction algorithm to estimate the 10-year risk of 11 common cancers. DESIGN:Prospective open cohort study using routinely collected data from 753 QResearch general practices in England. We used 565 practices to develop the scores and 188 for validation. SUBJECTS:4.96 million patients aged 25-84 years in the derivation cohort; 1.64 million in the validation cohort. Patients were free of the relevant cancer at baseline. METHODS:Cox proportional hazards models in the derivation cohort to derive 10-year risk algorithms. Risk factors considered included age, ethnicity, deprivation, body mass index, smoking, alcohol, previous cancer diagnoses, family history of cancer, relevant comorbidities and medication. Measures of calibration and discrimination in the validation cohort. OUTCOMES:Incident cases of blood, breast, bowel, gastro-oesophageal, lung, oral, ovarian, pancreas, prostate, renal tract and uterine cancers. Cancers were recorded on any one of four linked data sources (general practitioner (GP), mortality, hospital or cancer records). RESULTS:We identified 228,241 incident cases during follow-up of the 11 types of cancer. Of these 25,444 were blood; 41,315 breast; 32,626 bowel, 12,808 gastro-oesophageal; 32,187 lung; 4811 oral; 6635 ovarian; 7119 pancreatic; 35,256 prostate; 23,091 renal tract; 6949 uterine cancers. The lung cancer algorithm had the best performance with an R(2) of 64.2%; D statistic of 2.74; receiver operating characteristic curve statistic of 0.91 in women. The sensitivity for the top 10% of women at highest risk of lung cancer was 67%. Performance of the algorithms in men was very similar to that for women. CONCLUSIONS:We have developed and validated a prediction models to quantify absolute risk of 11 common cancers. They can be used to identify patients at high risk of cancers for prevention or further assessment. The algorithms could be integrated into clinical computer systems and used to identify high-risk patients. WEB CALCULATOR:There is a simple web calculator to implement the Qcancer 10 year risk algorithm together with the open source software for download (available at http://qcancer.org/10yr/).
10.1136/bmjopen-2015-007825
Multicentre external validation of IOTA prediction models and RMI by operators with varied training.
Sayasneh A,Wynants L,Preisler J,Kaijser J,Johnson S,Stalder C,Husicka R,Abdallah Y,Raslan F,Drought A,Smith A A,Ghaem-Maghami S,Epstein E,Van Calster B,Timmerman D,Bourne T
British journal of cancer
BACKGROUND:Correct characterisation of ovarian tumours is critical to optimise patient care. The purpose of this study is to evaluate the diagnostic performance of the International Ovarian Tumour Analysis (IOTA) logistic regression model (LR2), ultrasound Simple Rules (SR), the Risk of Malignancy Index (RMI) and subjective assessment (SA) for preoperative characterisation of adnexal masses, when ultrasonography is performed by examiners with different background training and experience. METHODS:A 2-year prospective multicentre cross-sectional study. Thirty-five level II ultrasound examiners contributed in three UK hospitals. Transvaginal ultrasonography was performed using a standardised approach. The final outcome was the surgical findings and histological diagnosis. To characterise the adnexal masses, the six-variable prediction model (LR2) with a cutoff of 0.1, the RMI with cutoff of 200, ten SR (five rules for malignancy and five rules for benignity) and SA were applied. The area under the curves (AUCs) for performance of LR2 and RMI were calculated. Diagnostic performance measures for all models assessed were sensitivity, specificity, positive and negative likelihood ratios (LR+ and LR-), and the diagnostic odds ratio (DOR). RESULTS:Nine-hundred and sixty-two women with adnexal masses underwent transvaginal ultrasonography, whereas 255 had surgery. Prevalence of malignancy was 29% (49 primary invasive epithelial ovarian cancers, 18 borderline ovarian tumours, and 7 metastatic tumours). The AUCs for LR2 and RMI for all masses were 0.94 (95% confidence interval (CI): 0.89-0.97) and 0.90 (95% CI: 0.83-0.94), respectively. In premenopausal women, LR2-RMI difference was 0.09 (95% CI: 0.03-0.15) compared with -0.02 (95% CI: -0.08 to 0.04) in postmenopausal women. For all masses, the DORs for LR2, RMI, SR+SA (using SA when SR inapplicable), SR+MA (assuming malignancy when SR inapplicable), and SA were 62 (95% CI: 27-142), 43 (95% CI: 19-97), 109 (95% CI: 44-274), 66 (95% CI: 27-158), and 70 (95% CI: 30-163), respectively. CONCLUSION:Overall, the test performance of IOTA prediction models and rules as well as the RMI was maintained in examiners with varying levels of training and experience.
10.1038/bjc.2013.224
Prediction and Risk Stratification of Kidney Outcomes in IgA Nephropathy.
Chen Tingyu,Li Xiang,Li Yingxue,Xia Eryu,Qin Yong,Liang Shaoshan,Xu Feng,Liang Dandan,Zeng Caihong,Liu Zhihong
American journal of kidney diseases : the official journal of the National Kidney Foundation
RATIONALE & OBJECTIVE:Immunoglobulin A nephropathy (IgAN) is common worldwide and has heterogeneous phenotypes. Predicting long-term outcomes and stratifying risk are important for clinical decision making and designing future clinical trials. STUDY DESIGN:Multicenter retrospective cohort study of 2,047 patients with IgAN. SETTING & PARTICIPANTS:Derivation and validation cohorts composed of 1,022 Chinese patients with IgAN from a single center and 1,025 patients with IgAN from 18 renal centers, respectively. PREDICTORS:36 characteristics, including demographic, clinical, and pathologic variables. OUTCOMES:Combined event of end-stage kidney disease or 50% reduction in estimated glomerular filtration rate within 5 years after diagnostic kidney biopsy. ANALYTICAL APPROACH:A gradient tree boosting method implemented in the eXtreme Gradient Boosting (XGBoost) system was used to select the 10 most important variables from 36 candidate variables. Stepwise Cox regression analysis was used to derive a simplified scoring scale model (SSM) based on these 10 variables. Model discrimination and calibration were assessed using the C statistic and Hosmer-Lemeshow test. Risk stratification of the SSM was evaluated using Kaplan-Meier analysis. RESULTS:In the derivation and validation cohorts, 74 and 114 patients reached the outcome, respectively. XGBoost predicted the outcome with a C statistic of 0.84 (95% CI, 0.80-0.88) for the validation cohort. The SSM included 3 variables: urine protein excretion, global sclerosis, and tubular atrophy/interstitial fibrosis. Using Kaplan-Meier analysis, the SSM identified significant risk stratification (P < 0.001). LIMITATIONS:Retrospective study design, application for other ethnic groups needs to be verified. CONCLUSIONS:A prediction model using routinely available characteristics and based on the combination of a machine learning algorithm and survival analysis can stratify risk for kidney disease progression in the setting of IgAN. An online calculator, the Nanjing IgAN Risk Stratification System, permits easy implementation of this model.
10.1053/j.ajkd.2019.02.016
Screening and Bioinformatics Analysis of IgA Nephropathy Gene Based on GEO Databases.
BioMed research international
PURPOSE:To identify novel biomarkers of IgA nephropathy (IgAN) through bioinformatics analysis and elucidate the possible molecular mechanism. METHODS:The GSE93798 and GSE73953 datasets containing microarray data from IgAN patients and healthy controls were downloaded from the GEO database and analyzed by the GEO2R web tool to obtain different expressed genes (DEGs). Gene Ontology (GO), Kyoto Encyclopedia of Genes and Genomes (KEGG) enrichment analysis, protein-protein interaction (PPI), and Biological Networks Gene Oncology tool (BiNGO) were then performed to elucidate the molecular mechanism of IgAN. RESULTS:A total of 223 DEGs were identified, of which 21 were hub genes, and involved in inflammatory response, cellular response to lipopolysaccharide, transcription factor activity, extracellular exosome, TNF signaling pathway, and MAPK signaling pathway. CONCLUSIONS:TNF and MAPK pathways likely form the basis of IgAN progression, and JUN/JUNB, FOS, NR4A1/2, EGR1, and FOSL1/2 are novel prognostic biomarkers of IgAN.
10.1155/2019/8794013
Clinicopathological Features to Predict Progression of IgA Nephropathy with Mild Proteinuria.
Chen Ding,Liu Jian,Duan Shuwei,Chen Pu,Tang Li,Zhang Li,Feng Zhe,Cai Guangyan,Wu Jie,Chen Xiangmei
Kidney & blood pressure research
BACKGROUND/AIMS:In the past, little attention has been paid to patients with IgA nephropathy (IgAN) who had minimal proteinuria upon the onset. The aim of this study was to analyze the clinicopathological features and the prognostic factors in patients with IgA nephropathy. METHODS:Data of patients that had their first renal biopsy in our hospital and were diagnosed with primary IgAN with proteinuria <1 g/d from January 1995 to December 2014 were retrospectively examined. Clinical records of the clinicopathological features, renal function, and proteinuria were collected and investigated. The factors affecting the renal function and proteinuria were analyzed by Cox regression. The predictive efficiencies of clinical and pathological models were evaluated by Harrell concordance index (C-index). RESULTS:A total of 506 patients with IgA nephropathy were included in this study. (1) Baseline proteinuria greater than 0.5 g/d was positively associated with Oxford M, S, and T lesions. eGFR less than 90 mL/min/1.73 m2 were positively associated with Oxford T. (2) In the follow-up with a median of 50 months, 82 patients (16.2%) achieved complete clinical remission (CCR), whereas 54 patients (10.6%) showed an increase in creatinine by more than 50% (not progressing to end-stage renal disease). The cumulative proportion of creatinine increased >50%, and the values obtained by life-table analysis in 10, 15, and 20 years were 15%, 21%, and 22%, respectively. Significant differences were found in baseline age, proteinuria, and Oxford T between the group of creatinine increase >50% and the CCR group. (4) Multivariate COX regression showed that baseline age and proteinuria > 0.5 g/d were independent risk factors of adverse outcome. C-index suggested that the clinical model was more effective than the pathological models in predicting endpoint events. (5) Effect of the mean value during the follow-up on adverse endpoint events: Multivariate COX regression found that the mean proteinuria during follow-up was an independent influencing factor for the increase of creatinine by more than 50%. CONCLUSION:(1) Proteinuria > 0.5g/d and eGFR < 90 mL/min/1.73 m2 may predict more severe pathological changes; (2) With the increase in age and baseline proteinuria, the risks of adverse endpoint events would increase significantly; (3) Pathology could roughly predict the adverse endpoint events but is less efficient than the clinical indicators; (4) Data during follow-up suggested that the patients should regularly test their renal function and proactively control their proteinuria.
10.1159/000487901
Evaluating a New International Risk-Prediction Tool in IgA Nephropathy.
Barbour Sean J,Coppo Rosanna,Zhang Hong,Liu Zhi-Hong,Suzuki Yusuke,Matsuzaki Keiichi,Katafuchi Ritsuko,Er Lee,Espino-Hernandez Gabriela,Kim S Joseph,Reich Heather N,Feehally John,Cattran Daniel C,
JAMA internal medicine
Importance:Although IgA nephropathy (IgAN) is the most common glomerulonephritis in the world, there is no validated tool to predict disease progression. This limits patient-specific risk stratification and treatment decisions, clinical trial recruitment, and biomarker validation. Objective:To derive and externally validate a prediction model for disease progression in IgAN that can be applied at the time of kidney biopsy in multiple ethnic groups worldwide. Design, Setting, and Participants:We derived and externally validated a prediction model using clinical and histologic risk factors that are readily available in clinical practice. Large, multi-ethnic cohorts of adults with biopsy-proven IgAN were included from Europe, North America, China, and Japan. Main Outcomes and Measures:Cox proportional hazards models were used to analyze the risk of a 50% decline in estimated glomerular filtration rate (eGFR) or end-stage kidney disease, and were evaluated using the R2D measure, Akaike information criterion (AIC), C statistic, continuous net reclassification improvement (NRI), integrated discrimination improvement (IDI), and calibration plots. Results:The study included 3927 patients; mean age, 35.4 (interquartile range, 28.0-45.4) years; and 2173 (55.3%) were men. The following prediction models were created in a derivation cohort of 2781 patients: a clinical model that included eGFR, blood pressure, and proteinuria at biopsy; and 2 full models that also contained the MEST histologic score, age, medication use, and either racial/ethnic characteristics (white, Japanese, or Chinese) or no racial/ethnic characteristics, to allow application in other ethnic groups. Compared with the clinical model, the full models with and without race/ethnicity had better R2D (26.3% and 25.3%, respectively, vs 20.3%) and AIC (6338 and 6379, respectively, vs 6485), significant increases in C statistic from 0.78 to 0.82 and 0.81, respectively (ΔC, 0.04; 95% CI, 0.03-0.04 and ΔC, 0.03; 95% CI, 0.02-0.03, respectively), and significant improvement in reclassification as assessed by the NRI (0.18; 95% CI, 0.07-0.29 and 0.51; 95% CI, 0.39-0.62, respectively) and IDI (0.07; 95% CI, 0.06-0.08 and 0.06; 95% CI, 0.05-0.06, respectively). External validation was performed in a cohort of 1146 patients. For both full models, the C statistics (0.82; 95% CI, 0.81-0.83 with race/ethnicity; 0.81; 95% CI, 0.80-0.82 without race/ethnicity) and R2D (both 35.3%) were similar or better than in the validation cohort, with excellent calibration. Conclusions and Relevance:In this study, the 2 full prediction models were shown to be accurate and validated methods for predicting disease progression and patient risk stratification in IgAN in multi-ethnic cohorts, with additional applications to clinical trial design and biomarker research.
10.1001/jamainternmed.2019.0600
Prediction of ESRD in IgA Nephropathy Patients from an Asian Cohort: A Random Forest Model.
Liu Yexin,Zhang Yan,Liu Di,Tan Xia,Tang Xiaofang,Zhang Fan,Xia Ming,Chen Guochun,He Liyu,Zhou Letian,Zhu Xuejing,Liu Hong
Kidney & blood pressure research
BACKGROUND/AIMS:There is an increasing risk of end-stage renal disease (ESRD) among Asian people with immunoglobulin A nephropathy (IgAN). A computer-aided system for ESRD prediction in Asian IgAN patients has not been well studied. METHODS:We retrospectively reviewed biopsy-proven IgAN patients treated at the Department of Nephrology of the Second Xiangya Hospital from January 2009 to November 2013. Demographic and clinicopathological data were obtained within 1 month of renal biopsy. A random forest (RF) model was employed to predict the ESRD status in IgAN patients. All cases were initially trained and validated, taking advantage of the out-of-bagging(OOB) error. Predictors used in the model were selected according to the Gini impurity index in the RF model and verified by logistic regression analysis. The area under the receiver operating characteristic(ROC) curve (AUC) and F-measure were used to evaluate the RF model. RESULTS:A total of 262 IgAN patients were enrolled in this study with a median follow-up time of 4.66 years. The importance rankings of predictors of ESRD in the RF model were first obtained, indicating some of the most important predictors. Logistic regression also showed that these factors were statistically associated with ESRD status. We first trained an initial RF model using gender, age, hypertension, serum creatinine, 24-hour proteinuria and histological grading suggested by the Clinical Decision Support System for IgAN (CDSS, www.IgAN.net). This 6-predictor model achieved a F-measure of 0.8 and an AUC of 92.57%. By adding Oxford-MEST scores, this model outperformed the initial model with an improved AUC (96.1%) and F-measure (0.823). When C3 staining was incorporated, the AUC was 97.29% and F-measure increased to 0.83. Adding the estimated glomerular filtration rate (eGFR) improved the AUC to 95.45%. We also observed improved performance of the model with additional inputs of blood urea nitrogen (BUN), uric acid, hemoglobin and albumin. CONCLUSION:In addition to the predictors in the CDSS, Oxford-MEST scores, C3 staining and eGFR conveyed additional information for ESRD prediction in Chinese IgAN patients using a RF model.
10.1159/000495818
Validation of a prediction model for long-term outcome of aphasia after stroke.
Nouwens Femke,Visch-Brink Evy G,El Hachioui Hanane,Lingsma Hester F,van de Sandt-Koenderman Mieke W M E,Dippel Diederik W J,Koudstaal Peter J,de Lau Lonneke M L
BMC neurology
BACKGROUND:About 30% of stroke patients suffer from aphasia. As aphasia strongly affects daily life, most patients request a prediction of outcome of their language function. Prognostic models provide predictions of outcome, but external validation is essential before models can be used in clinical practice. We aim to externally validate the prognostic model from the Sequential Prognostic Evaluation of Aphasia after stroKe (SPEAK-model) for predicting the long-term outcome of aphasia caused by stroke. METHODS:We used data from the Rotterdam Aphasia Therapy Study - 3 (RATS-3), a multicenter RCT with inclusion criteria similar to SPEAK, an observational prospective study. Baseline assessment in SPEAK was four days after stroke and in RATS-3 eight days. Outcome of the SPEAK-model was the Aphasia Severity Rating Scale (ASRS) at 1 year, dichotomized into good (ASRS-score of 4 or 5) and poor outcome (ASRS-score < 4). In RATS-3, ASRS-scores at one year were not available, but we could use six month ASRS-scores as outcome. Model performance was assessed with calibration and discrimination. RESULTS:We included 131 stroke patients with first-ever aphasia. At six months, 86 of 124 (68%) had a good outcome, whereas the model predicted 88%. Discrimination of the model was good with an area under the receiver operation characteristic curve of 0.87 (95%CI: 0.81-0.94), but calibration was unsatisfactory. The model overestimated the probability of good outcome (calibration-in-the-large α = - 1.98) and the effect of the predictors was weaker in the validation data than in the derivation data (calibration slope β = 0.88). We therefore recalibrated the model to predict good outcome at six months. CONCLUSION:The original model, renamed SPEAK-12, has good discriminative properties, but needs further external validation. After additional external validation, the updated SPEAK-model, SPEAK-6, may be used in daily practice to discriminate between patients with good and patients with poor outcome of aphasia at six months after stroke. TRIAL REGISTRATION:RATS-3 was registered on January 13th 2012 in the Netherlands Trial Register: NTR3271 . SPEAK was not listed in a trial registry.
10.1186/s12883-018-1174-5
Development and validation of a dynamic outcome prediction model for paracetamol-induced acute liver failure: a cohort study.
Bernal William,Wang Yanzhong,Maggs James,Willars Christopher,Sizer Elizabeth,Auzinger Georg,Murphy Nicholas,Harding Damian,Elsharkawy Ahmed,Simpson Kenneth,Larsen Fin Stolze,Heaton Nigel,O'Grady John,Williams Roger,Wendon Julia
The lancet. Gastroenterology & hepatology
BACKGROUND:Early, accurate prediction of survival is central to management of patients with paracetamol-induced acute liver failure to identify those needing emergency liver transplantation. Current prognostic tools are confounded by recent improvements in outcome independent of emergency liver transplantation, and constrained by static binary outcome prediction. We aimed to develop a simple prognostic tool to reflect current outcomes and generate a dynamic updated estimation of risk of death. METHODS:Patients with paracetamol-induced acute liver failure managed at intensive care units in the UK (London, Birmingham, and Edinburgh) and Denmark (Copenhagen) were studied. We developed prognostic models, excluding patients who underwent transplantation, using Cox proportional hazards in a derivation dataset, and tested in initial and recent external validation datasets. Mortality was estimated in patients who had emergency liver transplantation. Model discrimination was assessed using area under receiver operating characteristic curve (AUROC) and calibration by root mean square error (RMSE). Admission (day 1) variables of age, Glasgow coma scale, arterial pH and lactate, creatinine, international normalised ratio (INR), and cardiovascular failure were used to derive an initial predictive model, with a second (day 2) model including additional changes in INR and lactate. FINDINGS:We developed and validated new high-performance statistical models to support decision making in patients with paracetamol-induced acute liver failure. Applied to the derivation dataset (n=350), the AUROC for 30-day survival was 0·92 (95% CI 0·88-0·96) using the day 1 model and 0·93 (0·88-0·97) using the day 2 model. In the initial validation dataset (n=150), the AUROC for 30-day survival was 0·89 (0·84-0·95) using the day 1 model and 0·90 (0·85-0·95) using the day 2 model. Assessment of calibration using RMSE in prediction of 30-day survival gave values of 0·1642 for the day 1 model and 0·0626 for the day 2 model. In the external validation dataset (n=412), the AUROC for 30-day survival was 0·91 (0·87-0·94) using the day 1 model and 0·91 (0·88-0·95) using the day 2 model, and assessment of calibration using RMSE gave values of 0·079 for the day 1 model and 0·107 for the day 2 model. Applied to patients who underwent emergency liver transplantation (n=116), median predicted 30-day survival was 51% (95% CI 33-85). INTERPRETATION:The models developed here show very good discrimination and calibration, confirmed in independent datasets, and suggest that many patients undergoing transplantation based on existing criteria might have survived with medical management alone. The role and indications for emergency liver transplantation in paracetamol-induced acute liver failure require re-evaluation. FUNDING:Foundation for Liver Research.
10.1016/S2468-1253(16)30007-3
Non-alcoholic fatty liver disease (NAFLD) fibrosis score predicts 6.6-year overall mortality of Chinese patients with NAFLD.
Xun Yun-Hao,Guo Jian-Chun,Lou Guo-Qiang,Jiang Yan-Ming,Zhuang Zhen-Jie,Zhu Meng-Fei,Luo Yan,Ma Xiao-Jie,Liu Jing,Bian Dong-Xue,Shi Jun-Ping
Clinical and experimental pharmacology & physiology
The non-alcoholic fatty liver disease (NAFLD) fibrosis score (NFS) has emerged as a useful predictor of long-term outcome in NAFLD patients. We evaluated the predictive performance of the NFS for overall mortality in a Chinese population with NAFLD. All NAFLD patients diagnosed ultrasonographically at Xixi Hospital of Hangzhou between 1996 and 2011 were retrospectively recruited to the study. Outcome was determined by interview and causes of death were confirmed by medical records. The area under the receiver operating characteristic curve (AUCROC ) was used to determine the predictive accuracy of the NFS, BARD (body mass index, aspartate aminotransferase (AST)/alanine aminotransferase (ALT) ratio, diabetes) score, FIB-4 index and the AST/platelet ratio index (APRI) for mortality. Data from a total of 180 eligible patients (median age 39 years; 96 men) were analysed, with 12 deaths over a median follow-up period of 6.6 years (range 0.5-14.8 years). Using Cox model analysis, the NFS as a continuous variable was identified as the only predictor for all-cause mortality (hazard ratio 2.743, 95% confidence interval (CI) 1.670-4.504). The NFS yielded the highest AUCROC of 0.828 (95% CI 0.728-0.928, P < 0.05), followed by the FIB-4 index, APRI and BARD score (AUCROC 0.806 (P < 0.05), 0.732 (P < 0.05) and 0.632, respectively). The data indicated that the NFS is a useful predictor of 6.6-year all-cause mortality for Chinese patients with NAFLD.
10.1111/1440-1681.12260
A PDGFRβ-based score predicts significant liver fibrosis in patients with chronic alcohol abuse, NAFLD and viral liver disease.
Lambrecht Joeri,Verhulst Stefaan,Mannaerts Inge,Sowa Jan-Peter,Best Jan,Canbay Ali,Reynaert Hendrik,van Grunsven Leo A
EBioMedicine
BACKGROUND:Platelet Derived Growth Factor Receptor beta (PDGFRβ) has been associated to hepatic stellate cell activation and has been the target of multiple therapeutic studies. However, little is known concerning its use as a diagnostic agent. METHODS:Circulating PDGFRβ levels were analysed in a cohort of patients with liver fibrosis/cirrhosis due to chronic alcohol abuse, viral hepatitis, or non-alcoholic fatty liver disease (NAFLD). The diagnostic performance of PDGFRβ as individual blood parameter, or in combination with other metabolic factors was evaluated. FINDINGS:sPDGFRβ levels are progressively increased with increasing fibrosis stage and the largest difference was observed in patients with significant fibrosis, compared to no or mild fibrosis. The accuracy of sPDGFRβ-levels predicting fibrosis could be increased by combining it with albumin levels and platelet counts into a novel diagnostic algorithm, the PRTA-score, generating a predictive value superior to Fib-4, APRI, and AST/ALT. The sPDGFRβ levels and the PRTA-score are independent of liver disease aetiology, thus overcoming one of the major weaknesses of current non-invasive clinical and experimental scores. Finally, we confirmed the diagnostic value of sPDGFRβ levels and the PRTA-score in an independent patient cohort with NAFLD which was staged for fibrosis by liver biopsy. INTERPRETATION:The PRTA-score is an accurate tool for detecting significant liver fibrosis in a broad range of liver disease aetiologies. FUND: Vrije Universiteit Brussel, the Institute for the Promotion of Innovation through Science and Technology in Flanders (IWT-Flanders) (HILIM-3D; SBO140045), and the Fund of Scientific Research Flanders (FWO).
10.1016/j.ebiom.2019.04.036
Clinical prediction models in psychiatry: a systematic review of two decades of progress and challenges.
Molecular psychiatry
Recent years have seen the rapid proliferation of clinical prediction models aiming to support risk stratification and individualized care within psychiatry. Despite growing interest, attempts to synthesize current evidence in the nascent field of precision psychiatry have remained scarce. This systematic review therefore sought to summarize progress towards clinical implementation of prediction modeling for psychiatric outcomes. We searched MEDLINE, PubMed, Embase, and PsychINFO databases from inception to September 30, 2020, for English-language articles that developed and/or validated multivariable models to predict (at an individual level) onset, course, or treatment response for non-organic psychiatric disorders (PROSPERO: CRD42020216530). Individual prediction models were evaluated based on three key criteria: (i) mitigation of bias and overfitting; (ii) generalizability, and (iii) clinical utility. The Prediction model Risk Of Bias ASsessment Tool (PROBAST) was used to formally appraise each study's risk of bias. 228 studies detailing 308 prediction models were ultimately eligible for inclusion. 94.5% of developed prediction models were deemed to be at high risk of bias, largely due to inadequate or inappropriate analytic decisions. Insufficient internal validation efforts (within the development sample) were also observed, while only one-fifth of models underwent external validation in an independent sample. Finally, our search identified just one published model whose potential utility in clinical practice was formally assessed. Our findings illustrated significant growth in precision psychiatry with promising progress towards real-world application. Nevertheless, these efforts have been inhibited by a preponderance of bias and overfitting, while the generalizability and clinical utility of many published models has yet to be formally established. Through improved methodological rigor during initial development, robust evaluations of reproducibility via independent validation, and evidence-based implementation frameworks, future research has the potential to generate risk prediction tools capable of enhancing clinical decision-making in psychiatric care.
10.1038/s41380-022-01528-4
Development and validation of a trans-ancestry polygenic risk score for type 2 diabetes in diverse populations.
Genome medicine
BACKGROUND:Type 2 diabetes (T2D) is a worldwide scourge caused by both genetic and environmental risk factors that disproportionately afflicts communities of color. Leveraging existing large-scale genome-wide association studies (GWAS), polygenic risk scores (PRS) have shown promise to complement established clinical risk factors and intervention paradigms, and improve early diagnosis and prevention of T2D. However, to date, T2D PRS have been most widely developed and validated in individuals of European descent. Comprehensive assessment of T2D PRS in non-European populations is critical for equitable deployment of PRS to clinical practice that benefits global populations. METHODS:We integrated T2D GWAS in European, African, and East Asian populations to construct a trans-ancestry T2D PRS using a newly developed Bayesian polygenic modeling method, and assessed the prediction accuracy of the PRS in the multi-ethnic Electronic Medical Records and Genomics (eMERGE) study (11,945 cases; 57,694 controls), four Black cohorts (5137 cases; 9657 controls), and the Taiwan Biobank (4570 cases; 84,996 controls). We additionally evaluated a post hoc ancestry adjustment method that can express the polygenic risk on the same scale across ancestrally diverse individuals and facilitate the clinical implementation of the PRS in prospective cohorts. RESULTS:The trans-ancestry PRS was significantly associated with T2D status across the ancestral groups examined. The top 2% of the PRS distribution can identify individuals with an approximately 2.5-4.5-fold of increase in T2D risk, which corresponds to the increased risk of T2D for first-degree relatives. The post hoc ancestry adjustment method eliminated major distributional differences in the PRS across ancestries without compromising its predictive performance. CONCLUSIONS:By integrating T2D GWAS from multiple populations, we developed and validated a trans-ancestry PRS, and demonstrated its potential as a meaningful index of risk among diverse patients in clinical settings. Our efforts represent the first step towards the implementation of the T2D PRS into routine healthcare.
10.1186/s13073-022-01074-2
Deep-learning models for the detection and incidence prediction of chronic kidney disease and type 2 diabetes from retinal fundus images.
Zhang Kang,Liu Xiaohong,Xu Jie,Yuan Jin,Cai Wenjia,Chen Ting,Wang Kai,Gao Yuanxu,Nie Sheng,Xu Xiaodong,Qin Xiaoqi,Su Yuandong,Xu Wenqin,Olvera Andrea,Xue Kanmin,Li Zhihuan,Zhang Meixia,Zeng Xiaoxi,Zhang Charlotte L,Li Oulan,Zhang Edward E,Zhu Jie,Xu Yiming,Kermany Daniel,Zhou Kaixin,Pan Ying,Li Shaoyun,Lai Iat Fan,Chi Ying,Wang Changuang,Pei Michelle,Zang Guangxi,Zhang Qi,Lau Johnson,Lam Dennis,Zou Xiaoguang,Wumaier Aizezi,Wang Jianquan,Shen Yin,Hou Fan Fan,Zhang Ping,Xu Tao,Zhou Yong,Wang Guangyu
Nature biomedical engineering
Regular screening for the early detection of common chronic diseases might benefit from the use of deep-learning approaches, particularly in resource-poor or remote settings. Here we show that deep-learning models can be used to identify chronic kidney disease and type 2 diabetes solely from fundus images or in combination with clinical metadata (age, sex, height, weight, body-mass index and blood pressure) with areas under the receiver operating characteristic curve of 0.85-0.93. The models were trained and validated with a total of 115,344 retinal fundus photographs from 57,672 patients and can also be used to predict estimated glomerulal filtration rates and blood-glucose levels, with mean absolute errors of 11.1-13.4 ml min per 1.73 m and 0.65-1.1 mmol l, and to stratify patients according to disease-progression risk. We evaluated the generalizability of the models for the identification of chronic kidney disease and type 2 diabetes with population-based external validation cohorts and via a prospective study with fundus images captured with smartphones, and assessed the feasibility of predicting disease progression in a longitudinal cohort.
10.1038/s41551-021-00745-6
Early prognosis in traumatic brain injury: from prophecies to predictions.
Lingsma Hester F,Roozenbeek Bob,Steyerberg Ewout W,Murray Gordon D,Maas Andrew I R
The Lancet. Neurology
Traumatic brain injury (TBI) is a heterogeneous condition that encompasses a broad spectrum of disorders. Outcome can be highly variable, particularly in more severely injured patients. Despite the association of many variables with outcome, prognostic predictions are notoriously difficult to make. Multivariable analysis has identified age, clinical severity, CT abnormalities, systemic insults (hypoxia and hypotension), and laboratory variables as relevant factors to include in models to predict outcome in individual patients. Advances in statistical modelling and the availability of large datasets have facilitated the development of prognostic models that have greater performance and generalisability. Two prediction models are currently available, both of which have been developed on large datasets with state-of-the-art methods, and offer new opportunities. We see great potential for their use in clinical practice, research, and policy making, as well as for assessment of the quality of health-care delivery. Continued development, refinement, and validation is advocated, together with assessment of the clinical impact of prediction models, including treatment response.
10.1016/S1474-4422(10)70065-X
AML: Predicting the Unpredictable.
Takahashi Koichi
Cell stem cell
Acute myeloid leukemia (AML) has been considered an unpredictable and unpreventable disease. Recently in Nature, Abelson et al. (2018) presented two models of AML risk prediction, one using the detection of high-risk age-related clonal hematopoiesis and the other using dynamic clinical attributes available from routine blood work.
10.1016/j.stem.2018.07.005
Development of a Novel Risk Prediction Model for Sudden Cardiac Death in Childhood Hypertrophic Cardiomyopathy (HCM Risk-Kids).
JAMA cardiology
Importance:Sudden cardiac death (SCD) is the most common mode of death in childhood hypertrophic cardiomyopathy (HCM), but there is no validated algorithm to identify those at highest risk. Objective:To develop and validate an SCD risk prediction model that provides individualized risk estimates. Design, Setting, and Participants:A prognostic model was developed from a retrospective, multicenter, longitudinal cohort study of 1024 consecutively evaluated patients aged 16 years or younger with HCM. The study was conducted from January 1, 1970, to December 31, 2017. Exposures:The model was developed using preselected predictor variables (unexplained syncope, maximal left-ventricular wall thickness, left atrial diameter, left-ventricular outflow tract gradient, and nonsustained ventricular tachycardia) identified from the literature and internally validated using bootstrapping. Main Outcomes and Measures:A composite outcome of SCD or an equivalent event (aborted cardiac arrest, appropriate implantable cardioverter defibrillator therapy, or sustained ventricular tachycardia associated with hemodynamic compromise). Results:Of the 1024 patients included in the study, 699 were boys (68.3%); mean (interquartile range [IQR]) age was 11 (7-14) years. Over a median follow-up of 5.3 years (IQR, 2.6-8.3; total patient years, 5984), 89 patients (8.7%) died suddenly or had an equivalent event (annual event rate, 1.49; 95% CI, 1.15-1.92). The pediatric model was developed using preselected variables to predict the risk of SCD. The model's ability to predict risk at 5 years was validated; the C statistic was 0.69 (95% CI, 0.66-0.72), and the calibration slope was 0.98 (95% CI, 0.59-1.38). For every 10 implantable cardioverter defibrillators implanted in patients with 6% or more of a 5-year SCD risk, 1 patient may potentially be saved from SCD at 5 years. Conclusions and Relevance:This new, validated risk stratification model for SCD in childhood HCM may provide individualized estimates of risk at 5 years using readily obtained clinical risk factors. External validation studies are required to demonstrate the accuracy of this model's predictions in diverse patient populations.
10.1001/jamacardio.2019.2861
Clinical variables and biomarkers in prediction of cognitive impairment in patients with newly diagnosed Parkinson's disease: a cohort study.
The Lancet. Neurology
BACKGROUND:Parkinson's disease is associated with an increased incidence of cognitive impairment and dementia. Predicting who is at risk of cognitive decline early in the disease course has implications for clinical prognosis and for stratification of participants in clinical trials. We assessed the use of clinical information and biomarkers as predictive factors for cognitive decline in patients with newly diagnosed Parkinson's disease. METHODS:The Parkinson's Progression Markers Initiative (PPMI) study is a cohort study in patients with newly diagnosed Parkinson's disease. We evaluated cognitive performance (Montreal Cognitive Assessment [MoCA] scores), demographic and clinical data, APOE status, and biomarkers (CSF and dopamine transporter [DAT] imaging results). Using change in MoCA scores over 2 years, MoCA scores at 2 years' follow-up, and a diagnosis of cognitive impairment (combined mild cognitive impairment or dementia) at 2 years as outcome measures, we assessed the predictive values of baseline clinical variables and separate or combined additions of APOE status, DAT imaging, and CSF biomarkers. We did univariate and multivariate linear analyses with MoCA change scores between baseline and 2 years, and with MoCA scores at 2 years as dependent variables, using backwards linear regression analysis. Additionally, we constructed a prediction model for diagnosis of cognitive impairment using logistic regression analysis. FINDINGS:390 patients with Parkinson's disease recruited between July 1, 2010, and May 31, 2013, and for whom data on MoCA scores at baseline and 2 years were available. In multivariate analyses, baseline age, University of Pennsylvania Smell Inventory Test (UPSIT) scores, CSF amyloid - (Aβ) to t-tau ratio, and APOE status were associated with change in MoCA scores over time. Baseline age, MoCA and UPSIT scores, and CSF Aβ to t-tau ratio were associated with MoCA score at 2 years (using a backwards p-removal threshold of 0·1). Accuracy of prediction of cognitive impairment using age alone (area under the curve 0·68, 95% CI 0·60-0·76) significantly improved by addition of clinical scores (UPSIT, Rapid Eye Movement Sleep Behaviour Disorder Screening Questionnaire [RBDSQ], Geriatric Depression Scale, and Movement Disorder Society Unified Parkinson's Disease Rating Scale motor scores; 0·76, 0·68-0·83), CSF variables (0·74, 0·68-0·81), or DAT imaging results (0·76, 0·68-0·83). In combination, the five variables showing the most significant associations with cognitive impairment (age, UPSIT, RBDSQ, CSF Aβ, and caudate uptake on DAT imaging) allowed prediction of cognitive impairment at 2 years (0·80, 0·74-0·87; p=0·0003 compared to age alone). INTERPRETATION:In newly diagnosed Parkinson's disease, the occurrence of cognitive impairment at 2 year follow-up can be predicted with good accuracy using a model combining information on age, non-motor assessments, DAT imaging, and CSF biomarkers. FUNDING:None.
10.1016/S1474-4422(16)30328-3
Use of Machine Learning Models to Predict Death After Acute Myocardial Infarction.
Khera Rohan,Haimovich Julian,Hurley Nathan C,McNamara Robert,Spertus John A,Desai Nihar,Rumsfeld John S,Masoudi Frederick A,Huang Chenxi,Normand Sharon-Lise,Mortazavi Bobak J,Krumholz Harlan M
JAMA cardiology
Importance:Accurate prediction of adverse outcomes after acute myocardial infarction (AMI) can guide the triage of care services and shared decision-making, and novel methods hold promise for using existing data to generate additional insights. Objective:To evaluate whether contemporary machine learning methods can facilitate risk prediction by including a larger number of variables and identifying complex relationships between predictors and outcomes. Design, Setting, and Participants:This cohort study used the American College of Cardiology Chest Pain-MI Registry to identify all AMI hospitalizations between January 1, 2011, and December 31, 2016. Data analysis was performed from February 1, 2018, to October 22, 2020. Main Outcomes and Measures:Three machine learning models were developed and validated to predict in-hospital mortality based on patient comorbidities, medical history, presentation characteristics, and initial laboratory values. Models were developed based on extreme gradient descent boosting (XGBoost, an interpretable model), a neural network, and a meta-classifier model. Their accuracy was compared against the current standard developed using a logistic regression model in a validation sample. Results:A total of 755 402 patients (mean [SD] age, 65 [13] years; 495 202 [65.5%] male) were identified during the study period. In independent validation, 2 machine learning models, gradient descent boosting and meta-classifier (combination including inputs from gradient descent boosting and a neural network), marginally improved discrimination compared with logistic regression (C statistic, 0.90 for best performing machine learning model vs 0.89 for logistic regression). Nearly perfect calibration in independent validation data was found in the XGBoost (slope of predicted to observed events, 1.01; 95% CI, 0.99-1.04) and the meta-classifier model (slope of predicted-to-observed events, 1.01; 95% CI, 0.99-1.02), with more precise classification across the risk spectrum. The XGBoost model reclassified 32 393 of 121 839 individuals (27%) and the meta-classifier model reclassified 30 836 of 121 839 individuals (25%) deemed at moderate to high risk for death in logistic regression as low risk, which were more consistent with the observed event rates. Conclusions and Relevance:In this cohort study using a large national registry, none of the tested machine learning models were associated with substantive improvement in the discrimination of in-hospital mortality after AMI, limiting their clinical utility. However, compared with logistic regression, XGBoost and meta-classifier models, but not the neural network, offered improved resolution of risk for high-risk individuals.
10.1001/jamacardio.2021.0122
Predicting Oncologic Outcomes in Renal Cell Carcinoma After Surgery.
Leibovich Bradley C,Lohse Christine M,Cheville John C,Zaid Harras B,Boorjian Stephen A,Frank Igor,Thompson R Houston,Parker William P
European urology
BACKGROUND:Predicting oncologic outcomes is important for patient counseling, clinical trial design, and biomarker study testing. OBJECTIVE:To develop prognostic models for progression-free (PFS) and cancer-specific survival (CSS) in patients with clear cell renal cell carcinoma (ccRCC), papillary RCC (papRCC), and chromophobe RCC (chrRCC). DESIGN, SETTING, AND PARTICIPANTS:Retrospective cohort review of the Mayo Clinic Nephrectomy registry from 1980 to 2010, for patients with nonmetastatic ccRCC, papRCC, and chrRCC. INTERVENTION:Partial or radical nephrectomy. OUTCOME MEASUREMENTS AND STATISTICAL ANALYSIS:PFS and CSS from date of surgery. Multivariable Cox proportional hazards regression was used to develop parsimonious models based on clinicopathologic features to predict oncologic outcomes and were evaluated with c-indexes. Models were converted into risk scores/groupings and used to predict PFS and CSS rates after accounting for competing risks. RESULTS AND LIMITATIONS:A total of 3633 patients were identified, of whom 2726 (75%) had ccRCC, 607 (17%) had papRCC, and 222 (6%) had chrRCC. Models were generated for each histologic subtype and a risk score/grouping was developed for each subtype and outcome (PFS/CSS). For PFS, the c-indexes were 0.83, 0.77, and 0.78 for ccRCC, papRCC, and chrRCC, respectively. For CSS, c-indexes were 0.86 and 0.83 for ccRCC and papRCC. Due to only 22 deaths from RCC, we did not assess a multivariable model for chrRCC. Limitations include the single institution study, lack of external validation, and its retrospective nature. CONCLUSIONS:Using a large institutional experience, we generated specific prognostic models for oncologic outcomes in ccRCC, papRCC, and chrRCC that rely on features previously shown-and validated-to be associated with survival. These updated models should inform patient prognosis, biomarker design, and clinical trial enrollment. PATIENT SUMMARY:We identified routinely available clinical and pathologic features that can accurately predict progression and death from renal cell carcinoma following surgery. These updated models should inform patient prognosis, biomarker design, and clinical trial enrollment.
10.1016/j.eururo.2018.01.005
Interrogating open issues in cancer precision medicine with patient-derived xenografts.
Nature reviews. Cancer
Patient-derived xenografts (PDXs) have emerged as an important platform to elucidate new treatments and biomarkers in oncology. PDX models are used to address clinically relevant questions, including the contribution of tumour heterogeneity to therapeutic responsiveness, the patterns of cancer evolutionary dynamics during tumour progression and under drug pressure, and the mechanisms of resistance to treatment. The ability of PDX models to predict clinical outcomes is being improved through mouse humanization strategies and the implementation of co-clinical trials, within which patients and PDXs reciprocally inform therapeutic decisions. This Opinion article discusses aspects of PDX modelling that are relevant to these questions and highlights the merits of shared PDX resources to advance cancer medicine from the perspective of EurOPDX, an international initiative devoted to PDX-based research.
10.1038/nrc.2016.140
Moving From Static to Dynamic Models of the Onset of Mental Disorder: A Review.
Nelson Barnaby,McGorry Patrick D,Wichers Marieke,Wigman Johanna T W,Hartmann Jessica A
JAMA psychiatry
Importance:In recent years, there has been increased focus on subthreshold stages of mental disorders, with attempts to model and predict which individuals will progress to full-threshold disorder. Given this research attention and the clinical significance of the issue, this article analyzes the assumptions of the theoretical models in the field. Observations:Psychiatric research into predicting the onset of mental disorder has shown an overreliance on one-off sampling of cross-sectional data (ie, a snapshot of clinical state and other risk markers) and may benefit from taking dynamic changes into account in predictive modeling. Cross-disciplinary approaches to complex system structures and changes, such as dynamical systems theory, network theory, instability mechanisms, chaos theory, and catastrophe theory, offer potent models that can be applied to the emergence (or decline) of psychopathology, including psychosis prediction, as well as to transdiagnostic emergence of symptoms. Conclusions and Relevance:Psychiatric research may benefit from approaching psychopathology as a system rather than as a category, identifying dynamics of system change (eg, abrupt vs gradual psychosis onset), and determining the factors to which these systems are most sensitive (eg, interpersonal dynamics and neurochemical change) and the individual variability in system architecture and change. These goals can be advanced by testing hypotheses that emerge from cross-disciplinary models of complex systems. Future studies require repeated longitudinal assessment of relevant variables through either (or a combination of) micro-level (momentary and day-to-day) and macro-level (month and year) assessments. Ecological momentary assessment is a data collection technique appropriate for micro-level assessment. Relevant statistical approaches are joint modeling and time series analysis, including metric-based and model-based methods that draw on the mathematical principles of dynamical systems. This next generation of prediction studies may more accurately model the dynamic nature of psychopathology and system change as well as have treatment implications, such as introducing a means of identifying critical periods of risk for mental state deterioration.
10.1001/jamapsychiatry.2017.0001
Impact of HPV vaccine hesitancy on cervical cancer in Japan: a modelling study.
Simms Kate T,Hanley Sharon J B,Smith Megan A,Keane Adam,Canfell Karen
The Lancet. Public health
BACKGROUND:Funding for human papillomavirus (HPV) vaccination in Japan began in 2010 for girls aged 12-16 years, with three-dose coverage initially reaching more than 70%. On June 14, 2013, 2 months after formal inclusion in Japan's national immunisation programme, proactive recommendations for the HPV vaccine were suspended following reports of adverse events since found to be unrelated to vaccination, but which were extensively covered in the media. Vaccine coverage subsequently dropped to less than 1% and has remained this low to date. We aimed to quantify the impact of this vaccine hesitancy crisis, and the potential health gains if coverage can be restored. METHODS:In this modelling study, we used the Policy1-Cervix modelling platform. We adapted the model for Japan with use of data on HPV prevalence, screening practices and coverage, and cervical cancer incidence and mortality. We evaluated the expected number of cervical cancer cases and deaths over the lifetime of cohorts born from 1994 to 2007 in the context of the vaccine hesitancy crisis. We assessed a range of recovery scenarios from 2020 onwards, including a scenario in which routine coverage is restored to 70%, with 50% catch-up coverage for the missed cohorts (aged 13-20 years in 2020). To estimate the impact of the vaccine crisis to date, we also modelled a counterfactual scenario in which 70% coverage had been maintained in 12-year-olds from 2013 onwards. FINDINGS:The vaccine crisis from 2013 to 2019 is predicted to result in an additional 24 600-27 300 cases and 5000-5700 deaths over the lifetime of cohorts born between 1994 and 2007, compared with if coverage had remained at around 70% since 2013. However, restoration of coverage in 2020, including catch-up vaccination for missed cohorts, could prevent 14 800-16 200 of these cases and 3000-3400 of these deaths. If coverage is not restored in 2020, an additional 3400-3800 cases and 700-800 deaths will occur over the lifetime of individuals who are 12 years old in 2020 alone. If the crisis continues, 9300-10 800 preventable deaths due to cervical cancer will occur in the next 50 years (2020-69). INTERPRETATION:The HPV vaccine crisis to date is estimated to result in around 5000 deaths from cervical cancer in Japan. Many of these deaths could still be prevented if vaccination coverage with extended catch-up can be rapidly restored. FUNDING:National Health and Medical Research Council Australia Centre of Research Excellence in Cervical Cancer Control, Japan Society for the Promotion of Science.
10.1016/S2468-2667(20)30010-4
Machine learning for real-time prediction of complications in critical care: a retrospective study.
Meyer Alexander,Zverinski Dina,Pfahringer Boris,Kempfert Jörg,Kuehne Titus,Sündermann Simon H,Stamm Christof,Hofmann Thomas,Falk Volkmar,Eickhoff Carsten
The Lancet. Respiratory medicine
BACKGROUND:The large amount of clinical signals in intensive care units can easily overwhelm health-care personnel and can lead to treatment delays, suboptimal care, or clinical errors. The aim of this study was to apply deep machine learning methods to predict severe complications during critical care in real time after cardiothoracic surgery. METHODS:We used deep learning methods (recurrent neural networks) to predict several severe complications (mortality, renal failure with a need for renal replacement therapy, and postoperative bleeding leading to operative revision) in post cardiosurgical care in real time. Adult patients who underwent major open heart surgery from Jan 1, 2000, to Dec 31, 2016, in a German tertiary care centre for cardiovascular diseases formed the main derivation dataset. We measured the accuracy and timeliness of the deep learning model's forecasts and compared predictive quality to that of established standard-of-care clinical reference tools (clinical rule for postoperative bleeding, Simplified Acute Physiology Score II for mortality, and the Kidney Disease: Improving Global Outcomes staging criteria for acute renal failure) using positive predictive value (PPV), negative predictive value, sensitivity, specificity, area under the curve (AUC), and the F measure (which computes a harmonic mean of sensitivity and PPV). Results were externally retrospectively validated with 5898 cases from the published MIMIC-III dataset. FINDINGS:Of 47 559 intensive care admissions (corresponding to 42 007 patients), we included 11 492 (corresponding to 9269 patients). The deep learning models yielded accurate predictions with the following PPV and sensitivity scores: PPV 0·90 and sensitivity 0·85 for mortality, 0·87 and 0·94 for renal failure, and 0·84 and 0·74 for bleeding. The predictions significantly outperformed the standard clinical reference tools, improving the absolute complication prediction AUC by 0·29 (95% CI 0·23-0·35) for bleeding, by 0·24 (0·19-0·29) for mortality, and by 0·24 (0·13-0·35) for renal failure (p<0·0001 for all three analyses). The deep learning methods showed accurate predictions immediately after patient admission to the intensive care unit. We also observed an increase in performance in our validation cohort when the machine learning approach was tested against clinical reference tools, with absolute improvements in AUC of 0·09 (95% CI 0·03-0·15; p=0·0026) for bleeding, of 0·18 (0·07-0·29; p=0·0013) for mortality, and of 0·25 (0·18-0·32; p<0·0001) for renal failure. INTERPRETATION:The observed improvements in prediction for all three investigated clinical outcomes have the potential to improve critical care. These findings are noteworthy in that they use routinely collected clinical data exclusively, without the need for any manual processing. The deep machine learning method showed AUC scores that significantly surpass those of clinical reference tools, especially soon after admission. Taken together, these properties are encouraging for prospective deployment in critical care settings to direct the staff's attention towards patients who are most at risk. FUNDING:No specific funding.
10.1016/S2213-2600(18)30300-X
Prediction of breast cancer-related outcomes with the Edmonton Symptom Assessment Scale: A literature review.
Milton Lauren,Behroozian Tara,Coburn Natalie,Trudeau Maureen,Razvi Yasmeen,McKenzie Erin,Karam Irene,Lam Henry,Chow Edward
Supportive care in cancer : official journal of the Multinational Association of Supportive Care in Cancer
PURPOSE:The Edmonton Symptom Assessment Scale (ESAS) is a validated tool used in patients with varied cancer diagnoses to measure patient symptoms. The present manuscript will review the literature assessing the ability of the ESAS to predict patient-related outcomes in breast cancer patients. METHODS:A literature search was conducted of Cochrane Central Register of Controlled Trials databases, Ovid MEDLINE, and Embase for English articles that investigated the use of predictive modelling with the ESAS in the breast cancer population. Study type, publication year, sample size, patient demographics, predicted outcomes, and strongest predictive factors/symptoms were summarized for each study. RESULTS:A total of nine articles were included in this review. Five articles used the ESAS in predictive models to determine patient time to death. ESAS was also used to predict emergency department visits, determine symptoms associated with decreased quality of life, and generate a Health Utility Score. Lack of appetite was the most common ESAS symptom, as it was reported in five studies to be associated with decreased survival. In four of the nine articles, an additional survey investigating physical functioning was used in combination with ESAS to strengthen the predictive models. CONCLUSIONS:Included studies support the use of ESAS in predictive models, particularly for predicting survival. Using the ESAS as a predictive tool allows for more accurate time to death predictions, potentially improving symptom management and preventing overtreatment of palliative patients near the end of life.
10.1007/s00520-020-05755-9
Early Prediction of Disease Progression in Small Cell Lung Cancer: Toward Model-Based Personalized Medicine in Oncology.
Buil-Bruna Núria,Sahota Tarjinder,López-Picazo José-María,Moreno-Jiménez Marta,Martín-Algarra Salvador,Ribba Benjamin,Trocóniz Iñaki F
Cancer research
Predictive biomarkers can play a key role in individualized disease monitoring. Unfortunately, the use of biomarkers in clinical settings has thus far been limited. We have previously shown that mechanism-based pharmacokinetic/pharmacodynamic modeling enables integration of nonvalidated biomarker data to provide predictive model-based biomarkers for response classification. The biomarker model we developed incorporates an underlying latent variable (disease) representing (unobserved) tumor size dynamics, which is assumed to drive biomarker production and to be influenced by exposure to treatment. Here, we show that by integrating CT scan data, the population model can be expanded to include patient outcome. Moreover, we show that in conjunction with routine medical monitoring data, the population model can support accurate individual predictions of outcome. Our combined model predicts that a change in disease of 29.2% (relative standard error 20%) between two consecutive CT scans (i.e., 6-8 weeks) gives a probability of disease progression of 50%. We apply this framework to an external dataset containing biomarker data from 22 small cell lung cancer patients (four patients progressing during follow-up). Using only data up until the end of treatment (a total of 137 lactate dehydrogenase and 77 neuron-specific enolase observations), the statistical framework prospectively identified 75% of the individuals as having a predictable outcome in follow-up visits. This included two of the four patients who eventually progressed. In all identified individuals, the model-predicted outcomes matched the observed outcomes. This framework allows at risk patients to be identified early and therapeutic intervention/monitoring to be adjusted individually, which may improve overall patient survival.
10.1158/0008-5472.CAN-14-2584