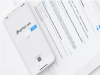
An EMT-related gene signature for the prognosis of human bladder cancer.
Cao Rui,Yuan Lushun,Ma Bo,Wang Gang,Qiu Wei,Tian Ye
Journal of cellular and molecular medicine
The transition from non-muscle-invasive bladder cancer (NMIBC) to muscle-invasive bladder cancer (MIBC) is detrimental to bladder cancer (BLCA) patients. Here, we aimed to study the underlying mechanism of the subtype transition. Gene set variation analysis (GSVA) revealed the epithelial-mesenchymal transition (EMT) signalling pathway with the most positive correlation in this transition. Then, we built a LASSO Cox regression model of an EMT-related gene signature in BLCA. The patients with high risk scores had significantly worse overall survival (OS) and disease-free survival (DFS) than those with low risk scores. The EMT-related gene signature also performed favourably in the accuracy of prognosis and in the subtype survival analysis. Univariate and multivariate Cox regression analyses demonstrated that the EMT-related gene signature, pathological N stage and age were independent prognostic factors for predicting survival in BLCA patients. Furthermore, the predictive nomogram model was able to effectively predict the outcome of BLCA patients by appropriately stratifying the risk score. In conclusion, we developed a novel EMT-related gene signature that has tumour-promoting effects, acts as a negative independent prognostic factor and might facilitate personalized counselling and treatment in BLCA.
10.1111/jcmm.14767
Periodontal regeneration versus extraction and dental implant or prosthetic replacement of teeth severely compromised by attachment loss to the apex: A randomized controlled clinical trial reporting 10-year outcomes, survival analysis and mean cumulative cost of recurrence.
Cortellini Pierpaolo,Stalpers Gabrielle,Mollo Aniello,Tonetti Maurizio S
Journal of clinical periodontology
BACKGROUND:Periodontal regeneration can change tooth prognosis and represents an alternative to extraction in teeth compromised by severe intra-bony defects. The aim of this study was to compare periodontal regeneration (PR) with tooth extraction and replacement (TER) in a population with attachment loss to or beyond the apex of the root in terms of professional, patient-reported and economic outcomes. METHODS:This was a 10-year randomized controlled clinical trial. 50 stage III or stage IV periodontitis subjects with a severely compromised tooth with attachment loss to or beyond the apex were randomized to PR or TER with either an implant- or a tooth-supported fixed partial denture. Subjects were kept on a strict periodontal supportive care regimen every 3 months and examined yearly. Survival and recurrence analysis were performed. RESULTS:88% and 100% survival rates were observed in the PR and TER groups. Complication-free survival was not significantly different: 6.7-9.1 years for PR and 7.3-9.1 years for TER (p = .788). In PR, the observed 10-year attachment gain was 7.3 ± 2.3 mm and the residual probing depths were 3.4 ± 0.8 mm. Recurrence analysis showed that the 95% confidence interval of the costs was significantly lower for PR compared with TER throughout the whole 10-year period. Patient-reported outcomes and oral health-related quality-of-life measurements improved in both groups. CONCLUSIONS:Periodontal regeneration can change the prognosis of hopeless teeth and is a less costly alternative to tooth extraction and replacement. The complexity of the treatment limits widespread application to the most complex cases but provides powerful proof of principle for the benefits of PR in deep intra-bony defect.
10.1111/jcpe.13289
Estimating restricted mean survival time and expected life-years lost in the presence of competing risks within flexible parametric survival models.
Mozumder Sarwar I,Rutherford Mark J,Lambert Paul C
BMC medical research methodology
BACKGROUND:Royston-Parmar flexible parametric survival models (FPMs) can be fitted on either the cause-specific hazards or cumulative incidence scale in the presence of competing risks. An advantage of modelling within this framework for competing risks data is the ease at which alternative predictions to the (cause-specific or subdistribution) hazard ratio can be obtained. Restricted mean survival time (RMST), or restricted mean failure time (RMFT) on the mortality scale, is one such measure. This has an attractive interpretation, especially when the proportionality assumption is violated. Compared to similar measures, fewer assumptions are required and it does not require extrapolation. Furthermore, one can easily obtain the expected number of life-years lost, or gained, due to a particular cause of death, which is a further useful prognostic measure as introduced by Andersen. METHODS:In the presence of competing risks, prediction of RMFT and the expected life-years lost due to a cause of death are presented using Royston-Parmar FPMs. These can be predicted for a specific covariate pattern to facilitate interpretation in observational studies at the individual level, or at the population-level using standardisation to obtain marginal measures. Predictions are illustrated using English colorectal data and are obtained using the Stata post-estimation command, standsurv. RESULTS:Reporting such measures facilitate interpretation of a competing risks analysis, particularly when the proportional hazards assumption is not appropriate. Standardisation provides a useful way to obtain marginal estimates to make absolute comparisons between two covariate groups. Predictions can be made at various time-points and presented visually for each cause of death to better understand the overall impact of different covariate groups. CONCLUSIONS:We describe estimation of RMFT, and expected life-years lost partitioned by each competing cause of death after fitting a single FPM on either the log-cumulative subdistribution, or cause-specific hazards scale. These can be used to facilitate interpretation of a competing risks analysis when the proportionality assumption is in doubt.
10.1186/s12874-021-01213-0
Survival analytical techniques were used to assess agreement of a quantitative variable.
Llorca Javier,Delgado-Rodríguez Miguel
Journal of clinical epidemiology
BACKGROUND AND OBJECTIVE:Survival-agreement plots have been suggested as a new graphical approach to assess agreement in quantitative variables. We propose that survival analytical techniques can complement this method, providing a new analytical insight for agreement. METHODS:Two survival-agreement plots are used to detect the bias between to measurements of the same variable. The presence of bias is tested with log-rank test, and its magnitude with Cox regression. RESULTS:An example on C-reactive protein determinations shows how survival analytical methods would be interpreted in the context of assessing agreement. CONCLUSION:Log-rank test, Cox regression, or other analytical methods could be used to assess agreement in quantitative variables; correct interpretations require good clinical sense.
10.1016/j.jclinepi.2004.10.011
The Extrapolation Performance of Survival Models for Data With a Cure Fraction: A Simulation Study.
Kearns Benjamin,Stevenson Matt D,Triantafyllopoulos Kostas,Manca Andrea
Value in health : the journal of the International Society for Pharmacoeconomics and Outcomes Research
OBJECTIVES:Curative treatments can result in complex hazard functions. The use of standard survival models may result in poor extrapolations. Several models for data which may have a cure fraction are available, but comparisons of their extrapolation performance are lacking. A simulation study was performed to assess the performance of models with and without a cure fraction when fit to data with a cure fraction. METHODS:Data were simulated from a Weibull cure model, with 9 scenarios corresponding to different lengths of follow-up and sample sizes. Cure and noncure versions of standard parametric, Royston-Parmar, and dynamic survival models were considered along with noncure fractional polynomial and generalized additive models. The mean-squared error and bias in estimates of the hazard function were estimated. RESULTS:With the shortest follow-up, none of the cure models provided good extrapolations. Performance improved with increasing follow-up, except for the misspecified standard parametric cure model (lognormal). The performance of the flexible cure models was similar to that of the correctly specified cure model. Accurate estimates of the cured fraction were not necessary for accurate hazard estimates. Models without a cure fraction provided markedly worse extrapolations. CONCLUSIONS:For curative treatments, failure to model the cured fraction can lead to very poor extrapolations. Cure models provide improved extrapolations, but with immature data there may be insufficient evidence to choose between cure and noncure models, emphasizing the importance of clinical knowledge for model choice. Dynamic cure fraction models were robust to model misspecification, but standard parametric cure models were not.
10.1016/j.jval.2021.05.009
Methods for Improving the Variance Estimator of the Kaplan-Meier Survival Function, When There Is No, Moderate and Heavy Censoring-Applied in Oncological Datasets.
Frontiers in public health
In case of heavy and even moderate censoring, a common problem with the Greenwood and Peto variance estimators of the Kaplan-Meier survival function is that they can underestimate the true variance in the left and right tails of the survival distribution. Here, we introduce a variance estimator for the Kaplan-Meier survival function by assigning weight greater than zero to the censored observation. On the basis of this weight, a modification of the Kaplan-Meier survival function and its variance is proposed. An advantage of this approach is that it gives non-parametric estimates at each point whether the event occurred or not. The performance of the variance of this new method is compared with the Greenwood, Peto, regular, and adjusted hybrid variance estimators. Several combinations of these methods with the new method are examined and compared on three datasets, such as leukemia clinical trial data, thalassaemia data as well as cancer data. Thalassaemia is an inherited blood disease, very common in Pakistan, where our data are derived from.
10.3389/fpubh.2022.793648
Long-term survival in renal transplant recipients with graft function.
Ojo A O,Hanson J A,Wolfe R A,Leichtman A B,Agodoa L Y,Port F K
Kidney international
UNLABELLED:Long-term survival in renal transplant recipients with graft function. BACKGROUND:Death with graft function (DWGF) is a common cause of graft loss. The risks and determinants of DWGF have not been studied in a recent cohort of renal transplant recipients. We performed a population-based survival analysis of U.S. patients with end-stage renal disease (ESRD) transplanted between 1988 and 1997. METHODS:Registry data were used to evaluate long-term patient survival and cause-specific risks of DWGF in 86,502 adult (>/=18 years) renal transplant recipients. RESULTS:Out of 18,482 deaths, 38% (N = 7040) were deaths with graft function. This accounts for 42. 5% of all graft loss. Patient survival with graft function was 97, 91, and 86% at 1, 5, and 10 years, respectively. The risk of DWGF decreased by 67% (RR = 0.33, P < 0.001) between 1988 and 1997. The adjusted rate of DWGF was 4.6, 0.8, 2.2, and 1.4 deaths per 1000 person-years for cardiovascular disease, stroke, infections, and malignancy, respectively. The suicide rate was 15.7 versus 9.0 deaths per 100,000 person-years in the general population (P < 0. 001). In multivariate analysis, the following factors were independently and significantly predictive of DWGF: white recipient, age at transplantation, ESRD caused by hypertension or diabetes mellitus, length of pretransplant dialysis, delayed graft function, acute rejection, panel reactive antibody> 30%, African American donor race, age> 45 years, and donor death caused by cerebrovascular disease. CONCLUSIONS:Patients with graft function have a high long-term survival. Although DWGF is a major cause of graft loss, the risk has declined substantially since 1990. Cardiovascular disease was the predominant reported cause of DWGF. Other causes vary by post-transplant time period. Attention to atherosclerotic risk factors may be the most important challenge to further improve the longevity of patients with successful renal transplants.
10.1046/j.1523-1755.2000.00816.x
Survival estimation through the cumulative hazard with monotone natural cubic splines using convex optimization-the HCNS approach.
Computer methods and programs in biomedicine
BACKGROUND AND OBJECTIVES:In survival analysis both the Kaplan-Meier estimate and the Cox model enjoy a broad acceptance. We present an improved spline-based survival estimate and offer a fully automated software for its implementation. We explore the use of natural cubic splines that are constrained to be monotone. Apart from its superiority over the Kaplan Meier estimator our approach overcomes limitations of other known smoothing approaches and can accommodate covariates. Unlike other spline methods, concerns of computational problems and issues of overfitting are resolved since no attempt is made to maximize a likelihood once the Kaplan-Meier estimator is obtained. An application to laryngeal cancer data, a simulation study and illustrations of the broad application of the method and its software are provided. In addition to presenting our approaches, this work contributes to bridging a communication gap between clinicians and statisticians that is often apparent in the medical literature. METHODS:We employ a two-stage approach: first obtain the stepwise cumulative hazard and then consider a natural cubic spline to smooth its steps under restrictions of monotonicity between any consecutive knots. The underlying region of monotonicity corresponds to a non-linear region that encompasses the full family of monotone third-degree polynomials. We approximate it linearly and reduce the problem to a restricted least squares one under linear restrictions. This ensures convexity. We evaluate our method through simulations against competitive traditional approaches. RESULTS:Our method is compared to the popular Kaplan Meier estimate both in terms of mean squared error and in terms of coverage. Over-fitting is avoided by construction, as our spline attempts to approximate the empirical estimate of the cumulative hazard itself, and is not fitted directly on the data. CONCLUSIONS:The proposed approach will enable clinical researchers to obtain improved survival estimates and valid confidence intervals over the full spectrum of the range of the survival data. Our methods outperform conventional approaches and can be readily utilized in settings beyond survival analysis such as diagnostic testing.
10.1016/j.cmpb.2020.105357
Comparative study of joint analysis of microarray gene expression data in survival prediction and risk assessment of breast cancer patients.
Yasrebi Haleh
Briefings in bioinformatics
Microarray gene expression data sets are jointly analyzed to increase statistical power. They could either be merged together or analyzed by meta-analysis. For a given ensemble of data sets, it cannot be foreseen which of these paradigms, merging or meta-analysis, works better. In this article, three joint analysis methods, Z-score normalization, ComBat and the inverse normal method (meta-analysis) were selected for survival prognosis and risk assessment of breast cancer patients. The methods were applied to eight microarray gene expression data sets, totaling 1324 patients with two clinical endpoints, overall survival and relapse-free survival. The performance derived from the joint analysis methods was evaluated using Cox regression for survival analysis and independent validation used as bias estimation. Overall, Z-score normalization had a better performance than ComBat and meta-analysis. Higher Area Under the Receiver Operating Characteristic curve and hazard ratio were also obtained when independent validation was used as bias estimation. With a lower time and memory complexity, Z-score normalization is a simple method for joint analysis of microarray gene expression data sets. The derived findings suggest further assessment of this method in future survival prediction and cancer classification applications.
10.1093/bib/bbv092
Issues possibly associated with misinterpreting survival data: A method study.
Peinemann Frank,Labeit Alexander Michael
Journal of evidence-based medicine
OBJECTIVE:Proper interpretation of survival data of clinical cancer studies may be difficult and pitfalls related to the nature of Kaplan-Meier analyses might end up in mistaken inferences. The purpose of the present work is to raise awareness of those pitfalls and to prevent errors in future studies. STUDY DESIGN AND SETTING:While evaluating a randomized controlled trial, we came across some issues possibly associated with misinterpreting survival data. We thoroughly reviewed the reporting of survival analyses, statistical approaches, baseline characteristics, and choice of primary end point. The reported data were derived from people with high-risk neuroblastoma. Thus, the trial focused on survival. We reenacted survival functions by deducing the data of various treatment groups from pictured survival functions to estimate the concerning hazard ratios. RESULTS:Opposed to the reporting of the trial, we did not identify a significant difference between treatment groups with respect to overall survival. We were not able to appreciate an effective crossing of survival curves. With respect to event-free survival, we focused on comparable treatment groups and we did not identify a significant difference between treatment groups, thereby again opposing the reporting of the trial. CONCLUSIONS:The present work exemplifies statistical issues that were apparently difficult to detect and that are possibly associated with misinterpreting survival functions. These issues include assumed crossing of survival curves, statistical approach changed in follow-up, different pretreatment between groups, and event-free survival used as primary outcome. Careful handling might prevent similar potential misinterpretation in future studies.
10.1111/jebm.12301
A new semi-supervised learning model combined with Cox and SP-AFT models in cancer survival analysis.
Scientific reports
Gene selection is an attractive and important task in cancer survival analysis. Most existing supervised learning methods can only use the labeled biological data, while the censored data (weakly labeled data) far more than the labeled data are ignored in model building. Trying to utilize such information in the censored data, a semi-supervised learning framework (Cox-AFT model) combined with Cox proportional hazard (Cox) and accelerated failure time (AFT) model was used in cancer research, which has better performance than the single Cox or AFT model. This method, however, is easily affected by noise. To alleviate this problem, in this paper we combine the Cox-AFT model with self-paced learning (SPL) method to more effectively employ the information in the censored data in a self-learning way. SPL is a kind of reliable and stable learning mechanism, which is recently proposed for simulating the human learning process to help the AFT model automatically identify and include samples of high confidence into training, minimizing interference from high noise. Utilizing the SPL method produces two direct advantages: (1) The utilization of censored data is further promoted; (2) the noise delivered to the model is greatly decreased. The experimental results demonstrate the effectiveness of the proposed model compared to the traditional Cox-AFT model.
10.1038/s41598-017-13133-5
Testing for Differences in Survival When Treatment Effects Are Persistent, Decaying, or Delayed.
Journal of clinical oncology : official journal of the American Society of Clinical Oncology
A statistical test for the presence of treatment effects on survival will be based on a null hypothesis (absence of effects) and an alternative (presence of effects). The null is very simply expressed. The most common alternative, also simply expressed, is that of proportional hazards. For this situation, not only do we have a very powerful test in the log-rank test but also the outcome is readily interpreted. However, many modern treatments fall outside this relatively straightforward paradigm and, as such, have attracted attention from statisticians eager to do their best to avoid losing power as well as to maintain interpretability when the alternative hypothesis is less simple. Examples include trials where the treatment effect decays with time, immunotherapy trials where treatment effects may be slow to manifest themselves as well as the so-called crossing hazards problem. We review some of the solutions that have been proposed to deal with these issues. We pay particular attention to the integrated log-rank test and how it can be combined with the log-rank test itself to obtain powerful tests for these more complex situations.
10.1200/JCO.21.01811
A Novel Attention-Mechanism Based Cox Survival Model by Exploiting Pan-Cancer Empirical Genomic Information.
Cells
Cancer prognosis is an essential goal for early diagnosis, biomarker selection, and medical therapy. In the past decade, deep learning has successfully solved a variety of biomedical problems. However, due to the high dimensional limitation of human cancer transcriptome data and the small number of training samples, there is still no mature deep learning-based survival analysis model that can completely solve problems in the training process like overfitting and accurate prognosis. Given these problems, we introduced a novel framework called SAVAE-Cox for survival analysis of high-dimensional transcriptome data. This model adopts a novel attention mechanism and takes full advantage of the adversarial transfer learning strategy. We trained the model on 16 types of TCGA cancer RNA-seq data sets. Experiments show that our module outperformed state-of-the-art survival analysis models such as the Cox proportional hazard model (Cox-ph), Cox-lasso, Cox-ridge, Cox-nnet, and VAECox on the concordance index. In addition, we carry out some feature analysis experiments. Based on the experimental results, we concluded that our model is helpful for revealing cancer-related genes and biological functions.
10.3390/cells11091421
Adjusted Survival Curves Improve Understanding of Multivariable Cox Model Results.
The Journal of arthroplasty
Kaplan-Meier survival curves are the most common methods for unadjusted group comparison of outcomes in orthopedic research. However, they may be misleading due to an imbalance of confounders between patient groups. The Cox model is frequently used to adjust for confounders, but graphical display of adjusted survival curves is not commonly utilized. We describe the circumstances when adjusted survival curves are useful in orthopedic research, describe and use 2 different methods to obtain adjusted curves, and illustrate how they can improve understanding of the multivariable Cox model results. We further provide practical strategies for identifying the need for and performing adjusted survival curves. Please visit the followinghttps://youtu.be/ys0hy2CiMCAfor a video that explains the highlights of the paper in practical terms.
10.1016/j.arth.2021.06.002
Predicting cardiovascular risk from national administrative databases using a combined survival analysis and deep learning approach.
International journal of epidemiology
BACKGROUND:Machine learning-based risk prediction models may outperform traditional statistical models in large datasets with many variables, by identifying both novel predictors and the complex interactions between them. This study compared deep learning extensions of survival analysis models with Cox proportional hazards models for predicting cardiovascular disease (CVD) risk in national health administrative datasets. METHODS:Using individual person linkage of administrative datasets, we constructed a cohort of all New Zealanders aged 30-74 who interacted with public health services during 2012. After excluding people with prior CVD, we developed sex-specific deep learning and Cox proportional hazards models to estimate the risk of CVD events within 5 years. Models were compared based on the proportion of explained variance, model calibration and discrimination, and hazard ratios for predictor variables. RESULTS:First CVD events occurred in 61 927 of 2 164 872 people. Within the reference group, the largest hazard ratios estimated by the deep learning models were for tobacco use in women (2.04, 95% CI: 1.99, 2.10) and chronic obstructive pulmonary disease with acute lower respiratory infection in men (1.56, 95% CI: 1.50, 1.62). Other identified predictors (e.g. hypertension, chest pain, diabetes) aligned with current knowledge about CVD risk factors. Deep learning outperformed Cox proportional hazards models on the basis of proportion of explained variance (R2: 0.468 vs 0.425 in women and 0.383 vs 0.348 in men), calibration and discrimination (all P <0.0001). CONCLUSIONS:Deep learning extensions of survival analysis models can be applied to large health administrative datasets to derive interpretable CVD risk prediction equations that are more accurate than traditional Cox proportional hazards models.
10.1093/ije/dyab258
Survival analysis: time-dependent effects and time-varying risk factors.
Dekker Friedo W,de Mutsert Renée,van Dijk Paul C,Zoccali Carmine,Jager Kitty J
Kidney international
In traditional Kaplan-Meier or Cox regression analysis, usually a risk factor measured at baseline is related to mortality thereafter. During follow-up, however, things may change: either the effect of a fixed baseline risk factor may vary over time, resulting in a weakening or strengthening of associations over time, or the risk factor itself may vary over time. In this paper, short-term versus long-term effects (so-called time-dependent effects) of a fixed baseline risk factor are addressed. An example is presented showing that underweight is a strong risk factor for mortality in dialysis patients, especially in the short run. In contrast, overweight is a risk factor for mortality, which is stronger in the long run than in the short run. In addition, the analysis of how time-varying risk factors (so-called time-dependent risk factors) are related to mortality is demonstrated by paying attention to the pitfall of adjusting for sequelae. The proper analysis of effects over time should be driven by a clear research question. Both kinds of research questions, that is those of time-dependent effects as well those of time-dependent risk factors, can be analyzed with time-dependent Cox regression analysis. It will be shown that using time-dependent risk factors usually implies focusing on short-term effects only.
10.1038/ki.2008.328
Foster Care Reentry: A survival analysis assessing differences across permanency type.
Goering Emily Smith,Shaw Terry V
Child abuse & neglect
Foster care reentry is an important factor for evaluating the overall success of permanency. Rates of reentry are typically only measured for 12-months and are often evaluated only for children who exit foster care to reunification and not across exit types, also known as 'permanency types'. This study examined the odds of reentry across multiple common permanency types for a cohort of 8107 children who achieved permanency between 2009 and 2013. Overall, 14% of children reentered care within 18-months with an average time to reentry of 6.36 months. A Kaplan-Meier survival analysis was used to assess differences in reentry across permanency types (including reunification, relative guardianship and non-relative guardianship). Children who achieved guardianship with kin had the lowest odds of reentry overall, followed by guardianship with non-kin, and reunification with family of origin. Children reunifying against the recommendations of Children and Family Services had the highest odds of reentry. A Cox regression survival analysis was conducted to assess odds of reentry across permanency type while controlling for demographics, services, and other risk factors. In the final model, only permanency type and cumulative risk were found to have a statistically significant impact on odds of reentry.
10.1016/j.chiabu.2017.03.005
A novel dynamic Bayesian network approach for data mining and survival data analysis.
BMC medical informatics and decision making
BACKGROUND:Censorship is the primary challenge in survival modeling, especially in human health studies. The classical methods have been limited by applications like Kaplan-Meier or restricted assumptions like the Cox regression model. On the other hand, Machine learning algorithms commonly rely on the high dimensionality of data and ignore the censorship attribute. In addition, these algorithms are more sophisticated to understand and utilize. We propose a novel approach based on the Bayesian network to address these issues. METHODS:We proposed a two-slice temporal Bayesian network model for the survival data, introducing the survival and censorship status in each observed time as the dynamic states. A score-based algorithm learned the structure of the directed acyclic graph. The likelihood approach conducted parameter learning. We conducted a simulation study to assess the performance of our model in comparison with the Kaplan-Meier and Cox proportional hazard regression. We defined various scenarios according to the sample size, censoring rate, and shapes of survival and censoring distributions across time. Finally, we fit the model on a real-world dataset that includes 760 post gastrectomy surgery due to gastric cancer. The validation of the model was explored using the hold-out technique based on the posterior classification error. Our survival model performance results were compared using the Kaplan-Meier and Cox proportional hazard models. RESULTS:The simulation study shows the superiority of DBN in bias reduction for many scenarios compared with Cox regression and Kaplan-Meier, especially in the late survival times. In the real-world data, the structure of the dynamic Bayesian network model satisfied the finding from Kaplan-Meier and Cox regression classical approaches. The posterior classification error found from the validation technique did not exceed 0.04, representing that our network predicted the state variables with more than 96% accuracy. CONCLUSIONS:Our proposed dynamic Bayesian network model could be used as a data mining technique in the context of survival data analysis. The advantages of this approach are feature selection ability, straightforward interpretation, handling of high-dimensional data, and few assumptions.
10.1186/s12911-022-02000-7
Squamous Cell Carcinoma With Bone Invasion: A Systematic Review and Pooled Survival Analysis.
Dermatologic surgery : official publication for American Society for Dermatologic Surgery [et al.]
BACKGROUND:Bone invasion has long been recognized as a poor prognostic indicator for cutaneous squamous cell carcinoma (SCC). Survival analyses of factors associated with SCC with bone invasion have not been published. OBJECTIVE:To analyze all published demographic, clinical, and treatment data for SCC with bone invasion and assess the impact of prognostic variables on disease progression, disease-specific death, and overall mortality. MATERIALS AND METHODS:A systematic review and pooled-survival analysis was performed using individual patient data from case reports. Progression-free survival (PFS), disease-specific survival (DSS), and overall survival (OS) were estimated by Kaplan-Meier analysis. RESULTS:The study included 76 cases of SCC with bone invasion from 49 publications. Recurrent tumors and nonsurgical treatment modality were predictors of disease progression in univariable analysis and tumors of the trunk, head, and neck were predictors of disease progression in multivariable analysis. At 5 years from bone invasion diagnosis, patients had a PFS, DSS, and OS rate of 66.7%, 71.7%, and 66.2%, respectively. CONCLUSION:Cases of SCC with bone invasion had poor DFS, DSS, and OS rates, with worse outcomes imparted to tumors of the trunk, head, and neck.
10.1097/DSS.0000000000003553
Dynamic prediction and analysis based on restricted mean survival time in survival analysis with nonproportional hazards.
Yang Zijing,Wu Hongji,Hou Yawen,Yuan Hao,Chen Zheng
Computer methods and programs in biomedicine
BACKGROUND AND OBJECTIVE:In the process of clinical diagnosis and treatment, the restricted mean survival time (RMST), which reflects the life expectancy of patients up to a specified time, can be used as an appropriate outcome measure. However, the RMST only calculates the mean survival time of patients within a period of time after the start of follow-up and may not accurately portray the change in a patient's life expectancy over time. METHODS:The life expectancy can be adjusted for the time the patient has already survived and defined as the conditional restricted mean survival time (cRMST). A dynamic RMST model based on the cRMST can be established by incorporating time-dependent covariates and covariates with time-varying effects. We analyzed data from a study of primary biliary cirrhosis (PBC) to illustrate the use of the dynamic RMST model, and a simulation study was designed to test the advantages of the proposed approach. The predictive performance was evaluated using the C-index and the prediction error. RESULTS:Considering both the example results and the simulation results, the proposed dynamic RMST model, which can explore the dynamic effects of prognostic factors on survival time, has better predictive performance than the RMST model. Three PBC patient examples were used to illustrate how the predicted cRMST changed at different prediction times during follow-up. CONCLUSIONS:The use of the dynamic RMST model based on the cRMST allows for the optimization of evidence-based decision-making by updating personalized dynamic life expectancy for patients.
10.1016/j.cmpb.2021.106155
Survival analysis using a 5-step stratified testing and amalgamation routine (5-STAR) in randomized clinical trials.
Mehrotra Devan V,Marceau West Rachel
Statistics in medicine
Randomized clinical trials are often designed to assess whether a test treatment prolongs survival relative to a control treatment. Increased patient heterogeneity, while desirable for generalizability of results, can weaken the ability of common statistical approaches to detect treatment differences, potentially hampering the regulatory approval of safe and efficacious therapies. A novel solution to this problem is proposed. A list of baseline covariates that have the potential to be prognostic for survival under either treatment is pre-specified in the analysis plan. At the analysis stage, using all observed survival times but blinded to patient-level treatment assignment, "noise" covariates are removed with elastic net Cox regression. The shortened covariate list is used by a conditional inference tree algorithm to segment the heterogeneous trial population into subpopulations of prognostically homogeneous patients (risk strata). After patient-level treatment unblinding, a treatment comparison is done within each formed risk stratum and stratum-level results are combined for overall statistical inference. The impressive power-boosting performance of our proposed 5-step stratified testing and amalgamation routine (5-STAR), relative to that of the logrank test and other common approaches that do not leverage inherently structured patient heterogeneity, is illustrated using a hypothetical and two real datasets along with simulation results. Furthermore, the importance of reporting stratum-level comparative treatment effects (time ratios from accelerated failure time model fits in conjunction with model averaging and, as needed, hazard ratios from Cox proportional hazard model fits) is highlighted as a potential enabler of personalized medicine. An R package is available at https://github.com/rmarceauwest/fiveSTAR.
10.1002/sim.8750
Prognostic and Predictive Impact of Circulating Tumor DNA in Patients with Advanced Cancers Treated with Immune Checkpoint Blockade.
Cancer discovery
The utility of circulating tumor DNA (ctDNA) as a biomarker in patients with advanced cancers receiving immunotherapy is uncertain. We therefore analyzed pretreatment ( = 978) and on-treatment ( = 171) ctDNA samples across 16 advanced-stage tumor types from three phase I/II trials of durvalumab (± the anti-CTLA4 therapy tremelimumab). Higher pretreatment variant allele frequencies (VAF) were associated with poorer overall survival (OS) and other known prognostic factors, but not objective response, suggesting a prognostic role for patient outcomes. On-treatment reductions in VAF and lower on-treatment VAF were independently associated with longer progression-free survival and OS and increased objective response rate, but not prognostic variables, suggesting that on-treatment ctDNA dynamics are predictive of benefit from immune checkpoint blockade. Accordingly, we propose a concept of "molecular response" using ctDNA, incorporating both pretreatment and on-treatment VAF, that predicted long-term survival similarly to initial radiologic response while also permitting early differentiation of responders among patients with initially radiologically stable disease. SIGNIFICANCE: In a pan-cancer analysis of immune checkpoint blockade, pretreatment ctDNA levels appeared prognostic and on-treatment dynamics predictive. A "molecular response" metric identified long-term responders and adjudicated benefit among patients with initially radiologically stable disease. Changes in ctDNA may be more dynamic than radiographic changes and could complement existing trial endpoints..
10.1158/2159-8290.CD-20-0047
Association of Systemic Inflammation and Sarcopenia With Survival in Nonmetastatic Colorectal Cancer: Results From the C SCANS Study.
Feliciano Elizabeth M Cespedes,Kroenke Candyce H,Meyerhardt Jeffrey A,Prado Carla M,Bradshaw Patrick T,Kwan Marilyn L,Xiao Jingjie,Alexeeff Stacey,Corley Douglas,Weltzien Erin,Castillo Adrienne L,Caan Bette J
JAMA oncology
Importance:Systemic inflammation and sarcopenia are easily evaluated, predict mortality in many cancers, and are potentially modifiable. The combination of inflammation and sarcopenia may be able to identify patients with early-stage colorectal cancer (CRC) with poor prognosis. Objective:To examine associations of prediagnostic systemic inflammation with at-diagnosis sarcopenia, and determine whether these factors interact to predict CRC survival, adjusting for age, ethnicity, sex, body mass index, stage, and cancer site. Design, Setting, and Participants:A prospective cohort of 2470 Kaiser Permanente patients with stage I to III CRC diagnosed from 2006 through 2011. Exposures:Our primary measure of inflammation was the neutrophil to lymphocyte ratio (NLR). We averaged NLR in the 24 months before diagnosis (mean count = 3 measures; mean time before diagnosis = 7 mo). The reference group was NLR of less than 3, indicating low or no inflammation. Main Outcomes and Measures:Using computed tomography scans, we calculated skeletal muscle index (muscle area at the third lumbar vertebra divided by squared height). Sarcopenia was defined as less than 52 cm2/m2 and less than 38 cm2/m2 for normal or overweight men and women, respectively, and less than 54 cm2/m2 and less than 47 cm2/m2 for obese men and women, respectively. The main outcome was death (overall or CRC related). Results:Among 2470 patients, 1219 (49%) were female; mean (SD) age was 63 (12) years. An NLR of 3 or greater and sarcopenia were common (1133 [46%] and 1078 [44%], respectively). Over a median of 6 years of follow-up, we observed 656 deaths, 357 from CRC. Increasing NLR was associated with sarcopenia in a dose-response manner (compared with NLR < 3, odds ratio, 1.35; 95% CI, 1.10-1.67 for NLR 3 to <5; 1.47; 95% CI, 1.16-1.85 for NLR ≥ 5; P for trend < .001). An NLR of 3 or greater and sarcopenia independently predicted overall (hazard ratio [HR], 1.64; 95% CI, 1.40-1.91 and HR, 1.28; 95% CI, 1.10-1.53, respectively) and CRC-related death (HR, 1.71; 95% CI, 1.39-2.12 and HR, 1.42; 95% CI, 1.13-1.78, respectively). Patients with both sarcopenia and NLR of 3 or greater (vs neither) had double the risk of death, overall (HR, 2.12; 95% CI, 1.70-2.65) and CRC related (HR, 2.43; 95% CI, 1.79-3.29). Conclusions and Relevance:Prediagnosis inflammation was associated with at-diagnosis sarcopenia. Sarcopenia combined with inflammation nearly doubled risk of death, suggesting that these commonly collected biomarkers could enhance prognostication. A better understanding of how the host inflammatory/immune response influences changes in skeletal muscle may open new therapeutic avenues to improve cancer outcomes.
10.1001/jamaoncol.2017.2319
Proportion and number of cancer cases and deaths attributable to potentially modifiable risk factors in the United States.
CA: a cancer journal for clinicians
Contemporary information on the fraction of cancers that potentially could be prevented is useful for priority setting in cancer prevention and control. Herein, the authors estimate the proportion and number of invasive cancer cases and deaths, overall (excluding nonmelanoma skin cancers) and for 26 cancer types, in adults aged 30 years and older in the United States in 2014, that were attributable to major, potentially modifiable exposures (cigarette smoking; secondhand smoke; excess body weight; alcohol intake; consumption of red and processed meat; low consumption of fruits/vegetables, dietary fiber, and dietary calcium; physical inactivity; ultraviolet radiation; and 6 cancer-associated infections). The numbers of cancer cases were obtained from the Centers for Disease Control and Prevention (CDC) and the National Cancer Institute; the numbers of deaths were obtained from the CDC; risk factor prevalence estimates were obtained from nationally representative surveys; and associated relative risks of cancer were obtained from published, large-scale pooled analyses or meta-analyses. In the United States in 2014, an estimated 42.0% of all incident cancers (659,640 of 1570,975 cancers, excluding nonmelanoma skin cancers) and 45.1% of cancer deaths (265,150 of 587,521 deaths) were attributable to evaluated risk factors. Cigarette smoking accounted for the highest proportion of cancer cases (19.0%; 298,970 cases) and deaths (28.8%; 169,180 deaths), followed by excess body weight (7.8% and 6.5%, respectively) and alcohol intake (5.6% and 4.0%, respectively). Lung cancer had the highest number of cancers (184,970 cases) and deaths (132,960 deaths) attributable to evaluated risk factors, followed by colorectal cancer (76,910 cases and 28,290 deaths). These results, however, may underestimate the overall proportion of cancers attributable to modifiable factors, because the impact of all established risk factors could not be quantified, and many likely modifiable risk factors are not yet firmly established as causal. Nevertheless, these findings underscore the vast potential for reducing cancer morbidity and mortality through broad and equitable implementation of known preventive measures. CA Cancer J Clin 2018;68:31-54. © 2017 American Cancer Society.
10.3322/caac.21440
SurvBenchmark: comprehensive benchmarking study of survival analysis methods using both omics data and clinical data.
GigaScience
Survival analysis is a branch of statistics that deals with both the tracking of time and the survival status simultaneously as the dependent response. Current comparisons of survival model performance mostly center on clinical data with classic statistical survival models, with prediction accuracy often serving as the sole metric of model performance. Moreover, survival analysis approaches for censored omics data have not been thoroughly investigated. The common approach is to binarize the survival time and perform a classification analysis. Here, we develop a benchmarking design, SurvBenchmark, that evaluates a diverse collection of survival models for both clinical and omics data sets. SurvBenchmark not only focuses on classical approaches such as the Cox model but also evaluates state-of-the-art machine learning survival models. All approaches were assessed using multiple performance metrics; these include model predictability, stability, flexibility, and computational issues. Our systematic comparison design with 320 comparisons (20 methods over 16 data sets) shows that the performances of survival models vary in practice over real-world data sets and over the choice of the evaluation metric. In particular, we highlight that using multiple performance metrics is critical in providing a balanced assessment of various models. The results in our study will provide practical guidelines for translational scientists and clinicians, as well as define possible areas of investigation in both survival technique and benchmarking strategies.
10.1093/gigascience/giac071
Survival analysis of cancer patients in Portugal following the reference centre model implementation.
The European journal of health economics : HEPAC : health economics in prevention and care
Cancer has affected around eighteen million people all over the world in 2018. In Portugal, cancer was diagnosed in sixty thousand individuals during 2018, being the second leading cause of death (one in every four deaths). Following the European Directive 2011/24/EU, the Portuguese Health System has been recognizing oncology Reference Centres (RCs), which are focused on delivering best-in-class treatment for cancer patients. This paper performs a survival analysis of cancer patients in Portugal, having hospital episodes with discharge date after the official recognition, in 2016, of the first RCs for hepatobiliary, pancreatic, sarcomas and oesophageal cancer. The aim is to assess the impact of RCs on the survival probability of these patients. For each cancer type, survival curves are estimated using the Kaplan-Meier methodology, and hazard ratios are estimated for different covariates, using multivariate Extended Cox models. The results obtained support the implementation and encourage the further extension of the RC model for oncology in Portugal, as cancer patients treated in an oncology RC, overall, have a better survival probability when compared to patients who had no episode in an RC. These results are clearer for hepatobiliary and pancreatic cancer, but also visible for sarcomas and oesophageal cancer.
10.1007/s10198-022-01461-x
Survival Analysis of Treatment Efficacy in Comparative Coronavirus Disease 2019 Studies.
Clinical infectious diseases : an official publication of the Infectious Diseases Society of America
For survival analysis in comparative coronavirus disease 2019 trials, the routinely used hazard ratio may not provide a meaningful summary of the treatment effect. The mean survival time difference/ratio is an intuitive, assumption-free alternative. However, for short-term studies, landmark mortality rate differences/ratios are more clinically relevant and should be formally analyzed and reported.
10.1093/cid/ciaa1563
Survival analysis with change-points in covariate effects.
Lee Chun Yin,Lam K F
Statistical methods in medical research
We apply a maximal likelihood ratio test for the presence of multiple change-points in the covariate effects based on the Cox regression model. The covariate effect is assumed to change smoothly at one or more unknown change-points. The number of change-points is inferred by a sequential approach. Confidence intervals for the regression and change-point parameters are constructed by a bootstrap method based on Bernstein polynomials conditionally on the number of change-points. The methods are assessed by simulations and are applied to two datasets.
10.1177/0962280220922258
Focus on Survival Analysis for Eye Research.
McGuinness Myra B,Kasza Jessica,Wu Zhichao,Guymer Robyn H
Investigative ophthalmology & visual science
Analysis of time-to-event data, otherwise known as survival analysis, is a common investigative tool in ophthalmic research. For example, time-to-event data is useful when researchers are interested in investigating how long it takes for an ocular condition to worsen or whether treatment can delay the development of a potentially vision-threatening complication. Its implementation requires a different set of statistical tools compared to those required for analyses of other continuous and categorial outcomes. In this installment of the Focus on Data series, we present an overview of selected concepts relating to analysis of time-to-event data in eye research. We introduce censoring, model selection, consideration of model assumptions, and best practice for reporting. We also consider challenges that commonly arise when analyzing time-to-event data in ophthalmic research, including collection of data from two eyes per person and the presence of multiple outcomes of interest. The concepts are illustrated using data from the Laser Intervention in Early Stages of Age-Related Macular Degeneration study and statistical computing code for Stata is provided to demonstrate the application of the statistical methods to illustrative data.
10.1167/iovs.62.6.7
Period analysis for 'up-to-date' cancer survival data: theory, empirical evaluation, computational realisation and applications.
Brenner H,Gefeller O,Hakulinen T
European journal of cancer (Oxford, England : 1990)
Long-term survival rates are the most commonly used outcome measures for patients with cancer. However, traditional long-term survival statistics, which are derived by cohort-based types of analysis, essentially reflect the survival expectations of patients diagnosed many years ago. They are therefore often severely outdated at the time they become available. A couple of years ago, a new method of survival analysis, denoted period analysis, has been introduced to derive more 'up-to-date' estimates of long-term survival rates. We give a comprehensive review of the new methodology, its statistical background, empirical evaluation, computational realisation and applications. We conclude that period analysis is a powerful tool to provide more 'up-to-date' cancer survival rates. More widespread use by cancer registries should help to increase the use of cancer survival statistics for patients, clinicians, and public health authorities.
10.1016/j.ejca.2003.10.013
Applying mixture cure survival modeling to medication persistence analysis.
Pharmacoepidemiology and drug safety
PURPOSE:Standard survival models are often used in a medication persistence analysis. These methods implicitly assume that all patients will experience the event (medication discontinuation), which may bias the estimation of persistence if long-term medication persistent patients rate is expected in the population. We aimed to introduce a mixture cure model in the medication persistence analysis to describe the characteristics of long-term and short-term persistent patients, and demonstrate its application using a real-world data analysis. METHODS:A cohort of new users of statins was used to demonstrate the differences between the standard survival model and the mixture cure model in the medication persistence analysis. The mixture cure model estimated effects of variables, reported as odds ratios (OR) associated with likelihood of being long-term persistent and effects of variables, reported as hazard ratios (HR) associated with time to medication discontinuation among short-term persistent patients. RESULTS:Long-term persistent rate was estimated as 17% for statin users aged between 45 and 55 versus 10% for age less than 45 versus 4% for age greater than 55 via the mixture cure model. The HR of covariates estimated by the standard survival model (HR = 1.41, 95% CI = [1.35, 1.48]) were higher than those estimated by the mixture cure model (HR = 1.32, 95% CI = [1.25, 1.39]) when comparing patients with age greater than 55 to those between 45 and 55. CONCLUSIONS:Compared with standard survival modeling, a mixture cure model can improve the estimation of medication persistence when long-term persistent patients are expected in the population.
10.1002/pds.5441
Survival Analysis with High-Dimensional Omics Data Using a Threshold Gradient Descent Regularization-Based Neural Network Approach.
Genes
Analysis of data with a censored survival response and high-dimensional omics measurements is now common. Most of the existing analyses are based on specific (semi)parametric models, in particular the Cox model. Such analyses may be limited by not having sufficient flexibility, for example, in accommodating nonlinearity. For categorical and continuous responses, neural networks (NNs) have provided a highly competitive alternative. Comparatively, NNs for censored survival data remain limited. Omics measurements are usually high-dimensional, and only a small subset is expected to be survival-associated. As such, regularized estimation and selection are needed. In the existing NN studies, this is usually achieved via penalization. In this article, we propose adopting the threshold gradient descent regularization (TGDR) technique, which has competitive performance (for example, when compared to penalization) and unique advantages in regression analysis, but has not been adopted with NNs. The TGDR-based NN has a highly sensible formulation and an architecture different from the unregularized and penalization-based ones. Simulations show its satisfactory performance. Its practical effectiveness is further established via the analysis of two cancer omics datasets. Overall, this study can provide a practical and useful new way in the NN paradigm for survival analysis with high-dimensional omics measurements.
10.3390/genes13091674
Systematic Review of Survival Analysis in Leprosy Studies-Including the Following Outcomes: Relapse, Impairment of Nerve Function, Reactions and Physical Disability.
International journal of environmental research and public health
Leprosy is a public health problem in South American, African and Oceanian countries. National programs need to be evaluated, and the survival analysis model can aid in the construction of new indicators. The aim of this study was to assess the period of time until the outcomes of interest for patients with or exposed to leprosy by means of survival analysis surveys. This review researched articles using the databases of PubMed, Science Direct, Scopus, Scielo and BVS published in English and Portuguese. Twenty-eight articles from Brazil, India, Bangladesh, the Philippines and Indonesia were included. The Kaplan-Meier method, which derives the log-rank test, and Cox's proportional hazards regression, which obtains the hazard ratio, were applied. The mean follow-up until the following outcomes were: (I) leprosy (2.3 years) in the population who were exposed to it, (II) relapse (5.9 years), (III) clinical manifestations before, during and after treatment-nerve function impairment (5.2 years), leprosy reactions (4.9 years) and physical disability (8.3 years) in the population of patients with leprosy. Therefore, the use of survival analysis will enable the evaluation of national leprosy programs and assist in the decision-making process to face public health problems.
10.3390/ijerph191912155